
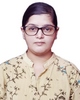
- Open Access
- Authors : Rahat Zehra , Dr. Madhulika Singh , Dr. Jyoti Verma
- Paper ID : IJERTV9IS100096
- Volume & Issue : Volume 09, Issue 10 (October 2020)
- Published (First Online): 13-10-2020
- ISSN (Online) : 2278-0181
- Publisher Name : IJERT
- License:
This work is licensed under a Creative Commons Attribution 4.0 International License
Study of Land use Land Cover Change Detection in Paonta Sahib Tehsil of Himachal Pradesh
Rahat Zehra
*Research Scholar, Amity Institute of Geo Informatics and Remote Sensing,
Amity University, Noida, U.P.
Dr. Madhulika Singp Advisor, Amity Institute of Geo Informatics and Remote Sensing, Amity University, Noida, U.P.
Dr. Jyoti Verma2 Assistant Professor, University of Allahabad, Prayagraj, U.P.
Abstract– With the ever-increasing population the prerequisite for arable lands, infrastructural development; resource management becomes an obligation to encourage optimization of resources and policy implementation. For this study a land- use change detection was attempted for the first time on the novel area of Paonta Sahib Tehsil, Sirmaur district (Himachal Pradesh) for the years 2014 and 2019. The classification was achieved through the USGS Land use Classification Scheme at Level I classes viz. Agricultural Land, Built-up, Forest, Waterbody, and Barren using a supervised classification technique. Ensuing, a positive change of +0.79% in Agriculture land and Waterbody with+0.17% was observed. Nevertheless, a negative change in Forest, Barren, and Built-up classes with
-0.14%,-0.54%, and -0.03% respectively. The overall accuracy for 2014 and 2019 was 0.81 and the Kappa coefficient for the same years was maintained at 0.76.
Keywords: Paonta Sahib Tehsil, Land Use Land Cover(LULC), Land-Use Change Detection, Maximum Likelihood classifier, Supervised Classification.
-
INTRODUCTION
In todays scenario, only a few areas are untouched by the mankind. They have modified the land cover as and when time commanded. The term land cover is the naturally occurring earth i.e. the physical form whereas the land use is the alterations done on. Often the course of alteration, degradation, and segmentation of the natural features leads to an endangered ecological system. The land use of an area correspondingly varies with natural factors like episodes of climate changes, flooding, and landfalls, etc. where the changes are evident spatially and temporally. Here, land use encompasses the presence of forest cover, river body, settlements, agricultural fields, and barren areas. The development in the urban infrastructure of the region has attracted numerous large and small scale industries. Distinguished sectors of power, mining, limestone, pharmaceuticals, dairy and food processing, etc. are found scattered in the study area. The regions lush green forest covers; picturesque hill and valley delicacy with some indigenous flora and fauna species is apt for recreational activities. With the constant surge in demand for food grain production, the region has a significant share of productive agricultural lands leading to various perishable goods manufacturing industries in the area. Along with water resources being utilized for domestic and agricultural purposes the region has a Giri hydel project for power necessities. Mapping the intensity of deviations on a Spatio- temporal scale becomes reliable and economically cheap with the advent of satellite remote sensing techniques as
mentioned by Kachhwala in 1985. Further, as Chilar in 2000 stated the association with GIS platform aids for data visualization, analysis, update, and retrieval. Plentiful studies have recognized the significance of preprocessing techniques of remote sensing in accomplishing precise and dependable results of change detection analysis (Jensen, 2005; Lu et al., 2004; Scheidt et al., 2008). Likewise, Daniel et al. in 2002 had worked upon the comparison of various techniques of land use land cover change detection wherein he used traditional post-classification cross-tabulation, cross-correlation analysis, neural networks, knowledge- based expert systems, and image segmentation and object- oriented classification.
Yet another work by Amin et al. in 2012 studied LULC mapping of Srinagar city; Kashmir Valley. Mehta et al. in 2012 had presented an integrated approach of remote sensing and GIS for LULC study of the arid environment of the Kutch region in Gujarat between the years 1999 and 2009. Sharma et al. in 2012 introduced Land Consumption Rate (LCR) and Land Absorption Coefficient (LAC) to help in the quantitative assessment of changes between the years 1976 and 2008 in Bhagalpur city; Bihar. (Rawat & Kumar, 2015). As quantified by Cheolmin Kim in 2016; consistent monitoring of LULC is critical for understanding the impact of anthropological and natural changes on Earth at spatiotemporal scales. Henceforth, LULC information is indispensable for planning and enactment of welfare schemes by monitoring the fluctuating land use pattern that in turn affects the radiation and water budget; natural emissions, and biodiversity.
For this study, satellite data of Landsat 8 OLI was acquired from USGS Earth Explorer for the years 2014 and 2019. After preprocessing the data; a hybrid classification approach involving supervised and unsupervised classification techniques were used. Ground truth verification using Garmin eTrex GPS was performed for higher accuracy. The land use studies describe the present use of land and help in predicting future trends for the same. To achieve this objective Maximum likelihood classifier was used.
-
MATERIALS AND METHODS
The study area shown in fig 1 the Paonta Sahib Tehsil is one of the 13 tehsils in the Sirmaur district of Himachal Pradesh lying in the Shiwalik range of Himalayan mountain system with humid subtropical climate prevailing throughout the year. Landsat 8 OLI data set was acquired from USGS Earth Explorer (http://earthexplorer.usgs.gov/)
for the years 2014 and 2019 with the spatial resolution of 30 mt. The image preprocessing was performed in the ERDAS Imagine version 2014 to obtain False Colour Composite. With the layers stacked; the subset was performed to extract the Area of Interest. Land use of the area under study was classified on USGS Land use Classification Scheme incorporated by National Remote Sensing Center, India; into Level I classes namely Agricultural Land, Built-up, Forest, Waterbody, and Barren using the supervised classification technique with maximum likelihood algorithm in ERDAS Imagine. The land classes of interest were carefully nominated to classify the remote data in the surveyed area. Cheolmin Kim in 2016 had mentioned the inevitability of a classification scheme that has taxonomically clear definitions of classes. The classes must be mutually exclusive, comprehensive, and hierarchical (Jensen2005).
For the unsure areas, the ground verification was performed; based on which the re-coding was done to correct the misclassified areas. The classification is incomplete without accuracy assessment. Thus, confusion matrix was generated for the same that contained information about actual and
predicted classifications done by a classification system. Cheolmin Kim in 2016 mentioned that to perform accuracy evaluation, Kappa Coefficient, and overall classification accuracy is the minimum requirements to be calculated from the confusion matrix. The kappa analysis is a standard procedure of evaluating a single confusion matrix that would specify the probability of correct classification by discarding the probability of accidentally correct classification. The kappa coefficient considers the overall statistical agreement of a confusion matrix for calculation of overall classification accuracy wherein the total number of correctly classified pixels is divided by the total number of sample points. Further, Land use land cover change detection was performed using the post-classification detection techniqe. The pixel-based comparison was performed to generate the change information on a pixel basis. The changes were noted with the -from and to conversion table to obtain the qualitative and quantitative datasets of the period.
Fig. 1. Location map of the study area Paonta Sahib Tehsil with subset of Sirmaur district and Himachal Pradesh.
-
RESULTS AND DISCUSSION
Results obtained from the satellite imageries were diagrammatically represented in Fig 2 and 3 and numerically in Table1. Fig 2 displays Land Use Land Cover in 2014 and Fig 3 shows the Land Use Land Cover of 2019. Further Fig 4 depicts the Transition of Land Use Land Cover from 2014 to 2019. From an analysis of the images; as summarized in Table1 it was noted that in 2014 Forest was the eminent Land Use category with a share of 51.31% (392 sq.km ) area followed by Agricultural land at 21.86% (167 sq.km) area
and Barren with 18.06%(138 sq. km) area. The Built-Up had a share of 7.98 %( 61 sq.km) and Waterbody was 0.79% (6sq.km) area. In 2019; it was observed that forest was still the prominent Land Use category with a share of 43.98% (336 sq.km ) area followed by Agriculture land at 39.14% (299sq.km) area and Barren with 8.25%(63 sq. km) area. The Built-Up had a share of 7.72 %( 59 sq.km) and Waterbody was 0.92% (7sq.km) area. Data described in Table2 divulge both a positive and negative change in the study area over the years. There was a positive increase of
+ 0.79% (132 sq. km) area in Agriculture land and +0.17%
(1 sq. km) area of the Waterbody. Though, a negative change of -0.54% (75 sq.km) area of Barren, -0.14 %( 56 sq. km) area in the Forest and -0.03 % (2 sq.km) area in Built- Up was observed.
To summarize the infringement of Land Use Land Cover classes during the years a change detection matrix as in Table 2 was generated which exposes that;
-
About 28.97% area of Agricultural land has been converted into the Forest and 22.82% into Barren.
-
Around 45.03% of the Built-Up area was converted into Agricultural land and 17.01% was left as Barren with the Forest being 7.13%.
-
Further, a share of 5.55% area underneath Forest was converted into Built-Up and 3.35% into Agricultural land.
-
Nearly 9.53% area of the Barren segment was utilized for Agriculture practices and 4.82% was reclaimed as Built-Up.
-
The 44.77% of the total area occupied by Waterbodies was converted to Barren and 14.94% was used up for Agriculture.
The precision of the classification technique was supported by the overall accuracy for both the years 2014 and 2019 as 0.81 with the Kappa coefficient for the same years being 0.76; in compliance with Rwanga, S.S., and Ndambuki,
-
(2017). The details of the Confusion matrix for the years have been listed in Tables 3 and 4
Fig. 2. Paonta Sahib Land Use Land Cover in 2014
Fig. 3. Paonta Sahib Land Use Land Cover in 2019
TABLE 1. AREA UNDER VARIOUS LAND USE LAND COVER CLASSES IN 2014 AND 2019 AND THEIR PERCENTAGE CHANGE.
LULC Class
2014
Percentage Area
2019
Percentage Area
Percentage Change
Area (in sq.km)
Area (in sq.km)
Area(in sq.km)
Percentage
Built Up
61
7.98
59
7.72
2
-0.03
Agriculture Land
167
21.86
299
39.14
-132
0.79
Forest
392
51.31
336
43.98
56
-0.14
Barren
138
18.06
63
8.25
75
-0.54
Waterbody
6
0.79
7
0.92
-1
0.17
Total
764
100.00
764
100.00
0
0
Fig. 4. Transition of Land Use Land Cover from 2014 to 2019
TABLE 2 INFRINGEMENT IN AREA UNDER VARIOUS LAND USE LAND COVER IN 2014-2019
Agriculture%
Built Up%
Forest%
Barren %
Waterbody %
Agriculture
40.73
45.03
3.35
9.53
14.94
Built Up
6.89
30.05
5.55
4.82
11.69
Forest
28.97
7.13
90.11
1.02
0.36
Barren
22.82
17.01
0.83
83.65
44.77
Waterbody
0.59
0.78
0.16
0.98
28.25
Total
100.00
100.00
100.00
100.00
100.00
TABLE 3 CONFUSION MATRIX FOR LAND USE LAND COVER IN 2014
LULC Classes
Built Up
Agriculture Land
Waterbody
Forest
Barren
Ground Truth Total
Built Up
63
5
2
4
6
80
Agriculture Land
10
56
6
1
7
80
Forest
0
4
0
46
0
50
Barren
13
5
0
3
69
90
Waterbody
0
0
68
0
2
70
Total Pixels
86
70
76
54
84
370
TABLE4 CONFUSION MATRIX FOR LAND USE LAND COVER IN 2019.
LULC Classes
Built Up
Agriculture Land
Waterbody
Forest
Barren
Ground Truth Total
Built Up
63
7
0
1
13
84
Agriculture Land
1
67
1
2
4
75
Waterbody
3
0
46
0
13
62
Forest
0
1
0
47
0
48
Barren
8
2
4
1
49
64
Total pixels
75
77
51
51
79
333
Transformations in the agricultural cropping pattern and diversification in the crops owing to rapid urbanization and industrialization; the study area has witnessed massive land- use changes specifically in the low lying areas towards the water bodies. As mentioned by Sharma et al in 2007 and Singh in 1997; the incorporation of the horticultural cash crops has led to a chnge in land use and cropping patterns with specialized types of machinery. This change along with ever-increasing population mass and unfortunate government policy measures have endangered forest sustainability; embellished deforestation and degradation. The impact is clear with enormous pharmaceutical industries developed in areas of Paonta Sahib City and Nahan converting them into the belt of industrial zones. Chowdhry in 2008 stated that the introduction of the Himachal Pradesh Nautor Land Rules in 1968 hampered the land use of the area wherein the government redistributed land up to one acre to suitable landless people for agriculture and horticulture practices. Gupta in 2007 cited the result of the practices of land distribution had exaggerated the deforestation in the state. Singh & Khanduri in 2011 had advocated that land- use study is of vital significance in determining the economic, cultural, and social development of man in the spatiotemporal extent. This study promotes the usage of satellite imageries which have an imperative role in quantifying the spatial and temporal spectacles which is difficult with conventional mapping techniques.
-
-
CONCLUSION
The land-use change imitates the significant impact of human actions on its natural resources and the environment. Scrutiny of the Spatio-temporal pattern of land use and the underlying factors give way forward to sustainable practices
government agencies with easier techniques of Remote Sensing and GIS generated spatial data.
ACKNOWLEDGEMENT
I, Rahat Zehra, would like to acknowledge and thank Dr. Madhulika Singh (Ph.D. Supervisor, Advisor, Amity Institute of Geo Informatics and Remote Sensing, Amity University, Noida, U.P) and Dr. Jyoti Verma (Co-Guide, University of Allahabad, Prayagraj, U.P) for their untiring efforts with the research work; by helping and guiding me in all aspects without worrying about their circumstances.
REFERENCES
-
Jensen, J.R., 2005. Digital change detection. Introductory Digital Image Processing, A Remote Sensing perspective. Pearson Prentice Hall, New York, pp. 467494.
-
Singh, P., & Khanduri, K. (2011). Land use and Land cover change detection through Remote Sensing & GIS Technology: Case study of Pathankot and Dhar Kalan Tehsils , Punjab. International Jorunal of Geomatics and Geosciences, 1(4), 839 846.
-
Vishwa, B., Kaur, B., & Simrit, K. (2013). Land use/cover change and its implications for Kullu district of Himachal Pradesh, India. International Journal of , 3(3). http://www.indianjournals.com/ijor.aspx?target=ijor:ijggs&vol ume=3&issue=3&article=013
-
Rawat, J. S., & Kumar, M. (2015). Monitoring land use/cover change using remote sensing and GIS techniques: A case study of Hawalbagh block, district Almora, Uttarakhand, India. Egyptian Journal of Remote Sensing and Space Science, 18(1), 7784. https://doi.org/10.1016/j.ejrs.2015.02.002
-
Shah, S., & Sharma, D. P. (2015). Land use change detection in Solan forest division, Himachal Pradesh, India. Forest Ecosystems, 2(1). https://doi.org/10.1186/s40663-015-0050-7
-
Sharda, S., Rishi, M., Brar, K. K., & Kaur, G. (2015). Comparative Detection of Land Use and Land Cover Using
and maintenance plans as suggested by Shah and Sharma in 2015. From the above discussion, it is apparent that there needs to be a critical analysis of the hilly areas of India. The conversion of forest into agriculture lands and built up has
-
Remote Sensing and Gis Tools. 5(6), 18.
Kim, C. (2016). Land use classification and land use change analysis using satellite images in Lombok Island, Indonesia. Forest Science and Technology, 12(4), 183191. https://doi.org/10.1080/21580103.2016.1147498
endangered the native species and unprecedented loss to the climate. There is an urgent need for proper government policies and planning to evade haphazard growth of industrial and residential built-up areas on grounds of forest area. With the overall accuracy of 0.81 and Kappa coefficient of 0.76, it is recommended by the team that future work on individual land use categories could be performed in detail to give a holistic new approach to the planners and
-
Rwanga, S. S., & Ndambuki, J. M. (2017). Accuracy
Assessment of Land Use/Land Cover Classification Using Remote Sensing and GIS. International Journal of Geosciences, 08(04), 611622. https://doi.org/10.4236/ijg.2017.84033
-
Nivedita Priyadarshini, K., Kumar, M., Rahaman, S. A., & Nitheshnirmal, S. (2018). a Comparative Study of Advanced Land Use/Land Cover Classification Algorithms Using Sentinel-2 Data. ISPRS – International Archives of the Photogrammetry, Remote Sensing and Spatial Information
Sciences, XLII5(November), 665670.
https://doi.org/10.5194/isprs-archives-xlii-5-665-2018
-
Vaidya, P., Bhardwaj, S. K., & Sood, S. (2018). Land use and land cover changes in Kullu valley of Himachal Pradesh. Indian Journal of Agricultural Sciences, 88(6), 902906.
-
Qadir, J., & Singh, P. (2019). Land use/cover mapping and assessing the impact of solid waste on water quality of Dal Lake catchment using remote sensing and GIS (Srinagar, India). SN Applied Sciences, 1(1). https://doi.org/10.1007/s42452-018- 0027-6
-
Navin, M. S., & Agilandeeswari, L. (2019). Land use Land Cover Change Detection using K-means Clustering and Maximum Likelihood Classification Method in the Javadi Hills, Tamil Nadu, India. International Journal of Engineering and Advanced Technology, 9(1S3), 5156.
https://doi.org/10.35940/ijeat.a1011.1291s319
-
Twisa, S., & Buchroithner, M. F. (2019). Land-use and land- cover (LULC) change detection in Wami river basin, Tanzania. Land, 8(9). https://doi.org/10.3390/land8090136