
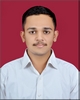
- Open Access
- Authors : Yash N. Jaiswal , Vikram R. Khanzode
- Paper ID : IJERTV9IS110254
- Volume & Issue : Volume 09, Issue 11 (November 2020)
- Published (First Online): 07-12-2020
- ISSN (Online) : 2278-0181
- Publisher Name : IJERT
- License:
This work is licensed under a Creative Commons Attribution 4.0 International License
Defect Rate Reduction in Biscuit Production Industry using SPC Technique
Yash N. Jaiswal
Department of Industrial Engineering
Shri Ramdeobaba College of Engineering and Management
Nagpur, India
Vikram R. Khanzode
Department of Industrial Engineering
Shri Ramdeobaba College of Engineering and Management
Nagpur, India
Abstract—Variability in a manufacturing process causes defects in the final products which in turn hampers quality, productivity, profitability, and ultimately, the customer satisfaction. The main objective of this study is to minimize the defect rate and variability in the final product, i.e. biscuit packages, by using Statistical Process Control (SPC) tools in a biscuit production unit thereby increasing its productivity, profitability and competitive advantage in the market. Several problems in production have been scrutinized by using SPC tools like Pareto analysis, Cause-and-effect diagrams, attribute control charts (p-charts) and process capability analysis. For the sake of this study, several types of defects from two main categories have been considered namely biscuit defects and packaging defects. On executing the Pareto analysis, three most contributing types of defects were found which when summed up constituted approximately 82% of the total defects. These defects are Breakage (41%), Blisters (26%) and Off registration (15%).
For the above-mentioned defects, cause-and-effect diagrams were constructed to pin point towards the possible root causes of those problems. On the basis of these root causes, certain improvements were recommended. Attribute control charts were plotted and process capability analysis was carried out for data of proportion of defect collected before and after the improvement recommendations were implemented. It was found that the process of production went from being erratically out of control to being well within the control limits after the improvement recommendations were implemented. Hence, the application of SPC tools in regularly monitoring the production processes for defects is extremely effective in improving the productivity and profitability of a production unit.
Keywords— Statistical Process Capability, Pareto Analysis, Cause-and-effect diagram, Process Capability, Control Charts)
I. INTRODUCTION
Quality is the degree to which consumer needs are met by a set of inherent characteristics of a good or service. It aims to improve an enterprise’s profitability. A rise in efficiency often leads to an increase in output and a decline in the rejection rate. In marketing plans, efficiency goals are typically used as a standard of product or service excellence. Product or service efficiency impacts both internal and external consumers alike.
Reducing the defect rate of finished products (i.e., biscuits & cookies) using Statistical Process Control (SPC) tools is the main objective of this research. Statistical process control (SPC) is a quality control methodology that uses statistical tools to measure and monitor the manufacturing process. Using lean and SPC methods, significant biscuit defects, origins, and techniques for fixing them have been scrutinized. To minimize process uncertainty, thus enhancing product quality, a biscuit manufacturing plant was chosen to conduct this analysis.
This research begins by defining all potential sources of defects in our finished goods and identifying the most regularly occurring ones. Then, suggestions for regulating these defects are given [1].
II. METHODOLOGY / DATA ANALYSIS
At the beginning of this study, data were gathered for each defect’s type and frequency with the product, i.e., biscuit packets. These defects can be classified into two main categories, namely biscuit defects and packaging defects. These two categories of defects can be further broken down as follows:
1. Biscuit defects: Breakage, blisters, cream oozing, hard bite, shrinkage, reverse shell, and biscuit spreading.
2. Packaging defects: Off registration, met layers, sealing defects, pinholes, underweight packages, overweight packages, and loose packaging.
These defect types include seven biscuit defects and seven packaging defects, 14 in all.
A. Data Collection
In the next step, the sampling of the product was done for six weeks. This allowed us to calculate the final product’s rejection rate due to each type of defect, as shown in Table I.
TABLE I. DATA COLLECTED FOR TYPES OF DEFECTS OVER SIX WEEKS
Type of
Week 1
Week 2
Week 3
Week 4
Week 5
Week 6
Total Defects
Breakage
31
25
35
28
35
33
187
Blisters
16
20
20
21
20
22
119
Off Registration
14
10
13
7
11
12
67
Cream oozing
3
1
3
5
3
7
22
Hard bite
0
3
4
1
1
1
10
Pin Holes/ cuts
3
1
0
1
1
1
7
Shrinkage
2
0
1
1
2
1
7
Overweight packets
2
1
1
0
2
0
6
Met layer
1
0
3
0
1
1
6
Sealing defects
2
1
1
1
0
1
6
Underweight Packets
1
0
1
1
1
1
5
Loose packaging
0
1
1
2
0
1
5
Reverse shell
0
0
1
1
1
1
4
Biscuit spreading
1
1
0
0
2
0
4
Total Number of defects
76
64
84
69
80
82
455
Number of items inspected
725
725
725
725
725
725
Total Production
58000
58000
58000
58000
58000
58000
B. Pareto Analysis
Based on the 80/20 theory, Pareto Analysis is a method used for corporate decision making. It is a technique of decision-making that statistically divides a small range of input variables, either beneficial or unfavorable, as having the greatest effect on an outcome. The Pareto analysis is based on the premise that 20% of the work will produce 80% of the gain of a project or, conversely, 80% of the issues are attributed to 20% of the causes.
A Pareto chart was designed based on data obtained in Table I, identifying the most common types of defects (Fig. 1). Figure 1 shows that there are three most frequently occurring types of defects.
These are Breakage, Blisters, and Off registration, which account for about 41%, 26%, and 15% of the total defects each, respectively. These three major types of defects sum up to be 81.98% of the real defects overall. Hence, for this study’s scope, the causes of the mentioned types of defects will be investigated.
Fig. 1 Pareto Chart of Type of defects
C. Cause and Effect Diagram
A cause-effect diagram or fishbone diagram is a schematic tool used for graphically showing them to analytically categorise potential causes for a certain form of defect, signifying a cause-and-effect correlation between hypotheses. Although the cause-and-effect graphs display multiple variables that need to be studied, the focus is on the most possible cause(s) that eventually leads to the rejection of the substance [3]. A cause-and-effect diagram for the three major forms of defects from Table I has been prepared for this review, namely Breakage, Blisters, and Off registration.
Breakage: System misalignment, incorrect baking, lack of care, lack of ergonomic considerations, lack of operator expertise, improper handling of equipment are the key causes of breakage defects (Fig. 2).
Blisters: Sluggish roller drive speed, high temperature, unnecessarily long baking process, lack of preventive maintenance are the major causes of blistering in the biscuits (Fig. 3).
Off Registration: The root causes of off registration defect in the packaging process are poor gear conditions, malfunctioning web page, incorrect tolerances in measuring instruments, inadequate operator training. (Fig. 4)
Fig. 2 Cause-and-effect diagram for Breakage defect
Fig. 3 Cause-and-effect diagram for Blistering defect
Fig. 4 Cause-and-effect diagram for Off Registration defect
D. Control Charts
Control charts, also known as process-behavior charts, are a statistical process control tool used to ascertain if a manufacturing or business process is in a state of control. These are a type of graphical tools for statistical process monitoring [4].
According to the nature of data collected, there are two main categories of control charts, i.e., variable control chart and attribute control chart. A variable control chart is plotted to control process parameters, the data of which is continuous in nature. On the other hand, an attribute control chart is used to plot out data that tends to classify or count observations. A typical example of quality characteristic classification would be classifying units as “defective” and “non-defective.” Attribute control charts are further organized into p charts, np charts, u charts, and c charts [4]. For this study, we used a p chart because p charts are used to plot data dealing with the proportion of defective products in a particular sample. We have collected data thirty-five samples of 100 units each (i.e., 35×100) for the initial assessment and presented them in Table II. For this data, we have used a software package called Minitab to plot out a p-chart, as shown in Fig. 5.
TABLE II. SAMPLING FOR THE PROPORTION OF DEFECTIVES BEFORE IMPROVEMENT
Sr. No.
Number of
Proportion
Sr. No.
Number of Defects
Proportion
1
11
0.11
18
13
0.13
2
29
0.29
19
8
0.08
3
12
0.12
20
16
0.16
4
13
0.13
21
35
0.35
5
22
0.22
22
8
0.08
6
12
0.12
23
25
0.25
7
19
0.19
24
10
0.1
8
15
0.15
25
16
0.16
9
5
0.05
26
30
0.3
10
17
0.17
27
14
0.14
11
14
0.14
28
32
0.32
12
13
0.13
29
7
0.07
13
16
0.16
30
11
0.11
14
21
0.21
31
34
0.34
15
18
0.18
32
10
0.1
16
11
0.11
33
9
0.09
17
4
0.04
34
23
0.23
35
4
0.04
Fig. 5 P-chart to illustrate the process condition before improvement suggestions
E. Process Capability
Process Capability: Process capability indicates how the process can produce items within the control limit. Process capability compares the output of an in-control process to the specification limits by calculating the ratio of the spread between the process specifications to the spread of the process values, as measured by six process standard deviation units, also known as the “process width” [6].
Process capability analysis has been done for the data presented in Table II using the Minitab toolkit (Fig. 6). Referring to Fig. 5, it is clear that the process is erratically out of control, with 7 data points falling out of the control limits.
Fig. 6 Process Capability Analysis before improvement suggestions
III. IMPROVEMENT SUGGESTIONS FOR ROOT CAUSES:
For combating such low process control witnessed in Fig. 5, specific improvements were proposed concerning the operator, the machine set-up, the material, and the environment. Table III shows suggestions and action plan for different causes:
TABLE III. ACTION PLAN AND SUGGESTIONS FOR ROOT CAUSE
Category
Symptom
Root Cause
Suggestion
Operator
Subpar quality produced
Often there is much carelessness among the operators with regards to quality
The personnel should be trained and motivated, and a positive attitude towards quality should be imparted to them. This will create an error-proof work culture.
Machine set-up
Overbaking
Improper speed and feed rate of the conveyor in the baking process.
The conveyor should be programmed correctly to get the correct conveyor feed rate.
Materials
An improper mixture of the materials
Erratic flow of water in the mixture
Use of moisture sensors in the process of mixing to sense extra moisture.
Environment
Under weighed or Overweighed packages
Inappropriate adjustment of the room temperature
Ensuring proper ventilation.
IV. COMPARISON BETWEEN INITIAL AND FINAL DATA
After incorporating the proposed suggestions for improvement, another dataset of thirty-five samples of 100 units each (i.e., 35×100) was collected for defective units (Table IV).
P chart was plotted based on data from Table IV (Fig. 7), which shows that the proportion of defects is within the control limits. These findings have been corroborated by the Process Capability Analysis of Table IV data (Fig. 8). Hence, it can be concluded that the process was in control.
TABLE IV SAMPLING FOR THE PROPORTION OF DEFECTIVES AFTER IMPROVEMENT
Sr. No.
Number of Defects
Proportion
Sr. No.
Number of Defects
Proportion
1
8
0.08
18
9
0.09
2
9
0.09
19
4
0.04
3
7
0.07
20
9
0.09
4
9
0.09
21
5
0.05
5
7
0.07
22
9
0.09
6
9
0.09
23
10
0.1
7
10
0.1
24
5
0.05
8
4
0.04
25
5
0.05
9
6
0.06
26
8
0.08
10
8
0.08
27
9
0.09
11
4
0.04
28
4
0.04
12
8
0.08
29
11
0.11
13
5
0.05
30
7
0.07
14
8
0.08
31
5
0.05
15
4
0.04
32
8
0.08
16
8
0.08
33
4
0.04
17
4
0.04
34
9
0.09
35
10
0.1
Fig. 7 P-chart to illustrate the process condition after improvement implementation
Fig. 8 Process Capability Analysis after improvement implementation
V. RESULTS
Finally, the sampling of the product was done for three weeks. This allowed us to calculate the final product’s rejection rate due to each type of defect, as shown in Table V.
To compare the data in Table I and Table V, which are six weeks data and three weeks data, respectively, we take the average number of defects of each type in Table I and Table V and compare them in Table VI. Percent changes between the two defect averages have also been recorded in Table VI.
TABLE V. DATA OF DEFECTS COLLECTED AFTER IMPROVEMENT OVER A SPAN OF THREE WEEKS
Type of defects
Week 1
Week 2
Week 3
Total defects
Breakage
15
14
16
45
Blisters
8
7
9
24
Off Registration
3
5
4
12
Cream oozing
1
0
2
3
Hard bite
0
2
2
4
Pin Holes/ cuts
1
0
0
1
Shrinkage
1
0
0
1
Overweight packets
0
1
1
2
Met layer
0
0
2
2
Sealing defects
1
0
1
2
Underweight Packets
0
0
0
0
Loose packaging
0
1
1
2
Reverse shell
0
0
0
0
Biscuit spreading
0
0
0
0
Total Number of defects
30
30
38
98
Number of items inspected
725
725
725
Total Production
58000
58000
58000
TABLE VI COMPARISON BETWEEN AVERAGE DEFECTS BEFORE AND AFTER THE STUDY
Type of defects
Average Before
Average After
% Decrease
Breakage
31.17
15.00
51.87
Blisters
19.83
8.00
59.66
Off Registration
11.17
4.00
64.18
Cream oozing
3.67
1.00
72.73
Hard bite
1.67
1.33
20.00
Pin Holes/ cuts
1.17
0.33
71.43
Shrinkage
1.17
0.33
71.43
Overweight packets
1.00
0.67
33.33
Met layer
1.00
0.67
33.33
Sealing defects
1.00
0.67
33.33
Underweight Packets
0.83
0.00
100.00
Loose packaging
0.83
0.67
20.00
Reverse shell
0.67
0.00
100.00
Biscuit spreading
0.67
0.00
100.00
Total
75.83
32.67
56.92
VI. CONCLUSION
From the detailed research, the following points can be concluded:
• The management and line staff of the plant should be properly qualified to use Statistical Quality Control (SQC) tools and software because quality is an important element in customer loyalty and the market’s competitive advantage.
• Appropriate ergonomic conditions should be established to allow the operators without getting fatigued and thereby committing fewer errors.
• To avoid blistering and breakage of the biscuits, the baking process must be carried out at a specific temperature..
• The factory’s management and line workers should be adequately trained to use Statistical Quality Control (SQC) tools.
• Quality management maps are an incredibly powerful mathematical tool to classify certain performance issues or adjustments due to external factors..
• In conclusion, the management should aspire to reduce the defect rate of final products, i.e., packaged biscuits, through Statistical Process Control (SPC) tools. SPC tools are incredibly useful in improving process performance by reducing process variability and improving production efficiency by lowering rejections and rework.
REFERENCES
[1] Harpreet Singh Oberoi, Mamta Parmar, Harpreet Kaur, Rahul Mehr, “SPC (Statistical Process Control): A Quality Control Technique for Confirmation to Ability of process,” International Research Journal of Engineering and Technology (IRJET), ISSN:2395-0072, Vol. 03, Issue: 06. [2] E.L. Grant and R.S. Leavenworth, Statistical quality control (4th ed., McGraw-Hill Co., New York, 1972). [3] Pranay S. Parmar, Vivek A. Deshpande, “Implementation of Statistical Process Control Techniques in Industry: A Review, “International Journal of Emerging Technologies and Innovative Research, ISSN:2349-5162, Vol.1, Issue 6, page no. pp583-587. [4] S. Bisgaard, Statistical Tools for Manufacturing, Manufacturing Review, 6(3), 1993, 192-200. [5] Sahil Sardana, Rajender Kumar, Manjinder Bajwa, Piyush Gulati, Application of SPC Tool For Finding Variation In The Process Output A Case Study. International Journal of Industrial Engineering Research and Development, 2(1), 2011, pp. 46–58. [6] M. Suozzi, 1999, Process capability study, Internal Document, Member of Technical Staff, Hughes Aircraft Company, Tucson, Arizona, 1-16, Nov. 27, 1990. [7] Ravi Terkar, Dr. Hari Vasudevan, and Dr. Vilas Kalamkar, Remanufacturing For Profitability and Green Growth Sustainability: A Case Study of Printer Cartridge Sector in India, International Journal of Production Technology and Management (IJPTM), 4(1), 2013, pp. 17–30. [8] D.H. Stamatis, Six sigma fundamentals (Productivity Press, New York, 2004). [9] W.A. Shewhart, Economic Control of the manufactured product’s quality (D.Van Nostrand, Co., New York, 1931). [10] Mahesh B.P.; Prabhuswamy M.S. (2010) “Process variability reduction through Statistical Process Control For quality improvement”; International Journal for Quality Short Scientific Paper”; Vol.4; No. 3; 2010. [11] Bimal and Dangayach G.S. (2009); “Performance improvement through statistical process control: a longitudinal study”; “International Journal of Globalisation and Small Business”; Vol 3; pp 55-72