
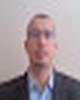
- Open Access
- Authors : Mbarek El Bounjimi , Georges Abdul-Nour
- Paper ID : IJERTV10IS100149
- Volume & Issue : Volume 10, Issue 10 (October 2021)
- Published (First Online): 03-11-2021
- ISSN (Online) : 2278-0181
- Publisher Name : IJERT
- License:
This work is licensed under a Creative Commons Attribution 4.0 International License
Smart Asset Management in Power Industry: A Review of The Key Technologies
Mbarek El Bounjimi , Georges Abdul-Nour
1 Université de Québec à Trois-Rivières, Institut de recherche sur les PME 3351 Bd des Forges, Trois-Rivières, QC G8Z 4M3, Canada
Abstract:- Power companies are asset-intensive and facing the challenge of keeping their assets reliable as long as possible while reducing costs and risks. With the digital transformation Industry 4.0, Asset management (AM) today is smarter and data- driven than before. Smart Asset Management (SAM) emerges as a data-driven and integrated ecosystem that collect and analyze assets data to enable informed decision-making and higher asset performance. This article reviews the key enabling technologies and solutions of SAM as well as the future research avenues.
Keywords Asset management, Digital Technologies, Industry 4.0, Power industry, Review
-
INTRODUCTION
Asset management (AM) is a key function in asset-intensive companies. In electric power industry, AM represents 20 to 30% operational expenses and 15-20% of capital investment expenses (replacement) (McKinsey,2018). Toronto Hydro Corporation, for example, estimated that about 30% of its power distribution assets have already exceeded their theoretical useful life (AÉC, 2015).
The main objectives of AM are to optimize the life cycle of assets, improve preventive maintenance and prepare an effective plan for investing in new assets. The ability to develop optimal investment strategies to achieve long-term performance goals with a low level of risk is crucial in power industry. To be successful, organizations must find powerful tools that can handle big data from assets.
Today, power companies can manage their assets more efficiently thanks to technological advancements. Sensors, communication devices, and other hardware to track and control objects remotely have become increasingly affordable and reliable. International experience shows that the digital transformation of power systems results in lower power losses, higher reliability and creates a number of new services to customers. Digitalization opens up new possibilities for power companies, such as artificial intelligence (AI), a branch of computing that simulates smart human behavior on computers in the form of software applications. AI also incorporates machine learning: applications accumulate knowledge from experience and use it to predict plausible scenarios. AI is crucial for managing and optimizing the increasingly complex infrastructure of power generation, grids, homes, and any connected devices. This technology helps make the grid smarter and allows power companies to successfully manage their assets and operations.
These changes allow companies to move away from the silo- based asset management to cross-functional management. At the same time, from traditional planning of maintenance based
heavily on knowledge and experience towards a data-driven planning approach.
Smart asset management model based on big data analytics and AI improves operational performance in a number of ways, which companies should prioritize based on regulatory requirements, asset criticality, and other factors. Implementation of Smart Asset Management (SAM) solutions can reduce utilities capital and operations and maintenance (O&M) spend by 20 percent or more (Belmont et al 2020). In its recent benchmarking about power utility performance, McKinsey 2020, confirm this finding and reported that an Asian power utility transformed its asset management, capturing savings of 20 percent in maintenance Opex and replacement Capex while maintaining superior service quality. Unfortunately, less than 5% of power companies have implemented a smart asset management to help them optimizing maintenance, operations and asset investment decisions (Deloitte,2019).
In this sense, the objective of this paper is to explore the adoption of SAM solutions in power industry. The remainder of this paper is organized as follows: the following section presents the research methodology. Then, we review the SAM in power industry context, followed by the SAM key enabling technologies and the literature reviews finding. Finally, in Section 5, conclusions and main findings.
-
RESEARCH METHODOLOGY
This paper aims to exploring the SAM adoption in electric power industry. To this aim, the paper answers the following Research Question: What are the enabling technologies and tools of SAM?.
Relevant publications are located with the help of google scholar with the objective to collect, organize, and synthesize existing SAM knowledge. The literature sources are investigated in Google scholar and IEEExplore. Due to the lack of precise key words defining the topic, the keywords were not predetermined before the search but they have gradually identified during the reading process. This search indicated that the concept of SAM is still in its early years in academic research, while it is more recognized and discussed among practitioners. To include professional reports, Google search engine is also used to complete the search.
Asset Performance Management
-
SMART ASSET MANAGEMENT (SAM) IN ELECTRIC POWER INDUSTRY
Asset Management (AM) in electric power systems can be broadly classified into four main categories based on the
possible time scales, i.e., real-time, short-term, mid-term, and long-term (Khaliq et al 2015). Real-time AM mainly covers the key power system resiliency principles and deals with the unexpected outages. Short-term AM is for maximizing the return on asset investments. Optimized maintenance scheduling falls within the mid-term AM. The investment planning of grid expansion falls within the scope of long-term AM.
In order to respond to first research question, we will therefore explore the literature on Data-driven AM decision- making and the enabling digital technologies for smart analytics in power industry. The dataset to be collected should be selected based on its value-added to support AM decision- making and to meet organizational objectives. Consequently, critical assets as well as their management decisions need to be identified.
Effective and smart AM requires power company to:
-
Understand its information needs to meet stakeholder (internal and external) requirements, including regulatory, information and reporting requirements (ISO 55001).
-
Identify the most critical assets to meet organizational objectives (Marquez et al 2020).
-
Identify the types of decisions that will have the greatest potential impact on achieving AM and organizational objectives. These decisions could include (IIMM, 2006; SAM, 2016):
Strategic decisions
-
Asset management strategy
-
Asset acquisition/creation/renewal/retirement
-
Capital investment decisions Tactical decisions
-
Life Cycle Value Realization
-
Operations and maintenance planning
-
Asset life cycle risk analysis
-
Asset reliability engineering
-
Shutdowns and outage strategy
-
Spare parts supply and inventory Operational decisions
-
Asset condition assessment
-
Prognostics and diagnostics
-
Maintenance execution and management
-
Asset usage reporting
-
-
Identify data and information needed to support the achievement of organizational objectives (ISO 55001). The required data could take many different forms, including:
-
Data about the assets condition and performance;
-
Data relating to operations and maintenance management and planning;
-
Data relating to safety, environmental and legal requirements;
-
Data relating to asset life cycle performance, cost and risks.
-
Data about asset investment planning
-
SAM has been addressed in the literature from several angles. Smart AM was addressed by Nel& Jooste (2016). The authors concluded that the key feature of Smart AM is therefore its
ability to interpret asset performance assessment in real-time, using technological intelligence, such as SCADA, CMMS, and EAM. Deloitte (2019) highlights how the maturation of the Internet of things (IoT) technology and lower-cost sensors enabling more data-driven decisions drive the development of Asset Performance Management (APM) solutions in asset- intensive industries. A mature APM program takes a holistic approach connecting systems across the business, from enterprise resource planning (ERP), to safety and quality as well as inventory management helping to optimize maintenance, operations and asset investment decisions to drive financial results (Deloitte,2019). Asset reliability is one of the most critical aspect of AM in power companies. To keep their physical assets these organizations are currently implementing Asset Performance Management (APM) solutions, which connect asset data to the organizations management information (IT) systems.
The greatest feature of smart AM is therefore its ability to interpret asset information in real time, allowing for flexible asset management decisions to be based on knowledge directly from the source. This further allows for a shift from asset status monitoring to asset performance monitoring, using technological intelligence. In the context of SAM technology, tools such as SCADA, CMMS, and EAM play a fundamental role in the effective application of SAM to assess asset performance. Edwards (2019) presents a framework for digital AM in the context of Building Information Modelling (BIM). In their recent work, Elbounjimi & Abdul-Nour,(2020) define Asset Management 4.0 as: An integrated and smart value- driven asset management . They identified five dimensions to develop the Asset Management 4.0 framework: Integrated planning, Value-driven decision-making, Enablers and capabilities, Asset information management and Performance measurement & improvement. Marquez et al (2020) define Smart AM (SAM) as software platforms to collect and analyze data from industrial assets. The authors present the main modules of Predix Platform developed by General Electric As well known APM solution for power industry.
Power companies have large capital budgets associated with their assets (McKinsey, 2018). These companies continue to work to better connect their assets with organizational value in order to increase their return-on-assets.
Power companies look to deploy more powerful analytics associated with the Internet of Things (IoT) and even artificial intelligence (AI) through software applications (Deloitte, 2019, AMCL, 2019, Belmont et al 2020) like Asset Performance Management (APM) and Asset Investment Planning (AIP). These solutions connect the production physical assets to all related departments using real-time data on the health and performance of assets. By being able to better manage, predict, and optimize their assets, operations and asset management can play a bigger role in the financial outcomes of capital asset organization.
After an in-depth analysis of the literature related to smart asset management (SAM) and based on previous work about AM 4.0, we define SAM as: An smart IT-IoT ecosystem integrating Enterprise resource planning (ERP), Asset Investment Planning (API), Asset Performance Management (APM) and Enterprise Asset Management (EAM). According to this definition, we propose a generic IT-IoT architecture of
SAM, shown in Figure 1. This architecture was adapted from APM architecture proposed by Gartner, (2017).
Figure 1 Generic IT architecture for SAM (modified from Gartner,2017)
-
-
SAM KEY ENABLING TECHNOLOGIES
Enterprise asset management (EAM)
EAM also exists under the name Computerized maintenance management systems (CMMS). CMMS helps streamline and automate maintenance tasks, providing communications for maintenance-related activities. EAM is broader and more comprehensive than CMMS. Koronios et al.(2005) refer to data quality in enterprise asset management (EAM), and consider CMMS and EAM as necessary to translate the huge available asset data into meaningful management information to optimize their operation and control [in] the total asset base
Asset Performance Management (APM)
Asset Performance Management (APM) is defined by Gartner (Gartner, 2017) as: a set of capabilities of data capture, integration, visualization and analytics tied together for the explicit purpose of improving the reliability and availability of physical assets. Asset Performance Management is a step ahead of Predictive Maintenance. In fact, APM includes predictive maintenance, condition monitoring and RCM. Predictive maintenance (PdM) leverages condition monitoring data like vibration analysis or thermal imaging to predict asset failure. APM advances a step by using smart analytics to anticipate asset failure with a higher degree of confidence and automatically triggers the right action to the right person. APM aims at improved operational performance, including safety, reliability, availability and integrity. APM enhances Enterprise Asset Management (EAM) foundation, using AI, advanced analytics and real-time data from sensors and devices. APM includes software application, Internet of things (IoT) infrastructure and Cloud or in-house database. Big data analytica(BDA) tools are often integrated into the APM software. Implementation of a digital APM solution can reduce utilities capital and operations and maintenance (O&M) spend by 20 percent or more (Belmont et al 2020). Some statistics captured by Schneider Electric APM include: 20% increase in asset availability, 20% reduction in operations and maintenance costs, 35% reduction in inventory costs and 20% increase in workforce efficiency.
Technology leaders including Siemens, GE, ABB, DNV GL, and Bentley have developed embedded APM solutions. Figure2 shows capabilities of each APM solution.
Figure 2 APM capabilities by vendor (Gartner,2017)
Asset Investment Planning (AIP)
AIP software applications have the potential to provide significant benefits to asset-intensive companies, including power utilities (AMCL, 2019). AIP integrates asset condition, asset criticality and the impact of time into their analysis of alternative investment scenarios. This decision support system enables organizations to assess trends, maintain or improve required service levels, and make more informed-based investment planning decisions. Moreover, unlike Excel spreadsheets, there is no limit to the amount of asset data an AIP solution can handle. By using big data analytics and machine learning algorithms, The AIP is designed to integrate information from the maintenance, production, engineering and financial departments in an effort to optimize and make optimal asset investment decisions and ensure both capital and operations & maintenance activities are aligned across all time horizons.
Internet of Things (IoT)
IoT forms the foundation of an smart AM system. IoT is considered as the network that enables smart things to be connected through the Internet (Ozger et al. 2018). The basic architecture of IoT platform is the middleware layer between the hardware and application layers (Figure 3). IoT provides many capabilities to grid such as security, real-time monitoring, awareness, control, and intelligence, which enable the grid to be more secure, and reliable (Ozger et al. 2018).
Figure 3 IoT as a middleware layer (Marquez et al,2020)
Recently, there are more and more IoT platforms dedicated to the electicity industry in the market. for example, General Electric (GE), PREDIX as an industrial IoT-based platform for a variety of industries including power industry.
Big Data Analytics (BDA)
Smart grid is associated with a vast amount of data from various sources (Figure 4), including power system operation (generation, transmission, and distribution, customers) and weather. A recent survey (C-Consulting,2015) about 1000 electric utility and industry respondents across ten countries depicted that majority (80%) of the electric utilities consider BDA as crucial for future smart grid and source of new business opportunities. However, only 20% of the utilities have implemented some BDA, and few among them have fully taken advantage. BDA provide considerable opportunities to make data-driven decisions as well as developing prediction models. BDA in smart grid are found in the following forms (Rabie et al 2020):
-
Domain and off-domain data prediction (called predictive analytics);
-
Natural language processing;
-
Classification, regression, and clustering (called
Figure 4 Key characteristics of smart grid big data (C-Consulting, 2015)
Data Mining and Machine Learning);
-
Statistical analysis;
-
Cognitive simulation, perception, and pattern recognition (called Artificial Intelligence);
-
Advanced data visualization.
As shown in Figure 5, the main steps of BDA development are data acquisition and ingestion, data storage, data analytics, and operational integration in power grid.
Figure 5 Key stages of BDA process (Bhattarai et al 2019)
ABB has developed a smart big data platform, called ABB Asset Health Center, which include asset conditions
monitoring and systems expertise to establish end-to-end asset management, minimizing costs and risks, improving reliability, and optimizing operations across the electric utility (ABB,2011). Another smart asset management platform has introduced by OSI-Soft for the purpose of real-time monitoring of asset health (Chavero, 2016). Bhattarai et al 2019 and Rabie et al 2020 present comprehensive state-of-the- art review of BDA opportunities and challenges in power industry. Until now, there are no standard BDA architecture developed for power industry. However, some architectures suitable for this industry are available (Bhattarai et al 2019.), such as, GE grid IQ insight architecture, IBM big data architecture, SAP big data architecture and Oracle big data architecture. In addition, there are many open-source big data processing engines like Apache Hadoop, Appache Drill, Spark and High-performance computing (HPC). Due to interconnexion between power and Telcom infrastructure, the power companies are facing increasing cyber-security risks.
Digital Twins (DT)
DT represents the next wave of development in modeling and simulation. DT can model complex asset system such as a generator or transformer, and by leveraging sensor (real-time) data and historical data, they can be used for maximizing asset performance and predicting when and how a failure could occur (Negrui et al 2017). Additional benefits for power companies could include improving reliability, optimizing operations and maintenance costs, and manage assets more effectively. Predictive maintenance is one of the most common uses of digital twins today. For example, a digital twin of a generator can inform when maintenance is needed based on comparing the predicted behavior to the actual behavior. Using simulation and predictive analytics, the DT can estimate the asset or component remaining useful life (RUL), which is very interesting data for maintenance management. As a result, If done correctly, digital twin allow the organization to perform simulation and what-if analysis on their physical assets. By combining the IoT with AI, DT become critical in improving productivity (Errandonea et al,2020).
However, effectiveness of a DT rests on its digital copy (model) of the physical asset. If its not, operational efficiency could decrease rather than increase. The effects of bad data on such a system can easily be staggering. Another data challenge is the use of edge technology, which involves data collection away from central processing centers and closer to the data source (such as IoT devices in the field).
Artificial Intelligence (AI) and Machine Learning (ML)
AI and ML are widely applied in diagnosis/prognostics, predictive maintenance, and Asset lifecycle Management decision-making and optimisation. Image Interpretation by AI has become an essential predictive maintenance tool in any ongoing inspection program. It is widely used for substation surveys and safety checks before planned maintenance work. This helps prevent costly downtime and loss of equipment. Machine learning (ML) are applied here to spot image patterns of what healthy equipment looks like. ML algorithms are
Predictive Maintenance (PdM) is an advanced level of Condition-based maintenance (CBM). CBM consists of anticipating a maintenance activity based on evidence of degradation and deviation from the normal behavior of the asset (Nikolaev et al., 2019). These anomalies are detected thanks to advanced technologies such as IoT and cloud computing, which are applied to monitor the condition of the asset. The main difference between CBM and PdM is that PdM enables to predict the remaining useful lifetime of component.
Traditional CBM can be enhanced by ML algorithms and Digital Twin to diagnose and acquire detailed status. Recent surveys list interesting developments of AI and ML for power systems (Azad, et al 2019; Kezunovic et al 2020).
It can lead to architectures that are more complex when different assets are simultaneously involved. Figure, 6 shows the typical process of PdM. Big data algorithms can detect changes in patterns in SCADA data, which is a fundamental element for asset managers.
Figure 6 PdM development process (Compare et al,2019)
PdM uses big data analytics, more specifically, machine learning and deep learning algorithms to make predictions about asset malfunction. This can significantly reduce costly unplanned downtime, as well as extend the remaining useful life of machinery and production equipment.
In the field of asset management, and more particularly maintenance, useful predictions are the predictions concerning component failures. Different techniques can be used to merge all available information for performing predictive maintenance to be as accurate as possible. There might be data-driven or model driven approaches. On the one hand, the data-driven approach is a Big data-oriented technique where a large volume of data on the state of the asset is required, which can be obtained with the appropriate sensor deployment. Based on the information available, a data analysis algorithm is developed. It provides results on the trends observed before the behavior of the asset (Hossain et al 2019, Azad, et al 2019, Kezunovic et al 2020). Duchesne, et al 2020 review the recent works applying machine learning (ML) techniques in the context of energy systems' reliability assessment and control.
-
-
CONCLUSION
To get the most out of digital and smart technologies, power companies should rethink their asset strategies to reduce operational expenses (Opex), capital expenses (Capex), as well as improving their assets reliability. Today, these companies can benefit from the high opportunities offered by
IoT, Asset performance management (APM), Asset Investment Planning (AIP), Digital Twins, and AI/ML technologies, in particular in order to identify in advance the risks of breakdowns through asset performance assessment and predictive maintenance. To this end, measurement data from current network operation, basic infrastructure data, geographic or meteorological data could be collected and analysed. AI and ML can improve power grid reliability with automated decision-making solutions.
As future researc, it will be important to:
-
Studying SAM in other industries;
-
identifying the success factors of SAM;
-
Developing a roadmap for the establishment of SAM in electricity utilities.
14 © 2020 Wiley Periodicals LLC / DOI 10.1002/gas CLIMATE AND ENERGY SEPTEMBER 2020 quickly understand the cost savings associated with rolling out a digital APM strategy. On the average, a utility that rolls out a digital APM strategy can save approximately 30 percent in operational expenditure and approximately 20 percent in capital expenditure compared to traditional asset management practices.
13 Maintenance schedules can be better pre-dicted and planned to ensure maximum opti-mization of labor and resources with a digital APM strategy. With a holistic, centralized view of asset health, crew work can be optimized by capability and geography when possible to reduce unnecessary trips to the same area for different asset types. Utilities can build an ef-fective and efficient maintenance program by shifting focus to assets with high data opacity.
CONCLUSION
Digital APM development is still in its in-fancy, with considerable room for expansion and innovation in the coming years. Utilities are moving from traditional static-run to fail-ure with break-fix procedures, to an integrated EAM and analytics-based APM program that can improve reliability and operational met-rics, while also delivering financial benefits with direct cost savings and societal benefits from reduced outages. Digital APM is also a not an all or noth-ing approach. Utilities can take incremental steps, or a phased approach, through the ma- turity model with corresponding benefits. Conservative tactical investments in people and processes can have a just as great or greater effect than similar technology investments. Utilities do not have to invest in a multiyear, extremely expensive, businesswide transfor-mational effort to start benefiting from initiat-ing an APM approach to managing and main- taining its asset base. An asset and operational evaluation are the easiest way to find the best place to start. extending them past preventative replacement s tandards, saving money without sacrific -ing reliability. One example is utilities use of drones to capture and monitor cracks, or lack thereof, in the tops of poles, thus prescribing replacement when needed or delaying until required.
-
COMPARATIVE BENEFIT ANALYSIS
Implementing EAM and digital APM by utilities leads to asset optimization throughout the entire organization. They allow utilities to draft asset strategies based on real-time data generated and analyzed from in-service de- vices and equipment. Organizational strategy management around assets becomes enhanced through a uniform decision- making process and a centralized database of asset informa- tion and history. This helps increase workflow efficiency and builds a backbone for predictive analytic practices. According to GE Digital, the installation of Internet of Things (IoT) de-vices (sensors) at multiple utilities across 5,000 assets in 900 power plants resulted in about 125 terabytes of data being analyzed daily and delivered over 200 billion tags. This reduced unplanned downtime by up to 5 percent and reduced false alarms by up to 75 percent.
-
This benefit translates into monetary value results in substantial cost savings for utilities implementing digital APM strategies as com-pared to the traditional methods of asset man-agement. Unexpected failures can be reduced by 55 percent due to real-time monitoring, data ingestion, analytics, and visualization.An EAM system that incorporates finan-cial data and reporting can enable utilities to
11 Guntrip, T. (2018, July 19). SCE ies drones for inspec- tions. T&D World. Retrieved from https://www.tdworld.com/electric-utility- operations/article/20971476/sce- ies-drones-for- inspections; Ryan, C. (2020, July 8). Drone vs
helicoptersUtility inspections. Retrieved from https://www. exelonclearsight. com/drone- vs-
helicopters- utility- inspections/; SDG& E. ( n. d.). Drones working to en-sure public safety. Retrieved from https://www.sdge.com/ wild re-safety/aviation- services
REFERENCES
-
ACÉ (2015). Innovation dans le secteur de lélectricité vers la Vision 2050. Association canadienne de lélectricité (ACÉ) 2015. https://electricity.ca/wp- content/uploads/2015/02/Innovationdanslesecteurdelelectricite.pdf
-
AMCL,2019. Asset Investment Planning. https://www.amcl.com/aip- solutions-demystified/
-
ABB: Using smart grid data to power end-to-end asset management. White paper, 2011.
-
Belmont, D., Hade, K., & Sefah, K. (2020). Digital Asset Performance ManagementTaking Utility Asset Management to the Next Level. Climate and Energy, 37(2), 9-14.
-
Bhattarai, B. P., Paudyal, S., Luo, Y., Mohanpurkar, M., Cheung, K., Tonkoski, R. & Manic, M. (2019). Big data analytics in smart grids: state-of-the-art, challenges, opportunities, and future directions. IET Smart Grid, 2(2), 141-154.
-
Azad, S., Sabrina, F., & Wasimi, S. (2019, November). Transformation of Smart Grid using Machine Learning. In 2019 29th Australasian Universities Power Engineering Conference (AUPEC) (pp. 1-6). IEEE.
-
Chavero, M.(2016). New smart asset management strategies in TSO industry enabled by a real-time data infrastructure. White paper, 2016.
-
C.Consulting: Big data blackout: are utilities powering up their data analytics?. Technical report, 2015
-
Compare, M., Baraldi, P., & Zio, E. (2019). Challenges to IoT- Enabled Predictive Maintenance for Industry 4.0. IEEE Internet of Things Journal, 7(5), 4585-4597
-
Deloitte. Daecher, Dipankar , Dunn, and Sniderman(2019). Asset performance management-Driving value beyond predictive maintenance.
-
Duchesne, L., Karangelos, E., & Wehenkel, L. (2020). Recent developments in machine learning for energy systems reliability management. Proceedings of the IEEE.
-
Edwards, R. (2019). Digital Asset Management. In Asset Management: Adding value to asset dependent businesses (pp. 183- 194). ICE Publishing.
-
El Bounjimi,M, Abdul-Nour,G. A conceptual framework for Asset Management 4.0. Conférence Internationale de Modélisation, Optimisation et Simulation – MOSIM 2020. 12-14 Novembre 2020.Agadir,Maroc.
-
Errandonea, I., Beltrán, S., & Arrizabalaga, S. (2020). Digital Twin for maintenance: A literature review. Computers in Industry, 123, 103316.
-
Gartner,2017. Asset Performance Management https://www.gartner.com/en/information-technology/glossary/asset- performance-management-apm.
-
Nikolaev, S., Belov, S., Gusev, M., & Uzhinsky, I. (2019, July). Hybrid Data-Driven and Physics-Based Modelling for Prescriptive Maintenance of Gas-Turbine Power Plant. In IFIP International Conference on Product Lifecycle Management (pp. 379-388). Springer, Cham.
-
Ozger M, Cetinkaya O, Akan O (2018) Energy harvesting cognitive radio networking for IoT-enabled smart grid. Mobile Netw Appl 23(4):956966
-
Marquez, A., Gomez Fernandez, J. F., MartÃnez-Galán Fernández, P., & Guillen Lopez, A. (2020). Maintenance Management through Smart Asset Management Platforms (SAMP). Emerging Factors, Key Impact Areas and Data Models. Energies, 13(15), 3762.
-
McKeinsey2018, Sousa, González, RodrÃguez González, and Tai(2018) Harnessing the power of advanced analytics in transmission and distribution asset management
-
McKinsey,2020. Diego Hernandez Diaz, Jesús Rodriguez Gonzalez, Gonçalo Pinheiro, and Alexander Weiss. The new imperative for grid operators.
-
Hossain, E., Khan, I., Un-Noor, F., Sikander, S. S., & Sunny, M. S.
H. (2019). Application of big data and machine learning in smart grid, and associated security concerns: A review. IEEE Access, 7, 13960-13988.
-
Keznovic, P. Pinson, Z. Obradovic, S. Grijalva, T. Hong, and R. Bessa,( 2020) Big data analytics for future electricity grids, Electric Power Systems Research, vol. 189, p. 106788,.
-
Koronios, A., Lin, S., & Gao, J. (2005, November). A data quality model for asset management in engineering organisations. In ICIQ.
-
Duchesne, E. Karangelos, and L. Wehenkel,( 2020) Recent developments in machine learning for energy systems reliability management, Proceedings of the IEEE,.
-
Khaliq, S. A., Mahmood, M. N., & Das, N. (2015). Towards a best practice asset management framework for electrical power distribution organisations. In 2015 IEEE PES Asia-Pacific Power and Energy Engineering Conference (APPEEC) (pp. 1-5). IEEE.
-
Negri, E., Fumagalli, L., & Macchi, M. (2017). A review of the roles of digital twin in cps-based production systems. Procedia Manufacturing,11,939-948.
-
Nel, C. B. H., & Jooste, J. L. (2016). A technologically-driven asset management approach to managing physical assets-a literature review and research agenda for smart asset management. South African Journal of Industrial Engineering, 27(4), 50-65.
-
Rabie, A. H., Saleh, A. I., & Ali, H. A. (2020). Smart electrical grids based on cloud, IoT, and big data technologies: state of the art. Journal of Ambient Intelligence and Humanized Computing, 1- 32.