
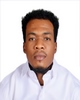
- Open Access
- Authors : Otusanya G.T , Olajide A.O , Alade A.O, Afolabi T.J , Akanbi A. A, Owoeye S.O, Adekola W.A
- Paper ID : IJERTV9IS070390
- Volume & Issue : Volume 09, Issue 07 (July 2020)
- Published (First Online): 28-07-2020
- ISSN (Online) : 2278-0181
- Publisher Name : IJERT
- License:
This work is licensed under a Creative Commons Attribution 4.0 International License
Kinetics Modelling of Biosorption of Bromothymol Blue onto Gliricidia Sepium and Acacia POD Composite
*1, 2 Otusanya G.T., 1,2 Olajide A.O., 1,2,3Alade A.O., 1,2,3Afolabi T.J.,
1, 2 Akanbi A.A., 1Owoeye S.O., and 1Adekola W.A.
1Department of Chemical Engineering, Faculty of Engineering and Technology, Ladoke Akintola University of Technology, Ogbomoso, Nigeria
2 Bioenvironmental, Water and Engineering Research Group (BWERG), Ladoke Akintola University of Technology, Ogbomoso, Nigeria
3 Science and Engineering Research Group (SAERG), Ladoke Akintola University of Technology, Ogbomoso, Nigeria
Abstract:- This study reports the removal of bromothymol blue from wastewater onto Gliricidia sepium (G. sepium) and Acacia pod (A. pods) composite. The developed biocomposite mixed ratio was varied from 5 to 95 percentage composition and the effective mixture was evaluated based on methylene blue number (MBN) test. Adsorption Capacity (QE) and Removal Efficiency (RE) of the seven (7) experimental runs were studied. Cubic and quartic model were developed for RE and QE response respectively. The surface characteristics of the biosorbent was chemically modified. Fourier Transform Infrared (FTIR) was studied. Batch adsorption was investigated and effect of temperature, and biosorbent dosage were studied at various contact time and at initial concentration of 10 mg/l. Suitable kinetics model (Pseudo-first-order, Pseudo-second-order and Elovich) were studied. Cubic and Quartic model was developed for RE & QE response respectively. The RE (%) and QE (mg/g) have the highest values (96.9736 and 0.969736) at Run 3 (0.95 G. sepium: 0.05 A. pods) with lowest values at Run 2 (0.05 G. sepium: 0.95 A. pods). There was appearance and disappearance of the O-H stretch, C=O, C-F, , -H stretch groups in the unmodified and modified G. sepium pod at different peaks, while appearance and disappearance of C-Cl stretch, C=O, N-H bend and O-H stretch groups were noticed in the modified and unmodified
-
pod. The result follows kinetic of pseudo-second-order and Elovich-rate models. It was observed that G. sepium and A. pods composite are effective precursors for the development of composite adsorbent due to its high yield, good QE & RE and increase in rate of adsorption.
Key words: Biosorption, Wastewater, Gliricidia sepium, Acacia pods, Kinetics, Bromothymol blue,
-
INTRODUCTION
Over the years, quality of water has been deteriorating rapidly due to the anthropogenic activities, population growth, unplanned urbanization, rapid industrialization and unskilled utilization of natural water resources. Furthermore, the increased awareness of the importance of providing impacts due to the current environmental strategies has pushed the research community towards the development of robust, economically feasible and environmentally friendly processes capable of removing pollutants from water and at same time to safeguard the health of affected populations (Sabino, Giusy, Mariangela, & Michele, 2016).
Todays highly industrialized environment is charged with a plethora of potentially toxic chemicals. The presence of harmful pollutants in the discharged wastewaters often contaminates the surface water and soil. Pollution of aquatic habitats and soil is a worldwide problem that can result in uptake and accumulation of toxic chemicals in food chains and are also harmful to the flora and fauna of affected habitats. Contamination of soil occurs when chemicals are deliberately released, by spill or underground leakage. Pesticides also play a vital role in environmental pollution. The persistent use of synthetic pesticides in agriculture, silviculture and even animal husbandry has created several difficulties on public health (Zamani, Sendi, & Ghadamyari, 2011). Problems associated with the hazards to man and environment are not confined to the developing countries, but extended to developed nations as well. The contamination of soils and groundwater with petroleum compounds is among the most prevalent problems worldwide (Alquati, Papacchini, Riccardi, Spicaglia, & Bestetti, 2005).
Industrial developments in recent years have left their impression on the environmental society (Mohamed, 2013). Many industries like the textile industry used dyes to colour their products and thus produce wastewater containing organics with a strong colour, where in the dyeing processes the percentage of dye lost waste water is 50% of the dye because of the low levels of dye-fibre fixation. Discharge of these dyes into effluents affects the people who may use these effluents for living purposes such as washing, bathing and drinking. Textile industries are one of the most common and essential sectors in the world. On the other hand, high volume of water consumption and varying wastewater characteristics due to many products, such as dyes, biocides, carriers, detergents, etc. used in the process are the factors that have caused a continuous effort to find appropriate technologies to treat textile industry wastewater (Eremektar, Selcuk, & Meric, 2007).
Recently, Low-cost by-products from agricultural, household and industrial sectors have been recognized as a sustainable solution for waste water treatment. They allow achieving the removal of pollutants from wastewater and at same time to contribute to the waste minimization, recovery and reuse. Despite numerous reviews published in the last few years, a direct comparison of
data obtained using different sorbents is difficult nowadays because of inconsistencies in the data presentation (Sameera, Naga, Srinu, & Ravi, 2011). However, there is minuscule information on kinetic study on wastewater treatment using low-cost materials. The aim of this research is to study the kinetics of less expensive adsorbents for the elimination of dyes from wastewater. Agricultural waste biocomposite Gliricidia sepium and Acacia pods will be used as adsorbents for the elimination of bromothymol blue from wastewater.
-
MATERIALS AND METHODS
-
Materials
The materials, Gliricidia sepium and Acacia pods were procured within the vicinity of Ladoke Akintola University of Technology (LAUTECH), Ogbomoso, Oyo state, Nigeria. They were washed with detergent and distilled water in order to completely kill the microbes present, afterwards sun-dried to constant mass (Alade et al., 2012). They were subsequently blended into smaller and uniform particle size using a blender and sieve analyzer respectively. The grinded G. sepium and A. pods were subsequently modified using hydrogen peroxide continuously until a clear solution was achieved. It was then oven dried till constant mass and kept safely for further use.
-
Adsorption of Methylene blue
Adsorption of Methylene-blue dye was carried out according to the procedures described by (Alade et al, 2012), with slight modifications. Grinded and decolorized biocomposite of G. sepium and A. pods (1 g, 0.95:0.05) soaked in 100 ml methylene blue dye (10 mg/L), then left for 24 hours. The solution was decanted and taken for UV analysis using a spectrophotometer to determine the un-adsorbed dye concentrations.
-
Design of Experiment
The design of the experiment is generally undertaken to minimize time, materials and invariably cost, involve in experimental design. Design-Expert software (Version 11.0.4.0). The experimental design information is expressed in Table 1 below.
Table 1. Experimental design information
Study Type
Mixture
Design Type
p>Simplex Lattice Design Model
Cubic
Build Time (ms)
2.00
Subtype
Randomized
Runs
7
Blocks
No Blocks
The independent variables were adsorption temperature (room temperature), dosage (1 g), concentration (10mg/L), and time (24 hrs), giving two response variables, response 1 is the adsorption capacity while response 2.00 is the removal efficiency. The experimental values are expressed in Table 2 below.
Table 2. Response derivatives
Response
Name
Units
Observations
Analysis
Transform
Model
R1
Adq
mg/g
5
Polynomial
Power
Cubic
R2
RE
%
6
Polynomial
Power
Quartic
Table 3. Component Mixture
Component Unit
Level
low
High
Gliricidia sepium
%
5
95
Acacia pod
%
5
95
Table 4. Experimental design of biocomposite mix used
Run
Gliricidia sepium (%)
Acacia (%)
Absorbance (abs)
Adsorption capacity (QE) mg/g
Removal efficiency (RE) (%)
1
65
35
2
5
95
3
95
5
4
35
65
5
72.5
27.5
6
50
50
7
27.5
72.5
-
Fourier Transmission Infrared Spectroscopy Analysis
The spectrum is a graph which contains percent transmittance along Y axis and frequency or wavelength along X axis. By studying the peak between a particular frequency i.e. gap or band, type of the functional group present was predicted. For the case of Mango seed kernel and Papaya seeds, FTIR shows the change in properties of the surface of biosorbent on addition of RR dye (Tariku, 2016).
-
Batch Adsorption of Bromothymol Blue from wastewater using Gliricidia sepium and Acacia seed pod Biosorbent
Certain quantity of the stock solution was taken to conduct batch experiments for dye adsorption. The parameter of the factors that affect the adsorption process (adsorbent dosage, concentration of adsorbate, temperature and contact time will be studied). For this experiment, the process of adsorption of bromothymol blue on G. sepium and Acacia seed pod were studied (Bhanuprakash & Belagali, 2017). A known amount of this solution will be taken in different numbers of beakers for different masses of the adsorbent and thereafter, adsorption will be studied with different concentrations of the bromothymol blue (Bhanuprakash & Belagali, 2017).
The percentage removal of the dye by each adsorbent was calculated from the relation:
Re =
× 100% (2.1)
Where Co and Ce are the concentrations (mg/L) of the dye initially and at equilibrium time (Bhattacharya, Naiya, Mandal, & Das, 2008).
The adsorbed amount qe was calculated from the relation:
qe = ()
-
Effect of Varying Factors
(2.2)
Temperature and biosorbent dosage were varied on the adsorption rate and removal efficiency at initial concentration of 10 mg/l across various contact time.
-
Effect of Biosorbent Dosage
The effect of biosorbent dose on the adsorption of 10 mg/l of Bromothymol blue is studied across dose 1g, 1.5 g and 2 g.
-
Effect of Temperature
The effect of temperature on the adsorption of 10 mg/l of Bromothymol blue is studied across temperature 40o, 50o and 60o Celsius.
-
-
Adsorption kinetics
Contact time from experimental results can be used to study the rate-limiting step in the adsorption process in terms of the kinetic energy. The overall adsorption process can be controlled either by one or more steps such as pore diffusion, surface diffusion or a combination of more than one step. Lagergens first order equation and Hos second order equation are such examples of kinetic models commonly used to describe these adsorption kinetic models (Ho, 2006).
-
The pseudo first-order kinetic
The pseudo first order kinetic equation of Lagergren model is given as such equations (Lagergren, 2009). Pseudo first order equation refers to the assumption of the rate of change of solute uptake with time, which is directly proportional to the difference in the saturation concentration and the amount of solid uptake with time (Khaled, et al 2009).
=
(
) (2.3)
1
where qe and qt are the amount of adsorbed waste (mg/g) at an equilibrium and at any instant of time t (min), respectively and k1 is the rate constant of pseudo first order adsorption operation (min).
-
The Pseudo second order Kinetic
The pseudo second order kinetic equation is given as (Ho, 1995):
= (
)2 (2.4)
The pseudo second order model is based on the assumption that the rate-limiting step may stem from the chemical adsorption involving valence forces through the sharing or exchange of electrons between the adsorbent and adsorbate (Ho & McKay, 1999).
The linearize equation for pseudo second order is written as
1
= + (2.5)
22
A plot of against t gives a straight line with a slope of 1/qe and an intercept of 1
22
-
The Elovich Model
-
The equation defining the Elovich model is based on a kinetic principle assuming that the adsorption sites increases exponentially with adsorption, which implies a multilayer adsorption. It is expressed by the relation:
= exp( ) (2.6)
The linearize equation for the Elovich model is written as;
= (2.7)
A plot of against qe gives a straight line with a slope of -1/qm and an intercept of
-
-
RESULTS AND DISCUSSION
-
Adsorption capacity and Removal Efficiency of Methylene Blue onto the Biocomposite
The experimental design with seven (7) runs for which data was provided in Table 2.4 for adsorption capacity (mg/g) and removal efficiency (%) gives the following results expressed in Table 5 & 6 below for which mixture loading is L-Pseudo, with transformation power of lambda, raised to the power of -3 having no constant. Suitable models for adsorption capacity and removal efficiency were usually selected based on the highest order polynomials.
The Quartic model has the highest R2 value (0.9999) and lowest standard deviation (0.0010) for the adsorption capacity while in Removal efficiency the Cubic model has the highest R2 value (1.0000) and lowest standard deviation (0.0096) and expressed in the model summary statistics, table 5 & 6 below was selected accordingly for this study. The suitability of the model was further supported with the least value (0.0138) of the Predicted Residual Error Sum of Squares (PRESS) for the adsorption capacity, while that of removal efficiecy has a PRESS value of 0.0347. The positive and negative coefficients indicated positive and negative influences of the independent variables on the selected responses.
Table 5. Model Summary Statistics for Adq
Source
Sequential p-value
Adjusted R²
Predicted R²
Std. Dev.
R²
PRESS
Linear
0.1960
0.2192
-1.4079
0.0341
0.3753
0.0180
Quadratic
0.1269
0.5778
-0.8613
0.0251
0.7467
0.0139
Cubic
0.6361
0.4506
-72.4908
0.0286
0.7802
0.5479
Quartic*
0.0162
0.9993
-0.8570
0.0010
0.9999
0.0138
*Suggested Results are means of duplicate values.
Table 6. Model Summary Statistics for RE
Source
Sequential p-value
Adjusted R²
Std. Dev.
R²
PRESS
Linear
0.0941
0.5486
0.5119
0.6615
3.60
Quadratic
0.0741
0.9034
0.2368
0.9517
3.09
Cubic*
0.0182
0.9998
0.0096
1.0000
0.0347
*Suggested Results are means of duplicate
-
ANOVA of Adsorption capacity (Cubic model) and Removal Efficiency (Quartic model)
The analysis of variance (ANOVA) procedure was used to determine the significance of variables and to substantiate the adequacy of the quadratic regression model obtained in this study. The significance of the model was based on the principle of the Fishers statistical test (F-test) and it generates the F-value, which represents the ratio of the mean square of regression to the mean error. Significance of the model terms were further tested based on lower probability (p-value) which may lie between 90
% confidence level (Hegazi, 2012). Lack of fit, which is usually preferred to be insignificant, was also used as diagnostic test to determine the adequacy of any model developed (Halder 2015). The results of the ANOVA are presented in Table 7.
Mixture Component coding is L_Pseudo for adsorption capacity and removal efficiency. Sum of squares is Type III Partial. The Model F-value for adsorption capacity and removal efficiency are 1766.28 and 8446.28, respectively. These values imply there are 1.78% and 0.80% chances that an F-value this large could occur due to noise. P-values less than 0.0500 indicate model terms are significant.
Table 7. ANOVA for Response Surface model Analysis for adsorption capacity
Adsorption Capacity
Removal Efficiency
Source
Sum of Squares
Mean Square
F-value
p- value
Sum of Squares
Mean Square
F-value
p-value
Model
0.0075
0.0019
1766.28
0.0178
2.32
0.7741
8446.28
0.0080
¹Linear Mixture
0.0028
0.0028
2652.12
0.0124
1.54
1.54
16761.38
0.0049
AB
0.0006
0.0006
596.44
0.0261
0.6438
0.6438
7024.29
0.0076
AB(A-B)
0.0011
0.0011
1025.40
0.0199
0.1121
0.1121
1222.61
0.0182
Residual
1.055E-06
1.055E-06
0.0001
0.0001
Cor Total
0.0075
2.32
P> 0.10
-
Fit Statistics for Adsorption capacity and removal efficiency
The adsorption capacity negative Predicted R² of -0.8570 implies that the overall mean may be a better predictor of your response than the current model. In some cases, a higher order model may also predict better. While for removal efficiency, a positive Predicted R² 0.9851 is close to the Adjusted R² of 0.9998 which is what one normally expect; i.e. the difference is not greater than 0.2. It may indicate a large block effect or a possible problem with your model and/or data if the difference is greater than 2. Things to consider are model reduction, response transformation, outliers, etc. All empirical models should be tested by doing confirmation runs.
Adeq Precision measures the signal to noise ratio. A ratio greater than 4 is desirable. The ratio of adsorption capacity and removal efficiency are 129.454 and 210.834 respectively, these values indicate an adequate signal. These model can be used to navigate the design space. Table viii gives indication of the lower and upper limits for the adsorption capacity and removal efficiency, it also expresses lower and upper weights which are the same for both adsorption capacity and removal efficiency.
-
Constraints and Solutions
The constraint of the biocomposite mix ratio are presented in Table 9. The plots are expressed in fig. 3.1, 3.2, 3.3 and 3.4, constituting the mix ratio making up the biocomposite for both RE and QE.
Table 8. Statistics for adsorption capacity
Adsorption Capacity
Removal Efficiency
Properties
Values
Values
Std. Dev.
0.0010
0.0096
Mean
1.16
95.62
C.V. %
0.0887
0.0100
R²
0.9999
1.0000
Adjusted R²
0.9993
0.9998
Predicted R²
-0.8570
0.9851
Adeq Precision
129.4540
210.8337
Table 9. Required constr
aints showing the li
mit of the re
sponse
Name
Goal
Lower Limit
Upper Limit
Lower Weight
Upper Weight
Importance
A:A
is in range
5
95
1
1
3
B:B
is in range
5
95
1
1
3
Adq
Minimize
1.09658
1.21784
1
1
3
RE
Minimize
95.1679
96.9736
1
1
3
Table 10. Solutions
Number
A
B
Adq
RE
Desirability
1
5.000
0.970
96.974
1.000
2
93.253
6.747
0.944
95.197
0.062
3
95.000
5.000
0.952
95.169
0.015
1. 22
1. 2
1. 18
Predicted
Predicted
1. 16
1. 14
1. 12
Predicted vs. Actual
0.98
0.97
0.96
Adq (mg/g)
Adq (mg/g)
0.95
0.94
0.93
Two Component Mix
1. 1
1. 08
1. 08 1. 1 1. 12 1. 14 1. 16 1. 18 1. 2 1. 22
Actual
0.92
0.91
A: 5
B: 95
27.5
72.5
50
50
A: A (%)
B: B (%)
72.5 95
27.5 5
Fig. 3.1 Plot of Predicted versus Actual values for adsorption capacity
97
96. 5
Predicted
Predicted
96
95. 5
Predicted vs. Actual
97.5
97
RE (%)
RE (%)
96.5
96
95.5
Two Component Mix
95
95 95. 5 96 96. 5 97
Actual
95
A: 5
B: 95
27.5
72.5
50
50
A: A (%)
B: B (%)
72.5 95
27.5 5
Fig. 3.2 Plot of Predicted versus Actual values for removal efficiency
Two Component Mix
1.000 Desirability 0.931
0.94
Two Component Mix
0.800 0.92
Prediction 0.90558
Desirability
Desirability
Adq (mg/g)
Adq (mg/g)
0.600 0.9
0.400 0.88
0.200 0.86
0.000 0.84
A: 5
27.5
50
72.5
95
A: 5
27.5
50
72.5
95
B: 95
72.5
50
A: A (%)
B: B (%)
27.5
5
B: 95
72.5
50
A: A (%)
B: B (%)
27.5
5
Two Component Mix
94
92
Prediction 90.5355
90
RE (%)
RE (%)
88
86
84
82
80
A: 5
B: 95
27.5
72.5
50
50
A: A (%)
B: B (%)
72.5 95
27.5 5
Fig. 3.3 Plots of RE, Adq and desirability
5 95 5 95
A:A = 5 B:B = 95
0.936419 0.969736 95.1679 96.9736
Adq = 0.969727 RE = 96.9742
Desirability = 1.000
Solution 1 out of 3
Desirability = 1.000
Solution 1 out of 3
Fig. 3.4 Responses of the RE, Adq and desirability plots
-
-
Fourier Transform Infrared Spectroscopy (FTIR)
The presence of various functional groups presents on the surface of the adsorbent and their role in adsorption was analyzed using FTIR (Fourier transform infrared) spectrum within the range of 4004000cm1 using Thermo-Fisher FT-IR analyzer (SCIENTIFIC, NICOLET 5700). The presence of these functional group in the activated carbon will be responsible for adsorption for different heavy metal from aqueous solution (Rai et al., 2015). The FTIR spectra of G. sepium unmodified, G. sepium modified, Acacia unmodified and Acacia modified are represented in Table 11 and 12 respectively. The IR peak observed in the G. sepium unmodified ranges from 612.2 to 3957.6 while the modified ranges from 609.5 to 3964.2. It was observed from table xi that the peak height for the unmodified G. sepium ranges from 5.0 to 16.1 while the peak height for the modified ranges from 1.9 to 8.1.
In Acacia pod, it was observed that the unmodified IR peak ranges from 636.6 to 3964.2 while the IR peak for the modified ranges from 610.2 to 3964.1. The peak height for the unmodified and the modified ranges from 2.9 to 14.0 and 0 to 9.0 respectively. Some changes were observed in the functional groups of both samples and these were presented in table 11 and 12. There was the appearance of the C= stretch at peak of 5.7 cm-1 and O-H stretch at peak of 5.7 cm-1 after the modification of G. sepium. It was also observed that in Acacia there was an appearance of the N-H stretch at peak height of 4.1 cm-1 and C-O stretch at a peak height of 5.9 cm-1. The FTIR helps to reveal whether a reduction, appearance, disappearance or broadening of the peaks after the modification with hydrogen peroxide has occurred by the spectrum of the sample (Olajire, Abidemi, Lateef, & Benson, 2017).
Table 11. FTIR Spectra changes and peak height for Gliricidia sepium
Untreated G. sepium
Treated G. sepium
Peak
Bond Type
Peak height
Peak
Bond Type
Peak height
Remark
612.2
726.3
775.3
874.5
989.2
1023.1
1076.8
1155.0
1208.6
1281.0
1469.3
1544.0
1639.2
1709.0
1757.5
1911.1
2004.7
2081.7
2152.1
2226.4
2300.0
2404.0
2443.0
2533.1
2585.4
2693.9
2762.5
2825.8
2909.3
2957.1
3049.8
3127.4
3219.8
3327.0
3400.8
3460.1
3497.6
3569.6
3616.0
3700.8
3773.1
3838.4
3898.4
3957.6
C-Br stretch
=C-H bend C-Cl stretch
=C-H bend C-F stretch C-F stretch C=O stretch C=O stretch
C-F stretch C-H bend
N-H bend C=O stretch C=O stretch
C=O symmetric
O-H stretch O-H stretch O-H stretch
O-H stretch O-H stretch C-H stretch C-H stretch
=C-H stretch
=C-H stretch
N-H symmetric
O-H stretch O-H stretch O-H
O-H stretch N-H stretch N-H stretch N-H stretch N-H stretch N-H stretch N-H stretch N-H stretch
8.4
10.2
10.1
11.4
11.1
8.6
6.1
6.2
11.3
11.2
10.6
9.6
8.1
7.6
9.1
15.1
14.2
14.2
16.1
15.4
15.0
14.1
14.3
13.0
12.3
12.3
11.3
11.6
9.1
9.7
9.0
7.5
6.7
6.7
6.3
5.0
5.7
7.4
6.6
12.3
10.6
10.7
10.6
11.5
609.5
737.1
787.0
874.3
949.8
1013.7
1059.8
1119.8
1240.5
1272.8
1359.8
1444.9
1494.1
1552.0
1677.6
1732.9
1794.9
2016.8
2077.8
2281.4
2352.0
2482.1
2604.8
2724.9
2785.1
2855.2
2922.2
3022.6
3084.0
3162.1
3223.2
3306.3
3451.4
3517.9
3583.7
3661.6
3725.0
3782.4
3840.3
3903.7
3964.2
=C-H bend C-F stretch C-F stretch C-O stretch
C-O stretch
=C-F stretch C-F stretch C-H bend C=C stretch N-H bend C=O stretch C=O stretch C=O stretch
O-H stretch O-H stretch O-H stretch O-H stretch O-H stretch C-H stretch
=C-H stretch
=C-H stretch
N-H symmetric
O-H stretch
O-H stretch O-H stretch N-H stretch N-H stretch N-H stretch N-H stretch N-H stretch N-H stretch N-H stretch
4.4
4.3
5.6
5.1
4.6
3.9
3.0
3.4
4.9
4.2
6.3
4.8
5.7
4.5
4.2
3.8
5.7
6.9
6.9
7.0
8.1
6.2
5.4
5.7
5.5
5.3
4.4
5.0
4.1
3.6
3.5
3.7
2.0
1.9
2.6
3.9
5.3
5.4
5.6
4.3
6.0
Shifted downward Shifted upward Shifted upward
Shifted downward Shifted downward Shifted downward Shfted downward Disappear
Appear
Shifted downward Appear
Shifted downward Appear
Shifted upward Shifted upward Shifted upward Shifted upward
Disappear Disappear Appear
Disappear
Shifted downward Shifted downward Appear
Shifted upward Shifted upward Shifted upward Disappear
Shifted downward Shifted downward Shifted downward Appear
Shifted downward Appear
Shifted downward Shifted upward Shifted upward Shifted upward Shifted upward Shifted upward Shifted upward Shifted upward Shifted upward
-
r stretch C-H bend
-
l stretch
Table 12. FTIR Spectra changes and peak height for Acacia pod
Untreated Acacia
Treated Acacia
Peak
Bond Type
Peak height
Peak
Bond Type
Peak height
Remark
636.6
725.1
788.3
877.7
966.7
1074.3
1147.1
1243.0
1287.9
1364.0
1463.8
1530.2
1694.2
2020.6
2082.4
2248.1
2342.4
2443.1
2515.2
2606.7
2717.4
2787.0
2852.5
2889.8
2938.4
3038.6
3101.3
3200.7
3306.6
3367.1
3433.8
3485.4
3538.3
3590.7
3650.5
3763.2
3842.4
3884.7
3964.2
C-Cl stretch C-Br stretch
C Cl stretch
= C H bend
= C H bend C F stretch C F stretch C F stretch C F stretch C F stretch C H bend C = H bend
C=O stretch
O- H stretch
O- H stretch O-H stretch O- H stretch
O- H stretch O- H stretch O- H stretch
=C-H stretch O- H stretch
O- H stretch O H stretch O- H stretch O- H stretch O- H stretch O H stretch O H stretch O H stretch N H stretch N H stretch N H stretch N H stretch
7.6
7.6
7.5
8.7
6.4
4.6
4.9
7.2
8.6
9.9
7.9
8.0
3.6
11.9
12.3
13.6
14.0
13.3
11.7
11.6
10.7
9.8
7.2
8.7
6.0
6.7
5.4
4.9
3.0
2.9
4.0
4.1
3.4
3.5
5.4
9.7
9.2
8.7
9.9
610.2
714.1
769.8
875.1
982.3
1073.5
1148.6
1233.7
1291.0
1380.2
1470.7
1555.2
1655.2
1683.9
1748.3
2004.3
2078.6
2253.0
2365.9
2432.0
2533.8
2587.2
2691.2
2749.8
2832.7
2886.4
2963.9
3051.3
3101.6
3141.9
3203.7
3271.0
3384.9
3441.4
3501.0
3561.6
3617.8
3687.8
3753.5
3846.1
3964.1
C-Cl stretch
C-Br stretch C Cl stretch
= C H bend
= C H bend C F stretch C F stretch C F stretch C F stretch C F stretch C – C bend
C = H bend C = O stretch C = O stretch
C = O stretch
O H stretch O H stretch O H stretch O H stretch O H stretch O H stretch O H stretch
=C H stretch O H stretch O H stretch O H stretch O H stretch O H stretch O H stretch O H stretch O H stretch O H stretch O H stretch N H stretch N H stretch
N H stretch
4.8
6.4
7.3
7.7
2.1
0.5
0
0.4
0.6
4.5
6.2
6.1
6.4
6.2
5.9
8.5
8.4
8.3
2.3
8.9
5.9
9.0
7.4
9.0
6.8
4.7
3.5
5.4
4.4
4.0
1.7
0.4
1.1
1.8
0.9
-0.1
-0.5
0.1
0.9
2.3
4.1
Appear Disappear
Shifted downward Shifted downward Shifted downward Shifted upward Shifted downward Shifted upward Shifted downward Shifted upward Shifted upward Shifted upward Shifted upward Appear
Appear Disappear Appear
Shifted upward
Shifted upward Shifted downward Shifted downward Shifted downward Shifted downward Shifted downward Shifted upward Shifted upward Shifted upward Appear
Shifted upward Shifted downward Shifted upward Shifted downward Shifted upward Shifted downward Shifted upward Shifted upward Shifted downward Shifted upward Disappear
Shifted upward
-
-
Effect of Biosorbent Dosage
-
QE and RE study
Effect of biosorbent dose of the adsorbent composite mix was greatly influenced in the adsorption process using G. sepium and Acacia pods. The dose of biosorbent at various time from 15 minutes to 210 minutes were investigated for biosorbent dose 1g, 1.5 g and 2 g and the results is given. It was observed from the plot of QE against time shows that the adsorption capacity increased with increasing time from 15- 210 minutes. It was also observed that the adsorption capacity decreased with increasing biosorbent dose.
It was observed that the maximum adsorption capacity for all considered dose occurred at 210 minutes and the values are estimated to be 0.819767 mg/g, 0.624031 mg/g, and 0.485465 mg/g for 1 g, 1.5 g and 2 g respectively. The lowest adsorption capacities were observed to occur at 15 minutes with the data estimated as 0.491279 mg/g, 0.554264 mg/g and 0.44186 mg/g for 1 g, 1.5 g and 2 g respectively. The trend from the adsorption capacity results from studied concentrations shows that adsorption capacity decreases with increase in biosorbent dose and it is in agreement with results in a similar study by Amuda et al., (2013).
Biosorbent dose also affects the removal efficiency during adsorption process. The removal efficiencies were observed to increase with an increase in time from 15-210 minutes also with an increase in biosorbent dose across 1 g, 1.5 g and 2 g. The maximum experimented RE for all concentrations were calculated to be 82 %, 94 % and 97 % for 1 g, 1.5 g and 2 g respectively. The lowest removal efficiency for concentration 1 g, 1.5 g and 2 g are 49%, 83% and 88 % respectively. The trend from this
QE
QE
RE
RE
results shows that increase in biosorbent dose increases the removal efficiency of the adsorbate and it is in consonance with previous study by Amuda et al., (2014).
1g 1.5g 2g
0.9
0.8
0.7
0.6
0.5
0.4
0.3
0.2
0.1
0
0 50 100 150 200 250
TIME (mins)
120 1g 1.5g 2g
100
80
60
40
20
0
15 30 45 60 80 100 120 150 180 210
TIME (mins)
Fig. 3.5 Plot of QE against Time
Fig. 3.6 Plot of RE against Time
-
Kinetics Study
Pseudo-First Order Kinetic models
The plot of ln (Qe-Qt) against time describes the relationship between the pseudo-first order kinetics constant as obtained from the slope and intercept of the plot for 1 g, 1.5 g and 2 g. The kinetic parameter for each of the concentration obtained are K1, qe cal, qe experimental and R2. The qe cal parameter are estimated to be 0.3350, 0.0930 and 0.0495 for biosorbent dose 1 g, 1.5 g and 2 g respectively. The qe experimental parameter obtained are 0.819767, 0.624031 and 0.485465 for biosorbent dose 1 g,
1.5 g and 2 g respectively. It is generally observed that there is a variation between the qe cal and the qe exp for all the dose; as such it indicates that pseudo-first order poorly fit the data (Skrkalj and Malina, 2011).
The K1 values estimated are 0.0117, 0.0134, 0.0097 for biosorbent dose 1 g, 1.5 g and 2 g respectively. The estimated R2 parameter are 0.9550, 0.9704, 0.9814 for biosorbent dose 1 g, 1.5 g and 2 g respectively. The R2 values is less than that of Pseudo-second order (0.9968,0.9996 0.9996) for the three biosorbent dose and Elovich R2 values 0.9936, 0.9866 for dose 1g and 1.5g respectively. This buttress the point that pseudo-first order model is poorly fit for the data.
Pseudo-Second Order Kinetic models
The plot of t/q against t was used to evaluate K2, qe exp, qe cal and R2 for the pseudo-second order model equation parameters. The K2 obtained for 1 g, 1.5 g and 2g biosorbent dose are 0.0932, 0.0782 and 0.0766 respectively. The qe cal parameter are estimated to be 0.8540, 0.6312 and 0.4872 for biosorbent dose 1 g, 1.5 g and 2 g respectively. The qe experimental parameter obtained are 0.819767, 0.624031 and 0.485465 for biosorbent dose 1 g, 1.5 g and 2 g respectively.
The closeness between the qe cal and qe exp for both concentration and this suggests that pseudo-second order fitted the adsorption data better than Pseudo-first order. The estimated R2 parameter for the model is 0.9968,0.9996, 0.9996 for biosorbent dose 1 g, 1.5 g and 2 g respectively. The R2 values is well fitted compared to Pseudo-first order (0.9550, 0.9704, 0.9814) and Elovich (0.9936, 0.9866, 0.9601). High R2 values obtained for pseudo-second order further emphasizes the suitability over pseudo-first order and Elovich model.
Elovich Kinetic models
The plot of ln qe/ce against qe describes the relationship between the Elovich kinetics constant as obtained from the slope and intercept of the plot for 1g, 1.5 g and 2g. The kinetic parameter for each of the concentration obtained are KE, qm and R2. The qm parameter estimated are -0.2166, -0.0664, -0.0313 for biosorbent dose 1 g, 1.5 g and 2 g respectively. The KE were estimated to be -0.0440, -0.0116 and -8.19E-6 for biosorbent dose 1 g, 1.5 g and 2 g respectively. The correlation coefficient R2 for the Elovich model is estimated to be 0.9936, 0.9866, 0.9601 for biosorbent dose 1 g, 1.5 g and 2 g respectively. The R2 values are well fitted compared to the pseudo-first order kinetic model values (0.9550, 0.9730, 0.8699).
0
-0.5
log (qe-qt)
log (qe-qt)
-1
-1.5
-2
-2.5
0 50 100 150 200
500
450
400
350
t/qt
t/qt
300
250
200
150
100
50
1g 1.5g 2g
1g Time (t)
1.5g 0
2g Linear (1g)
0 100
Time
200 300
Fig. 3.7 Pseudo-first order kinetic plot of Ln (Qe – Qt) against time (mins) at different dosage
Fig. 3.8 Pseudo-second Order plot of T/Q against Time (t) at different biosorbent dose
1
0.5
0
0.35 0.45 0.55 0.65 0.75 0.85
ln qe/Ce
ln qe/Ce
-0.5
-1
-1.5
-2
-2.5
-3
qe
Fig. 3.9 Elovich plot of ln qe/ce against qe for effect of biosorbent dosage
-
-
Effect of Temperature
-
QE and RE study
-
Effect of temperature has a great influence in the adsorption process using G. sepium and Acacia pods. The effect of temperature at various time from 15 minutes to 210 minutes were investigated for temperature 40o, 50o & 60o Celsius and the results is given. It was observed from the plot of QE against time that the adsorption capacity increased with increasing time from 15- 210 minutes. It was also observed that the adsorption capacity decreases with increasing temperature from 40o-60o Celsius.
The maximum adsorption capacity for all temperatures were observed to occur at 210 minutes and the values are estimated to be 0.755814 mg/g, 0.715116 mg/g, and 0.700581 mg/g for 40o, 50o and 60o Celsius respectively. The lowest adsorption capacities were observed to occur at 15 minutes with the data estimated as 0.531977 mg/g, 0.436047 mg/g and 0.43314 mg/g for 40o, 50o and 60o Celsius respectively. The trend from the adsorption capacity results from studied concentrations shows that adsorption capacity decreases with increase in temperature and it is in agreement with results in earlier reports (Satapathy et al., 2013).
Removal efficiency has been greatly affected by temperature during adsorption process. The removal efficiencies were observed to increase with an increase in time from 15-210 minutes but decreases with an increase in temperature across 40o, 50o and 60o Celsius. The maximum experimented RE for all temperatures were calculated to be 76%, 72% and 70% for 40o, 50o and 60o Celsius respectively. The lowest removal efficiency for the temperature 40o, 50o and 60o Celsius are 53%, 44% and 43% respectively. The trend from this results shows that increase in temperature decreases the removal efficiency of the adsorbate. This phenomenon indicates that the adsorption process was exothermic in nature it is in consonance with earlier studies by (Satapathy et al. 2013; Girish and Murty 2014; Inyinbor et al. 2016 a, b).
3.4.2 Kinetics Study
40 deg 50 deg
0.8 60 deg
0.7
0.6
0.5
0.4
0.3
0.2
0.1
0
-20 30 80 130
TIME (mins)
40 deg 50 deg 60 deg
80
70
60
50
40
30
20
10
0
15 30 45 60 75 90 105 120
TIME (mins)
Fig. 3.10 Plot of QE against Time
Fig. 3.11 Plot of RE against Time
QE
QE
RE
RE
Pseudo-First Order Kinetic models
The qe cal parameter are estimated to be 0.3330, 0.4600 and 0.5000 for temperature 40o, 50o and 60o Celsius respectively. The qe experimental parameter obtained are 0.755814, 0.715116 and 0.700581 for temperature 40o, 50o and 60o Celsius respectively.
The K1 values estimated are 0.0200, 0.0214, 0.0253 for temperature 40o, 50o and 60o Celsius respectively. The estimated R2 parameter are 0.9757, 0.9537, 0.9251 for temperature 40o, 50o and 60o Celsius respectively. The R2 values is less than that of Pseudo-second order (0.9937, 0.9859, 0.9890) and Elovich (0.9980, 0.9860, 0.9994), from these values it can be deducted that it is a poorly fitted data compared to other models studied.
Pseudo-Second Order Kinetic models
The K2 obtained for temperature 40o, 50o and 60o Celsius are 0.1039, 0.0614, 0.0639 respectively. The qe cal parameter from table 4.20 are estimated to be 0.8041, 0.8030 and 0.7935 for temperature 40o, 50o and 60o Celsius respectively. The qe experimental parameter obtained are 0.755814, 0.715116 and 0.700581 for temperature 40o, 50o and 60o Celsius respectively. There is closeness between the qe cal and qe exp for all temperature and this suggests that pseudo-second order fitted the adsorption data better than Pseudo-first order. The estimated R2 parameter for the model is 0.9937,0.9859, 0.9890 for temperature 40o, 50o and 60o Celsius respectively. The R2 values is well fitted compared to Pseudo-first order (0.9757, 0.9537, 0.9251) but less of a good fit compared to Elovich (0.9980, 0.9860, 0.9994). High R2 values obtained for pseudo-second order further emphasizes the suitability over pseudo-first order.
Elovich Kinetic models
The qm parameter estimated are -0.2247, -0.1812, -0.2409 for temperature 40o, 50o and 60o Celsius respectively. The KE were estimated to be -0.0465, -0.0211 and -0.0521 for temperature 40o, 50o and 60o Celsius respectively. The correlation coefficient R2 for the Elovich model are estimated to be 0.9980, 0.9860, 0.9994 for temperature 40o, 50o and 60o Celsius respectively. The R2 values are well fitted compared to the pseudo-first order kinetic model values (0.9757, 0.9537, 0.9251) and pseudo-second order (0.9937, 0.9859, 0.9890) and this emphasizes the suitability of the model.
0
-0.2
-0.4
-0.6
-0.8
log (qe-qt)
log (qe-qt)
-1
-1.2
-1.4
-1.6
-1.8
0 20 40 60 80 100
Time (t) mins
40 deg 50 deg
200
180
160
140
t/qt
t/qt
120
100
80
60
40
20
0
-20 30 Time (t) mins80 130
60 deg Linear (40 deg)
Linear (40 deg)
Linear (50 deg)
Linear (50 deg)
Linear (60 deg) Linear (60 deg)
Fig. 3.12 Pseudo-first order kinetics plot of Ln (Qe-Qt) against Time at different temperatures
Fig. 3.13 Pseudo-second order plot of T/Q against Time (min) at different temperatures
-1
-1.20.35 0.55 0.75
-1.4
ln qe/Ce
ln qe/Ce
-1.6
-1.8
-2
-2.2
-2.4
-2.6
-2.8
-3
qe
40 deg cel 50 deg cel 60 deg cel
Fig. 3.14 Elovich kinetics model plot of ln qe/ce against qe at different temperatures
-
-
CONCLUSION
The biosorption of Bromothymol blue from wastewater onto G. sepium and A. pod composite has been studied. The biocomposite,
G. sepium and A. pod were modified with 0.1M of diluted Hydrogen peroxide (1: 100) of different mixture ratio of 7 experimental runs as suggested by the design expert software. The most effective run with highest QE and RE is Run 3 (0.95 G. sepium: 0.05
A. pod). Two-Level factorial design in the Design of Expert was an appropriate tool to evaluate the quality of the model which gave R2 to be 0.9999 and 0.9851 for adsorption capacity and removal efficiency respectively. The FTIR suggests the surface chemistry of the G. sepium and Acacia pods, it indicates the large presence of O-H, C-O, N-H and C=C and there was appearance and disappearance of the O-H stretch, C=O, C-Cl, C-F, stretch groups in the unmodified and modified G. sepium pod at different peaks, while appearance of C-H, C-Cl, O-H, N-H bend and stretch groups where noticed in the modified and unmodified A. pod at different peaks. A decrease in the QE was observed as the biosorbent dose increases while RE was observed to increase as time and dosage increases. QE and RE decreases as temperature increases but increases with time. Pseudo second- order and Elovich models were observed to be suitable as they express the kinetic model well. It can be deduced that G. sepium and A. pod are effective agricultural materials for the development of a biocomposite as adsorbent in adsorption study due to its high yield, good adsorption capacity, removal efficiency and increase in rate of adsorption.
-
CONFLICT OF INTEREST There is no conflict of interest associated with this work.
-
REFERENCES
-
Abas, A., Ismail, M., Kamal, L., & Izhar, S. (2013). Adsorption Process of Heavy Metals by Low-Cost Adsorbent: A Review. World Applied Sciences Journal, 28(11), 1518-1530.
-
Adegoke, K., & Bello, O. (2014). Dye sequestration using agriculturalwastes as adsorbents. Water Resources and Industry, 12(2015), 8-24.
-
Ahalya, N., Ramachandra, T., & Kanamadi, R. (2003). Biosorption of heavy metals. 7(4), 71-79.
-
Bello, O., Bello, O., & Lateef, I. (2014, June). Adsorption characteristics of mango leaf (mangifera Indica) as adsorbent for malachite green dye removal fron aqueous solution. Covenant Journal of Physical and Life Sciences , 2(1), 1-13.
-
Bhanuprakash, M., & Belagali, S. (2017). Study of Adsorption Phenomena by Using Almond Husk for Removal of Aqueous Dyes. Current World environment, 12(1), 80-88.
-
Bhattacharya, A., Naiya, T., Mandal, S., & Das, S. (2008). Adsorption, Kinetics and Equilibrium Studies on Removal of Cr(VI) from Aqueous Solutions using Different Low cost adsorbents. Chemical Engineering Journal, 137, 529-541.
-
D., A. (n.d.). Agricultural based activated carbon for the removal of dyes from aqueous solutions. A review. Journal of Harzadous Material, 167(1- 3), 1-9
-
Dada, A., Inyinbor, A., & Oluyori, A. (2012, Sept-Oct). Comparative Adsorption Of Dyes Unto Activated Carbon Prepared From Maize Stems And Sugar Cane Stems. Journal of Applied Chemistry (IOSR-JAC), 2(3), 38-43.
-
El-Halwany, M. (2010). Study of adsorption isotherms and kinetic models for methyleneblue adsorption on activated carbon developed from Egyptian rice hull. Desalination, 250(1), 208-213.
-
Eremektar, G., Selcuk, H., & Meric, S. (2007). Investigation of the relation between COD fractions and the toxicity in a textile finishing industry wastewater: effect of preozonation. Desalination. Desalination, 211(1-3), 314-320.
-
Farida, M. S., Hamed, M., Heba, A. B., Zakaria, A., & Shalabi, M. (2015, May). Adsorption Kinetics of Bromophenol Blue and Eriochrome Black T using Bentonite Carbon Composite Material. International Journal of Scientific & Engineering Research, 6(5), 679-688.
-
Febrianto, J., Kosasih, A., Sunarso, J., Ju, Y., Indraswati, N., & Ismadji, S. (2009). Equilibrium and kinetic studies in adsorption of heavy metals using biosorbent. Journal of Harzadous Materials, 162(2-3), 616-645.
-
Ghoreishi, S., & R.Haghighi. (2003). Chemical catalytic reactiotextile effluents and biological oxidation for treatment of non biodegradable. Chemical Engineering Journal, 95(1-3), 163-169.
-
Ho, Y. S. (1995). Adsorption of Heavy Metals from Waste streams by Peat. Water Research, 33(11), 2469-2479.
-
Ho, Y. S. (2006). Review of second-order models for adsorption systems, Journal of Hazardous Materials. Journal of Harzadous Materials, 136, 681- 689.
-
Ho, Y. S., & McKay, G. (1999). Pseudo second order model for sorption processes. Process Biochemistry,. Process Biochemistry, 34, 451-465.
-
Ho, Y. S., & Ofomaja, A. E. (2006). Biosorption thermodynamics of cadmium on coconut copra meal as biosorbent. Biochemicaingl Enigineer Journal, 30, 117-123.
-
Jimoh, O. T., Muriana, M., & Izuelumba, B. (2011). Sorption of Lead (II) and Copper (II) ions from Aqueous Solution by Acid Modified and Unmodified Gmelina Arborea (Verbenaceae) Leaves. Journal of Emerging Trends in Engineering and Applied Sciences, 2(5), 734-740.
-
Khaled, A., Nemr, A. E., Sikaily, A., & Abdelwahab, O. (2009). Treatment of artificial textile dye effluent containing Direct Yellow 12 by orange peel carbon. . ournal of Bioremediation Desalination, 238(1-3), 210-232.
-
Lagergren, S. (2009). About the theory of so-called adsorption of soluble substances,. Kungliga Svenska Vetenskapsakademiens, Handlingar, 24, 1- 39.
-
Mehrorang, G., Habibolah, K., Amin, H., Mostafa, R., & Alireza, A. (2012). Oxidized multiwalled carbon nanotubes as effluent adsorbents for bromothymol blue. Toxicology and Environmental Chemistry, 94(5), 873-883.
-
Mirdamadian, S., Emtiazi, G., Golabi, M., & Ghanavati, H. (2010). Biodegradation of Petroleum and Aromatic Hydrocarbons by Bacteria Isolated from Petroleum-Contaminated Soil. Journal of Petroleum Environ. Biotechol, 1(102), 2.
-
Muhammad, J., & Muhammad, N. (2010, march 26). Thermodynamics and kinetics of Adsorption of dyes from aqeous media unto Alumina. Journal Chemical Society of Pakistan, 32(4).
-
Jimoh O. T., & Izuelumba, B. (2011). Sorption of Lead (II) and Copper (II) ions from Aqueous Solution by Acid Modified and Unmodified Gmelina Arborea (Verbenaceae) Leaves. Journal of Emerging Trends in Engineering and Applied Sciences (JETEAS) 2(5), 2(5), 734-740.
-
Olajire, A., Abidemi, J., Lateef, A., & Benson, N. (2017). Adsorptive desulphurization of model oil by Ag nanoparticles- modified activated carbon prepared from brewer's spent grains. Journal of Environment Chemical Engineering, 5, 147-159.
-
Olayinka, O. K., Oyedeji, O. A., & Oyeyiola, O. A. (2009). Removal of chromium and nickel ions from aqueous solution by adsorption on modified coconut husk. African Journal of Environmental Science and Technology, 3(10), 286-293.
-
Rashed, M. (2013). Adsorption Technique for the Removal of Organic Pollutants from water and wastewater. Journal of Environmental Management
, 16, 167.
-
Shah, B., Shah, A., & ingh, R. R. (2009). Sorption isotherms and kinetics of chromium uptake from wastewater using natural sorbent material.
International Journal of Environmental Science and Technology, 6(1), 77-90.
-
Shen, D., Fan, J., Zhou, W., Gao, B., Yue, Q., & Kang, Q. (2009). Adsorption kinetics of isotherm of anionic dyes onto organo-bentonite from single and multi-solute system. Journal of Harzdous Materials, 172, 99-107.
-
Shilpi, A., Hardreza, S., Majid, M., Abdel, S., Gomaa, M., Amir, O., . . . Vinod, K. (2016). Efficient removal of toxic bromothymol blue and methylene blue from wastewater by polyvinyl alcohol. Journal of Molecular Liquids, 218, 191-197.
-
Tariku, A. (2016). Removal of reactive red dyes from textile wastewater by biosorption using mango and papaya seeds. Addis Ababa Institute of Technology, Environmental Engineering. Addis Ababa: Addis Ababa University.
-
Zamani, S., Sendi, J., & Ghadamyari, M. (2011). Effect of Artemisia Annua L(Asterales: Asteraceae) Essential Oil on Mortality, Development, Reproduction and Energy Reserves of Plodia Interpunctella(Hübner). Journal of Biofertility Biopestici, 2, 105.
-
Zhou, Y., Chen, L., Lu, P., Tang, X., & Lu, J. (2011). Removal of bisphenol A from aqueous solution using modified fibric peat as a novel biosorbent.
Separation Purification Technology, 81(2), 184-190.
-
Zhu, L., & Ma, J. (2008). Simultaneous removal of acid dye and cationic surfactant from water by bentonite in one-step process, Chem. Eng. Chemical Engineering Journal, 139(3), 503-509.
-
Zollinger, H. (2004). Color chemistrysynthesis, Properties and Application of Organic Dyes and Pigments. Angewandte Chemie International Journal, 43(40), 5291-5292.