
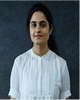
- Open Access
- Authors : Onkar Pathak , Shrutika Mukund Patil
- Paper ID : IJERTV9IS090271
- Volume & Issue : Volume 09, Issue 09 (September 2020)
- Published (First Online): 22-09-2020
- ISSN (Online) : 2278-0181
- Publisher Name : IJERT
- License:
This work is licensed under a Creative Commons Attribution 4.0 International License
How Significant is the Contribution of Quick Changeovers on Quality
Shrutika Mukund Patil
Lead Consultant, Kanzen Institute Asia Pacific Pvt Ltd
Mumbai, India
Onkar Arun Pathak
Operations Management, Welingkar Institute of Management Development and Research Mumbai, India
Abstract Quality is an uncompromisable factor for any product or service today. With initiatives like Zero Defect, Six Sigma there is a significant improvement in quality. These initiatives are well cashed by Global companies, but their awareness and implementation in Small Medium Enterprises has still miles to go. Quality issues hamper the productivity of an Organization. The rework created due these quality issues result in lower outputs, inefficient use of resources and increased lead time. The research studies that how the application of Quick Changeovers helps improve the Quality of a Small Medium Enterprise. The research objectifies that Small Medium Enterprises can apply Quick Changeovers in a very cost – effective manner and experience tremendous improvements in the Quality. The study has been performed with 100 Small Medium Enterprises where the impact of Quick Changeover has been observed, studied and measured with respect to the Quality.
Keywords Quality, Quick Changeovers, Productivity, Effectiveness, Efficiency, Startup defects, Adjustment Defects, Tool Maintenance, Material Wastage
-
INTRODUCTION
The Suppliers market guides the Small Medium Enterprises by the Equation: Profit = Price Cost. (Jaedicke, Robert K and Robichek, Alexander A, 1964). Price as one of the important factors is often dictated by the market. The only factor that organizations can control in order to improve their profits is Cost. The contribution of Quality to effective cost reduction has been significant. (Reitsperger). Organizations that have quality issues tend to minimize their own profits because a lot of the organizations valuable time is consumed by rework. This rework creates a compromise on the production quantity, increases delivery time, decreases operator efficiency, and increases the cost of the product. (Schiffauerova, 2006). Quality defects often create an everlasting impact on the reputation of the brand. Small Medium Enterprises deliver quality products to the final customers but there is a lot of internal rework that is required to deliver this assured quality. When the Toyota Production System was developed, quality was the foundational deliverable that the company believed in. Small Medium Enterprises often overlook quality over the production quantities. The organizational structure involves a production department and a Quality department. The performance of Production department is measured in terms of Quantity whereas the performance of the Quality Department is based on the Number of Defects prevented from reaching the customer. As we see there is a clear demarcation in the core deliverables of the organizations departments a lot of defects are created by the production to achieve numbers and are then
sent to the quality department. The quality department then checks the output and crafts out the pieces involved for rework. (Inderfurth*). Organizations need to understand that quality is the main deliverable of the production team followed after with quantity. Defects can be described quite elaborately but a few of the commonly known root causes for defects are because of Startup processes. (Hallem) Whenever the machine restarts after a brief stop- like because of maintenance, Changeovers, power failure etc. the machine has the tendency to produce defects for a first few pieces and then the defects tend to be eliminated. Another commonly occurring root cause for the generation of defects are the specification adjustments required in the machine (Karasu, 2014). These adjustments are predominantly common after changeovers where new product specifications need to incorporate. Tool maintenance also proves to be a very significant root cause for the generation of defects. (Lange, 1992). Quick Changeovers are inevitable today for the primary reason: Providing variety to the customer without holding unnecessary inventory. (Slechta, 2004) Small Medium Organizations fear changeovers because the time available for production is consumed and defects are common until the production with the new changeover has stabilized. (Milgrom, 1995). The concept of Quick Changeovers developed by the Toyota Production System not only enable quick changeovers but also ensure that quality is built into the products right from the source as soon as the quick changeover is implemented.
-
RESEARCH METHODOLOGY
-
Structuring Quality measurements with respect to four attributes as mentioned below;
-
Startup defects
-
Material Wastage
-
Adjustment defects
-
Tool maintenance
-
-
Statistical Formulation of Hypothesis
-
Designing Questionnaire for Small Medium Enterprises to analyze the impact of Quick Changeovers on the Quality
-
Data Collection for a Sample of 103 Small Medium Enterprises who have the business experience of implementing Quick Changeovers
-
Analysis through application of statistical tests
-
-
CONTRIBUTION OF QUICK CHANGE OVER To study the effect of the quick change over on the quality,
structured the quality measurements with respect to 4 attributes cited below;
-
Startup Defects
Start-up time is the time spent tweaking the equipment after it has been restarted. Quick Changeover reduces adjustments as a part of setup and promotes quality on the first piece right after the start-up of the equipment.
-
Material Wastage
Understanding the demand driven manufacturing and develop the relationship between demand and lot size with the effort through quick change over. This will reduce the excess inventory that helps to decrease the wastage of material because of the expiry of the shelf life of the material.
-
Adjustment Defects
Adjustment defects are the defects produced due to the long operation of adjustment of the equipment for example the fastening of screws. By minimizing the number of fasteners or by implementing quick release fasteners on the machine; by the use of LDT's or servos to assist with removals, replacements and adjustments. The use of tool-less changeovers when possible and the use of automated systems helps to achieve quick change overs and hence reduces the adjustment defects.
-
Tool Maintainenance
Ensuring all the tools are always ready and in perfect condition so that the maximization of equipment effectiveness helps to reduce the change over time effectively.
-
-
FORMULATION OF HYPOTHESIS AND TESTING The objective of this study is to test whether the quick changeover really contribute in quality improvement? If so, study the percentage improvement of the attributes structured
for quality measurement.
-
Hypothesis
: There is no significant contribution of quick change over on quality.
: There is a significant contribution of quick change over on the quality.
-
Analysis of the responses collected from SMEs
To study the impact of quick changeovers on the quality, designed a questionnaire in to two stages, primary stage is to understand the product portfolio of the organization and second stage is to know the results of improvement in the quality after implementation of quick changeovers. The
primary part of the questionnaire helped to understand the prodct portfolio of the organization, experience of the organization in implementation of lean, time consumed for changeover before and after implementation of quick changeover. The secondary part of the questionnaire deals with quality improvement measurement structured with the four attributes as mentioned. The secondary part helped to understand the contribution of quick changeover to reduce the startup defect, to reduce material wastage, to reduce adjustment defects and the effect of checklist prepared during quick changeovers improves the tool maintenance. The responses of 103 small medium enterprises has been recorded. The recorded responses were in the form of 5 point Likert scale as shown in the figure no. The Likert scale shows the level of agreement or disagreement from Strongly Disagree with point 1, Disagree with point 2,Neutral with point 3, Agree with point 4 and Strongly Agree with point 5. The level of agreement or disagreement is quantified in terms of percentage improvement in the quality measurements.
TABLE I. 5 POINT LIKERT SCALE
Level of Agreement or Disagreement
Point
Percentage Improvement
Strongly Disagree
1
0 %
Disagree
2
0% -5%
Neutral
3
5% -10%
Agree
4
10%-15%
Strongly Agree
5
Above 15%
The responses recorded from 103 small medium enterprises were filtered in terms percentage improvement of the four attributes that measures the quality. The graphical representation in the figure no 1 shows the responses of the SMEs. The quick change overs result in to the improvement of above 15% in 22% of the organizations, improvement in the quality by 10% to 15% in 44% organizations. 15% organizations says, there is a improvement in the quality by 5% – 10%. Where 9% of the organization feels that there was an improvement of 0% 5% in the quality. In 10% organizations, there was no significant improvement in the quality after implementation of the quick changeovers.
Fig. 2. SMEs Response Infographic
Fig. 3. SMEs Response for four Quality Measurement Attributes
-
Desciminant Function Analysis to test the formulated hypothesis statistically
Analysis Case Processing Summary:
Analysis Case processing table summarizes the analysis dataset in terms of valid and excluded cases. This summary justifies not only the reasons why SPSS might exclude an observation from the analysis, but also the number N and percent of cases falling into each category i.e. valid or one of the exclusions are presented. The Case processing summary table shows that, the total number of observations
(N) are 103 and there is no discriminating variable is missing or excluded. Hence, all of the observations in the dataset are 100% valid.
Group statistics:
The group statistics table presents the distribution of observations in to five groups within percentage improvement. The number of observations falling into each of the five groups. In this case, incorporated the default weight of 1 for each observation in the dataset, so the weighted number of observations in each group is equal to the unweighted number of observations in each group.
Function:
Table 3 indicates the first or second canonical linear discriminant function. The number of group variables are greater than the number of discriminant variables hence, the number of functions is equal to the number of discriminating
Variables. In this case, Quality parameter has four discriminating variables, so four functions are calculated. Each function acts as projections of the data onto a dimension that best separates or discriminates between the groups.
Eigenvalue:
The magnitude of the eigenvalues of the matrix product of the inverse of the within-group sums-of-squares and cross- product matrix and the between-groups sums-of-squares and cross-product matrix. These eigenvalues are related to the canonical correlations and describe how much discriminating ability a function possesses. The magnitudes of the eigenvalues are indicative of the functions discriminating abilities. The larger the eigenvalue is, the more amount of variance shared the linear combination of variables. In this test, Startup Defect function has the largest Eigen value of
-
and possess 92.2 % variance. Wilks Lambda:
Wilks Lambda is one of the multivariate statistic calculated by SPSS. It is the product of the values of [1 – (canonical correlation) ^2]. In this case, our canonical
correlations are 0.761, 0.294, 0.123 and 0.080, so the Wilks Lambda testing of first canonical correlations is (1-
0.761^2) × (1- 0.294^2) × (1- 0.123^2) × (1-
0.080 ^2) = 0.376. In this way the magnitudes of the Wilks Lambda for the remaining canonical correlations are 0.894, 0.979, and 0.994 respectively. Wilks' Lambda test is to test which variable contribute significance in discriminant function. The closer Wilks' lambda is to 0, the more the variable contributes to the Discriminant function. In this case, start up defect variable has magnitude closer to zero i.e. 0.376
as compared to other variables. Hence, we can conclude that startup defect variable contributes more in the discriminant function.
Significance:
Significance is the p-value associated with the Chi-square statistic of a given test. The null hypothesis that the quick change over has no significance contribution on the quality is
evaluated with regard to this p-value. For 95% confidence level, the p-value of startup defect variables is 0.00, thus the null hypothesis is rejected. The performed statistical test proves that the quick change over has a significant contribution on the quality and quick change over contributes majorly in preventing the startup defects.
TABLE II. ANALYSIS CASE PROCESSING
Unweighted Cases
N
Percent
Valid
103
100.0
Excluded
Missing or out-of-range group
codes
0
.0
At least one missing
discriminating variable
0
.0
Both missing or out-of-range
group codes and at least one missing discriminating variable
0
.0
Total
0
.0
Total
103
100.0
TABLE III. GROUP STATISTICS
PERCENT
IMPROVEMENT
Mean
Std. Deviation
Valid N (listwise)
Unweighted
Weighted
1.00
Startup defect
2.5000
.97183
10
10.000
Material wastage
3.3000
1.15950
10
10.000
Adjustment defect
3.2000
1.03280
10
10.000
Tool maintenance
3.6000
.96609
10
10.000
2.00
Startup defect
3.4444
.72648
9
9.000
Material wastage
3.3333
1.00000
9
9.000
Adjustment defect
3.6667
.86603
9
9.00
Tool maintenance
4.0000
1.00000
9
9.000
3.00
Startup defect
3.8125
.65511
16
16.000
Material wastage
3.6875
.70415
16
16.000
Adjustment defect
3.7500
.85635
16
16.000
Tool maintenance
3.9375
.57373
16
16.000
4.00
Startup defect
4.1111
.57296
45
45.000
Material wastage
4.2889
.50553
45
45.000
Adjustment defect
4.2222
.55958
45
45.000
Tool maintenance
4.1333
.89443
45
45.000
5.00
Startup defect
4.7826
.42174
23
23.000
Material wastage
4.7391
.54082
23
23.000
Adjustment defect
4.4348
.72777
23
23.000
Tool maintenance
4.5217
.59311
23
23.000
Total
Startup defect
4.0000
.87447
103
103.000
Material wastage
4.1165
.83197
103
103.000
Adjustment defect
4.0485
.80900
103
103.000
Tool maintenance
4.1262
.83642
103
103.000
Function
Eigenvalue
% of Variance
Cumulative %
Canonical Correlation
1
1.377(a)
92.2
92.2
.761
2
.095(a)
6.3
98.6
.294
3
.015(a)
1.0
99.6
.123
4
.006(a)
.4
100.0
.080
Function
Eigenvalue
% of Variance
Cumulative %
Canonical Correlation
1
1.377(a)
92.2
92.2
.761
2
.095(a)
6.3
98.6
.294
3
.015(a)
1.0
99.6
.123
4
.006(a)
.4
100.0
.080
TABLE IV. EIGEN VALUE
TABLE V. WILKS LAMBDA
Test of Function(s)
Wilks' Lambda
Chi-square
df
Sig.
1 through 4
.376
95.357
16
.000
2 through 4
.894
10.919
9
.281
3 through 4
.979
2.094
4
.718
4
.994
.619
1
.431
RESULTS AND DISCUSSION
To measure the effect of the quick change over on the quality, structured the quality measurements with respect to 4 attributes as shown in the table no 6. The significance value of the test is less than 0.05 so, we have rejected the null hypothesis and accepted the alternate hypothesis that, Quick change over has a significant contribution on quality. Statistical test interprets that Startup Defect function has the largest Eigen value of 1.337 and possess 92.2 % variance in Discriminant function. . In this case, start up defect variable has magnitude closer to zero i.e. 0.376 as compared to other variables. Hence, we can conclude that startup defect variable contributes more in the discriminant function.
TABLE VI. RESULTS
Variable
Eigen Value
Wilks Lambda
Sig.
Startup Defect
1.377
0.376
0.00
Material Wastage
0.095
0.894
0.281
Adjustment Defect
0.015
0.979
0.718
Tool Maintenance
0.06
0.994
0.431
CONCLUSION
This primary research made think about the unwavering contribution of the Quick changeover in evaluating the percentage improvement in the quality as well as the impact of
Quick Changeovers on startup defects, Material Wastage, Adjustment Defects and Tool maintenance. Discriminant
Function analysis was performed to evaluate the involvement of quick change over on the four listed attributes for quality Measurements. The result of this discriminant test summarized that, startup defects has the highest Eigen value with Wilks
Lambda value closer to zero compared with other variables. The p value of performed statistical test is 0.00, hence by rejecting the null hypothesis it is proved that the quick change over has a significant contribution on the quality and it contributes majorly in preventing the startup defects.
REFERENCES
-
Hallem, S. a. (n.d.). Uprooting software defects at the source. Queue.
-
Inderfurth*, K. a. (n.d.). Lot sizing in a production system with rework and product deterioration. International Journal of Production Research.
-
Jaedicke, Robert K and Robichek, Alexander A. (1964). Cost-volume- profit analysis under conditions of uncertainty. The Accounting Review, 39.
-
Karasu, M. K.-O. (2014). Improvement of changeover times via Taguchi empowered SMED/case study on injection molding production. Measurement.
-
Lange, K. a. (1992). Tool life and tool quality in bulk metal forming. CIRP annals.
-
Milgrom, P. a. (1995). Complementarities and fit strategy, structure, and organizational change in manufacturing. Journal of accounting and economics.
-
Reitsperger, W. D. (n.d.). Product quality and cost leadership: compatible strategies? MIR: Management International Review.
-
Schiffauerova, A. a. (2006). A review of research on cost of quality models and best practices. Emerald Group Publishing Limited.
-
Slechta, S. a. (2004). System and processes for performing quick changeovers on assembly lines.
-