
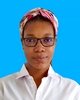
- Open Access
- Authors : Gbedevi Akouyo Yvette , Dr. Kevin Zhang , Tchaye-Kondi Jude
- Paper ID : IJERTV10IS080214
- Volume & Issue : Volume 10, Issue 08 (August 2021)
- Published (First Online): 03-09-2021
- ISSN (Online) : 2278-0181
- Publisher Name : IJERT
- License:
This work is licensed under a Creative Commons Attribution 4.0 International License
GELR: A Bilingual Ewe-English Corpus Building and Evaluation
Gbedevi Akouyo Yvette
School of Computer Science, Beijing Institute of Technology No. 5, South Street, Zhongguancun, Haidian District, Zip code: 100081 Beijing, China
Dr. Huaping Zhang
Big Data Search and Mining Lab Beijing Institute of Technology, No. 5, South Street, Zhongguancun, Haidian District, Zip code: 100081 Beijing, China
Tchaye-Kondi Jude
School of Computer Science Beijing Institute of Technology, No. 5, South Street, Zhongguancun, Haidian District, Zip code: 100081 Beijing, China
Abstract The availability of bilingual corpora greatly favors the progress of research in the linguistic field for popular languages such as English, French, Chinese, etc. but whereas under resources languages such as Ewe, are confronted with limited or nonexistent corpora. But setting up bilingual corpus for a language of turns out to be possible with online textual data in several languages. This paper discusses the process of setting up a bilingual Ewe-English corpus by the following stages: Data collection, Data preprocessing (manual or automatic), and Sentence Alignment with a quality of 96% on average. The quality of our corpus is assessed by building a Neural Machine Translation System through the Bahdanau Attention Mechanism for each pair of languages. Obviously, the results obtained do not fit into the performance of other Machine Translation systems, because having an efficient automatic translation system requires a bilingual corpus with very high resources, which is not the case with our corpus that we have created. Gayc Ewe Language Resources is made up of a 26K word Ewe dictionary, a 3K Bilingual dictionary and a well-aligned bilingual corpus of almost 43K sentences. The output format of our bilingual corpus is TMX.
KeywordsBilingual corpus; text alignment; computation linguistic; Ewe language
-
INTRODUCTION
Corpus is an invaluable resource for tasks in areas such as researching knowledge, machine learning, natural and linguistic language processing (Véronis, 2000). A text corpus is a very large collection of text (often several billion words) produced by real users of the language and employed to analyze the use of words, sentences of the language in general. Some corpora have a very well-defined scope, journalistic texts and biomedical for instance, while others have a broader objective.
There are many different kinds of corpora. They can contain written or spoken (transcribed) language, modern or old texts from one language or several languages. Corpora can consist of texts in one language only or of texts in more than one language. For texts that are the same in all languages after translation, the corpus is called parallel corpus. Our work focus on building a Ewe corpus essentially based on bilingual (i.e. English and Ewe) texts crawled from the web pages, books, articles, as there are few works already done on it.
A Machine Translation system is obviously based on the availability of bilingual corpora. The Machine Translation system is easier to set up for languages with enough digital resources, because the more digitally presented the language is, the higher the probability will be to have a very large bilingual corpus. Neuronal machine translation is a recently proposed approach to machine translation. Unlike traditional statistical machine translation, neural machine translation aims to build a unique neural network that can be tuned together to maximize translation performance. The models recently proposed for the automatic translation of neurons often belong to a family of encoder-decoders and encode a source sentence into a vector of fixed length from which a decoder generates a translation (Marathe, 2020).
To provide Ewe communities with tools that will increase the vitality and visibility of their language and support its use in a variety of contexts, as well as to help linguists in their efforts to learn more about human cognition study of linguistic diversity, the GELR (Gayc Ewe Language Resources) project was developed by using bilingual textual data to help in Natural language processing and linguistic documentation. To achieve this goal, a critical mass of textual data is required. In this paper, we describe the corpus created for the Ewe language and illustrate our method of evaluating our corpus. the previous work on bilingual corpus building is provided in section II. Section III, is about some basic information on the Ewe language building. Section IV, describes the data collection process and provides a detailed overview of the corpus. In the rest of the paper (Section V and section VI) is concentrated on the subset used for evaluation. And Section VII draws a conclusion of this study.
-
RELATED WORK
In terms of bilingual corpus, a lot of work has been done with satisfactory results, (Felipe & Martin, 2019), they worked on the establishment of a corpus of biomedical abstracts in English, Spanish, and Portuguese. Their corpus is based on the BVS database, which contains biomedical texts from several sources in Latin America and Carib. The corpus contains the English/Spanish, English/ Portuguese language pairs as well as a trilingual subset of English/Portuguese / Spanish sentences. They did a careful evaluation of their work through NMT experiments with OpenNMT system, presenting superior performance regarding BLUE score and manual evaluation.
Their work focuses on the BULBasaa corpus, a Bas`a`a-French bilingual corpus composed of both controlled (elicity) and uncontrolled (natural) speech. Although Bas`a`a was an under- language resources, but it presents basic grammatical properties fairly well documented, which allows the evaluation of linguistic documentation methods (Hamlaoui, et al., 2018). In this work they have described a set of techniques which have was developed during the parallel collection texts for the Russian-English language pair and have built a corpus of parallel sentences for formation of a statistical machine translation system. They discussed verification issues potential parallel texts and filtering documents automatically translated. Their evaluation leads to a quality of 1-millionentence corpus which, according to them, can be a useful resource for machine translation research. (Antonov & Misyurev, 2011)
Automatic translation is today effective and efficient thanks to this work especially the creation of the neural cell LSTM (Long Short-Term Memory) which offered the possibility of working with relatively long sequences, using a machine learning paradigm by (Sepp & Jurgen, 1997), as well as sequence-to-sequence work, based on LSTM (Sutskever, Vinyals, & V., 2014) and especially to the creation of the Attention Mechanism, which was first introduced by Bahdanau et al., 2015.
The aforementioned works show some achievements in the field of building bilingual corpus. But when we refer to our subject of research, we can observe that there has been no prior work in this context. And the fact of building our Ewe language resources for NLP usage will constitute a first for the Ewe Language Processing.
-
EWE LANGUAGE There are a variety of languages spoken around the world and
some of them are known as under-resourced languages because of the little work that is done on it in linguistic terms or because of its low usage rate unlike the English, Chinese, French or Russian language which have a large user community, such is the case of Ewe. In this section we provide the reader with a brief overview of the Ewe language.
Ewe is a language of wider communcation in Ghana where it is spoken by 2.25 million people as a first language and by another half a million people as a second language (Eberhard, 2020). The language is used in daily activities, such as in markets and in the areas of traditional culture and religion. It is taught in primary and secondary schools across the country. It is also used in print and electronic media. In Togo Ewe is spoken by 2 million people as a language of communication in Togo. It is the predominant language in the south of the country where it is taught in primary schools. It is used as a lingua franca by speakers of different languages in central Togo. It is also used on radio and television, as well as in newspapers. Ewe has three distinct dialects. Most of the differences between the dialects are related to phonology. All dialects are mutually intelligible. The written language is based on the Alo spoken along the coast between the mouth of the Volta and Lomé.
-
Western dialects, or Ewe itself, which include the Alo and highland varieties
-
Central dialects that include Watyi, G and Adya
-
Oriental dialects that include G, Fõ and Maxi Like any language, Ewe respects grammar rules and spelling.
Ewe is a tonal language and it meaning changes by tonal variations. It has a variety of peculiar consonants and diagraphs. Six of the letters in Ewe do not appear in western languages. The international encryption standards usually do not take in consideration these letters.
-
-
CORPUS BULDING
In this section, we describe the detail steps in developing our bilingual corpus. Figure 1 shows the diagram of the steps followed for the construction of the bilingual corpus.
Figure 1: Architecture og GAYC Ewe Language Resources Building
-
Textual Data Collection
Data collection was done thanks to the multitude of documents that can be accessed today on the web. The data collected comes from various sources such as:
-
Literary texts
Books published in electronic format are becoming more and more common. Although the vast majority are subject to copyright restrictions, some are not.
-
Religious Texts
The Bible has been translated into over 400 languages and other religions books are also very common. Many websites also provide much of their content in several languages.
-
International law
Important legal documents, such as the Universal Declaration of Human Rights, are available free of charge in many different languages.
-
Websites
Today there are several types of bilingual websites that are full of documents. This means that a web crawler can be pointed to these websites to retrieve all accessible pages.
-
-
Preprocessing of collected data
After collecting the data, we used a number of pre- treatment techniques. This phase is a succession of three steps, the result of each step will be used later. The three steps are performed on each of the data in Ewe and in English separately.
Table 1: Special letters in Ewe Alphabet
Writing
Pronunciations
[] [~] [] [] [] [] Ny ny
[] [] õ
[] First, we eliminated the special characters from each collection to simply get the text content (for example, delete smileys, etc.) and make sure that the special characters needed are in their place. Although the alphabet of the Ewe language is based on Latin, it has certain peculiarities with certain letters which are often misinterpreted by the computer. These letters are shown in Table 1.
Ewe is mainly a nasal tongue, hence the presence of the tilde which marks nasalization. In second position, we set up an Ewe-English dictionary by making use of MySQL with the words of the websites which we obtained thanks to crawling. Then we will proceed to text alignment. There is a various methodology for text alignment such as sentence alignment, word and expression alignment, clause and sentence structure alignment and structural alignment, but for our study we will choose sentence alignment.
There are several documented algorithms and tools available to perform sentence level alignment. They can be divided into three categories: based on length, based on a dictionary or lexicon, or based on partial similarity.
-
Text alignment
There are several types of alignment that can be done on textual data, namely document alignment, paragraph alignment, sentence alignment and word alignment. In our work we opted for the sentence alignment technique.
Generally, sentence aligners take as input the texts to be aligned and, in some cases, additional information, such as dictionaries, to help establish matches. A typical sentence alignment algorithm starts by calculating the alignment scores, trying to find the most reliable initial alignment points labeled "Anchor points". This score can be calculated based on the similarity in terms of length, words, lexicon or even syntax tree (Tiedemann, 2010). After finding the anchor points, the process is repeated, trying to align the midpoints. Typically, this ends when no new matches are found. Alignment is performed without allowing cross-matching, which means that the sentences in the source text must match in the same order the target text.
Table 2: Extract of Ewe and English Universal Declaration of Human Rights Sentences
EWE
ENGLISH
AMEGBET E ABLEVINYENYE U
KPEOZINYA KPKPLYIEME
Universal Declaration of Human
Rights Preamble
KPEODZINYA OO
1
Wodzi amegbetwo kata ableviwoe eye wodzena
bubu kple gomekpkp sse.
Article I
All human beings are born free and equal in dignity and rights.
KPEODZINYA OO 13-LIA 1.
Amesiame kp m ai tsa ayi duk esiae me, an afisiafi si dzroe la, gake amea nakp gb be yemetso duk si me yele la
e liowo o.
Article 13 1.
Everyone has the right to freedom of
movement and residence within the borders of each State.
Our sentence alignment is composed by three files: two plain text files containing Ewe text as the source text and English text as the target one. It contains a sequence of pairs [(s1, t1), (s2, t2), (s3, t3),, (sn, tn)]. Each si th , segment in the source text has its beginning position given by si, and the end position given by Si+1 – 1. The corresponding segment in the target text is delimited by ti and ti+1 -1.
For sentence alignment, we use the LF alignment tool, a wrapper around the Hunalign algorithm, which provides an easy-to-use and complete solution for sentence alignment, including preloaded dictionaries for several languages.
Hunalign uses sentence length information from Gale-Church to automatically create a dictionary based on this alignment. Once the dictionary is constructed, the algorithm realigns the input text in a second iteration, this time by combining the sentence length information with the dictionary.
Parallel summaries are provided to the aligner, who perform sentence segmentation followed by sentence alignment. After aligning the sentences, the following post-processing steps were performed: (i) deleting all non-aligned sentences; (ii) deletion of all sentences of less than three characters, as they are likely to make noise.
-
Output of GAYC Ewe Language Resources
The output of our corpus is in the form of a bilingual Ewe- English corpus in TX format with a creation date, creation id and text type, Ewe dictionary of more than 26K words, a bilingual Ewe-English dictionary of more than 3K words as well as annotated data for Ewe Part of Speech Tagging.
-
-
DESCRIPTION OF THE BUILT CORPUS
The textual resource constructed is a bilingual Ewe / English corpus composed of a total of 1185 texts collected mainly on the Holy Bible New World Translation texts and articles published on several websites especially the Jehovah's Witnesses1 site which is a real gold mine for the work of NLP because there are a multitude of publications in more than 300 languages and Jzyk Ewe – Ewe language – Eegbe2 a website for some Ewe texts collection (Djudjobgé, 2010). Most articles in the GELR corpus are open access documents. In order to avoid any copyright issues, we include in our datasets only open access documents.
-
EVALUATION
The last step in the process of creating parallel data qualified as a data set for a bilingual corpus is to ensure that the extracted sentences are aligned with the best possible quality.
High alignment quality is crucial for obtaining good SMT translation results. Bearing in mind that manual checking and automatic alignment assessment are time consuming, it is the alignments of identified parallel documents that are of the highest possible quality.
In (Toral, Poch, Pecina, & Thutmair, 2012), they have integrated a range of advanced technologies phrase and word aligners in the web service architecture. The state-of-the art sentence aligners such as Hunalign, GMA and BSA were evaluated.
Our experiment is processed in two steps, first step for manual evaluation and second step for neural machine translation. The dataset used is provided by the GELR and consist of 200 sentence pairs of Ewe and English for manual evaluation and 2000 sentence pairs for neural machine translation experiments. We have worked on a model that translated from Ewe to English.
-
Manual Evaluation
To assess the usefulness of our corpus, we ask some people with good knowledge of both Ewe and English to subsequently carry out a manual evaluation. Knowing that the Hunalign algorithm generally has good alignment between sentences, we also performed manual validation to assess the quality of the Phrases alignment. So, at random they selected 200 sentences, in the EE / EN dataset obtained after alignment. If the pair was completely aligned, we marked it as "Aligned"; if the pair was not completely aligned, for example due to segmentation errors, we considered it "partial"; otherwise,
1 www.jw.org
when the pair was not properly aligned, we considered it to be not aligned.
-
Qualitative Evaluation
Table 3: Sentence Alignment Qualitative Evaluation
Group of Text
Group 1
Group 2
Group 3
Group 4
Aligned
98.7%
98.7%
96.8%
92%
Unaligned
1.3%
1.22%
1.68%
6.6%
Invalid Pair
0%
0.01%
1.51%
1.1%
This part was carried out on all the aligned texts. We had to share in 4 groups all the texts for an effective alignment and also seeing that the tool used only authorizes a quota of 15K sentences per alignment (Table 2). Of all assessed sentences, 96% on average were correctly aligned, while 3% on average were not aligned due to their emptiness. The small percentage (1%) of invalid sentences is probably due to the use of the Hunalign algorithm with the dictionaries provided. Figure 2 shows the precision alignment for all batches of bilingual texts provided. The percentage of alignment is obtained by making the ratio between the number of aligned sentences and the total number of sentences contained in the target text.
Figure 2: Alignment Accuracy per batch of text
-
Machine Translation Evaluation
Before Machine Translation experiments, sentences were randomly divided into two disjoint data sets: training and validation. For the NMT experiment, we used the TensorFlow
2 (Marathe, 2020) (Tiedemann, 2010)
Figure 3: Attention Plot for Ewe-English Translation
implementation of Neural Machine Translation with Bahdanau Attention Mechanism on a
Keras model applying GRU Layer for the Encoder and the Decoder, with 1024 hidden unit and the word embedding of dimensions 256, on 100 epochs.
The Figure 3 present the Attention Plot for one sentence used as test for prediction.
Below, is the sentence translated by Our Neural Machine Translation model and presented with the Attention plot. We can notice that the translation proposed by our model is far different from the human translation.
Input: <start> nu ka tae amewo w a nu sia? <end> Human translation: Why do people do it?
Predicted translation: what is old brothers. <end>
The shades of yellow and green suggest higher weights of attention to the corresponding words in the source sequence in predicting the word of the target sequence.
Under-resource Machine Translation faces a problem of corpus size since Machine Translation System needs a couple of million sentences to outperform. Unfortunately, the result obtained is far from being good or close to the state-of-the-art results for neural machine translation. And this is just our first attempt to build such a kind Neural Machine Translation System from Ewe to English. Therefore, our model requires much more training and more data to arrive at an almost acceptable result.
-
Evaluated Bilingual Corpus Comparison
To make the comparison in relation to other bilingual corpora built from start to finish we opted for the English-Spanish corpus with more than 789,547 sentences and the English- Portuguese with 711,475 implemented by (Felipe & Martin,
2019). As well as for the English-Latvian from EU Bookshop corpus used by (Zaria, ikiforovs, & Skadi, 2015)
Table 4: Corpus comparison based on qualitative evaluation
Corpus
Qualitative Evaluation
English-Portuguese
95%
English-Spanish
96%
English-Latvian
98%
English-Ewe
96%
By comparing the quality of alignment of our corpus with other corpora, we can notice a satisfied result which corresponds to the average obtained by these corpora. In other words, almost 96% of the sentences of our corpus are well aligned.
-
-
CONCLUSION AND FUTURE WORKS
We built a parallel corpus from different textual data in Ewe and English. Our corpus is based on textual data from various sources such as literary, religious, international law and website which is full of texts in Ewe and English. The corpus contains the Ewe/English sentence pairs, Ewe dictionary and bilingual dictionary. We provided a manual evaluation of sentences regarding alignment quality, with average 96% of sentences correctly aligned and a Neural Machine Translation System using Badhanau Attention Mechanism.
For the future work we will keep on building our corpus by providing more data to make our corpus richer, more structured with a lot of annotated data to be used for work in Natural Language Processing such as Part-of-Speech Tagging, Named Entity Recognition, and building a better Machine Translation System than the current one etc…
ACKNOWLEDGMENT
This research work was funded by the Beijing Municipal Natural Science Foundation (Grant no. 4212026), National Science Foundation of China (Grant no. 61772075), and National Key Research and Development Project of China (Grant no. 2018YFC0832304). he authors are thankful to them for their financial support
This corpus is an open-source project and available on Kaggle on the following link. https://www.kaggle.com/yvicherita/ewe-language-corpus
REFERENCES
-
J. Véronis, "From the Rosetta stone to the information society : A survey of parallel text processing," pp. 1-24, 2000.
-
Y. Marathe, 24 April 2020. [Online]. Available: https://medium.com/analytics-vidhya/neural-machine-translation- using-bahdanau-attention-mechanism-d496c9be30c3.
-
S. Felipe and K. Martin, "BVS Corpus: A Multilingual Parallel Corpus of Biomedical Scientific Texts," arXiv:1905.01712v1 [cs.CL] 5 May 2019, 2019.
-
F. Hamlaoui, E.-M. Makasso, M. Muller, J. Engelmann, G. Adda, A. Waibel and S. Stuker, "BULBasaa: A Bilingual Bas` a`a-French Speech Corpus for the Evaluation of Language Documentation Tools," in Proceedings of the Eleventh International Conference on Language Resources and Evaluation (LREC 2018), Miyazaki, Japan, 2018.
-
A. Antonov and A. Misyurev, "Building a Web-based parallel corpus and filtering out machine translated text," in Proceedings of the 4th Workshop on Building and Using Comparable Corpora: Comparable Corpora and the Web, Portland, 2011.
-
H. Sepp and S. Jurgen, "LONG SHORT-TERM MEMORY," in Neural Computation 9(8):1735{1780, 1997.
-
I. Sutskever, O. Vinyals and L. Q. V., "Sequence to Sequence Learning with neural networks," in NIPS'14: Proceedings of the 27th International Conference on Neural Information Processing Systems, 2014.
-
D. M. G. F. S. C. D. F. Eberhard, " Ethnologue: Languages of the World.," Twenty-third edition. Dallas, Texas: SIL International., 2020. [Online]. Available: http://www.ethnologue.com.. [Accessed 2 March 2020].
-
J. Tiedemann, "Lingua-Align: An Experimental Toolbox for Automatic Tree-to-Tree Alignment," Proceedings of the Seventh International Conference on Language Resources and Evaluation (LREC'10), 2010.
-
A. K. Djudjobgé, "Texts," 2010. [Online]. Available: and Jzyk Ewe – Ewe language – Eegbe a website for some Ewe texts collection. [Accessed 2 March 2020].
-
A. Toral, M. Poch, P. Pecina and G. Thutmair, "Efficiency-based evaluation of aligners for industrial applications," Proceedings of the 16th EAMT Conference, 28-30 May 2012, Trento, Italy , 2012.
-
I. Zaria, P. ikiforovs and R. Skadi, "Word Alignment Based Parallel Corpora Evaluation and Cleaning Using Machine Learning Techniques," EAMT , 2015.