
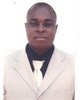
- Open Access
- Authors : E. Ubom , U. Ukommi , A. Ekanem
- Paper ID : IJERTV11IS070083
- Volume & Issue : Volume 11, Issue 07 (July 2022)
- Published (First Online): 26-07-2022
- ISSN (Online) : 2278-0181
- Publisher Name : IJERT
- License:
This work is licensed under a Creative Commons Attribution 4.0 International License
Effect of Site Characteristics on Calibration Quality of Indoor Positioning System
E. Uboma, U. Ukommia, A. J. Ekanema.
aDepartment of Electrical and Electronic Engineering, Akwa Ibom State University, Nigeria.
Abstract – The accuracy of an indoor Positioning System in determining the location of an asset depends on its quality of calibration; where sample measurements are taken to allow for a better accuracy on position calculation through measurements of the received signal-strength (RSS) and/or the signal-to-noise-ratio (SNR) between the asset and multiple setup access points (APs) to obtain the devices true position. This paper evaluates the effect of indoor environment characteristics in calibration quality of an indoor positioning system based on Ekahaus Real Time Location System (RTLS). Site surveys were conducted on selected test beds and the site maps were calibrated with the Ekahau site survey application. The different test environments presented different characteristics that affected their calibration quality which resulted in considerably high error in asset location positioning within the building. Findings showed that high blockages reduce the calibration quality of the indoor location system and emphasized on physical site surveys and empirical network planning exercises for congested warehouses.
Keywords: Indoor positioning, calibration, indoor asset location, environment characteristics.
1. INTRODUCTION
The growth of wireless networking has generated commercial and research interest in statistical methods to track people and things which is presently witnessing massive market demand and huge economic prospects [1], [2], and has been found very useful inside stores, hospitals, worship centres, warehouses, and factories, where Global Positioning System (GPS) devices generally do not work mainly because the GPS signals cannot provide indoor coverage as the signals are too weak to realize high precision and achieve positioning [3], [4].
The GPS however remains one of the most common and accepted technologies for outdoor positioning although it becomes infertile given its highly degraded or blocked satellite signal inside of buildings [5]. This is because GPS receivers need to see at least three (3) satellites which are relatively well distributed in the sky to calculate its 2D and 3D position. Hence in environments where the sky is blocked, positioning becomes difficult and in most cases impossible, making Indoor Positioning Systems (IPS) pioneered by [6] a ready alternative to provide the much needed accurate positioning required for indoor purposes. This service has been further enhanced by the proliferation of Wi-Fi wireless Internet access in cafes, college campuses, airports, hotels, playgrounds, seminar halls, market places, homes, museums, and libraries; where location estimates for wireless devices such as laptop computers, hand held devices, and electronic badges have
made human and material tracking achievable [7]. Its performance in an outdoor environment is also of interest as a Wi-Fi based positioning system can overcome the shortcomings of blockages associated with GPS. All these credits have given the indoor location systems the growing popularity they are having today.
During the last two decades, positioning techniques and technologies have been strongly researched and developed. In particular, real-time positioning systems have generated strong attention given the high impact they might have on the broad spectrum of applications where ubiquitous information and services are predominant [8].
II. LITERATURE REVIEWS
-
Wi-Fi Based Assets Localization Methods
The performance of WI-FI based IPS depends mostly on two factors namely; the number of the access points (APs) and the algorithm followed in the location positioning [9], the number of access points qualifies the coverage which defines the received signal strength (RSS) received between the target asset and the APs while the algorithm defines the steps applied to ensure the selection of the correct position of the asset, many of which have been proposed, example is the use of the Signal to Noise Ratio (SNR) in determining the data rate and distance from each AP [4]. The received signal strength (RSS) based approach is a simple and widely deployed approach for most indoor localization systems in the market today [10], [11], [12], [13], [14], and [15]. The RSS is usually measured in decibel-milliwatts (dBm) or milliWatts (mW) and is useful in the estimation of the distance between a transmitting device and a receiving device from the knowledge that the nearer the device the higher the RSS value. Since it is in an indoor environment, a simple propagation model can be used to determine the absolute distance between the APs and the target asset, an example of such model is found in [10] as
= 1010() + . (1)
This can be used to determine the absolute distance (d) in
meters, where is the pathloss in dB, A is the RSSI value
at a reference distance from the receiver defined as the
received signal strength indicator (a relative measurement of the RSS that has arbitrary units and is mostly defined by each chip vendor [16] at the target asset and n is the path loss exponent.
There are basically two classification of systems depending on the RSS as the means of determining the distance between the transmitter to the receiver, one of such group are the ones that makes use of dedicated infrastructures such as ultrasound emissions and the ultra-
wide band [17], and the other group adopt non-dedicated technologies like Bluetooth [18], radio frequency identification (RFID) [19] and wireless local area networks (WLAN) systems [20]. The non-dedicated technologies based systems are cheaper than the dedicated infrastructures although the dedicated infrastructure may provide enhanced performance but researchers say there might not be considerable difference in the services to compensate for the cost of the setup [21].
Using small battery powered Wi-Fi tags on the targeted assets, the location of the assets can be determined with proper calibration. Calibration being the process of carrying out multiple sample measurements between the transmitters and targets to allow for a better accuracy on position calculation based on propagation models and wireless sensor proximity based approaches. RSS calibration methods have been classified into deterministic and probabilistic approaches with the deterministic using deterministic inference methods, such as Triangulation and K-Nearest Neighbour averaging (KNN) [22] while the probabilistic approach rely on the likelihood of the target to be in a position if the RSS is of a given value [23] within its probability distribution.
Along with the RSS location method, many other methods
-
The Components of the Ekahau RTLS
The Ekahau RTLS solution for asset and people tracking consists of the following integrated components shown in Table 1, while Figure 1 shows its network design.
TABLE 1
EKAHUA SYSTEM COMPONENT LIST
Component |
Component Description and Use |
Ekahau Positioning Engine |
Server software for location tracking, Wi-Fi tag configuration and management. |
Ekahau Site Survey |
Application for creating and editing positioning models |
Ekahau Vision |
End-user application for grouping, locating and viewing the location of people and assets in real time, monitor events and invoke notifications and alarms. |
Ekahau Wi-Fi Tags |
Wi-Fi location tag for electronic identification |
Ekahau Positioning Client |
Software based solution to provide location tracking, capabilities for Wi-Fi enabled devices. |
TAG T301-A 192.168.10.3/24
have been proposed using the WI-FI system such as the inertial navigation systems [9], the signal heat map approach [24], the use of motion history information [25], and fingerprinting or Scene analysis [26]. This work attempts to
Channel 1 192.168.10.241/24
Channel 24
Channel 11
TAG T301-A 192.168.10.6/24
look at the calibration quality using the Ekahua kits in four locations with different characteristics at the University of Uyo in Nigeria.
-
METHODOLOGY
A. Brief Description of the Ekahau RTLS System
TAG T301-A 192.168.10.5/24
192.168.10.244/24
Cisco Switch
192.168.10.243/24
Ekahua Vision/Site Survey
The Ekahau Real-Time Location System is a wireless radio frequency (RF) solution that continually monitors and reports real-time locations of tracked resources. Ekahau's patented RF modeling technology leverages standard Wi-Fi access points, so no proprietary infrastructure is needed. By
TAG T301-A 192.168.10.7/24
Channel 2 192.168.10.242/24
192.168.10.250/24
VLAN 1
EPE 192.168.10.24/24
using standard 802.11 access points and Wi-Fi tags, any Wi-
Fi network can now work as the backbone and sensor network for a real-time location system. This is because wireless networks that are used for data and voice service can provide an excellent platform for building accurate location-tracking systems which relies on the standard
802.11 wireless networks to deliver a real-time location system [27]. This is very cost effective for those who already have an existing Wi-Fi network in place. Ekahau RTLS, helps in the identification of equipment positions and people wearing the tags or using mobile devices in the Wi-Fi network. This information can be readily updated automatically and can be delivered to other systems and personnel that require them. The fact that information about the needed equipment or persons is readily available leads to cost savings, because the assets can be utilized more efficiently and the workflow can be optimized. Services such as the automatic routing of security personnel to the location where a tag alarm button is pressed or a safety switch is activated are also incorporated into it.
Fig. 1. Network design for Ekahau RTLS test bed
-
Ekahua Equipment Setup Process
The process used in setting up the Ekahau RTLS consists of setting up the Cisco Access Point, general setup of the Cisco 2950 Switch, configuring the V-LAN, and then assigning IP addresses to the constituent devices, activation and association of the Tags, and the conduct of the site calibration.
-
Characteristics of the Indoor Test Beds
Four indoor sites were selected as test beds for this work namely; the Ground Floor Lecture Hall 3 (GD3), First Floor Lecture Hall 3 (FL3), Computer Laboratory and the Workshop; all located within the permanent site of the University of Uyo.
-
Characteristics of ground floor lecture hall 3 (GD3 Hall)
Fig. 2. Floor plan of GD3
Figure 2 shows the floor diagram of GD3 hall, it is a 12.02
x 8.73 2 ground floor hall with 8 ceiling fans, 6 fluorescent bulbs, white board and students desks and is located very
closed to the densely populated machine area of the Workshop. Being on the ground floor of a popular Campus GD/FL Block, there exist heavy movement of people, and remarkably high noise level by moving objects like vehicles.
-
Characteristics of first floor lecture hall 3 (FL3 Hall)
Fig. 3. Floor plan of FL 3
The floor plan of FL3 is shown in Figure 3 and it is a
11.04 x 9.01 2 hall and a student area although being on a first floor, it is not exposed to a high noise level such as
GD3 from moving vehicles, car horns and human traffic, but it also has 8 ceiling fans and 6 fluorescent lamps and not also closed to the workshop area.
-
Characteristics of the computer laboratory
Fig. 4. Floor plan of the Computer Laboratory
The computer laboratory is relatively far from heavy machines populated area (workshop) and also separated from the main halls and invariably exposed to less noise and
movements. It is 14.39 x 10.70 2 and also has 6 ceiling
fans, 6 fluorescent bulbs, and inverters, computers monitors
(CRT), a white board and voltage regulators. It is displayed in Figure 4.
-
Characteristics of the Engineering Workshop
Fig. 5. Site floor plan of the Engineering Workshop
The Engineering workshop is shown in Figure 5. It is a heavily loaded environment filled with machineries such as Lathe machines, Heavy wattage bulbs, and Guillotine shear machine, Drilling machine, Welding machines, Power Inverters, Metallic Vices, Iron Benders and many others. It
is 20.45 x 13.02 2 in size and has ceiling fans and some
fluorescent lightings too. It could be said to be the most
populated machine area in the campus although with
relatively low human traffic except for students carrying out their practical during the measurement drive.
-
Site Map Calibration Process
The floor plans were imported into the Ekahua Site Survey application and scaled to match the physical dimensions of the sites. The scaled site maps were calibrated and the site survey metrics were obtained.
This work deployed the fingerprinting method as supported by the Ekahua systems. The radio maps were created from the scaled (through physical measurements) floor plans drawn using 2D AutoCAD. The coverage and capacity requirements were set up, the WiFi adapters installed and AP list developed through network scanning. Passive (signal strength, SNR and ping test) and active (end- to-end connectivity- like latency, packet drops and AP roaming) survey parameters were measured and recorded to form the site map. Poor calibration was tested by switching off some AP and not covering the entire pixels in the map during the survey exercise to create survey points. Detail checks were then carried out and performance analysis measured by moving tags from place to place to confirm the quality of the calibration with the view gotten from the visualization tool of the software. Actual positions of items were compared to the offline positions of the items using well calibrated and with poorly calibrated maps. A result of an intentional poor calibration in the computer laboratory is as shown in Figure 10.
-
-
RESULTS AND DISCUSSIONS
-
Calibration Quality Analysis
A good calibration resulted into proper location of the tags to a high precision on the radio map, while a poor calibration lead to error in tag placement. The calibration exercise carried out using the Ekahua application for the different environment was as presented in section 4.B to section 4.E.
The legends used in indicating the quality of the calibration depicts a red colouration for low calibration quality, a yellow colour for a fair calibration and green colour for a high quality of calibration.
-
Calibration quality of ground floor lecture hall 3 (GD3)
Fig. 6. Calibration quality GD3
The map for GD3 in Figure 6 indicates more green fields showing the achievement of a high calibration in the survey carried out. Some of the areas tested showed a fair quality of calibration as indicated by the yellowish colourations.
-
Calibration quality of first floor lecture hall 3 (FL3)
Fig. 7. Calibration quality FL3
The calibration quality shown in Figure 7 for FL3 showed a remarkably good quality of calibration in the entire map which gives an indication of a high quality f the RSS in these areas.
-
Calibration quality for the Computer Laboratory
Fig. 8. Calibration quality Computer Laboratory
As shown in Figure 8, the two zones measured in the Computer Laboratory showed a considerably high calibration quality with poor calibrations recorded at the
fringes of the measure space. The visible purple colour is a result of the superimposed colours at the zones.
-
Engineering Workshop calibration quality
Fig. 9. Calibration Quality Workshop
The map in Figure 9 shows the calibration quality of the signals captured in the workshop; it has many areas with red colours interpreting low calibration in those areas. Most of the fields coloured red are restricted areas especially the rectangular portion (Welding Bay) at the top-left corner guided to prevent accidents to operators. Less movement of people takes place at these areas; some are no walk-throughs with serious obstruction to the WiFi signals. Tags that fall within the green portion will have better location accuracy on radio-map while those within the red areas will experience poor location accuracy. Unlike the other three test beds, The Engineering Workshop is prone to electromagnetic interference (EMI) and signal obstructions having different machining processes running as at the time of the measurement.
-
Effects of a poorly-calibrated map on asset tracking
Although Wi-Fi systems were not developed for positioning, its radio signals is being used for location estimation by understanding the relationship between the Received Signal Strength (RSS) and distance, this gives adequate predictions as regards the position of a target asset like notebook computers, smartphones and personal digital assistants (PDA) or any device or persons wearing a Wi-Fi enabled mobile devices generally referred to as Tags. Tags are placed on devices to track them and the accuracy of such asset location greatly depends on the calibration quality. Location fingerprinting is considered to be more reliable than dependent only on the RSS as there may be situations where there exist a change in the propagation characteristics of the environment as a result of many factors such as human movement, increase generated noise floor or as a result of reflection, diffraction and scattering of the signals hitting on objects. Hence fingerprinting deploy the use of a radio map which is constructed by measuring the RSS at a number of known locations called calibration points and
comparing the measured RSS of the target with the radio map to estimate target location.
Fig. 10. Effect of a Poorly-Calibrated Map
A poorly calibrated map therefore will provide a wrong benchmarking to the RSS and results in error in the prediction of a target location. This was confirmed with a deployment in the computer laboratory test bed. A poor calibration was carried out and used to estimate the position of a tag as shown in Figure 10. The position of the tag was wrongly estimated due to poor calibration deliberately caused by introducing reflectors on the benches which were later removed after the calibration. Figure 9 also shows the radio map of the workshop whose coverage was affected badly due to blockages of the signal path by the machines. Items places in areas with poor coverage indicated by the red colouration will be difficult to correctly be detected and be located.
-
-
CONCLUSION
As the use of indoor positioning system continues to grow wider since the GPS cannot provide the indoor users with the positioning accuracy they need for room-level tracking as Global Navigation Satellite Systems (GNSS) services are hardly ever available indoors due to the inability of satellite signals to penetrate walls and roofs, there is great need to evaluate the best methods of providing good accuracy in the real time estimation of asset location using the most affordable method of Wi-Fi calibration. This work looked at the importance of good calibration and the effects of some interfering sources in calibration quality and using the four testbeds studied, the first three (3) testbeds of GD3, FL3 and the computer laboratory exhibited better accuracy as the calibrated radio map showed better performance. This was
not the case with the heavily blocked multipath area of the workshop which had poor performance. These results confirmed that tags perform best in open environments where there is not much distortion in the propagation of RF signals [28] and therefore suggest a more detailed network coverage planning in heavily congested and EMI prone areas.
REFERENCES
[1] Cao, H., Wang, Y., Bi, J., Xu, S., Si, M., and Qi, H. Indoor Positioning Method Using WiFi RTT Based on LOS Identification and Range Calibration, ISPRS International Journal of Geo-Information, Vol. 9, 0627, 2020, pp 2-19
[2] Liu, H., Darabi, H., Banerjee, P., and Liu, J. Survey of wireless indoor positioning techniques and systems, IEEE Transactions on Systems, Man and Cybernetics – Part C: Applications and Reviews, Vol 37, No. 6, 2007, pp 10671080. [3] Olesen, D.M. Evaluation of GPS/BDS indoor positioning performance and enhancement Advances in Space Research, Volume 59, No. 3, 2017, pp 870876. [4] Esposito, C., Cotroneo D., and Ficco, M., "Calibrating RSS-Based Indoor Positioning Systems", IEEE International Conference on Wireless and Mobile Computing, Networking and Communications, Marrakech, Morocco, 12-14 October 2009, pp. 1-6, doi:10.1109/WiMob.2009.11. [5] Vera, R., Ochoa, S. F., Aldunate, R. G., EDIPS: an Easy to deploy Indoor Positioning System to support loosely coupled mobile work, Personal and Ubiquitous Computing, Volume 15, No. 4, 2011 pp 365 375. [6] Bahl, P., Padmanabhan, V.N. RADAR: An In-building RF-Based User Location and Tracking System, In Proceedings of the IEEE 9th Annual Joint Conference of the IEEE Computer and Communications Societies, Tel Aviv, Israel, 2630 March 2000; pp. 775784. [7] D. Madigan, D., Einahrawy, E., Martin, R. P., Ju, W., Krishnan P. and Krishnakumar, A. S., "Bayesian indoor positioning systems," Proceedings of the 24th Annual Joint Conference of the IEEE Computer and Communications Societies, Miami, FL, USA, vol. 2, 13-17 March 2005, pp. 1217-1227, doi:10.1109/INFCOM.2005.1498348.
[8] OConnor, A.C., Gallaher, M.P., Clark-Sutton, K., Lapidus, D.,Oliver, Z.T., Scott, T.J., Wood, D.W., Gonzalez, M.A., Brown, E.G., and Fletcher, J. Economic Benefits of the Global Positioning System (GPS) RTI Report Number 0215471, June 2019, sponsored by the National Institute of Standards and Technology, Research Triangle Park, NC: RTI International.
[9] Gao, Y., Yang, G., Li, G., Chang, E. Y., Wang, D., Wang, C., Qu, H., Dong, P., Zhang, F., XINS: the anatomy of an indoor positioning and navigation architecture, in Proceedings of the 1st International Workshop on Mobile Location-Based Service (MLBS 11), pp. 4150, Beijing, China, September 2011, https://doi.org/10.1145/2025876.2025884. [10] Kumar, P., Reddy L., and Varma, S., "Distance measurement and error estimation scheme for RSSI based localization in Wireless Sensor Networks," Fifth International Conference on Wireless Communication and Sensor Networks (WCSN), 15 19 December 2009, pp. 1-4, in Allahabad, India. doi: 10.1109/WCSN.2009.5434802 [11] Yang, Z., Zhou, Z., and Liu, Y., From RSSI to CSI: Indoor localization via channel response, ACM Computing Surveys (CSUR), vol. 46, no. 2, Article 25 p-32, 2013, DOI:http://dx.doi.org/10.1145/2543581.2543592
[12] Ladd, A. M., Bekris, K. E., Rudys, A., Kavraki, L. E. and Wallach,D. S., Robotics-based location sensing using wireless ethernet, Wireless Networks, vol. 11, no. 1-2, 2005, pp. 189204.
[13] Song, X., Fan, X., Xiang, C., Ye, Q., Liu, L., Wang, Z., He, X.,Yang, N., Fang, G., "A Novel Convolutional Neural Network Based Indoor Localization Framework With WiFi Fingerprinting," n IEEE Access, vol. 7, 2019, pp. 110698-110709, doi: 10.1109/ACCESS.2019.2933921.
[14] Sun, W., Xue, M., Yu, H., Tang H., and Lin, A., "Augmentation of Fingerprints for Indoor WiFi Localization Based on Gaussian Process Regression," in IEEE Transactions on Vehicular Technology, vol. 67, no. 11, Nov. 2018, pp. 10896-10905, doi: 10.1109/TVT.2018.2870160 [15] Zhang, J., Lyu, Y., Patton, J., Periaswamy S. C. G. and Roppel, T., "BFVP: A Probabilistic UHF RFID Tag Localization Algorithm Using Bayesian Filter and a Variable Power RFID Model," in IEEE Transactions on Industrial Electronics, vol. 65, no. 10, Oct. 2018, pp. 8250-8259, doi: 10.1109/TIE.2018.2803720 [16] Zafari, F., Gkelias, A., Leung, K. K., "A Survey of Indoor Localization Systems and Technologies", Communications Surveys& Tutorials IEEE, vol. 21, no. 3, 2019, pp. 2568-2599,
arXiv:1709.01015v3 [cs.NI] 16 Jan 2019.
[17] Niculescu D., and Nath, B., Ad hoc positioning system (aps) using AOA, in Procedure of the 22th annual Joint Conference of the IEEE Computer and Communications Societies, San Franciso, CA, USA, March 30 – April 3, 2003., pp. 17341743. IEEE Computer Society2003, ISBN 0-7803-7752-4.
[18] Topak, F., Pekericli, M.K., and Tanyer, A. M. Technological Viability Assessment of Bluetooth Low Energy Technology for Indoor Localization, Journal of Computing in Civil Engineering 2018, volume 32, issue 5. https://doi.org/10.1061/(ASCE)CP.1943-5487.0000778
[19] Zhang, D., Yang, L. T., Chen, M., Zhao, S., Guo M. and Zhang, Y. "Real-Time Locating Systems Using Active RFID for Internet of Things," in IEEE Systems Journal, vol. 10, no. 3, September 2016, pp. 1226-1235, doi: 10.1109/JSYST.2014.2346625 [20] Hills, A., Schlegel, J., and Jenkins, B. Estimating signal strengths in the design of an indoor wireless network, in IEEE Transactions on Wireless Communications, vol. 3, no. 1, January 2004, pp. 1719. [21] Hazas, M., Scott, J., and Krumm, J. Location-aware computing comes of age, in IEEE Computer magazine, vol. 37, no. 2, Feb. 2004, pp. 9597. [22] Liu, H., Darabi, H., Banerjee, P., and Liu, J., Survey of wireless indoor positioning techniques and systems, IEEE Transactions on Systems, Man, and Cybernetics, Part C (Applications and Reviews), vol. 37, no. 6, 2007, pp. 10671080. [23] Zafari, F., Papapanagiotou, I. and Christidis, K. Microlocation for Internet-of-Things-Equipped Smart Buildings, IEEE Internet of Things Journal, vol. 3, no. 1, 2016, pp. 96112. [24] Cypriani, M., Lassabe, F., Canalda, P., and Spies F. Wi-Fi-based indoor positioning: Basic techniques, hybrid algorithms and open software platform. In IPIN 2010, IEEE, 2010. International Conference on Indoor Positioning and Indoor Navigation. Zurich, Switzerland, Sep 15 – Sep 17, 2010. Pp 110. IEEE Xplore DOI: 10.1109/IPIN.2010.5648232. [25] Lassabe, F., Canalda, P., Chatonnay, P. and Spies, F. Indoor Wi-Fi positioning: techniques and systems. Annals of telecommunications, Volume 64, No. 9, 2009, pp 651664. [26] Youssef M. and Agrawala, A. The horus WLAN location determination system, Wireless Network 14, ,2008, pp 357374. https://doi.org/10.1007/s11276-006-0725-7 [27] Ekahau Incorporation (RFID-over-Wi-Fi) Delivering Business Intelligence through Location 2009. [28] P. M. Asuquo, K. M. Udofia, Performance Evaluation of Ekahau RTLS in Indoor Environments, IOSR Journal of Computer Engineering (IOSR-JCE) e-ISSN: 2278-0661, ISSN: 2278-8727,Volume 20, Issue 4, Ver. II (Jul – Aug 2018), PP 12-20.