
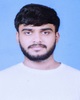
- Open Access
- Authors : Riteek Behera , Omkar Wadekar , Aditya K Sasmal
- Paper ID : IJERTV11IS030126
- Volume & Issue : Volume 11, Issue 03 (March 2022)
- Published (First Online): 29-03-2022
- ISSN (Online) : 2278-0181
- Publisher Name : IJERT
- License:
This work is licensed under a Creative Commons Attribution 4.0 International License
Development of Supply Chain Management using Big Data Analytics
Riteek Behera1, Omkar Wadekar2, Aditya Sasmal3
Department of Information Technology
Vidyalankar Institute of Technology – Vidyalankar Marg, Wadala (East), Mumbai-400037, India
AbstractBig Data Analytics offers a wide range of possibilities in todays business transformation. While big data has exceptionally magnetized the attention of both practitioners and researchers, especially in the marketing sectors and financial services, is a heap of recommendations that big data analysis can assume more vital parts in Supply Chain Management (SCM). This paper proposes to investigate these suggestions. The examination ranges from the fundamentals of sizably voluminous data analytics, its taxonomy, and the caliber of competency of sizably voluminous data analytics solutions in each of them, to implementation concerns and best systems. Big Data sanctions more elaborate supply chains. Surely, Big Data has the potential to transform supply chain dynamics. In this paper, we analyze Big Data use, opportunities, and challenges in the different processes of the Supply Chain Operation Reference (SCOR) model. Determinately, some incipient examples of advanced analytics applications will be offered as a way of unveiling some of the relatively unexplored boundaries in big data analytics research [4].
KeywordsAnalytics, Big Data, Business Transformation, Data Science, Predictive Analytics, Supply Chain Management, SCOR Model.
-
INTRODUCTION
Significant business players who adopted big data as an incipient model have offered illimitable promises of business transformation and operational efficiency amendments, particularly in supply chain management. A few delineations stand out enough to be noticed by both practitioners and researchers. AMAZON uses big data, generally to screen, track and secure 1.5 billion items in its inventory that is laying around at 200 fulfillment centers across the world, then relies on predictive analytics'' to predict when customers will purchase a product and re-ship it to the most proximate depot to the final destination which is called anticipatory shipping. Wal-Mart handles huge customer data every hour and imports it into databases that have more than 2.5 petabytes, and they ask the suppliers to tag shipments with a Radio Frequency Identification system (RFID) that engenders 100 to 1000 times the data of conventional bar code systems. PS deployment of telematics in their freight segment availed in their ecumenical redesign of logistical networks. SCM organizations have such an excess of data that McAfee and Brynjolfsson (2012) announced: "organizations gather a larger amount of data than they know how to manage". This is viewed as a benchmark for warehouse data management, marketing, or transport. Nonetheless, authenticity reveals that these cases are not just anecdotes of prosperity, they are the face of a
vicissitude where failure to acclimate could mean irrelevance.
While many organizations have high prospects from Big Data Analytics (BDA) in their supply chain, the authentic use is circumscribed, and many firms struggle to unveil its business value (Pearson et al.2014) [4]. In the pursuit to change what is happening and a readiness to direct the SCM practice to gain on BDA, this research intends to fill the information hole between the data science and supply chain management domain, it is all about the linkage between data, technology, and functional erudition in BDA and procurement, transport, warehouse operations, and marketing.
In the current scenario, the competition is shifting from Firm versus Firm to Supply Chain versus Supply Chain perspective [13]. So, the ability to optimize supply chains is the priority for companies to have a competitive advantage. Focus on supply chain management made companies rethink their competitive strategies so they incline, perforce towards collaboration with exchanging a plethora of data. The use of big data offers essential value in such regions as product development, market demand predictions, supplying decisions, distribution optimization, and customer feedback [7].
This paper will redefine what BDA betokens with regards to SCM and how it contrasts and advanced from previous analytical technology, foster a scientific categorization of big data inside SCM that distinguishes and consigns the various sources and kinds of information appearing in current supply chains, and recommend a few utilizations of
BDA and show potential high qualities this technology offers to solve complex problem in SCM. In the principal segment and the later segment, we present the Supply Chain Operations Reference (SCOR) model as the standard diagnostic implement for supply chain management.
-
BIG DATA
The term big data was first used in 1997 by NASA researchers to allude to the visualization challenge for a computer system with huge data sets. From that point forward, scientists and experts in information management have been interested and it has turned into a peculiarity in various areas. Big data has a positive impact in sundry domains such as supply chains, incrementing sales, managing customer allegiance in marketing and perfecting real-time routes, abbreviating costs in transport, minimizing risk in finance. Big data covers five dimensions: velocity, volume, variety, veracity, and value. The coordination sector is ideally placed from the methodological advancement of big data. Big data is initially driven by supply chain management and services such as finance, manufacturing, information technology. Recently, Auto-ID technology has been used in the supply chain. Big data additionally brings an incipient source of competitive advantages for coordination involvers to carry out supply chain management to obtain enhanced visibility, that too adjust under demand and ability fluctuations on a real-time substratum. The Council of Supply Chain Management Professionals (CSCMP) is presently pursuing two correlative projects, which intend to address how big data affects coordination and supply chain management.
-
SUPPLY CHAIN MANAGEMENT (SCM) Supply chain members are interconnected by a paramount physical flow that incorporates raw materials, work in process inventories, finished products, returned items, information flows, and financial flows [8]. In todays competitive and globalized business climate, manufacturers have been exploring innovative technologies and methodologies to carry out and support competitive advantage. One of the procedures which have wide acknowledgment and acquiescence among academicians and specialists is Supply Chain Management. Managing the incrementing involution in supply chains is compulsory for companies to compete better in the global market. The multifaceted nature of supply chains is related to material and data streams between supply chain partners.
-
SUPPLY CHAIN COMPLEXITY
Intricacies in the supply chain are related to material and data streams between store network accomplices. Generally, these streams are sorted out successively from provider to client. Today, data streams don't pursue this straight shape. Data streams rather now resemble a synchronous trade, particularly through electronic trades between all production network accomplices.
A store network forms many parts or components of various kinds, which are connected straightforwardly or by implication. These different components and their interrelationships are eminent for the multifaceted nature
happening in a framework. There are some key attributes of mulifaceted nature happening in a production network framework, which should be talked about to understand the effect of these qualities on the event of intricacy.
We summarize them as follows:
-
Number of components: Products, processes, supply chain partners, relationships, interactions, goals, location [4].
-
Diversity: Cognate to the homogeneity or heterogeneity of a system.
-
Interdependency: Interdependence between items, products, and supply chain partners. Unpredictability expands to the direct extent to the incrementation of reliance.
-
Variety: Variety addresses the dynamical conduct of a system.
-
Uncertainty: Uncertainty is all difficulties to be able to take a clear picture of a system due to the lack of information or knowledge. The more uncertainty in a supply chain system the more intricacy occurs in this system.
There are internet works in the firm with a concurrent trade between all units. Indeed, the rise of data and communications technology eased exchange between all partners engendered a change in the organization of the supply chain flows.
-
-
SCOR:
Demonstrated by displaying coordination frameworks, Supply Chain Operation Reference (SCOR) will make regular dialects for performers in the store network and orchestrate their practices. It lays an instrument to analyze the business stream between a company's first and second- level clients and providers. The SCOR display offers clients the accompanying advantages: execution standard measurements to depict process execution and characterize key objectives, process-standard portrayals of the board procedures, and process connections. SCOR display depends on five fundamental administration forms:
-
Plan: Processes include amassing customer requisites, amassing information on available resources, and balancing requisites and resources to decide orchestrated capabilities and resource gaps.
-
Source: Processes describe the injunctively authorizing and receipt of goods and services.
-
Make: Processes depict the activities related to the transformation of materials or the causing of the substance for facilities.
-
Deliver: Processes depict the activities related to the incited, support, and satisfaction of client orders.
-
Return: Process depicts the activities related to the reversal flow of goods back from the customer.
-
-
RESEARCH METHODOLOGY
The leading examination in SCM using many strategies guarantees that differences are quality-related and not strategy-related, and additionally the way that every approach is progressively fitting for the improvement of a specific phase of the exploration. To manufacture a meaning of BDA and its related rundown of topics, the first segment of the examination was tied in with comprehension of BDA in its terms. Like most of the zones near Big Data, BDA significance is mostly what individuals have made of it. The orderly writing audit changed a wide range of documentation first into a delimited arrangement of topics, and after that incorporated into separated information. The investigation of the subjects structure was brought about by thorough portrayal of its highlights, explicitly in the SCM setting, and created a sturdy base of discernment and considerable legitimization on which the resulting periods of the research were constructed. The incorporation of the contextual investigation in this work was to keep up common sense at the center. Contextual analyses explored synchronous BDA precedents, ordinarily in developing practices, in this way being an effective method for including the most recent patterns found in the business. Both business cases from the writing and those detailed through semi-organized meetings with experts at a reputed counselling organization in the UK were used. The joint methodical writing audit and contextual investigations were used to make a toolset that depends on scholarly sources and additionally common-sense experience that was useful and helpful to use.
-
The Next Step: Make Big Data Actionable: The ability to get to and break down the correct data is just the start. Utilizing Business Intelligence (BI) devices and detailing can help make responding to operational concerns significantly more noteworthy. What makes information genuinely significant is how an association
uses it to drive important change and make a lithe store network. Here are a couple of approaches to using the information you gather inside your store network tasks.
-
Better Transportation Planning: The present propelled coordinations innovation helps track significant information and use it to enhance the stream of products all through the whole inventory network. For instance, a Transportation Executive's Framework can give exact inbound and outbound measurements to follow shipment recurrence, recognize endpoint goals, and substantially more. This far-reaching picture can help envision future delivery designs, secure fundamental limits, and guarantee ideal stock dimensions.
-
Enhance End-to-End Visibility: A proficient production network must track and follow the conclusion-to-end development of merchandise. Information from a Transport Management System (TMS) can help with constant move directing or following the area of every shipment and, in case of anomalies amid a multi-stop conveyance, continuous load areas can help empower course change by abstaining from paying late punishments or having a driver come up short on hours. This is particularly valuable while building predictable moves. Thinking about the potential on the way delays ahead of time enables shippers to make course deviation designs before the conveyance or get is affected. Quite different from the times of trusting that the client will reveal to you that their conveyance has not arrived.
-
Advanced Planning and Forecasting: Preparing in advance is necessary for fruitful inventory network tasks. Utilizing information for arrangement ahead of time can help envision what may happen in the future. Directing "considering the possibility that" situations can enable associations to alleviate hazards when interruptions appear, for example, bad climate or a limit lack. Strong inventory network information ought to be used to figure out future effects and imperatives. A few shippers venture to impart this information to their transportation suppliers so they can start situating gear and drivers in front of the interest. This is a significantly more proactive and key method than "dialing" for everyday limits.
-
Improved Capacity Planning: Progressed investigation can help make an ideal system amid the acquirement and offering process. The ability to figure out these exercises can help bring down bearer costs and, whenever taken care of accurately, oversee varieties. To get the ideal outcomes, it is incumbent upon shippers to offer bundles that have consistent circles, visits, and multi-stop developments. By using arrangements ahead of time situations, shippers can prove the future for transporters to offer versus static, verifiable path information. Take a gander at your association's estimate for signs that there will be volume increments at a given plant or circulation focus (DC) and after that display the effect for your transportation suppliers to see
how your system will adjust. They will be amazingly energetic about any premonition you can give.
-
Improve Customer Service: Information investigation considers straightforwardness with clients, helping
Also, using ability of a 3PL accomplice can enable shippers to gather further experiences into their production network. Blending profound industry aptitude and bits of knowledge about limits, courses, climate conditions and more with huge information can more readily help approve, envision, and foresee future results. Sharing best practics for examination is a key to keeping everybody pushing ahead and considering approaches to progress. This helps shippers more viably using the information they need to streamline their transportation procedures, increment effectiveness, decrease costs, upgrade client benefit and enhance transporter connections.
VIII. DATA SYNTHESIS FOR BDA IN SCM
The given figure shows a Kamada-Karwai network and their distance forces between the 52 data sources and each of the 4 SCM Levers. All those data sources which are linked to only one level are present in the periphery. And those data sources which are equipollent associated with the whole supply chain are present in the core. Most of the data sources which are present in the periphery having high calibers of symmetry decide data sets with incidence only one of the four SCM functions or then again having a much solid sodality with one of the four SCM switches, without being used across the entire SCM venture. Several data sources can be assembled, for instance, location information between marketing and transportation or data shipment orders between procurement and transportation. However, most of the sources are eased by a single domain. In propitious conditions, it is not indispensable that all the sources would share the same degree of incidence across the whole SCM levers. All the types of data have a categorical area where they are more serviceable, but many datasets prefer only one
them gain understanding into any changes of expense or administration. Furthermore, using prescient examination to computerize forms consider enhanced arrangement setting and conveyance following, guaranteeing that the end client gets items on time.
VII. PUT DATA TO WORK FOR YOU
Nobody will contend that information entrance can significantly affect an association. In any case, getting to and using information isn't as straightforward as one may think. It's imperative to have a vital way to deal with enormous amount of information and mindfully outline an arrangement for getting to and using it. Basic advances incorporate setting up Key Performance Indicators (KPIs), actualizing explicit aims, and recognizing what should be practiced with the data being assembled. Effective associations approach this test with the mentality of the assignment being a development versus unrest. At that point try to hover back, contrast last sending outcomes and starting aims and KPIs, roll out vital improvements pushing ahead aims and recognize what should be practiced with the data being assembled. Effective associations approach this test with the mentality of the assignment being a development versus unrest. At that point try to hover back, contract last sending outcomes and starting aims and KPIs and roll out vital improvements pushing ahead.
area (52%). In most cases, the area which the data prefer is the area in which they are first engendered and the credence that amongst a sizable fraction would integrate value in other areas, and this makes us suspect systemic data silos. This engenders a boundary for BDA execution because of the underlined significance of aggregated layers of data from multiple sources to upgrade the predictive capabilities of these models. For instance, an acquisition division that has administrative motivating forces to apply a BDA model which screens raw matter evaluation, to foresee the best chance to buy at a low in the market. If they don't include in their model in-transit inventory or inventory costs associated with the final products, using the raw materials when there are enough final products in stock, even at low prices, can be favorable for the company by creating higher inventories and pushing costs downstream.
Research by DellAnno and Dukatz found many different leveraged data sources unlock value by promoting data connections and gaining actionable insights quickly [4]. Addressing key difficulties on the development, processing, and interactivity of data will help organizations to achieve the modern data supply chain. Daugherty et Al (2014) detailed that just one out of five associations incorporate their information across the venture. To amend the situation, they presented a model of data intelligent transportation throughout the organization, and this will break down the data silos, for the most part, assembled and had by a single department, and enable data to flow freely for the benefit of the entire organization.
FINDING 3
SCM Big Data consists of bigger data storehouses that are dispersed between business abilities and outside sources, are essentially not interconnected, and don't supply end-to-end
visibility of SCM. As a reason for BDA models which produce exact experiences which are important to the association overall and not exclusively to single cycles. Most of the organizations tried to make disparate data sources accessible by aggregating their data into a single point of access [4].
SOME APPLICATIONS OF BIG DATA ANALYTICS IN SCM
One of the applications is to supply some aid to practitioners to understand where they can begin incorporating Big Data Analytics across their supply chains, sanctioning them to potentially solve intricate problems related to such.
CONCLUSION
We concur with Waller and Fawcett who argue that antecedent research had not yet felicitously consummated the gap between supply chain functional knowledge, the supply chain data, and BDA procedures and this was the motivation to introduce this paper base up, inducing the essential advantages for BDA from the understanding of the information sources present in the supply chain and from the use of BDA models to unmitigated issues in SCM.
REFERENCES
[1] Zhong, Ray Y., Newman, Stephen T., Huang, George O., Lan, Shulin (2016). Big Data for Supply Chain Management in the Service and Manufacturing Sectors: Challenges, Opportunities, and Future Perspectives. [2] Abla Chaouni Benabdellah, Asmaa Benghabrit, Imane Bouhaddou, El Moukhtar Zemmouri (2016). Big Data for Supply Chain Management: Opportunities and Challenges. [3] Ivan Varela Rozados, Benny Tjahjono. Big Data in Supply Chain Management: Trends and Related Research (2014). [4] Benny Tjahjono, Big Data Analytics in Supply Chain Management: Trends and Related Research (2014). [5] Md. Tahiduzzaman, Mustafizur Rahman, Samrat Kumar Dey, Md. Sumon Rahman, S. M. Akash, Big data, and its impact on the digitized supply chain (2017). [6] Kazi Shahadat Hossain, The impact of big data analytics on supply chain management in the perspectives of Bangladesh large scale enterprise. (2017). [7] Mohamed Awwad, Pranav Kulkarni, Rachit Bapna and Aniket Marathe Big Data Analytics in Supply Chain: A Literature Review (2018). [8] Abla CHAOUNI BENABDELLAH, Asmaa BENGHABRIT,Imane BOUHADDOU, El Moukhtar ZEMMOURI Big Data for Supply Chain Management: Opportunities and Challenges. (2016).
[9] FASIKA BETE GEORGISE, KLAUS-DIETER THOBEN,MARCUS SEIFERT Identifying the Characteristics of the Supply Chain (2014).
[10] SCOR Model, Association for Supply chain management https://scor.ascm.org/processes/introduction. [11] Subhodip Pal. Big Data – Holds the answers of Supply Chain Analytics (2021).