
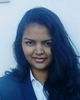
- Open Access
- Authors : Vidhi Kalpesh Sheth , Vrushali Kalpesh Sheth , Bhakti Kiran Rathod , Dr. Kranti Vithal Ghag
- Paper ID : IJERTV9IS060943
- Volume & Issue : Volume 09, Issue 06 (June 2020)
- Published (First Online): 04-07-2020
- ISSN (Online) : 2278-0181
- Publisher Name : IJERT
- License:
This work is licensed under a Creative Commons Attribution 4.0 International License
AI Power Hand Gesture Control Vehicle
Ms. Vidhi Kalpesh Sheth
Information Technology Department Shah and Anchor Kutchhi Engineering College
Mumbai, India
Ms. Vrushali Kalpesh Sheth
Information Technology Department Shah and Anchor Kutchhi Engineering College
Mumbai, India
Abstract There are lots of technologies or techniques emerging in the auto vehicles sector. So being in 21st century the Artificial Intelligence and the Internet of Things has given a boost to all these sectors being related to the auto-vehicles. Earlier, the robotics vehicles was being controlled via wired cable or a bluetooth device which had a lot of drawback, due to which it ensures less compatibility and also the vehicles in real world application such as military was not having that impact of Artificial Intelligence or Internet of things due to which there were more a lot of efforts or human task had to be undertaken. In this work, detailed survey and comparative analysis of the robotic vehicle is being presented[8]. Most of the systems, use IOT and AI which reduces human efforts, increased compatibility, ensure security and less human interaction required. Some of the robotic vehicles were controlled wirelessly via hand gesture even at very remote areas. Such type of vehicles can also be considered in real world application like military/defense, surveillance at the border and similarly during the war. If such type of systems is implemented with the power of AI, it would help to detect the enemy and notify the user.
KeywordsArtificial intelligence; Gesture control vehicle; Hand gesture recognition; Robotic car
-
INTRODUCTION
A Gesture Controlled Vehicle is a kind of vehicle which can be controlled by the users hand gesture not by old buttons. Human hand gestures are natural and with help of wireless communication[1]. It is easier to interact with vehicle in a friendly way than usual remote-controlled vehicle. In fig 1 it shows that the vehicle moves according to your hand movement from a distance. The vehicle moves forward, backward, right and left respectively. The vehicle stops
when the hand is kept parallel to ground. There is a camera mounted on vehicle which is capable of zooming in and out as well as capable of rotating 360 degrees. The output video will stream constantly on the android based smart phone or laptop[5]. The structure of Vehicle uses Arduino Uno, RF transmitter, receiver module, accelerometer, GPS, webcam. The Arduino Uno reads analog output values i.e. x- axis and y-axis values of accelerometer which changes depending on hand motion and converts that analog value to respective digital values[3]. The digital values are processed by microcontroller and send the command to vehicle via RF transmitter. This command are received by RF receiver and processed by microcontroller to drive the motor to a particular direction[6].
Ms. Bhakti Kiran Rathod
Information Technology Department Shah and Anchor Kutchhi Engineering College
Mumbai, India
Dr. Kranti Vithal Ghag
Information Technology Department Shah and Anchor Kutchhi Engineering College
Mumbai, India
Fig 1: Hand Movements to control Car
-
LITERATURE SUREVEY
-
The first paper surveyed is (2019) Deep Learning Algorithm Using Virtual Environment Data for Self Driving Car[1]. The approaches of this paper were an autonomous driving technique announced by NVidia using car games. In this paper they had used different datasets such as Learning data with an end to end method. The performance of this paper is about 80%. The Precision and Recall of this paper is about 81%and 85%.
-
The second paper surveyed is (2006) A Cognitive agent based approach to varying behaviors in computer generated forces system to model Scenarios
like coalitions[2]. The approach of this paper is Dynamic Variation in behavior of entities in military based computer generated forces system scenarios. The datasets which they were used is Methodology based on the application of Autonomous cooperative building blocks in order to handle the CGF. The performance, precisions and recall of this paper is 77%, 74% and 73%.
-
The third paper surveyed is (2017) Military based vehicle to greed and vehicle to vehicle micro greed system architecture and implementation[3] This paper describes a real-life military use of the Vehicle-to- Grid / Vehicle-to-Vehicle (V2G-V2V) based micro grid network. The device offers a plug-and-play, very quick forming, and smart, aggregated, and effective power solution for a military base contingency that can be set up in less than 20 minutes and is ready to produce up to 240kW of 3-phase (3Ø) 208Y/120VAC. The system uses Transmission- Integrated Generators (TIGs) vehicles to produce 600VDC power for vehicle hotel loads (i.e. electrification of non- propulsion and auxiliary loads) and off-board loads (tents / shelters, contact centers or other electrical loads). This
initiative includes four military combat vehicles-two M1152 HMMWV vehicles fitted with 30kW of on-board vehicle power (OBVP) And two MaxPro Dash MRAP vehicles fitted with 120kW 3000 Transmission- Integrated Generators (3TIGs) with V2 G and V2V capacity, four 60kW DC-to-3Ø AC power converters with 600 VDC bus distribution systems and four 22,8 kWh of Energy Storage Units (ESU). The performance, precision and recall of this paper are 70%, 75% and 65%.
-
The fourth paper surveyed is (2016) Probabilistic risk based security assessment of power system considering incumbent threat and uncertainties[4]. In- depth Power Systems (PS) security analyzes includes consideration of vulnerabilities to natural and human- related threats that can trigger multiple dependent continent genes. At the other hand, these events also lead to high impact on the system, so it can become difficult to make decisions aimed at improving protection. The risk associated with each contingency may be the introduction of uncertainty. The performance, precision and recall of this paper are 69.50%, 80% and 75%.
-
The fifth paper surveyed is (2019) Design and implementation of hand movements controlled robotic vehicle with wireless live streaming feature[5]. This paper describes the design and implementation of a robotic
vehicle that can be operated from all directions using a wireless camera mounted at the top of the vehicle to relay live video streaming to the end of the user. This thus avoids the hassle of gesture recognition and image processing software, or even the use of switches or joysticks to guide a robot's movement in various directions, and provides the user with a wireless monitoring facility. They have design a robotic controlled vehicle wirelessly. Person can control the movement in forward direction, backward direction, left and right direction by only using the hand movement gesture. Also this system has use on board camera to provide facility from remote places. The performance, precision and recall are 84%, 86% and 91%.
-
The sixth paper surveyed is (2019) Smart glove and hand gesture based control interface for multipolar
aerial vehicles[6]. This project's research objective performs a comparative performance analysis between real- time image processing and object recognition of Artificial Intelligence while implementing an autonomous wheelchair obstacle avoidance device. The proposed framework for the identification of obstacles is accomplished by applying camera sensor with the application of Artificial Intelligence techniques in image processing. In designing the object recognition algorithm, a pr-trained Con- volitional Neural Network model known as Mobile Net SSD and Deep Neural Network (DNN) module in the Open CV library (for live video streams) are used. The performance, precision and recall of this project are 89.79%, 60% and 85%.
-
The seventh paper surveyed is (2017) Study of Evaluation method of in vehicle gesture control[7]. In a simulated driving cockpit with an Eye-Tracker involved, a usability test for in-vehicle gesture control was performed.
In the study, 14 usability- related data types were collected from which 11 usability-related indexes were
chosen to construct a Fuzzy Comprehensive Evaluation System. Through this method, the most appropriate gesture solution was identified for each task, which can provide reference for the future application of gesture control inside the vehicle. The performance, precisions and recall of this paper are 75%, 34% and 70%.
-
The eight paper surveyed is (2018) Development of intelligent riding comfort monitoring system for
automated vehicle[8]. This paper demonstrates the conceptual framework for reflection to the real driving power of the intelligent riding comfort monitoring system. The experiment is structured for the precise analysis of human behavior. In certain types of automated vehicles, the proposed scheme will theoretically be extended to enhance the perceived efficiency of automated driving. The performance, precisions and recall of this paper are 68%, 80% and 78%.
-
The ninth paper surveyed is (2018) Wrist Cam: A wearable sensor for hand trajectory gesture recognition and intelligent human robot
interaction. A wearable wrist-worn camera sensor (Wrist Cam) was shown here to identify movements of the hand trajectories. The user's hand's moving velocity was deduced from the corresponding Speeded up Robust Features (SURF) key points of the video sequence's moving history. In addition, continuous gesture segmentation was accomplished by detecting the predefined gesture starting signal from the image's hand field, which was segmented by the Lazy Snapping algorithm. In their research, the Dynamic Time Warping (DTW) algorithm identified 10 types of gestures and 1350 gesture samples collected from 15 subjects at 3 separate scenes, and the results achieved an overall accuracy of identification up to 97.6 percent. However, by guiding a cooperative robot to drawletters on paper, the practicability of the proposed device was further demonstrated. The performance, precision and recall of this paper are 79%, 68% and 65%.
-
-
COMPARATIVE ANALYSIS
Table 1: Comparative Analysis of technique for Hand Gesture Controlled Vehicl[1] [2] [3] [4] [5] [6] [7] [8]
Sr. No
Refe Rence
.No
Year Of Pub cation
Title
Authors
Datasets
Approach
Performance (accuracy)
Precision
Re- call
1.
2019
Deep Learning
Algorithm using Virtual Environment
Data for Self
Driving Car
Juntae Kim,
Bokyeong Kim, Geunyoung Lim,
Youngi Kim,
Changseok Bae
Learning
Data with an end-to-end method.
An
Autonomous Driving technique
announced by
Nvidia using Car games.
80%
81%
85%
2.
2006
A cognitive agen
based approach to varying behaviours in computer generated force System to mode Scenarios like
coalitions
Martyn
Fletcher
Methodology
Based on the Application of Autonomous Cooperative Building blocks In order to handle the
CGF.
Dynamic
Variation in Behaviour of Entities in military based Computer generated forces system
Scenarios.
77%
74%
73%
3.
2017
Military based
Vehicle to greed and Vehicle to vehicle micro greed system architecture and implementation
M.Abul
Masrur
The survillence
Required area being
considered Must be noted Or mapped.
A real life
Military application of V2V V2G
based microgri system.
70%
75%
65%
4.
NOV
2016
Probablistic risk
Based security Assessment of Power system Considering Incumbent threat and uncertainties
Emanuele
Ciapessoni, Diego Cirio
In depth
Security Analyses of Power system To consider
Vulnerabilities
To human Related threat
N-1 criterian
Is still deemed as a good Tradeoff Between Completeness And Computational
Time.
69.50%
80%
75%
5.
2019
Design and
implementation Of hand
Movements
Controlled robotic vehicle with wireless live streaming feature.
Md.R.Raihan,
R.Hasan, F.Arifin, M.R.Haider, S.Nashif
The
Identification of x and y Axis of variou Hand
Movement.
Real time
Wireless live Streaming Via gesture Controlled Vehicle.
84%
86%
91%
6.
OCT
2019
Smart glove and
Hand gesture Based control Interface for Multirotar aeria vehicles
Kianoush
Haratiannejadi, Neshant Elhami
Fard, Rastko R.Selmic
Various
angles of glove
movemennt recognition to control
the vehicle
Self validation
for multi rotor aerial vehicle
89.79%
60%
85%
7
2017
Study on the
Evaluation Method of In Vehicle gesture Control.
Jun Ma,
Yuchan Du.
A lot of angles
Of the hand movements are considered to control the car and that data is stored in gesture database.
14 kinds ofdata
Related to the Usability were Collected in tes From which indexes that could apparent represent the usability comprehensive
Evaluation.
75%
34%
70%
8
2018
Development
Of Intelligent Riding Comfort Monitoring
System for
Automated Vehicle.
Young Eun
Song,
G wang s oo Le, e Kichang Lee, Chang Kim, Moon Hyung Song,
Jae Hwan Lim.
The
Electroence- Philography Information is strongly related with driving
Situations.
Intelligent
Riding comfort Monitoring
System using th Recurrent Neural Networks.
68%
80%
78%
9
2018
WristCam: A
Wearable
Sensor for hand Trajectory
Gesture Recognition And Intelligent Human-Robot Interaction.
Feiyu Chen,
Honghao Lv, Zhibo Pang, Junhui Zhang, Ying Gu, Yonghong Hou, Huayong yang, Geng Yang.
Hand Trajector
Gestures being Stroed ingestu Database to Identify
Movement of Vehicle.
The moving
Velocity of the users hand was deduced from t matched
speeded up robust features keypoint of the moving back- ground of the
vedio sequence
79%
68%
65%
-
DISCUSSION
Surveyed gesture-controlled vehicles were compared on the basis of accuracy, precision and recall in Table 1. Smart glove and hand gesture-based control interface for multirotor aerial vehicles with the dataset of studyin the various angles of glove movement recognition to control the vehicle achieved a remarkable accuracy of 89.79% is. The approach is used to satisfy the speed requirements. The hand gesture recognition with aerial vehicles is robust to scale changes as compared to other literature reviewed[6].
-
CONCLUSION
Now-a-days the demand for Artificial Intelligence based military vehicles has increased tremendously. In future we can extend the range of the vehicle by using the satellite. In fig 2 the gesture controlled systems gives an alternative way of controlling robots. With an advance in the AI a variety of features can be added to the Gesture Controlled Vehicle that can be used in various sector like defense, surveillance, industries, hospitals, etc. This technology or technique can be used to give a boost to the manufacturing of the vehicles and giving its contribution to the auto- mobile sectors. It is very efficient, compatible and easy to use. By converting this robotic car to an amphibious vehicle which can work on land as well as water and increases the range of its application. In future the similar concept can be used in robotic ARM making it controlled via wirelessly using Gesture.
Fig 2: Gesture controlled vehicle
REFERENCES
-
M. R. Raihan, R. Hasan, F. Arifin, S. Nashif, and M. R. Haider, Design and Implementation of a Hand Movement Controlled Robotic Vehicle with Wireless Live Streaming Feature, 2019 IEEE International Conference on System, Computation, Automation and Networking (ICSCAN), 2019.
-
Jun Ma and Yuchun Du, Study on the Evaluation Method of In- Vehicle Gesture Control, 2017 IEEE 3rd International Conference on Control Science and Systems Engineering.
-
M. A. Masrur, A. G. Skowronska, J. Hancock, S. W. Kolhoff, D.
Z. Mcgrew, J. C. Vandiver, and J. Gatherer, Military-Based Vehicle-to- Grid and Vehicle-to-Vehicle Micro gridSystem Architecture and Implementation, IEEE Transactions on Transportation Electrification, vol. 4, no. 1, pp. 157171,2018.
-
E. Ciapessoni, D. Cirio, G. Kjolle, S. Massucco, A. Pitto, and M. Sforna, Probabilistic risk-based security assessment of power systems considering incumbent threats and uncertainties, 2017 IEEE Power & Energy Society General Meeting, 2017
-
Yoon Lee, Shang Tan, Yeh Goh, Chern Lim. 2019 IEEE 3rd Advanced Information Management, Communicates, Electronic and Automation Control Conference (IMCEC) 2019.
-
Young Eun Song, Chang Il Kim, Moon Hyung Song, Gwang Soo Lee, Jae Hwan Lim, Kichang Leeand Moon Sik Kim, Development of Intelligent Riding Comfort Monitoring System for Automated Vehicle,2018.
-
L. Jiang, M. Xia, X. Liu, and F. Bai, Givs: Fine-Grained Gesture Control for Mobile Devices in Driving Environments, IEEE Access, vol. 8, pp. 4922949243, 2020.
-
Feiyu Chen, Honghao Lv, Zhibo Pang, Member, IEEE, Junhui Zhang, Yonghong Hou, Ying Gu, Huayong Yang, Member, IEEE, and Geng Yang, WristCam: A Wearable Sensor for Hand Trajectory Gesture Recognition and Intelligent Human-Robot Interaction. , 2018 IEEE.