
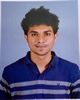
- Open Access
- Authors : Vishal C V , Nischay N
- Paper ID : IJERTV10IS100184
- Volume & Issue : Volume 10, Issue 10 (October 2021)
- Published (First Online): 08-11-2021
- ISSN (Online) : 2278-0181
- Publisher Name : IJERT
- License:
This work is licensed under a Creative Commons Attribution 4.0 International License
A Data Science and Machine Learning Driven Study on the Impact of Music on Emotions and Sleep Cycles of Listeners
Vishal C V¹
PG Student,
Data Science, IIIT, Bangalore
Nischay N²
Student,
Dept. of Computer Science and Engg,
JSS Academy of Technical Education, Bangalore
AbstractThe applications of Machine Learning and Data Science in music are umpteen. Emastered and Landr offer automated Sound Engineering services. Spotify and iTunes have innovative Data Science teams. Atom Music Audio offers amazing new musical sounds and instruments. With music now easily available, the music industry has had a prosperous 21st century so far. This, in turn, has given birth to a new niche field named Music Analytics, the amalgam of music and analytics. In a way, music is a product an intangible product. Like other products, it acts as an external stimulus, having the potential to affect our emotions, and mood in general. The paper aims to address the whys and whats of the music effect and the effect of music on sleep cycles.
Keywords Music, Sleep-Cycles, Stimulus, Emotions
-
INTRODUCTION
In a way, music is a product an intangible product. Like other products, it acts as an external stimulus, having the potential to affect our emotions, and mood in general. This paper discusses answers to why an individual listens to a particular song when in a certain mood and what are the features of a given song (genre, scales, instrument, speed) that cause this effect. An attempt to study the impact of music on sleep cycles, by linking our results to other articles and studies.
This paper is an intersection of Technology, Psychology and Arts. Exploratory Data Analysis has been performed and popular models have been run on the data. Apart from the typical compilation and reporting of results, an effort has also been made to understand the influence of certain aspects of music on the human mind. The outcomes have been shared and discussed with professional music composers and producers in order to gain clarity regarding correlation and causation. The observations and reasoning have been presented after thorough consideration.
-
LITERATURE REVIEW
Researchers Hussain-Abdulah Arjmand, Jesper Hohagen, Bryan Paton, and Nikki S. Rickard [1], by measuring the physiological index of emotion of 18 participants as they were exposed to music stimuli, studied how real the emotions that an individual reported they felt, when they listened to a particular piece of music. Alen S Cowen, Xia Fang, Disa Sauter, and Dacher Keltner [2], in their research, found that the feelings associated with music were found to occupy continuous gradients, contradicting discrete emotion
theories. They uncovered individuals true feelings associated with music. However, few papers have delved deep into the features of music that engender and transform feelings.
A piece of music carries with it a number of features such as frequency, tempo, time-signature, amplitude, note- pattern, lyrics and instrument-composition. First, it is essential to understand which of these features play prodigious roles in activating certain regions of the brain, promoting the release of hormones and in turn causing a transition in emotions. Once the impacts of features have been decoded, the nature of a given feature (lyric, chord, instrument or tempo) that enables it to drive the transition must be looked into.
Sleep Foundation Author Rob Newsom in his article Music and Sleep [3] elucidates why music affects sleep and what forms of music evoke sleep. He has made an effort to draw a relationship between music and sleep hygiene. Maren Jasmin Cordi, Sandra Ackermann, and Bjorn Rasch in their paper [4] decipher the effects of relaxing music on healthy sleep, by studying sleep patterns of 27 female subjects. But, do certain genres of music lead to better sleep than others? Does listening to a given genre of music impede peaceful sleep? Does an individuals general taste in music impact their sleep cycle? These are the questions our research aims to answer.
-
OBJECTIVES
The paper discusses answers to the following questions:
-
What are the characteristics of a song that impact the transition of emotions, and how do they do so?
-
Does listening to a particular genre of music induce better sleep?
As per the results obtained, ideal sleep music has been presented, by altering musical features of a song.
-
RESEARCH METHODOLOGY
Data was collected through Google Forms. 154 candidates responded to questions regarding the songs they listen to when they are insanely happy, sad and prior to falling asleep. They also included information about their proficiency in
music and sleep patterns. All questions in the survey were made compulsory in order to avoid excessive imputation/dropping of data during pre-processing. To ensure the veracity of data, the musical features (primary chords, tempo, time-signature, lyrics, primary instruments) of each song were manually assessed and entered into the database. Post data collection, there were about 20 features available for analysis and model building.
To describe and back the results obtained, multiple other articles based on the intersection of music and psychology were referred to, and have been presented in the paper.
First, the lyrics of each song were cleaned. Stop-words and words with less than 4 letters were dropped. One-hot- encoding was applied to every categorical variable. This type of encoding creates a new binary feature for each possible category and assigns a value of 1 to the feature of each sample that corresponds to its original category. After the application of one hot encoding, 83 features were available for training. The tempos and time-signatures of some songs varied in different parts of the song. For example, a song started off with vocals at no specific tempo or time-signature, before percussions came in to set the groove. To handle such instances, the missing values for tempos were imputed using MICE (Multiple Imputation by Chained Equations). MICE is a multiple imputation method used to replace missing data values in a data set under certain assumptions about the data missingness mechanism. Pythons IterativeImputer from FancyImpute was used for the same.
Standard Scaler was applied to sad_song_tempo and happy_song_tempo, which were the tempos of songs that candidates listened to when they were sad and happy respectively. The majority of classifiers calculate the distance between two points by the distance. If one feature has a broader range of values, the distance governs this particular feature. The range of all continuous features are normalized so that each feature contributes approximately proportionately to the final distance. Since the data was normally distributed, Standard Scaler was applied.
Fig 4.1. For a given x, standard scaler is calculated by subtracting the mean of all observations from x and dividing the resultant by the standard- deviation of all observations.
Fig 4.2. The distributions of tempos resemble a normal distribution.
After the preprocessing of data, k-means clustering was applied to the data frame. The k in k-means refers to the number of centroids. A centroid is the location representing the centre of a group, called the cluster. Every data point is allocated to a cluster by reducing the in-cluster sum of squares. The optimum number of k-means clusters can be determined using what is called the Silhouette Score.
Fig 4.3. Silhouette score index for each point is deermined by dividing the difference between bi( the distance between point i and the centroid of the nearest cluster ) and ai(distance between point i and the centroid of the cluster it belongs to), by the maximum of a and b.
The Silhouette score can take any value between -1 and 1 (both inclusive), where -1 indicates extremely poor clustering and 1 indicates perfect clustering. A score greater than 0 indicates that the components of a cluster are native to that cluster to some extent. The silhouette score calculated after the k-means clustering algorithm was applied on the data set for n in [2,10] is shown below.
Fig 4.4. Silhouette scores
Another factor taken into account when determining the optimum number of clusters is the end point of the elbow in the elbow curve. The elbow method plots the value of the cost function (dispersion) produced by different values of n. The value of k at which improvement in distortion declines the most is called the elbow, at which we should stop dividing the data into further clusters. The elbow curve for n in [0,8] clusters is shown below.
Fig 4.5. The elbow curve confirmed that 3 is the optimum number of clusters. So, the dataset was divided into 3 clusters.
The features of each cluster were studied to understand the major differences. The results will be discussed in the Results and Interpretations section.
The next target was to fit a model that could predict whether or not an individual would face problems sleeping, in order to identify the major factors that influence or play a part in inducing peaceful sleep. This was treated as a classification problem, where X = problems falling asleep and y = All other features of the dataset. Several different k-fold-cross validated Multiple Logistic Regression, Random Forest classifier and XGBoost models were tried on the model.
In Logistic regression, the logistic curve acts as a divider, separating two different classes.
Fig 4.6. Simple Logistic Regression is given by the above formula
A random forest is a meta estimator that fits a number of decision tree classifiers on various sub-samples of the dataset and uses averaging to improve the predictive accuracy and control over-fitting.
Fig 4.7. A forest with 2 different trees
Boosting is an ensemble learning method that combines a set of weak learners (not necessarily the same category of learners) into a strong learner to minimize training errors. XGBoost is the most popular boosting algorithm.
Recursive Feature Elimination was used to select the best features. GridSearchCV was used to loop through predefined parameters and identify the model that performed best. The performance of the model was evaluated based on two values accuracy score and roc_auc score. Roc_auc stands for area under roc curve. The ROC curve shows the trade-off between sensitivity (or TPR) and specificity (1 FPR).
Fig 4.8. Regression Model
It is to be noted that some features such as lyrics and proficiency were eliminated from the supervised and unsupervised models. While the lyrical aspects were
analysed separately, we had too few data points that suggested that a candidate was proficient in playing music.
-
RESULTS AND INTERPRETATIONS
-
Exploratory Data Analysis
120 is a pseudo-default number in the music world. Most composers start composing a song at 120, and later lower/higher the tempo based on the feel of the composition. Tempo above the 120-benchmark is considered upbeat. The tempo of sleep songs that respondents listened to was analysed. An important observation was that only 16% of the respondents who listened to music at a tempo higher than 120 before going to sleep faced sleep-related difficulties, whereas a whopping 44% of the respondents who listened to music at a tempo lower than 120 before falling asleep faced sleep-related difficulties. Upbeat music is known to prevent the release of Cortisol, a stress hormone. This may well be the reason upbeat music listeners sleep better. A common misconception is that slower songs relax the mind. However, in researcher Ling Yius paper [4], it has been elucidated that songs with medium and slower tempo are known to cause stronger activation in the brain. In all probability, the stronger activation in other regions of the brain may overpower the hypothalamuss effort in arousing sleep. It is to be noted that of the respondents who listened to other non- musical sounds or narrations, 76% reported peaceful sleep, while the rest reported difficulties.
Fig 5.1.1. Collage of frequent words in songs respondents listen to when they are happy
Exploratory analysis on lyrical features confirmed some facts. The words dance and clap were repeated several times in happy songs. About 63% of these words came from the genre that was categorised as Electropop. Electropop is a genre which involves fusion of electronic sounds such as synthesisers, traditional guitars and pianos. What is it that gets people glued to dance music? The reaction of our brains to dance music is similar to the reactions of our brain to a rollercoaster ride.
Fig 5.1.2. Sound Waves of song Gangnam Style
The gif above demonstrates a visualization of the sound waves with respect to time for the song Gangnam Style, a dance song picked up from the response of a respondent. The rise and fall of the amplitudes and frequencies in electronic music replicate the feeling of building anticipation, producing dopamine, the happy hormone.
Fig 5.1.3. Sound Waves of song Night Changes
The gif above demonstrates a visualisation of the sound waves of Night Changes a song without electronic music characteristics. It can be seen that the amplitude of sound waves is lower and it does not demonstrate intense, periodic rise and fall behaviour.
-
Clustering Results
As mentioned in the previous section, the optimal number of clusters was determined to be 3. There was a noticeable difference among the clusters in happy song tempos. It can be seen that cluster 0 shows a lower happy song tempo (median below 120 BPM) whereas cluster 2 includes datapoints demonstrating a higher happy song tempo (median nearly 160).
Fig 5.2.1. Box Plot (id vs happy song tempo)
The actual impact of happy song tempos was realised when general statistics were derived.
Fig 5.2.2 Impact of happy song tempos
Let alone sleep song tempos, but in general happy song tempos also seemed to have an effect on sleep. Members of Cluster 0 exhibited an average happy song tempo of 130, with a whopping 87.5% of members having sleep problems. However, it is strange that these members had a higher sad song tempo. Members of Cluster 2 listened to other sounds, stories or nothing at all just before going to sleep. Members of Cluster 0 listened to songs with lower tempos (<120BPM) before going to sleep. This further confirms that people who listen to upbeat songs or other sounds/stories sleep better than the people who listen to slower songs before bed. The major instruments of sleep songs that members of clusters 0 and 1 listened to were visualised.
Fig 5.2.3 Cluster 0 'sleep song' major instruments
Fig 5.2.4 Cluster 1 'sleep song' major instruments
Vocal-dominant songs won the majority in cluster 0 and piano-dominant in cluster 1. However, neither were absolute majorities. Hence, nothing significant can be concluded. The supervised models were used to determine the importance of this particular feature.
-
Supervised Models Results
First, a cross-validated Random Forest Classifier model was trained. Selection of features was performed using Recursive Feature Elimination(RFE) and by varying the n_estimators parameter of Random Forest Classifier. GridSearchCV was used for the selecting parameters. The best model and testing results are indicated below.
Figure 5.3.1. Random Forest Classifier Results
Some of the important features that were detected by his model were classes of happy song major instruments (acoustic guitar, piano, strings etc), classes of happy song genre, classes of sad song genre, sleep song tempo , classes of sleep song major instruments and sleep song chords.
XGBoost did not yield favourable results. Nearly all XGBoost models seemed to overfit.
To obtain better results, a Logistic Regression model with features picked using RFE as well as statsmodels General Linear Model (GLM). Picking features using GLM involves studying p-values and Variation Inflation Factors (VIF). P- value is a measure of predictive power of a feature. A feature is considered an appreciable predictor if its p-value is less than 0.1 (which means the chance that the feature is insignificant is less than 10%). The VIF detects multicollinearity among predictors. Ideally, VIF must be below 10. After several steps of revising the number of features, testing VIFs and training and testing models, we arrived with 3 strong predictors.
Figure 5.3.2 Predictors
As the picture above suggests, all three predictors were related to the sleep songs. The respective VIFs were below 5, eliminating the possibility of multicollinearity.
Figure 5.3.3. Metrics
The GLM model trained with 3 variables yielded highly improved results.
Figure 5.3.4. Receiver Operating characteristic
The ROC AUC score was nearly 0.8, indicating a good model and eliminating the possibility of overfitting.
A probability mapping (Probability vs Accuracy, Sensitivity, Specificity) was also done to ensure that an optimum threshold was selected. The GLM allots 1(True) if the probability that the given input will yield a positive is greater than 0.5. Else, it allots 0(False). The probability map was visualised. The breakeven point is considered the best threshold.
As the graph below suggests, the break-even point corresponds to a probability of about 0.53-0.55.
Figure 5.3.5 Calculation of Cutoff between different metrics
Figure 5.3.6. Accuracy
The accuracy improved to around 71%. More importantly, the other metrics sustained strong values.
Figure 5.3.7. Precision and recall tradeoff
The precision-recall trade-off was also acceptable by any standards.
-
-
CONCLUSION AND FUTURE WORK
-
Despite EDA and unsupervised clustering suggesting that other features such as happy song tempos and sad song chords may have an impact on a persons sleep, the final GLM Logistic Regression model confirmed they were rather poor predictors. So, it was concluded that sleep song related features best indicate whether or not a person has trouble sleeping. Occams Razor stands strong! The relatively simpler models with a limited number of predictors always produce best results. A pop song in a major scale with a tempo of 130 and piano as the major instrument works best.
REFERENCES
-
Hussain-Abdulah Arjmand, Jesper Hohagen, Bryan Paton, and Nikki S. Rickard – Emotional Responses to Music: Shifts in Frontal Brain Asymmetry Mark Periods of Musical Change.
Available at https://doi.org/10.3389/fpsyg.2017.02044
-
Alen S Cowen, Xia Fang, Disa Sauter, and Dacher Keltner – What music makes us feel: At least 13 dimensions organize subjective experiences associated with music across different cultures.
Available at https://doi.org/10.3389/fpsyg.2017.02044
-
Rob Newsom – Article – Music and Sleep. Available at https://www.sleepfoundation.org/noise-and-sleep/music
-
Cordi MJ, Ackermann S, Rasch B. Effects of Relaxing Music on Healthy Sleep. Sci Rep. 2019 Jun 24;9(1):9079. doi: 10.1038/s41598-019-45608-y. PMID: 31235748; PMCID: PMC6591240.