
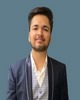
- Open Access
- Authors : Ria Irani, Raghav Singhal, Rayna Dsouza, Rohan Surana
- Paper ID : IJERTV12IS080059
- Volume & Issue : Volume 12, Issue 08 (August 2023)
- Published (First Online): 02-09-2023
- ISSN (Online) : 2278-0181
- Publisher Name : IJERT
- License:
This work is licensed under a Creative Commons Attribution 4.0 International License
Trends in Operations Research and Artificial Intelligence
Ria Irani
NMIMS Anil Surendra Modi School of Commerce
Rayna DSouza
NMIMS Anil Surendra Modi School of Commerce
Raghav Singhal
NMIMS Anil Surendra Modi School of Commerce
Rohan Surana
NMIMS Anil Surendra Modi School of Commerce
Rahul Dalal
NMIMS Anil Surendra Modi School of Commerce
Abstract
Purpose: Artificial Intelligence is the use of machines or software to think and make human intelligence-based decisions with the elimination of the error factor. Introduced by scientist John McCarthy in 1956, artificial intelligence is now being applied and used in all areas of business. Operations research has been the driving force behind decision-making for years. Artificial intelligence combined with operations research techniques is what businesses now are aiming to achieve to increase their efficiency and optimise their processes. This article aims to understand the extent of research already prevalent in this area, i.e. to which areas artificial intelligence and operations research processes have already been applied and to what extent. By understanding this the gaps existing in the area of study and the areas of possible future research can be identified. This article wishes to provide future academicians with a base for future research and make policymakers aware of how artificial intelligence and operations research techniques have been used in their areas of discipline and how they can aim to implement the same.
Approach: Due to the extent of vast literature available in this study, a systematic review through bibliometric analysis has been adopted. This method was used to get a qualitative and quantitative analysis of the data available. The data consists of 300 articles published between 1990 and 2022 extracted from the Scopus database after using relevant keywords pertaining to artificial intelligence and operations research.
Findings: Analysis of the papers in the dataset was done using citation analysis, page rank analysis, year-wise trends, source trends and co-occurrence network. By using these different analysis methods the current trends in publications were understood and further future possible areas of research were identified.
Keywords: Artificial intelligence; Operations research; Knowledge-based systems; Decision support systems; Machine learning.
-
INTRODUCTION
Artificial intelligence (AI), has been the focus of many peoples fantasies and the main plot of science fiction movies for years. However, it is now a reality that affects peoples daily lives whether they are aware or not (Thormundsson 2022). AI refers to the ability of systems to make automated data-driven decisions without the intervention of human beings. The term AI was defined by scientist John McCarthy in 1956 and today is the heart of almost everything in our lives (Thormundsson 2022). The purpose behind the use of AI is to leverage computers and machines to mimic the human brain and perform decision-making processes in various situations (IBM Cloud Education 2020). As a result, AI has been applied in different areas to amplify human intelligence in the most effective way.
Although prevalent since 1956, AI has just begun being used to its full potential to provide benefits in various areas. The increase in available data in different sectors has further pushed enterprises and challenged them to integrate AI into their processes (Mao,
Zhang, and Tang 2021). With the commencement of Industry 4.0, which is expected to impact all disciplines, industries and economies (Bernard Marr 2018), AI has begun to transform innumerable areas of our lives.
Operations research (OR) methods aim to support decision-making processes (Doumpos et al. 2022). Operations research has been used for years in traditional problems to find feasible solutions. OR can be defined as the application of systematic and mathematical systems to study and investigate the problems that support us with data to take the correct decision (Monks 2016). Today, similar to the use of AI, a number of areas have inculcated OR methods as a part of their decision-making and problem- solving process to find optimal solutions. Professionals from varying fields analyse problems, use OR models to improve solutions and minimise risk.
Over the past few years, developments in AI systems have greatly affected the traditional decision-making process that companies follow. With an increase in global competition due to the intense developments in AI processes, businesses in various disciplines have begun to apply AI and OR techniques simultaneously to provide a competitive advantage. Due to the constant developments in AI, OR techniques have also been seeing changes in traditional decision-making systems, which has had a positive impact on various fields. Hence there has been combined integration of AI and OR techniques in scheduling, day-to-day operations, fault detection, forecasting etc. that has helped provide quick real-time decisions.
Previously written reviews covering these two areas mainly focused on their application in specific disciplines. Research has not been done taking the bigger picture into consideration. Previous research has also not taken the effect of applications of AI and OR collectively in different areas. Furthermore, the applications of AI in any business area are extremely vast. Thus, after identifying and understanding the gaps prevailing in the current research this study has taken up a systematic literature review. In addition, this paper focuses on the identification of current trends in areas of AI and OR by reviewing the current environment and further providing an idea about possible future trends. This paper is to help understand the diverse areas of applications and how researchers can reap the benefits of future advances in AI and OR.
This paper aims to aid academicians to understand areas where they can expand and focus their future studies regarding AI and OR trends. It also wishes to provide policymakers with direction to implement upcoming developments in AI and OR processes to ensure the optimisation of resources.
The next section discusses the available literature and the evidence available on the trends of AI and OR. The third section states the objectives of the study and the fourth states the research methodology used to bring it to the dataset used for analysis. The section after that discusses the analysis done on the data collected. This is followed by a discussion of the findings and implications. The last part of this article is the conclusion and limitations of this paper.
-
LITERATURE REVIEW
2.1 International evidence on trends in OR and AI
Every piece of machinery or technology that mimics or replaces human intelligence through the use of computation is considered to be artificial intelligence (Dhamija and Bag 2020).
In recent years OR methodologies have been combined with AI techniques, including machine learning. While OR is used in various disciplines to help find the optimal solution to various problems, the use of AI techniques and tools in various fields helps increase human reliance on the accuracy of OR (Min 2010). Possibilities of successful implementation of artificial intelligence depends largely on big data (Khanra, Dhir, and Mäntymäki 2020). In modern time with data all around us, the use of artificial intelligence is only enhancing the accuracy of reaching optimal solutions of OR methodologies. Wide range of areas such as business, finance, agriculture, textile and medicine, are nowseeing the impact of increased advances in AI techniques.
(Ivanov and Sokolov 2012) undertook an attempt to identify an interdisciplinary perspective and modelling tools for future supply chains which will be collaborative with developments in the AI field. (Jamali, Leung, and Verderber 2020) analysed the application of OR techniques along with AI to optimise hospital layout. (Sikka, Sarkar, and Garg 2022) investigated and further suggested the use of AI in textile production process to help create greater operational precision and product quality. By using nonlinear regression and image processing, structural defects can be found thus helping to improve production processes(Sikka, Sarkar, and Garg 2022). Alternatively, (Sikka, Sarkar, and Garg 2022) outlines the need for AI-based algorithms to help officials with data offloading and real-time detection of natural calamities.
There has been an abundance of work done in the area of applications of AI and OR as shown in table 1. As can be seen prior studies in these two areas have focused on the application of the respective techniques in varying disinclines. Developments and trends in the fields of AI and OR have been reviewed by different experts from many different countries. As a result, we can understand that irrespective of the field proper implementation of AI and OR techniques can help provide a unique advantage to the management.
Therefore, this paper aims to understand the application of AI and similar variables in conjunction with OR through a systematic literature review, to identify the possible future scope in these areas.
-
OBJECTIVES OF THE STUDY
The objective of this study is firstly to understand the disciplines where research in artificial intelligence and operations research has been applied and further understand and explore areas it can be applied to. Building on the gaps identified by analysing the evidence prevalent in the domain, this study has aimed to answer the following questions:
RQ1: To what extent has recent research in the field of AI and OR influenced decision-making capabilities in various areas of discipline?
RQ2: Understand the current trends in the application of AI and OR in terms of disciplines, years, countries and sources.
RQ3: To find out what are the underlying clusters where AI and OR have been helpful over the years?
RQ4: What are the gaps and areas present for future research?
Keeping these four questions as a start a bibliometric analysis has been conducted of the existing literature. The outcome of our research is intended to help provide future academicians with areas of possible future trends in AI and OR application. We aim to provide a basis for future research by analysing past trends and addressing present gaps that should be taken into consideration for the future.
-
RESEARCH METHODOLOGY
4.1 Database, keywords and inclusion criteria
Bibliometric methods employ a quantitative approach for the description, evaluation, and monitoring of published research. These methods have the potential to introduce asystematic, transparent and reproducible review process and thus improve the quality of reviews. Bibliometric methods are a useful aid in literature reviews even before reading begins by guiding the researcher to the most influential works and mapping the research field without subjective bias. (Zupic and ater 2014).
In this paper in order to identify relevant literature pertaining to this study, a two-stage identification process has been followed. In the first stage, a keyword search was conducted on Scopus, the largest database for peer-reviewed articles. Currently, artificial intelligence and operations research have been applied to various areas such as business, agriculture, healthcare, disaster management etc. Hence, to have a complete overview of the trends in OR and AI papers from various disciplines is applicable to
this study, thus justifying choosing Scopus as a search platform. The keyword framework used included both general and specific keywords. A string of applicable primary keywords operations research AND artificial intelligence- were used to yield 6752 initial results. Further to refine the results only articles and journals written in English, as they are certified knowledge (Goyal and Kumar 2021), were included resulting in 451 hits. Since journals are favoured over conference proceedings as a publication, conference proceedings were not taken into consideration (Gupta et al. 2021).
Papers published in the 1970s and 1980s were excluded from the study as major advances in artificial intelligence were only seen after the 1990s. This brought down the number of papers to 415. In the second stage, the articles were further screened to maintain a focus solely on the application of AI and OR in different areas to ensure a proper understanding of the trends in these areas. This was done to ensure a unique selection where topics not directly related to artificial intelligence and operations research were excluded. After excluding papers not related to the areas 300 articles have been recognised and review papers as the final database used for the analysis. Figure 1 shows the complete data retrieval process.
-
Trends seen in the publications
5.1.1 Year-wise publications
-
-
DATA ANALYSIS AND RESULTS
Figure 2 reveals an increasing trend in the papers published regarding the use of AI and OR in business operations. There is a huge increase in the number of papers published from just 4 papers in this area in 1990 to 66 articles in 2021 and 49 in 2022. This area of research shows an 8.14% growth rate which shows a steady increase in the applications of AI and OR over the years. Although it is difficult to exactly identify when AI began, the concept began to be prominently present in 1956. But more so AI applications began to see an upsurge in their use post the 1990s with developments made in deep learning and expert systems (Rockwell Anyoha 2017). In addition, following COVID-19, an almost two-fold increase in the number of articles published can be seen, from 24 in 2019 to 38 in 2020 and 66 in 2021.
-
Publication outlets
The dataset of 300 articles are published in 166 different journals across the world. Figure 3 shows us the trends in the publications in the top 7 journals. Combined these top journals have published 85 articles in this area which accounts for 28.33% of the total dataset.
Sustainability (Switzerland) shows a remarkable increase in publications. There were no publications until 2018. During the COVID-19 phase, the number of papers published in this area of AI and OR skyrocketed to 19 papers published between 2018 to 2022. The Journal of Operational Research Society had a continuous increase in publications in this field from 1990 to around 2015, after which no papers were published in this area.
Other journals like Artificial Intelligence Review, International Journal of Production Research, Journal of Cleaner Production, Journal of Manufacturing Technology Management, etc. have a steady increase in the number of publications throughout the time period monitored. Figure 4 graphically illustrates the number of articles published in some of the relevant journals. The Sustainability (Switzerland), The Journal of the Operational Research Society and The International Journal of Production Research are the top 3 journals that are the most relevant when researching on topics like artificial intelligence in operations research.
-
Author influence and their associated institution and country
In this day and age with AI now being applied to almost every field of business, academicians are in a constant search for newer and better techniques to apply AI and OR in such a way as to increase the efficiency of processes(Dhamija and Bag 2020).
The data shows that there are 876 authors from 491 associated universities from 47 differnt countries. With the need for constant new developments and its constant evolvement in todays time, table 2 shows the top 10 authors along with the publications by them in this field. Although the number of articles published are not that high, with the increasing impact of AI and OR day by day we can expect this number to rise. With regard to author performance, we can say that Zhang Y, Vadera S, Sharma A, Liu Y, Lau HCW, Kumar A, Kobbacy Kah, Gupta S and Chou KL have had the same impact on the studies with each publishing 3 articles. Kobbacy Kah and Vedera S. were one of the academicians in 1998 to publish an article regarding the use of intelligence management systems in operations research. Choy KL published his first article in 2004 and then in 2011 published 4 articles. Other authors such as Sharma A, Agrawal R, Gupta S, Zhang Y have only recently started publishing articles in this area but yet are showing an increase in their publications year by year. This shows us that although there are constant developments in AI and OR applications we see a lot of gaps and untapped areas from the view of research study.
Table 3 shows the publications from different universities. As can be seen from the table the organisation with the highest level of activity in this area is Hongkong Polytechnic University ranked first out of 491 institutions, with 9 articles in the research field on the role of AI in future operations and breakthrough ideas fostering sustainability and innovation. It is followed by Aston University, the University Institute of Lisbon, and the University of Salford, each of which having 5 articles.
Table 4 states the countries associated with the publications. The United States of America is the leader in the number of total articles with 48 published studies followed by the United Kingdom (30 articles) and China (19 articles).
Although it can be seen that the U.S. has the most total citations at 2710, Israel and Spain have a higher average article citation of 169.50 and 108.33 respectively. This shows that countries that are developed as well as developing are working towards understanding the impact and further searching for ways for implementing the use of AI and OR to increase efficiency. Furthermore, these articles published can help provide a basis for future further research and help find newer areas of applications.
-
Citation network analysis
Citation analysis helps future academicians understand the credibility of the papers written in the past in the area of study. Citation refers to the number of times an article has been referred to by another author who is conducting a study in the same area (Dhamija and Bag 2020).
When compared to articles that have been mentioned less frequently, a widely cited paper is seen as more prominent and influential. The greatest way to gauge a research publication's impact is through citation analysis (Tsay and Bazerman 2009).
-
Global citations
Table 5 presents the top 10 articles that are most prestigious in this area of study due to the highest number of global citations by each. Global citations refer to the total number of times a paper has been cited by other articles in the same as well as a different area of study(Goyal and Kumar 2021).
Article by F. Herrera, M. Lozano & J.L. Verdegay in 1998 was ranked 1st with total global citations of 927. This was followed by Thrun S., Muscettola N. and Storer RH. with 703, 448 and 377 global citations respectively. With newer developments being made in this area post Covid-19 an increase in the citations of papers of the past few years are rising.
-
Source citations
-
Figure 5 is a diagrammatic representation of the maximum number of citations from some of the most reputable journals.
The International Journal of Production Research is evidently the most prestigious journal to publish research articles based on artificial intelligence and operations research. 251 citations have been taken from this journal. This makes this journal well-established and a reliable source of information.
The International Journal of Production Economics is next on the list with 129 citations, followed by The European Journal of Operational Research with 127 citations and Expert Systems with Applications with 125 citations. These journals are thus, credible sources of information for future academicians to refer to for information for research.
-
Page rank analysis
Although the number of citations received shows the prevalence of a paper, this does not prove that what the paper states in its study is reliable and is the best work out there (Lamboglia et al. 2021). Page rank was initially used to rank web pages on search engines, it is used today to understand the relevance of a paper based on the presence of prominent keywords.
To analyse using page rank it is presupposed that article A has been cited by other authors who have written articles (T1, T2,.Tn). C(Ti) is the number of references to other articles Ti has. The damping factor is denoted by d and is normally bound between 0 and 1. The page rank (PR) is calculated using the following formula (Ding et al.2009).
() =
(1 )
(1)
+ (
(1)
+ +
()
)
( )
Table 6 shows the top 10 papers ranked according to page rank analysis. From this, it can see that the papers ranked high in total global citations are completely different from those ranked according to page rank analysis.
Baryannis g. (2019) is the top publication based on page rank of 0.058. It is followed by Jarrahi m.h. (2018), Ivanov d. (2019), Ivanov d (2020) and Haenlein m. (2019). According to this method of analysis the recently written papers between 2018- 2020 have more importance and relevance to the current studies prevailing in applications of AI and OR.
-
Keyword analysis
The analysis of keywords allows for an understanding of the theme of the article and provides information about the sub-areas covered in the various studies. After examining 300 papers, 1573 keywords were identified. The table 7 shows the 10 most common keywords used in AI and OR research. The keyword artificial intelligence is used the most out of all in the literature, with 164 occurrences. This suggests that the term is used as a concept frequently across various different areas of study. The words following artificial intelligence in the ranking are decision support systems (50 occurrences), decision making (41 occurrences) and operations research (38 occurrences).
Further to understand the frequently used words in this area of study figure 6 depicts the keyword analysis diagrammatically for better understanding. The treemap depicts the most frequently used word(s) in the bibliometric data, which are quite significant. The top keywords used across the 300 articles, which include 278 articles and 22 review papers, have been included in the map. Here words such as artificial intelligence, decision support systems, decision making and operations research take up most of the map. While other keywords such as supply chain management, sustainable development and decision systems have an average of 2% each. Thus, showing an indirect application of these processes in AI and OR techniques.
Figure 7 shows the cumulative growth of the words used by academicians while researching in this area. From the graph, it can be gathered that study of AI applications with OR has grown exponentially during the last decade as technology and robotics specifically have advanced. The cumulative frequency stood at 49 until 2010, but it began to triple in the span of the next 12 years.
The significant finding from this analysis is that although there are a lot of areas in which processes of AI and ORs application have been researched upon, there is not an in-depth study done. This can be seen by the low percentage of the respective keywords.
-
Sankey Diagram
A Sankey or three plot diagram shows the relation or flow between authors, keywords used by them and authors referred to by them.
From figure 8 we can see the presence of the top and most relevant authors as seen in table 2 but there are other authors in this area too who have contributed to the study such as Dwivedi yk (2021), Biswas mi (2022), Bryde dj (2020) and Chowdhury s (2022).
As can be seen, the left-hand side of the figure shows the authors and papers including those which are not a part of the dataset that have been referred to by authors in the dataset. Authors such as Ivanov d, Bag s and Dhamija P are a part of our dataset they have referred to their other past work. Along with this, there are other new authors such as Baryannis g (2019), Davenport t (2018), Duan y (2019), Cavalcante i.m. (2019) and Breiman I (2001). Among all the past written papers Baryannis g has the most number of outflows (6). Most of the papers referred to by the authors are relatively recently written papers in the late 2010 s. Irrespective of this, Davis f.d. (1989) an author who wrote a paper in this study in 1989 is still being cited by many thus showing the relevance of this years-old paper in current times too.
The main theme of this research is around AI and OR, it can be observed that those areas are the ones to whom there are most outflows from the authors. But other words such as expert systems, big data, deep learning, machine learning and blockchain have also been used as keywords by authors in their study.
-
Thematic map
Thematic map is used to understand the top keywords in the area of study. The various clusters of themes are scaled on the basis of its relevance (X-axis – centrality) and development degree (Y-axis – density). The keywords used in the articles have been divided between the four quadrants on the basis of relevancy.
The top right quadrant talks about motor themes this is identified by topics that are developed and important in this research field. What can be clearly seen from figure 9 keywords such as learning-systems, industrial research and automation are the main areas in applications of AI and OR. The frequency of these words in papers is high as they are important to the area of study. The lower right quadrant depicts a basic and broad topic while the lower left quadrant indicates topics in the study that are either emerging or disappearing. The basic themes of the articles are mainly surrounding artificial intelligence, decision support systems and decision making. These are furthermore supported by central themes like neural networks and machine learning. Keywords covered in this area refer to the topics that are the basis of the research field but also those that are applicable to various other areas of research too. Papers surrounding themes of disaster and technology have been seen to have a lower density, i.e., the development around these areas, and lower centrality, i.e., the relevance to the topic, which shows that these topics do now have a direct relationship with the main research field but may see an emergence in future studies. The last and top left quadrant represents the niche areas of the broad topics shown in the lower right quadrant. With high density due to more focused studies and low centrality because of its limited use to only specific study areas, the keywords in this quadrant are human intelligent robots, robotics, quality control case-based reasoning and cryptography. Quality control case-based reasoning and cryptography have a higher niche as well as centrality among the two clusters.
With developments in AI and OR processes being continuously made step by step the cluster in the emerging trends quadrant shows low density as well as centrality. Further with their application in more areas of academia as well as management more clusters can be seen in areas directly related to the topic as well as those that are niche.
-
Cluster analysis
5.9.1 Thematic categorisation
The term "data clustering" is the combining of articles that are identical in nature and cover the same broad domain. The process of cluster analysis is shown through the placement of nodes and edges in a diagram. Densely connected nodes represent a specific cluster. A cluster mainly consists of articles that cover the same area of study and these clusters are briefly related to each other. In this work, Bibliometrix was used to group data through network analysis.
Modularity mechanism is the method used to understand the weight of the particular words density in a particular cluster compared to that is not directly in its cluster. The Louvain algorithm has been used to get an accurate basis of clusters based on the keywords. Using this algorithm on Bibliometrix, with 200 nodes set up. Four distinct clusters can be seen in the area of study. Through these clusters, it was possible to see relationships between different studies and disciplines. This allowed for a better understanding of the data and how the studies were related to each other.
The co-occurrence network in figure 10 shows the articles that are part of the dataset divided into 4 different clusters. After careful understanding of each cluster formed these can be divided into the following areas they cover. The areas of study are 1. Artificial intelligence and Decision support systems/decision making, 2. Operations research, 3. Research and management and
-
Business development and information.
Each cluster encompasses different nodes that represent unique areas of study but that are interconnected together by the use of AI and OR techniques in their field.
Cluster 1 is focused on the general applications of AI in various fields with a strong relation shown between decision support systems and decision making. It is the largest of the four clusters. Artificial intelligence is vital in decision making as it helps to take decisions within seconds rather than having to manually analyse the alternatives available to take a decision.
Authors such as Sharma A (2021), Zhang Z (2021), Rai R (2021), Kaise Fm, Bright G, Walker A (2017), Radivojevic S and Milbredt O (2016) have written articles ranging from the use of AI along with decision-making tools to improve manufacturing processes to improving the flight schedules using the same. Further, the cluster can be seen branching to other areas such as construction industry, research, genetic algorithms, information technology, forecasting and supply chain. These topics that show less attraction to the main node of AI are the edges that connect this node with the other clusters.
Cluster 2 relates the articles under AI pertaining to OR. This cluster confirms the strong relationship and interdependence of the two topics on each other. It talks about the use of AI techniques in areas of operations research such as scheduling, computer simulation, optimisation, heuristic methods and problem-solving. Operations research largely involves optimisation and optimal usage of resources to solve problems while artificial intelligence is the use of human intelligence in computer systems to perform certain tasks. AI being applied in these areas of OR is essential in current times for quick, efficient and error-free solutions. Cluster 3 comprises of studies that show a mild relationship between AI , management and research. Although showing a distant network between the articles covering topics related to AI, management and research, the network between the second cluster can be seen as well. This cluster consists of topics such as supply chain management, information management, data analytics, automation, industrial research etc. From the network of similar words, it can be understood that this cluster although dependent on other processes is indirectly dependent on AI and OR simultaneously to enhance outcomes in these areas
for businesse. It can be seen that studies have spoken about the use of AI mainly and indirectly OR techniques to help create cost-effectiveness, develop surveys and improve manufacturing.
Cluster 4 is the smallest of the four clusters. With developments in the field of AI still being seen on a daily basis, the areas where AI is being applied too is expanding day on day. A distant network with the main nodes of the study, AI and OR have had their impact on business development and innovation. For example, a study talks about the development of decision-making systems for real-time accident handling in logistics (Ngai et al. 2012). Table 8 talks about the current areas covered by researchers in this area as well as possible future areas that can be covered to develop these clusters by future academicians. Further discussions shall talk more in-depth about the future prospects of these clusters.
-
DISCUSSION AND IMPLICATIONS
-
Findings
This article has aimed to understand the articles published between 1990-2022 relating to the topic of trends in AI and OR. This study has used a combination of bibliometric analysis and literature review to get a better understanding of the areas covered and future areas that can be covered.
RQ1: To what extent has recent research in the field of AI and OR influenced decision-making capabilities for various areas of discipline?
Businesses tend to choose solutions fuelled by AI and machine learning. AI is employed in by almost every company to help them make quick decisions and minimise the probability of error across various departments as well as fields. According to Forbes, 95% of businesses need efficient processing systems to process their unstructured data (Rohit Kulkarni 2019). These processing systems are all based on OR techniques combined with the wonders of AI to reduce the chance of human error to a minimal number and maximise the efficient use of resources.
From the analysis, it can be observed that although a topic prevalent before 1990 significant developments were only made post the 1990s yet there were only 4 documents published in that year. Following this, research in AI and OR increased drastically to 66 in 2021. This huge explosion in the numbers can be owed to the ability of AI to forecast disasters, early warning systems, decision-making systems in healthcare etc. Further, the increased importance of this topic can be highlighted by a sudden increase in the number of papers published by the most relevant source i.e., Sustainability (Switzerland) from 0 papers in 2018 to 15 papers in 2021 and 19 in 2022. Thus, proving that AI has exploited the decision-making capabilities of various industries.
The keyword (figure 11 and figure 12) along with the tree word (figure 6) show areas that have been influenced by the trends in AI and OR techniques. From the two figures, the areas in real life where these have had their impact can be seen. They are supply chain management, manufacturing, industrial research, quality control systems, economics, engineering, strategic planning, forecasting disasters, customer satisfaction, finance and healthcare. Thus it can be seen that across various fields AI and OR methods have had their impact, especially in recent times.
RQ2: Understand the current trends in the application of AI and OR in terms of disciplines, years, countries and sources.
The literature that has been analysed shows that the number of papers being published in the area of applications of AI and OR has been showing an upward trend over the years as can be seen in figure 2. The number of papers published have seen a huge upward trend post the pandemic indicating the increase in applications of AI which has led to an increase in the number of studies done in this area.
Further trends in this area were analysed by understanding the country-wise contribution. It can be seen that most of the countries that are on top of production are either developed or developing countries. This shows that AI and OR applications are more developed in these parts of the world due to the access they have to resources and developments in technology in these areas. We can see from table 9 U.S.A. and China have the most number of collaborations followed by the United Kingdom and Greece and China and Australia in second and third place respectively. The top five countries that have collaborated with each other are the U.S.A., China, the United Kingdom, France and Greece. Thus, showing that these countries are sharing information and working together to expand and deepen the research available in this area.
With respect to the sources that publish papers in this area, trends can be understood. Sustainability (Switzerland) has published papers surrounding areas such as implementation of AI systems in logistics to create sustainable manufacturing (Stanisawski and Szymonik 2021), turning crisis into opportunities by using AI and OR techniques(Chen and Biswas 2021) etc. Additional to understand the trends the authors' production over the years was analysed (table 2). It can be seen that Liu Y.
had written the first paper in this area in 2013 regarding the use of AI and decision-making systems to gain competitive advantage (Liu 2013) and then directly published one paper in 2020 about spatial interpolation (Zhu et al. 2020) and one in 2021 about Intelligent Connected Vehicles (ICV) (Zhou, Liu, and Chen 2021). Thus, a notable change in the topics researched upon over the years can be observed. A similar trend of increase in publications or entry into this area of study can be seen in other authors too such as Gupta S., Zhang Y., Sharma A., and Kumar A..
RQ3: To find out what are the underlying clusters where AI and OR have been helpful over the years?
Understanding the various clusters formed by the data set helps understand the current trends in AI and OR as well as possible future ones. To understand the clusters in present literature the use of thematic evolution has been used for analysis. Figure 13 has divided the available data set into two timelines- pre-Covid and post-Covid.
During the pre-covid time, the research papers have mainly focused on broad topics such as artificial intelligence, supply chain management, learning systems, operations research and information systems. These clusters can be seen present in the co-occurrence network (figure 10).
Post-Covid academicians focused their research on more niche or refined topics under these broad ones. Hence we can see the AI segment branching into smaller segments such as sustainable development, multiple criteria decision analysis, machine learning and food supply. Newer areas of research can also be seen such as disaster management, Covid-19 and algorithm. Thus it can be seen that over the years the clusters in AI and OR trends have shifted drastically from just researching on the overview topics to more focused topics. This can be owed to the constant developments being made in this area.
RQ4: What are the gaps and areas present for future research?
With continuous new inventions and advancements being made in the use and applications of AI as well as newer OR techniques being developed the gaps in research areas are highly prevalent especially post the pandemic.
The use of decision support systems are gaining popularity in organizations, especially in those with a need to make quick decisions. But due to expeditious developments in technology and its implementation in real-life applications, there is a gap between the research done and real-life applications. Further detailed analysis can be done in the context of business processes, such as decision-making, inventory management, and so on. This would allow for a better understanding of how to optimize and automate these processes and provide benefits for companies. Another gap seen during the analysis is the lack of coordination
between different AI processes thus conducting research i this area will help improve management. Future research in this area could also focus on the development of better methods for identifying and extracting useful information from big data. Along with this understanding of the advantages and disadvantages of implementing a particular AI and OR technique in a specific discipline should also be understood and researched upon.
-
Discussions
After conducting a systematic literature review combined with bibliometric analysis this article aims to provide future academicians as well as policymakers with a deeper understanding of the trends already present in the current literature and possible future trends that can be researched upon. In the current research available, this article is not the first to conduct a bibliometric analysis but is the first to understand the overall trends in the applications of AI and OR techniques across different disciplines. Currently, the reviews done in this area focus solely on one industry or area of discipline while this study has aimed to understand the overall impact. Unlike in the extent of literature available, this dataset consists of keywords ranging from artificial intelligence, operations research, automation, expert systems, heuristic methods to mathematical models, industrial research, forecasting, construction industry, hospitality, economics. Thus, showing an overall understanding on this topic.
Taking into account the extent of available literature and analysing it based on various different parameters such as keyword analysis, author and source analysis, thematic map, cluster analysis etc. there are areas future researchers can work on. Despite there being extensive developments in this area especially in the past two to three years post the pandemic the following areas can be worked on.
Firstly, use of decision support systems are being widely used to decrease the percentage of human error to a minimal extent. According to present research, decision systems are mainly being used in areas related to supply chain, construction, quality control and economics. Future researchers can work towards understanding how to implement efficient decision-making systems using OR techniques and AI technology in other fields of work such as agriculture, education, healthcare, accounting etc. also the lack of interoperability between different decision support systems makes it difficult for organizations to take full advantage of the technology. Future articles can work towards using AI advancements to solve this problem. This will not only help ensure the smooth functioning of different firms but also different departments within the same firm.
Second, every corporate entity aims to efficiently use its resources and increase profits. To do this they implement OR techniques in their day-to-day functioning. For years operations research were done manually to find optimal results but with the progress in technology, it has been computerised. Hence in the last few years, we have seen an increase in the number of papers in this area, thus showing an increase in its applications in the real world too. But these studies mainly have focused on the more academic side of the application. In the future researchers could analyse these techniques in the context of business processes, such as decision making, inventory management, and so on. This would allow for a better understanding of how to optimize and automate these processes in real life.
The third and fourth observation to some extent overlaps each other. Most of the management decisions taken to develop businesses today are not only being taken on theories that have been stated by academicians but today are analysing the past data and past methods that have worked to come up with unique ways to solve the problem arising today. Ensuring that the company is developing sustainably is also one of the top priorities of companies in modern time. Therefore, AI and OR techniques are being used to solve these problems and reach sustainability. Mainly the research classified in this cluster deals with manufacturing, supply chain management, automation and cost-effectiveness which show their inclination towards the manufacturing processes. More understanding and research should be done on AI and OR usage to help develop other areas of the business to reach the
mark of a sustainable business. Additionally, the fourth conclusion, although not having a direct or indirect impact on the main research areas covered by researchers, states that researchers should focus on other areas too such as the development of newer methods in both AI algorithms as well OR techniques.
And lastly although throughout the benefits AI and its advancements provide to all aspects of life have been spoken about, it's important to understand that there are always two sides to a coin. Current literature talks mainly about the current benefit of using AI and OR but it is important that academicians also talk about the ill effects of implementing these such as job loss, lack of creativity and need for constant development which leads to negative consequences eventually.
-
Implications
This paper has aimed to provide academicians as well as managers and policymakers a better understanding of areas developed and areas of possible advancement in the future relating to the application of OR and AI operations.
-
Theoretical contributions
By analysing and understanding the literature that currently prevails in the area of study, it has been possible to establish the relation between the use of AI and OR processes simultaneously across various fields. Moreover, the gaps prevalent in the area of study and weaknesses have been explored, to help give future researchers a better understanding of the direction where this research topic is moving as well as its future prospectus.
Firstly, the study has shown that AI and OR are now being applied to every aspect ranging from healthcare (Jamali, Leung, and Verderber 2020) to supply chain development (Helo and Hao 2021) to textile (Sikka, Sarkar, and Garg 2022) to research management (Turban and Trippi 1989). Further by identifying the clusters present in the currently available research, the interconnection between the various clusters can be seen. This shows researchers that although they plan to study one particular area that uses AI and OR applications it is extremely important to understand that area's interdependence on other areas. Also, areas that are not developed such as business development as seen in figure 10 can help provide areas for future research.
Applications of AI are broadly known by all, so having a study that encompasses the use of both AI and OR techniques will help improve the type as well as efficiency of future research techniques. With an understanding of areas where AI and OR simultaneously can be applied researchers could suggest in which area of study and the technique that would work best. Besides that, they could work to build newer algorithms to bridge the current gaps.
-
Managerial contributions
-
Since the development of AI, it has been used in various business fields to improve the efficiency of human work and to increase the efficiency of the business. With the advent of simultaneous implementation of AI and OR trends, it is very important for managers or decision makers to understand the studies and developments that have happened and further understand ways to create advantageous situations for the business to flourish. Future studies in this area and the development of management techniques should focus on understanding two things.
The first is to keep in mind the interdependence of different areas(departments) while taking managerial decisions regarding changes in the application of AI and OR in them. The second is understanding that manual decision-making can lead to errors hence the importance of implementing AI and OR techniques to improve efficiency and at the sam time learning to maintain an equilibrium between AI adoption and the employed human workforce. Managers may also work individually on each cluster to help improve the working as well as the revenue of the company.
-
-
CONCLUSION AND LIMITATION
-
This study was undertaken to understand the extent of the impact of AI and OR applications across different processes and evaluate the current trends to predict future trends in the different areas of social sciences, business, and accounting.
With the developments in AI algorithms and their applications in the past, this topic of study has been gaining increasing relevance year on year. AI techniques have been used for years in order to provide a technological advantage. The use of AI to make decisions and operations across various fields for years to come. But the application of AI and OR techniques simultaneously has seen very few implications in real-life studies. Thus, by conducting an in-depth literature review combined with bibliometric analysis, the study has tried to answer what areas are currently being explored in this area, what gaps lie in these areas and further what future areas will AI and OR see growth and usage in.
After ensuring a thorough analysis of a dataset of 300 articles published between 1990- 2022, it can be seen that the increase in the publication trend in this area of the study saw a major rise post the impact of the pandemic. Thus, showing the increased utilization of combined AI and OR techniques due to the need of the time. This study aims to contribute as a base for future researchers to understand the current body of knowledge on AI and OR trends and to furthermore guide academicians, managers and decision-makers in the direction of evolution and what things should be kept in mind while implementing AI and OR techniques in their study or policies respectively (Kumar et al. 2022). In addition, the main contribution of this paper is the analysis of thematic areas and clusters relating to different articles in the dataset. Analysing the data available from these two methods helped propose future areas of research for academicians and managers. Thus, by understanding the previous works of authors in this field, this study provides future authors direction and a comprehensive overview of the applications of AI and OR across various time periods.
As with any other paper, our study too has a few limitations. This study has conducted the literature review by retrieving data relating to the topic of analysis from the Scopus database, further after this, a number of rounds of filtering were conducted. This could have led to disregarding papers that could have been relevant to the study. Additionally, the analysis is done from the authors own understanding this too could lead to overlooking certain analyses.
REFERENCES
[1] Bernard Marr. 2018. The 4th Industrial Revolution Is Here – Are You Ready? Forbes. 2018. https://www.forbes.com/sites/bernardmarr/2018/08/13/the- 4th-industrial-revolution-is-here-are-you-ready/?sh=7fc1607b628b. [2] Chen, Y, and M I Biswas. 2021. Turning Crisis into Opportunities: How a Firm Can Enrich Its Business Operations Using Artificial Intelligence and Big Data during Covid-19. Sustainability (Switzerland) 13 (22). https://doi.org/10.3390/su132212656. [3] Dhamija, P, and S Bag. 2020. Role of Artificial Intelligence in Operations Environment: A Review and Bibliometric Analysis. TQM Journal 32 (4): 86996. https://doi.org/10.1108/TQM-10-2019-0243. [4] Doumpos, Michalis, Constantin Zopounidis, Dimitrios Gounopoulos, Emmanouil Platanakis, and Wenke Zhang. 2022. Operational Research and Artificial Intelligence Methods in Banking. European Journal of Operational Research. https://doi.org/https://doi.org/10.1016/j.ejor.2022.04.027. [5] Goyal, Kirti, and Satish Kumar. 2021. Financial Literacy: A Systematic Review and Bibliometric Analysis. International Journal of Consumer Studies45 (1): 80105. https://doi.org/https://doi.org/10.1111/ijcs.12605.
[6] Gupta, S, S Modgil, R Meissonier, and Y K Dwivedi. 2021. Artificial Intelligence and Information System Resilience to Cope With Supply ChainDisruption. IEEE Transactions on Engineering Management. https://doi.org/10.1109/TEM.2021.3116770.
[7] Helo, P, and Y Hao. 2021. Artificial Intelligence in Operations Management and Supply Chain Management: An Exploratory Case Study. Production Planning and Control. https://doi.org/10.1080/09537287.2021.1882690. [8] IBM Cloud Education. 2020. What Is Artificial Intelligence (AI)? – India | IBM. IBM. 2020. https://www.ibm.com/in-en/cloud/learn/what-is-artificial- intelligence. [9] Ivanov, D, and B Sokolov. 2012. The Inter-Disciplinary Modelling of Supply Chains in the Context of Collaborative Multi-Structural Cyber-Physical Networks. Journal of Manufacturing Technology Management 23 (8): 97697. https://doi.org/10.1108/17410381211276835. [10] Jamali, N, R K Leung, and S Verderber. 2020. A Review of Computerized Hospital Layout Modelling Techniques and Their Ethical Implications.Frontiers of Architectural Research 9 (3): 498513. https://doi.org/10.1016/j.foar.2020.01.003.
[11] Khanra, Sayantan, Amandeep Dhir, and Matti Mäntymäki. 2020. Big Data Analytics and Enterprises: A Bibliometric Synthesis of the Literature.Enterprise Information Systems 14 (6): 73768. https://doi.org/10.1080/17517575.2020.1734241.
[12] Kumar, Devinder, Rajesh Kr Singh, Ruchi Mishra, and Samuel Fosso Wamba. 2022. Applications of the Internet of Things for Optimizing Warehousing and Logistics Operations: A Systematic Literature Review and Future Research Directions. Computers & Industrial Engineering 171: 108455. https://doi.org/https://doi.org/10.1016/j.cie.2022.108455. [13] Lamboglia, Rita, Domenica Lavorato, Eusebio Scornavacca, and Stefano Za. 2021. Exploring the Relationship between Audit and Technology. A Bibliometric Analysis. Meditari Accountancy Research 29 (5): 123360. https://doi.org/10.1108/MEDAR-03-2020-0836. [14] Liu, Y. 2013. Sustainable Competitive Advantage in Turbulent Business Environments. International Journal of Production Research 51 (10): 2821 41. https://doi.org/10.1080/00207543.2012.720392. [15] Mao, H, T Zhang, and Q Tang. 2021. Research Framework for Determining How Artificial Intelligence Enables Information Technology ServiceManagement for Business Model Resilience. Sustainability (Switzerland) 13 (20). https://doi.org/10.3390/su132011496.
[16] Min, Hokey. 2010. Artificial Intelligence in Supply Chain Management: Theory and Applications. International Journal of Logistics Research and Applications 13 (1): 1339. https://doi.org/10.1080/13675560902736537. [17] Monks, Thomas. 2016. Operational Research as Implementation Science: Definitions, Challenges and Research Priorities. Implementation Science 11 (1): 81. https://doi.org/10.1186/s13012-016-0444-0. [18] Ngai, E W T, T K P Leung, Y H Wong, M C M Lee, P Y F Chai, and Y S Choi. 2012. Design and Development of a Context-Aware Decision Support System for Real-Time Accident Handling in Logistics. Decision Support Systems 52 (4): 81627. https://doi.org/10.1016/j.dss.2011.11.016. [19] Rockwell Anyoha. 2017. The History of Artificial Intelligence – Science in the News. Harvard University. 2017. https://sitn.hms.harvard.edu/flash/2017/history-artificial-intelligence/. [20] Rohit Kulkarni. 2019. Big Data Goes Big. Forbes. 2019. https://www.forbes.com/sites/rkulkarni/2019/02/07/big-data-goes-big/?sh=29921b0120d7. [21] Sikka, M P, A Sarkar, and S Garg. 2022. Artificial Intelligence (AI) in Textile Industry Operational Modernization. Research Journal of Textile andApparel. https://doi.org/10.1108/RJTA-04-2021-0046.
[22] Stanisawski, R, and A Szymonik. 2021. Impact of Selected Intelligent Systems in Logistics on the Creation of a Sustainable Market Position ofManufacturing Companies in Poland in the Context of Industry4.0. Sustainability (Switzerland) 13 (7). https://doi.org/10.3390/su13073996. [23] Thormundsson, Bergur. 2022. Artificial Intelligence (AI) Worldwide – Statistics & Facts | Statista. Statista. 2022.
https://www.statista.com/topics/3104/artificial-intelligence-ai-worldwide/#dossierKeyfigures.
[24] Tsay, Chia-Jung, and Max H Bazerman. 2009. A Decision-Making Perspective to Negotiation: A Review of the Past and a Look to the Future.Negotiation Journal 25 (4): 46780. https://doi.org/https://doi.org/10.1111/j.1571-9979.2009.00239.x.
[25] Turban, E, and R Trippi. 1989. The Utilization of Expert Systems in OR/MS: An Assessment. Omega 17 (4): 31122. https://doi.org/10.1016/0305- 0483(89)90045-5. [26] Zhou, F, Y Liu, and R Chen. 2021. Research on Collaborative Innovation of Intelligent Connected Vehicles Industry Based on Test Field: Embedded Case Study from the Perspective of Open Innovation. Sustainability (Switzerland) 13 (11). https://doi.org/10.3390/su13115880. [27] Zhu, D, X Cheng, F Zhang, X Yao, Y Gao, and Y Liu. 2020. Spatial Interpolation Using Conditional Generative Adversarial Neural Networks.International Journal of Geographical Information Science 34 (4): 73558. https://doi.org/10.1080/13658816.2019.1599122.
[28] Zupic, Ivan, and Toma ater. 2014. Bibliometric Methods in Management and Organization. Organizational Research Methods 18 (3): 42972.https://doi.org/10.1177/1094428114562629.
Tables
Figures
__..
__….,.
_ .,..
– …., …._……. …., _…,…
60
o
20
0
Year
Figure 2: Number of a111111al publi cations di,ring the timeframe J99Q to 2Q22
Source: Biblioshiny
..
I
..-. , —.-.,·-.-. (-q,r.._-……,,_.__..__
.._o.r_o,.11-· –
Figure 3: Source Dynamics
Source: Biblioshiny
http:/AYWW .ijert.01-g
ISSN: 2278-0181
algorith
ms, 2%
0
.Q..).
,..
..
.,
,..
,…
g
,..
g
,…
,0
.,
g
0
– – –
,..
..
0
0 0,…
'°
N N N N
Year
N
–
.,
,…
0
,..
0 0
N
,..
– OEaSIONM.AtOMG – 0£CISIONSUF'PORTSYSTEMS – DESION,l.l£n4000LOQ.Y()f.CM
Vol 12 Issue 08, Au,a,,ust-2023
scheduling. 3%
design/moth
neural odology/appr
decision support
systems, 7%
optimizat i on,
2%
netv,orks, 2% each, 2%
supply chain
management,
2%
heuristic learning
methods systems,
, 2% 2%
artificialintelligence,24%
mathem
sustai11table genetic atlcal
development, algorith models,
decision making, 6% 2o/ ms, 2% 2%
'
'
I
Figure 6: Tree mae
Source: Biblioshiny
150
:!)
u
c:
Q)
100
8
:"3'
E so
u
0
0g-: g-: -g:
Term — ARTIF!CiALINTEUJOENCE
g-:
0
g
..
g
0 0
–
,,….
Figure 7: Word Dynamics
Source: Biblioshiny