
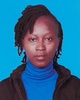
- Open Access
- Authors : Esse Sandrine Jecica
- Paper ID : IJERTV12IS040136
- Volume & Issue : Volume 12, Issue 04 (April 2023)
- Published (First Online): 24-04-2023
- ISSN (Online) : 2278-0181
- Publisher Name : IJERT
- License:
This work is licensed under a Creative Commons Attribution 4.0 International License
Survey on Visual Object Tracking Dataset
Esse Sandrine Jecica1
1School of Computer and Software
Nanjing University of Information Science and Technology (NUIST) Nanjing 210044, China
AbstractAlong with a significant amount of work on benchmark datasets required by trackers, including both long- term and short-term trackers, there has been a lot of research on visual object tracking in computer vision in recent years. Evaluation of visual object tracking has been made simpler by the development of benchmark datasets and toolkits. With more than 80 new datasets appearing in the last two years, researchers have access to a wide range of data to test and improve their tracking algorithms, leading to more accurate and reliable results. However, it is important to ensure that these datasets are diverse and representative of real-world scenarios to avoid overfitting and biased evaluations. /It is from the same view that three cutting-edge visual object tracking datasets TREK-150, EgoTracks, and BrackishMOTwill be briefly discussed in this survey. In addition, we will contrast the three most recent visual object datasets with earlier ones while outlining their unique advantages, disadvantages, and additional features. Finally, we'll share our expectations for upcoming studies.
Keywords Dataset, Computer Vision, Visual Object Tracking.
-
INTRODUCTION
With important applications ranging from robotics [1, 2] to augmented and mixed reality [3, 4, 5], visual object tracking in computer vision aims to capture the real-world perceptual problems faced by AI (artificial intelligence). It has attracted significant recent interest as an underserved but highly relevant domain of vision. Visual object tracking (VOT) is a fundamental vision problem that has been studied for over ten years. It involves the task of locating a target object in consecutive video frames and has numerous applications in several fields. Despite significant progress in recent years, VOT remains a challenging problem due to factors such as occlusion, motion blur, and changes in lighting conditions. However, VOT remains a less exploited computer vision field compared to others. This lack of interest is largely caused by the lack of a significant tracking dataset for testing and training. OTB [6], TrackingNet [7], Got10k [8], and LaSOT [9] are a few popular tracking datasets that the community has proposed in recent years. However, we find that the strong performance that state-of-the-art trackers achieve on these benchmarks does not translate well to video, demonstrating the need for such a tracking dataset. The distinction between first-person views and the more conventional third-person views of earlier datasets is what causes this performance gap. Some of these include large head movements from the person wearing the camera, object manipulations with the hands [10], frequent occlusions, quick changes in scale and pose, and potential changes in state or appearance. Additionally, first- person videos are frequently lengthy (sometimes depicting an agent or person's entire life), so the volume of the mentioned occlusions and transformations scales similarly. This
significantly increases the difficulty of tracking objects in first-person views compared to common scenarios considered in previous datasets, and their absence creates a blind spot in evaluation. Additionally, detection within tracking becomes especially crucial due to frequent object disappearances and reappearances.
Due to the small number and short duration of target object disappearances, many previous tracking datasets mainly concentrated on short-term tracking in third-person videos, which limited the ability to evaluate many of the challenges of long-term tracking. Short-term third-person video characteristics have also led to designs that rely on subtle changes in motion and appearance.
Notably, re-detection, occlusions, and short-term and longer-term tracking have long been recognized as difficult for VOT as a field, leading to recent benchmark construction efforts [11,12,13, 14] emphasizing these aspects. VOT has been continuously looking for new, better solutions to those problems, which has paid off because new discoveries are constantly emerging.
This study will focus mostly on:
-
TREK-150 [15] (TrackingEpic-Kitchens-150), which is obtained from the large and challenging First Person Vision (FPV) dataset EPIC-KITCHENS (EK) (Damen et al., 2018, 2021); First Person Vision (FPV) is the study and development of computer vision techniques that consider photos and videos taken by a person who is known as the camera wearer and who has a camera mounted on their head.TREK-150 provides 150 video sequences, which are densely annotated with the bounding boxes of a single target object the camera wearer interacts with.
-
BrackishMOT [16] is an underwater MOT dataset primarily used for underwater tracking. It can be divided into two categories: the more difficult uncontrolled natural underwater environments, and controlled environments like aquariums [17, 18]. The development of new datasets taken in different and more difficult environments is essential to advancing research in underwater MOT, and the BrackishMOT dataset is a significant contribution to this area.
-
EgoTracks [19], an ameliorated version of Ego4D described in [5], is a large-scale, long-term egocentric, or first- person visual object tracking dataset for training and evaluating long-term trackers. It is composed of unscripted, in-the-wild, egocentric videos of daily life activities, with more than 20,000 tracks from around 6,000 6-minute videos. It constitutes the first large-scale dataset for visual object tracking in egocentric videos in diverse settings, providing a new and significant challenge compared to previous datasets.
This survey discusses the three new aforementioned datasets. It will contain a general overview of the development of visual object tracking and its newly developed datasets,
then visual object tracking challenges, and finally eventual prospects.
-
-
PROGRESS ON VISUAL OBJECT TRACKING
Visual object tracking is the joint spatial-temporal localization of objects in videos. Multiple object tracking (MOT) models simultaneously detect, recognize, and track multiple objects. Without the use of detection or recognition, single object tracking (SOT) tracks a single object using an initial template that is provided. To study this significant issue, the community has developed several well-known benchmarks, including OTB [6], UAV [20], NfS [21], TC-128 [22], NUS-
PRO [23], GOT10k [8], VOT [24], TrackingNet [7], and
most recently TREK-150 [15] BrackishMOT [16] and EgoTracks [19]. Long and short-term tracking has gained more attention recently, but it presents special difficulties because of significant changes in location, displacements, disappearances, and reappearances. Some recently discovered datasets were crucial for the advancement in research in the field of VOT. We'll review three recently proposed datasets in this section.
-
TREK-150 Dataset
A brand-new dataset called TREK-150[15] has been put forth for research on the visual object tracking task, following the standard practice in the visual object tracking community that suggests setting up a small but well-described dataset to benchmark the tracking progress. It consists of 150 video sequences with a single target object labelled with a bounding box and attributes describing the target and scene's visual variation. The bounding box localization of hands and label for their state of interaction with the target object, to study the performance of trackers in the context of human-object interaction is provided. Two additional verb and noun attributes are also offered to specify the action taken by the subject and the target's class, respectively. Tables 1 and 2 compare the dataset's key statistics to the tracker evaluation benchmarks currently in use.
Table 1 Statistics of the proposed TREK-150 benchmark compared with other benchmarks designed for single visual object tracking evaluation part 1.
Attribute s
Benchmarks
OTB- 50
[48]OTB- 100
[49]TC- 128
[50]UAV1 23
[51]NUS- PRO
[52]Nfs [53]
frames across videos
Frame rate
30
FPS
30 FPS
30 FPS
30 FPS
30 FPS
240
FPS
Target object classes
10
16
27
9
8
17
Sequence attribute
11
11
11
12
12
9
Target absent labels
X
X
X
X
X
X
Labels for the interaction with the target
X
X
X
X
X
X
First Person Vision(FP V)
X
X
X
X
X
X
Action verbs
n/a
n/a
n/a
n/a
n/a
n/a
Attributes
Benchmarks
VOT-
[54]CDTB [55]
TOTB [56]
GOT- 10k [57]
LaSOT [58]
TREK- 150
Videos
60
80
225
180
280
150
Frames
20k
102k
86k
23k
685
97K
Min. frames across videos
41
406
126
51
1000
161
Mean frame across videos
332
1274
381
127
2448
649
Median frames across videos
258
1179
389
100
2102
484
Max frames across videos
1500
2501
500
920
9999
4640
Frame rate
30
FPS
30 FPS
30 FPS
10 FPS
30 FPS
60 FPS
Target object classes
30
23
15
84
70
34
Sequence
6
13
12
6
14
17
Target absent labels
Labels for the interaction with the target
X
X
X
X
X
First Person Vision (FPV)
X
X
X
X
X
Action verbs
n/a
n/a
n/a
n/a
n/a
20
Table 2 Statistics of the proposed TREK-150 benchmark compared with other benchmarks designed for single visual object tracking evaluation part 2
Attribute s
Benchmarks
OTB- 50
[48]OTB- 100
[49]TC- 128
[50]UAV1 23
[51]NUS- PRO
[52]Nfs [53]
Videos
51
100
128
123
365
100
Frames
29k
59k
55k
113k
135k
383k
Min. frames across videos
71
71
71
109
146
169
Mean frame across videos
578
590
429
915
371
3830
Median frames across videos
392
393
365
882
300
2448
Max
3872
3872
3872
3085
5040
20665
-
BrackishMOT Dataset
The underwater MOT domain has not seen a significant increase in novel algorithms for the past decade due to a lack of publicly available annotated underwater datasets. This has
limited the ability of researchers to develop and test new methods for underwater object detection and tracking, which are crucial for applications such as marine biology, underwater exploration, and search as well as rescue operations. Therefore, there is a need for more effort to create annotated datasets that can facilitate the development of novel algorithms in this domain. These datasets can be divided into two categories: controlled environments such as aquariums [17, 18] and more challenging uncontrolled natural underwater environments. One of the earliest datasets used for tracking fish captured in the wild was the Fish4Knowledge (F4K) dataset [25].
The F4K dataset was captured more than a decade ago in
-
Class distribution of the
-
brackishMOT dataset based on the number
of bounding boxes.
(b) Boxplot showing the distribution
of the traveled distance between consecutive frames measured in
tropical waters off the coast of Taiwan at a low resolution and low frame rate. Two underwater object tracking datasets, UOT32[26]and UOT100[27], were published with annotated underwater sequences sourced from YouTube videos. A high- resolution underwater MOT dataset, FISHTRAC [28], was recently proposed, but only three training videos (671 frames in total) with few objects and little occlusion have been published.
The BrackishMOT dataset is an important contribution to underwater MOT research, as it covers a diverse range of underwater ecosystems with less colorful fish and more turbid water. By expanding the scope of the research to include more diverse environments, the findings will have greater applicability and relevance to real-world scenarios, enabling researchers to develop more robust and effective underwater MOT systems that can be used in a variety of settings.
The Brackish Dataset [29] was published in 2019 to advance object detection in brackish waters. It consisted of 89 sequences of manually annotated bounding boxes of six coarse classes: fish, crab, shrimp, starfish, small fish, and jellyfish. Examples from the original daaset can be seen in Figure 1. In Figure 2, two charts illustrate the motion and class distribution for the dataset. The crab and starfish classes barely move compared to the rest and are well-camouflaged. The class distribution presented in Figure 2b shows that the dataset is imbalanced with few occurrences of the shrimp, fish, and jellyfish classes. As the small fish class exhibits erratic motion and appears in groups, it is deemed the most interesting class with respect to MOT.
Fig. 1 Image samples from the Brackish Dataset [30]. In most of the sequences containing the small fish class, there are multiple specimens forming a school of fish
Fig. 2 Plots describing the composition of the brackishMOT dataset with respect to motion and class distribution. For both plots, the data is from all the sequences
-
-
EgoTracks Datase
EgoTracks is a large-scale, long-term egocentric single object tracking dataset, consisting of a total of 22.42K tracks from 5.9K videos. Egocentric video datasets have been introduced
in the past decades [5][3031, 32, 33, 34]. offering a host
of interesting challenges such as activity recognition [35], [36], [37], [38], anticipation [39, 40, 41], video
summarization [42], [31], [43], [44], human-object
interaction [45], episodic memory [5], the visual query [5], and camera-wearer pose inference [46]. To tackle its challenges, tracking is leveraged in many methodologies [5], [47], [43], [45] yet few works have been dedicated to this fundamental problem on its own. However, those that have started to recognize the challenges of egocentric object tracking [15], [48] have started to recognize the challenges at smaller scales.
EgoTracks is a large-scale testbed for developing tracking
methods dedicated to egocentric videos. It is annotated on a subset of Ego4D [5]. Ego4D's baseline approach relies heavily on tracking methods such as Siam-RCNN and KYS for global and local tracking. It proposes many novel tasks, such as Episodic Memory, with tracking identified as a core component. EPIC-KITCHENS VISOR [13] was introduced concurrently, annotating short-term (12 sec on average) videos from EPIC-KITCHENS with instance segmentation masks. EgoTracks offers multiple unique values complementary to EPICVISOR: long-term tracking (6 min vs. 12 sec), significantly larger-scale (6.9k video clips vs. 158), and more diversified video sources (80+ scenes vs. kitchen-only). This task is closely related to long-term tracking, as finding an object in a video given a visual template is identical to the re-detection problem in the long- term tracking literature. In addition to a larger scale than previous datasets, the scenarios captured by EgoTracks represent a significantly harder challenge for SOTA trackers, suggesting room for improved tracking methodology.
Datasets
Attributes
Video Hours
Avg. Length (s)
Ann. FPS
Ann. Type
Egocentric
SOTA (P/AO)
ImageNet- Vid [63]
15.6
10.6
25
mask
No
YT-VOS [78]
5.8
4.6
5
mask
No
-/83.6
[30]DAVIS 17
[61]0.125
3
24
mask
No
-/86.3
[7]TAO [14]
29.7
36.8
1
mask
No
UVO [74]
2.8
10
30
mask
No
-/73.7
[58]EPIC- KITCHENS VISOR [13]
36
12
0.9
mask
Yes
-/74.2
[58]GOT-10k [31]
32.8
12.2
10
bbox
No
-/75.6 [9]
OxUvA [69]
14.4
141.2
1
bbox
No
LaSOT [20]
31.92
82.1
30
bbox
No
80.3/- [9]
TrackingNet [57]
125.1
14.7
28
bbox
No
86/- [9]
EgoTracks
602.9
367.9
5
bbox
Yes
45/54.1
Table 3 Object tracking datasets comparison
-
-
CHALLENGE FOR PRACTICAL VISUAL TRACKING SYSTEM
Visual tracking is difficult due to the complex and dynamic nature of real-world environments. These challenges can be classified into two types: robustness-related, and efficiency related. The robustness challenge requires a visual tracker to achieve high-accuracy results. However, the target's appearance in a video sequence can suffer from changes due to occlusion, deformation, fast motion, motion blur, rotation, being out of view, distractor effects, scale variation, and illumination change. Deep features can help alleviate these issues, but tracking performance is still poor in the presence of occlusion, objects out of view, and distractor objects. The most important details are that, in both occlusions and out of view, the target object may disappear, making it difficult to accurately relocate it. Additionally, when distractions that are visually similar to the target exist, the tracker may drift to the background distractor region. Adversarial attacks can also pose a threat to tracking robustness. The attack model adds imperceptible noise to the video frames, causing tracking failure. To achieve high efficiency, a diverse set of visual object-tracking datasets is needed. Deep-tracking approaches require huge computing resources, but when deployed on mobile devices, they may suffer from heavy time delays in reporting the target position, limiting their applications. To improve the performance and generalization of the tracker, a diverse set of visual objects tracking datasets is needed. The newly discovered datasets TREK-150, Braish MOT, and EgoTracks help improve tracking efficiency on mobile or edge devices while maintaining accuracy. The computer vision community has made significant progress in the development of algorithms capable of tracking arbitrary objects in unconstrained scenarios affected by those issues. The advancements have been possible thanks to the development of new and effective tracking principles (There are no sources in the current document.et al., 2010; Bertinetto et al., 2016b; Bhat et al., 2019; Dai et al., 2020; Danelljan et al., 2017a; Henriques et al., 2015; Guo et al., 2021; Zhang et al., 2020; Yan et al., 2021), and to the careful design of benchmark datasets (Fan et al., 2019; Galoogahi et al., 2017; Huang et al., 2019; Li et al., 2016; Mueller et al., 2016; Wu et al., 2015) and competitions (Kristan et al., 2017, 2019, 2020,
2021) that will represent the aforementioned challenging situations. However, all these research endeavours have considered mainly the classic third-person scenario in which the target objects are passively observed from an external point of view and where they do not interact with the camera wearer. It is a matter of fact that the nature of images and videos acquired from the first-person viewpoint is inherently different from the type of image captured from video cameras set to an external point of view.
-
FUTURE RESEARCH DIRECTION
Despite considerable progress in recent years, many problems remain unsolved in visual object tracking. In the following, we will discuss several potential research drections for visual tracking. First, one of the promising directions is to develop unsupervised tracking models. Currently, deep trackers usually require a large set of labelled videos for training. However, the annotation of these videos is expensive and time-consuming. Especially, as the model increases in the future, more labelled training data is desired, which may significantly hinder the further development of visual tracking. Addressing this, a potential solution is to develop unsupervised tracking models that can automatically learn from videos without human labels. Recently, several attempts have been made at unsupervised tracking. However, the performance of these unsupervised trackers falls far behind the supervised visual trackers. Further study is needed to investigate unsupervised visual tracking. Second, it is worth exploring an effective pre-training strategy for tracking. Existing tracking models often leverage the pre-trained image classification model for training. However, due to the domain gap, it may not be optimal to use the parameters of the image classification model. Instead, a dedicated, generic, pre-trained tracking model is needed. Self-supervised learning approaches can be borrowed to pre-train a universal large- scale tracking model. When developing new trackers, the pre- trained tracking model can be directly adopted for feature extraction without fine-tuning, simplifying the pipeline for new algorithm design. Third, it is crucial to exploit distractor information in videos for tracking (Datasets). Currently, most trackers aim to localize the target of interest while ignoring visually similar distractors in the videos (Datasets), resulting in drift or even failure. To alleviate this issue, a future direction is to simultaneously locate the target object and similar distractors to provide more information for distinguishing the target from the background. Finally, to improve the inference efficiency of visual trackers, a feasible solution is network distillation. Network distillation aims to transfer crucial knowledge from the large-scale teacher network to a small-scale student network. For visual tracking, given a trained teacher tracker, our goal is to train a student tracker that performs similarly during inference while running much faster.
-
CONCLUSION
Initially, extensive experiments were conducted to understand the performance of state-of-the-art trackers on the new datasets (TREK-150, BrackishMOT and EgoTracks datasets) and found that they struggled considerably. Moreover, it was found that the most challenging factors for trackers were the target being out of view, full occlusions, low resolution, and
the presence of similar objects or fast motion in the scene. In conclusion, we can say that the findings of the new visual object tracking datasets have significant implications for future research in the computer vision field in general and visual object tracking in particular. Thus, further investigations are needed to explore the long-term effects of these interventions and to develop more effective strategies for addressing this issue
ACKNOWLEDGMENT
The editors and anonymous reviewers are both acknowledged by the writers for their valuable comments.
REFERENCES
[1] M. Savva et al., Habitat: A platform for embodied AI research, in Proceedings of the IEEE International Conference on Computer Vision, 2019. doi: 10.1109/ICCV.2019.00943. [2] J. Duan, S. Yu, H. L. Tan, H. Zhu, and C. Tan, A Survey of Embodied AI: From Simulators to Research Tasks, IEEE Trans Emerg Top Comput Intell, vol. 6, no. 2, 2022, doi: 10.1109/TETCI.2022.3141105. [3] R. T. Azuma, A survey of augmented reality. Presence: Teleoperators and Virtual Environments, Chaos Solitons Fractals, vol. 42, no. 3, 1997. [4] M. Speicher, B. D. Hall, and M. Nebeling, What is mixed reality?, in Conference on Human Factors in Computing Systems – Proceedings, 2019. doi: 10.1145/3290605.3300767. [5] K. Grauman et al., Ego4D: Around the World in 3,000 Hours of Egocentric Video, in Proceedings of the IEEE Computer Society Conference on Computer Vision and Pattern Recognition, 2022. doi: 10.1109/CVPR52688.2022.01842. [6] Y. Wu, J. Lim, and M.-H. Yang, Online Object Tracking: A Benchmark Supplemental Material, 2013 IEEE Conference on Computer Vision and Pattern Recognition, 2013. [7] M. Müller, A. Bibi, S. Giancola, S. Alsubaihi, and B. Ghanem, TrackingNet: A Large-Scale Dataset and Benchmark for Object Tracking in the Wild, in Lecture Notes in Computer Science (including subseries Lecture Notes in Artificial Intelligence and Lecture Notes in Bioinformatics), 2018. doi: 10.1007/978-3-030- 01246-5_19. [8] L. Huang, X. Zhao, and K. Huang, Got-10k: A large high-diversity benchmark for generic object tracking in the wild, IEEE Trans Pattern Anal Mach Intell, vol. 43, no. 5, 2021, doi: 10.1109/TPAMI.2019.2957464. [9] H. Fan et al., Lasot: A high-quality benchmark for large-scale single object tracking, in Proceedings of the IEEE Computer Society Conference on Computer Vision and Pattern Recognition, 2019. doi: 10.1109/CVPR.2019.00552. [10] D. Shan, J. Geng, M. Shu, and D. F. Fouhey, Understanding Human Hands in Contact at Internet Scale, in Proceedings of the IEEE Computer Society Conference on Computer Vision and Pattern Recognition, 2020. doi: 10.1109/CVPR42600.2020.00989. [11] A. Moudgil and V. Gandhi, Long-Term Visual Object Tracking Benchmark, in Lecture Notes in Computer Science (including subseries Lecture Notes in Artificial Intelligence and Lecture Notes in Bioinformatics), 2019. doi: 10.1007/978-3-030-20890-5_40. [12] J. Valmadre et al., Long-Term Tracking in the Wild: A Benchmark, in Lecture Notes in Computer Science (including subseries Lecture Notes in Artificial Intelligence and Lecture Notes in Bioinformatics), 2018. doi: 10.1007/978-3-030-01219-9_41. [13] L. Huang, X. Zhao, and K. Huang, Globaltrack: A simple and strong baseline for long-term tracking, in AAAI 2020 – 34th AAAI Conference on Artificial Intelligence, 2020. doi: 10.1609/aaai.v34i07.6758. [14] P. Voigtlaender, J. Luiten, P. H. S. Torr, and B. Leibe, Siam R-CNN: Visual tracking by re-detection, in Proceedings of the IEEE Computer Society Conference on Computer Vision and Pattern Recognition, 2020. doi: 10.1109/CVPR42600.2020.00661. [15] M. Dunnhofer, A. Furnari, G. M. Farinella, and C. Micheloni, Visual Object Tracking in First Person Vision, Int J Comput Vis, vol. 131, no. 1, pp. 259283, 2023, doi: 10.1007/s11263-022-01694-6. [16] M. Pedersen, D. Lehotský, I. Nikolov, and T. Moeslund,BrackishMOT: The Brackish Multi-Object Tracking Dataset. 2023. [17] F. Romero-Ferrero, M. G. Bergomi, R. C. Hinz, F. J. H. Heras, and G.
G. de Polavieja, idtracker.ai: tracking all individuals in small or large collectives of unmarked animals, Nat Methods, vol. 16, no. 2, 2019, doi: 10.1038/s41592-018-0295-5.
[18] M. Pedersen, J. B. Haurum, S. H. Bengtson, and T. B. Moeslund, 3D- ZEF: A 3D zebrafish tracking benchmark dataset, in Proceedings of the IEEE Computer Society Conference on Computer Vision and Pattern Recognition, 2020. doi: 10.1109/CVPR42600.2020.00250. [19] H. Tang, K. Liang, K. Grauman, M. Feiszli, and W. Wang, EgoTracks: A Long-term Egocentric Visual Object Tracking Dataset. 2023. doi: 10.48550/arXiv.2301.03213. [20] [20] M. Mueller, N. Smith, and B. Ghanem, A Benchmark and Simulator for UAV Tracking-Supplementary Material, European Conference on Computer Vision (ECCV16), 2016. [21] H. K. Galoogahi, A. Fagg, C. Huang, D. Ramanan, and S. Lucey, Need for Speed: A Benchmark for Higher Frame Rate Object Tracking, in Proceedings of the IEEE International Conference on Computer Vision, 2017. doi: 10.1109/ICCV.2017.128. [22] P. Liang, E. Blasch, and H. Ling, Encoding Color Information for Visual Tracking: Algorithms and Benchmark, IEEE Transactions on Image Processing, vol. 24, no. 12, 2015, doi: 10.1109/TIP.2015.2482905. [23] A Li, M. Lin, Y. Wu, M. H. Yang, and S. Yan, NUS-PRO: A NewVisual Tracking Challenge, IEEE Trans Pattern Anal Mach Intell, vol. 38, no. 2, 2016, doi: 10.1109/TPAMI.2015.2417577.
[24] M. Kristan et al., A Novel Performance Evaluation Methodology for Single-Target Trackers, IEEE Trans Pattern Anal Mach Intell, vol. 38, no. 11, 2016, doi: 10.1109/TPAMI.2016.2516982. [25] D. Giordano, S. Palazzo, and C. Spampinato, Fish4Knowledge: Collecting and Analyzing Massive Coral Reef Fish Video Data, Intelligent Systems Reference Library, vol. 104, 2016. [26] L. Kezebou, V. Oludare, K. Panetta, and S. S. Agaian, Underwater Object Tracking Benchmark and Dataset, in 2019 IEEE International Symposium on Technologies for Homeland Security, HST 2019, 2019. doi: 10.1109/HST47167.2019.9032954. [27] K. Panetta, L. Kezebou, V. Oludare, and S. Agaian, Comprehensive Underwater Object Tracking Benchmark Dataset and Underwater Image Enhancement with GAN, IEEE Journal of Oceanic Engineering, vol. 47, no. 1, 2022, doi: 10.1109/JOE.2021.3086907. [28] T. Mandel et al., Detection confidence driven multi-object tracking to recover reliable tracks from unreliable detections, Pattern Recognit, vol. 135, 2023, doi: 10.1016/j.patcog.2022.109107. [29] M. Pedersen, J. B. Haurum, R. Gade, T. B. Moeslund, and N. Madsen, Detection of marine animals in a new underwater dataset with varying visibility, in IEEE Computer Society Conference on Computer Vision and Pattern Recognition Workshops, 2019. [30] D. Damen et al., Scaling Egocentric Vision: The EPIC-KITCHENS Dataset, in Lecture Notes in Computer Science (including subseries Lecture Notes in Artificial Intelligence and Lecture Notes in Bioinformatics), 2018. doi: 10.1007/978-3-030-01225-0_44. [31] Y. J. Lee, J. Ghosh, and K. Grauman, Discovering important people and objects for egocentric video summarization, in Proceedings of the IEEE Computer Society Conference on Computer Vision and Pattern Recognition, 2012. doi: 10.1109/CVPR.2012.6247820. [32] Y. C. Su and K. Grauman, Detecting engagement in egocentric video, in Lecture Notes in Computer Science (including subseries Lecture Notes in Artificial Intelligence and Lecture Notes in Bioinformatics), 2016. doi: 10.1007/978-3-319-46454-1_28. [33] H. Pirsiavash and D. Ramanan, Detecting activities of daily living in first-person camera views, in Proceedings of the IEEE Computer Society Conference on Computer Vision and Pattern Recognition, 2012. doi: 10.1109/CVPR.2012.6248010. [34] A. Fathi, J. K. Hodgins, and J. M. Rehg, Social interactions: A first- person perspective, in Proceedings of the IEEE Computer Society Conference on Computer Vision and Pattern Recognition, 2012. doi: 10.1109/CVPR.2012.6247805.E. Kazakos, A. Nagrani, A. Zisserman, and Di. Damen, EPIC-fusion: Audio-visual temporal binding for egocentric action recognition, in Proceedings of the IEEE International Conference on Computer Vision, 2019. doi: 10.1109/ICCV.2019.00559.
[35] Y. Li, T. Nagarajan, B. Xiong, and K. Grauman, Ego-Exo: Transferring Visual Representations from Third-person to First-person Videos, in Proceedings of the IEEE Computer Society Conference onComputer Vision and Pattern Recognition, 2021. doi: 10.1109/CVPR46437.2021.00687.
[36] W. Wang, D. Tran, and M. Feiszli, What Makes Training Multi- Modal Classification Networks Hard?, in Proceedings of the IEEE Computer Society Conference on Computer Vision and Pattern Recognition, 2020. doi: 10.1109/CVPR42600.2020.01271. [37] D. Ghadiyaram, D. Tran, and D. Mahajan, Large-scale weakly- supervised pre-training for video action recognition, in Proceedings of the IEEE Computer Society Conference on Computer Vision and Pattern Recognition, 2019. doi: 10.1109/CVPR.2019.01232. [38] Y. A. Farha, A. Richard, and J. Gall, When will you do what? – Anticipating Temporal Occurrences of Activities, in Proceedings of the IEEE Computer Society Conference on Computer Vision and Pattern Recognition, 2018. doi: 10.1109/CVPR.2018.00560. [39] A. Furnari and G. M. Farinella, Rolling-Unrolling LSTMs for Action Anticipation from First-Person Video, IEEE Trans Pattern Anal Mach Intell, vol. 43, no. 11, 2021, doi: 10.1109/TPAMI.2020.2992889. [40] R. Girdhar and K. Grauman, Anticipative Video Transformer, in Proceedings of the IEEE International Conference on Computer Vision, 2021. doi: 10.1109/ICCV48922.2021.01325. [41] A. G. Del Molino, C. Tan, J. H. Lim, and A. H. Tan, Summarization of Egocentric Videos: A Comprehensive Survey, IEEE Transactions on Human-Machine Systems, vol. 47, no. 1. 2017. doi: 10.1109/THMS.2016.2623480. [42] Y. J. Lee and K. Grauman, Predicting Important Objects for Egocentric Video Summarization, Int J Comput Vis, vol. 114, no. 1, 2015, doi: 10.1007/s11263-014-0794-5. [43] Z. Lu and K. Grauman, Story-driven summarization for egocentric video, in Proceedings of the IEEE Computer Society Conference on Computer Vision and Pattern Recognition, 2013. doi: 10.1109/CVPR.2013.350. [44] M. Liu, S. Tang, Y. Li, and J. M. Rehg, Forecasting human object interaction: Joint prediction of motor attention and egocentric activity, ArXiv, 2019. [45] H. Jiang and K. Grauman, Seeing invisible poses: Estimating 3D body pose from egocentric video, in Proceedings – 30th IEEE Conference on Computer Vision and Pattern Recognition, CVPR 2017, 2017. doi: 10.1109/CVPR.2017.373. [46] C. Lu, R. Liao, and J. Jia, Personal object discovery in first-person videos, IEEE Transactions on Image Processing, vol. 24, no. 12, 2015, doi: 10.1109/TIP.2015.2487868. [47] M. Dunnhofer, A. Furnari, G. M. Farinella, and C. Micheloni, Is First Person Vision Challenging for Object Tracking?, in Proceedings ofthe IEEE International Conference on Computer Vision, 2021. doi: 10.1109/ICCVW54120.2021.00304.
[48] Y. Wu, J. Lim and M. -H. Yang, "Online Object Tracking: A Benchmark," 2013 IEEE Conference on Computer Vision and Pattern Recognition, Portland, OR, USA, 2013, pp. 2411-2418, doi: 10.1109/CVPR.2013.312. [49] Wu Y, Lim J, Yang MH. Object Tracking Benchmark. IEEE Trans Pattern Anal Mach Intell. 2015 Sep;37(9):1834-48. doi: 10.1109/TPAMI.2014.2388226. PMID: 26353130. [50] P. Liang, E. Blasch and H. Ling, "Encoding Color Information for Visual Tracking: Algorithms and Benchmark," in IEEE Transactions on Image Processing, vol. 24, no. 12, pp. 5630-5644, Dec. 2015, doi: 10.1109/TIP.2015.2482905 [51] Mueller, M., Smith, N., Ghanem, B. (2016). A Benchmark and Simulator for UAV Tracking. In: Leibe, B., Matas, J., Sebe, N., Welling, M. (eds) Computer Vision ECCV 2016. ECCV 2016. Lecture Notes in Computer Science(), vol 9905. Springer, Cham. https://doi.org/10.1007/978-3-319-46448-0_27 [52] Li, A., Lin, M., Wu, Y., Yang, M. H., & Yan, S. (2016). NUS-PRO: Anew visual tracking challenge. IEEE Transactions on Pattern Analysis and Machine Intelligence, 38(2), 335349
[53] Galoogahi, H. K., Fagg, A., Huang, C., Ramanan, D., & Lucey, S. (2017). Need for speed: A benchmark for higher frame rate object tracking. In ICCV [54] Kristan, M., Matas, J., Leonardis, A., Felsberg, M., Pflugfelder, R., Kämäräinen, J.K., Zajc, L., Drbohlav, O., Lukeic, A., Berg, A., Eldesokey, A., Käpylä, J., Fernández, G., et al. (2019). The seventh visual object tracking VOT2019 challenge results. In ICCV [55] Lukezic, A., Kart, U., Kapyla, J., Durmush, A., Kamarainen, J. K., Matas, J., Kristan, M. (2019). CDTB: A color and depth visual object tracking dataset and benchmark. In ICCV [56] Fan, H., Miththanthaya, H. A., Harshit, S. R. Rajan, L. X., Zou, Z., Lin, Y., & Ling, H. (2021). Transparent object tracking benchmark. In ICCV. [57] Huang, L., Zhao, X., & Huang, K. (2019). GOT-10k: A large high diversity benchmark for generic object tracking in the wild. IEEE Transactions on Pattern Analysis and Machine Intelligence, 43(5), 15621577. [58] Fan, H., Lin, L., Yang, F., Chu, P., Den, G., Yu, S., Bai, H., Xu, Y.,Liao, C., & Ling, H. (2019). LaSOT: A High-quality Benchmark for Large-scale Single Object Tracking. In CVPR