
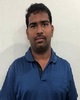
- Open Access
- Authors : Podilapu Asish Goutham , K. Venkata Subbaiah
- Paper ID : IJERTV11IS060128
- Volume & Issue : Volume 11, Issue 06 (June 2022)
- Published (First Online): 18-06-2022
- ISSN (Online) : 2278-0181
- Publisher Name : IJERT
- License:
This work is licensed under a Creative Commons Attribution 4.0 International License
Industrial Virtual Power Plant on Renewable Energy Integration in India
Podilapu Asish Goutham1
Department of Mechanical Engineering,
A.U college of Engineering,
Andhra University, 530003 Visakhapatnam, India
Prof. K. Venkata Subbaiap
Department of Mechanical Engineering,
-
college of Engineering, A
ndhra University, 530003 Visakhapatnam, India
Abstract An industrial demesne is one of the typical energy consumption strategy in power systems owing to the heavy commercial loads and their capacities to respond to electricity price changes. Thus energy integration in the industrial sector is significant. The conception of industrial virtual power plant (IVPP) has been proposed to deal with similar problems. This study demonstrates an IVPP model to manage areas in and co- industrial demesne, including energy storehouse systems, demand response (DR) supply and distributed powers. In addition, fuzzy proposition is used to change the deterministic system constraints to fuzzy parameters. The additional benefits of the IVPP possessors in day ahead trades, DR and energy storehouse systems can be listed economically. Thus the energy between the grid and IVPP can flow in both directions the excess renewable electricity of IVPP can be vended in the trade; when the electricity generated inside IVPP isn't enough for its use IVPP can also buy power through the trade.
Case studies grounded on two scripts demonstrate the effective solidarity between two IVPP areas. The confirmation results indicate that IVPP can optimize the force and demand areas in commercial premises there by de carbonizing the power systems.
Keywords- IVVP, Demand Response, Fuzzy, Renewable Energy
-
INTRODUCTION
The energy extremity is getting severe with the rapid fire growth of electricity demand. The quality of the conditions in fast growing husbandry is also deteriorating, particularly in industrial demesne with heavy loads.
Energy storage systems and controllable loads are introduced into the electricity systems to compensate for the essential intermittency and query of renewable powers.
The use of coal for energy product has proven to be one of the main contributors to climate change. Thermal shops are a source of carbon dioxide emigrations. Indeed moment, these sources of energy are wide. This air pollution is estimated to be get further than asthma attacks each time around the world.
To contribute this positive and sustainable trend, disquisition on wind energy and wind turbines must continue and take a vault forward.
Generation of electrical energy in centralized power
shops has multi advantages analogous as low cost of product, high effectiveness and easy control, reliable operation, etc. On the other hand, conventional generation has some disadvantages too.
High power losses, high cost of infrastructures, air pollution, reactionary powers reduction etc are the reasons that lead us to use renewable powers in decentralized generation schemes. The addition in the global warming and environmental issues causes further sweats for chancing a concession for reactionary powers and conventional generation.
The generality of virtual power plant (VPP) has been proposed as a successful system to aggregate distributed demand response (DR) and energy storage systems to maximize profit in the electricity trade.
A virtual power plant is a system that integrates several types of power sources to give a reliable overall power force. The sources constantly form a cluster of different types of dis patchable and non dis patchable, controllable or flexible weight systems that are controlled by a central authority and this system has benefits analogous as the capability to deliver peak weight electricity. Such a VPP can replace a conventional power plant while furnishing advanced effectiveness and farther strictness, which allows the system to reply better to load oscillations.
The centralized mode is a hotspot that the generality of VPP can be extended to multiple geographical areas, which is suitable for decentralized modes. Also different logically or physically separated factors of VPP can be controlled progressively via the multi agent system.
The fine optimization styles of VPP are predicated on direct programming (LP), mixed integer direct programming, intelligent algorithms and others.
A. OBJECTIVE OF THE STUDY
-
The end of a VPP is subject to its operation trade.
-
Virtual power shops are meant to network distributable energy means, which substantially include renewable sources of energy similar as hydro power, wind, biomass, and solar as well as energy
store house systems and flexible power consumers, which can also be appertained to as demand side operation or demand response.
-
The VPP is to read, cover, dispatch and optimize the consumption and generation of these energy areas.
-
VPP ensures effectiveness in energy areas Through the VPP from where different energy areas, whether the distributed energy asset, energystorehouse system or demand point operation are added up.
-
Effectiveness and trust ability in renewable energy can be boosted by striking a balance between power consumption and power generation by considering all the variables.
-
Ideal of the Virtual power factory is the integration of renewable sources of energy into the currentenergy trade.
-
Individual power shops may not meet all the trade demands because of the vast trade conditions for energy trust ability and vacuity, VPP combines different energy enterprises and thereby suitable to trade in a big trade and serve those with high energy requirements similar as commercial consumers.
-
VPP serves as a conciliator between the non commercial energy trade and the DERs and trades energy on behalf of the DER possessors because, by themselves, these possessors cannot share fairly in the trades.
-
-
METHODOLOGY
-
-
CONCEPTION OF VIRTUAL POWER PLANT
The conception of VPP was first conceived by Awerbuch as a virtual mileage that provides perceptivity into the paradigm shift of the restructured mileage assiduity.
VPP consists of several types of power generation areas, similar as wind and solar. It composes the VPP of distributed energy supplies, energy storehouse systems and dis patchable classical creators. Other scholars extended the conception of VPP to consumers.
-
WIND ENERGY
Wind Energy is a formation of solar energy. Wind energy describes the process by which wind is use to induce electricity.
Wind power works to ameliorate the performance, lower the costs, and accelerate the deployment of innovative wind and water power technologies. Greater use of the nation's abundant wind and water way for electric power generation will help stabilize energy costs, enhance energy security, and ameliorate our terrain.
-
WIND FARM DEVELOPMENT
-
Siting a wind ranch varies from one position to another, but there are some important matters for land possessor to consider
-
Understand your wind resource
-
Determine benefits of and walls to allowing your land to be developed
-
Establish access to capital
-
Identify dependable power purchaser or trade
-
Establish dialogue with turbine manufacturers and design inventors
Figure 1: Wind Power Plant
-
-
SOLAR ENERGY
Solar energy is the cleanest and most abundant renewable nergy source available and India has some of the richest solar supplies in the world. Solar technology can harness this energy for generating electricity, furnishing light or a comfortable interior terrain and heating water for domestic, marketable or commercial use.
Solar radiation is light also known as electromagnetic radiation that's emitted by the sun. While every position on Earth receives some sun over a time, the quantum of solar radiation that reaches any one spot on the Earths face varies.
Photovoltaic induce electricity directly from sun via an electronic process and can be used to power anything from small electronics similar as calculators and road signs up to homes and large marketable businesses. Solar energy is a veritably flexible energy technology it can be erected as distributed generation or as a central station, mileage scale solar power factory. Both of these styles can also store the energy theyproduce for distribution after the sun sets, using cutting edge solar and storehouse technologies. Solar exists within a complex and interrelated electricity system working alongside other technologies like wind power to transition a clean energy frugality.
All of these operations depend on probative policy fabrics at the original, state and civil position to
insure consumers and businesses have fair access to clean energy technologies like solar.
Figure 2: Solar Power Plant
-
DEMAND RESPONSE
Demand response provides an occasion for consumers to play a significant part in the operation of the electric grid by reducing or shifting their electricity operation during peak ages in response to time grounded rates or other forms of fiscal impulses.
Demand response programs are being used by some electric system initiatives and drivers as resource options for balancing force and demand. Similar programs can lower the cost of electricity in non commercial trades and in turn, lead to lower retail rates. Styles of engaging guests in demand response sweats include offering time grounded rates similar as time of use pricing, critical peak pricing, variable peak pricing, real time pricing and critical peak rebates.
It also includes direct freight control programs which give the capability for power companies to cycle air conditioners and water heaters on and off during ages of peak demand in exchange for a fiscal incitement and lower electric bills. The electric power assiduity considers demand response programs as decreasing precious resource option whose capabilities and implicit impacts are expanded by grid modernization sweats. For illustration, detectors can perceive peak place problems and use automatic switching to divert or reduce power in strategic places, removing the chance of load and the performing power failure. Advanced metering structure expands the range of time grounded rate programs that can be offered to consumers.
One of the pretensions of the Smart Grid R&D Program is to develop grid modernization technology, tools and ways to use demand response and help the power assiduity design, test and demonstrate integrated public electric communication information architectures with the capability to stoutly optimize grid operations and incorporate demand response through consumer participation.
Chao H, Quan D, Yang T (2018) used heritable algorithms to configure the power of each distributed generation.
Wind power auguring has changeable volatility and intermittency. Algorithms used in models with shifting wind power include fuzzy optimization, robust optimization and stochastic optimization.
Zhou R, Zhang J, Zhang H, etal. (2018) indicates that compared with stochastic optimization and robust optimization, fuzzy optimization has several advantages, analogous as no statistical crimes and conservative and malleable cast results, when dealing with wind power auguring problems. This study chooses fuzzy optimization.VPP can be applied to numerous situations.
Soltani M, Raoofat M, Rostami MA (2012) states that VPP can contribute in the frequency control tradeand give peak shearing service.
Baringo A (2018) has reported that a VPP multi element system participates in energy and reserve electricity trades as a single reality.
Dumbrava (2015) studied the possibility of VPP owners participating in the green instrument trade and in the day ahead trade simultaneously.
-
WIND POWER FORECASTING
Fuzzy model is used when estimating the wind power, the predicted value, predicted base value, predicting error and predicting error measure are calculated.
Wind power estimations are veritably important to reduce the query and schedule the electricity generation especially in power systems have a high rate wind power.
Wind power estimations are classified according to the two different criteria which are time span and structure.
Estimations are divided in four groups as long term, medium term, short term and veritably short term in term of timespan.
Long term estimations are from weeks to months or times and used for wind power and power system planning. Medium term estimations are from days to weeks and used unit commitment and conservation scheduling.
Short term estimations are from hours to days and used for profitable dispatch, reserve demand, intraday trade and day ahead trades.
Veritably short term cast are seconds to twinkles and used for wind turbine control and power systemfrequency control.
The estimations are also divided in three groups as physical, statistical and cold- blooded in terms of structure. In physical model, physical structure of wind power shops and numerical rainfall estimation data are considered. In statistical models, literal meteorological data and wind powerdata are used.
Wind power factory companies read their hourly
product of coming day and this process is done every day. In this study, wind power cast is carried out. The fuzzy expert system is named as cast system. A wind power factory hourly generated data of 2021 and the meteorological wind data of wind powerfactory locales are used to construct the cast.
Fuzzy model is suggested for the estimation of wind speed and the produced electrical power at a wind demesne.
Expansive simulation results are shown for two operation cases, furnishing wind speed estimations from 30 min to 1hour ahead. Fuzzy sense is an exploration area grounded on the principles of approximate logic and computational intelligence.
Fuzzy models are employed in cases where a system is delicate to model exactly or nebulosity and vagueness is encountered in the problem expression.
-
ENERGY STORAGE SYSTEMS
Energy Storage Systems relate to outfit that can store colourful types of energy safely, efficiently and accessibly. Lithium ion batteries are an illustration of an Energy Storage System.
At present time, lesser focus on renewable energy product is attaining instigation as a result of expansive public and private investment.
The Environmental Government Agencies and other government agencies are presently focuses on introducing ways to control the emigration of carbon dioxide. The pumped hydro storehouse trade is another member that's anticipated to major shares on a global scale and contributes to the growth of this trade. Compressed air storehouse is another energy storehouse technology that's anticipated to contribute to the growth of this trade.
Concentrated solar power is yet another member that is anticipated to boost the growth of the energy storehouse trade. This member allows for the storehouse of energy collected by the suns heat.
The energy collected can be used in a variety of operations, most specially powering electrical turbines. The crucial trends that are driving the energy storehouse systems trade include
The increased relinquishment of energy storehouse systems in the transportation sector Integration of software allowing for the operation of energy storehouse systems Increased Rlinquishment of renewable energy
Energy storehouse systems are applied in a variety of ways. The ensuing operations have added to the growth of this trade, and are anticipated to continue fuelling the growth of this trade.
Energy savings for transportation and grid operations arbitrage, which involves collecting energy when its affordable and extensively available and dealing it when the price rises and it's scarcely available
network operation, or managing capacity balance and the power quality of networks upgrade postponement, which involves the detention or elimination of expensive upgrades to electricity structure.
-
FUZZY LOGIC
The fuzzy sense is introduced by Lotfi Zadeh and is a fine tool uses verbal variables for dealing with query. It consists of three phases which are fuzzification interfence rules and defuzzification. A fuzzy set is created in fuzzification phase by using verbal variables and class functions.
When generally verbal variables are defined as numerous, low, medium, many, class functions are named triangular, trapezoidal, sigmoid, Gaussian and generalized bell shaped. In the alternate phase and conclusion is made accordingto the rules.
Rules correspond of if also conditions and a conclusion. Incipiently, the performing fuzzy production is counterplotted to a crisp production using the class functions in the defuzzification step.
Fuzzy sense is a well- known system with consecutive results. Fuzzy sense has been preferred in numerous studies because it gives successful results in numerous electric power problems.
-
MEMBERSHIP FUNCTION
A membership function for a fuzzy set A on the universe of discourse X is defined as µA: X [0,1] where each element of X is mapped to a value between 0 and 1. This value, called membership value or degree of membership, quantifies the grade of membership of the element in X to the fuzzy set A.
.Membership functions allow us to graphically represent a fuzzy set. The x axis represents the universe of discourse, whereas the y axis represents the degrees of membership in the [0,1] interval.
Simple functions are used to build membership functions. Because we are defining fuzzy concepts, using more complex functions does not add more precision. Below is a list of the membership functions we will use in the practical section of this tutorial.
-
Trapezoidal Function:
It is defined by a lower limit a, an upper limit d, a lower support limit b, and an upper support limit c, where a < b < c < d.
Figure 3: Trapezoidal Function
-
-
BENEFITS OF IVPP
-
Simple functions are used to build membership functions. Because we are defining fuzzy concepts, using more complex functions does not add more precision. Below is a list of the membership functions we will use in the practical section of this tutorial.
-
Using estimations that aren't affected by an up and coming rainfall change are ramped up while those creators that may be affected are ramped down or shut off. However, the control system will ramp up the power production of bio energy shops in order to
-
Lack of interconnectivity among the manufacturers is also one of the attributes, as different manufacturers incorporate different systems in their smart technology systems, thereby making commerce in the public pool delicate. This leads to business models from a prosumer angle to be weak.
-
IVPP MODEL
In this study, it's supposed that the IVPP participates in a centralized electricity trade. IVPP possessors maximize the overall profit of IVPP by integrating all areas in the commercial demesne. It's considered that the cost of wind power and solar is minimum (considered as zero). Therefore, this model only considers the cost of DR. When the power generation in VPP is lesser than the power consumption, the power will be vended to the grid; still, when the power consumption in IVPP is lesser than the power generation, thepower will be bought from the grid.
Figure 4: IVPP Structure
-
INDUSTRIAL LOAD MODELLING
Power consumption characteristics and capacity slipping capacity models are used. Power consumption characteristics represents the reducible quantum of commercial capacity i in time t and fixed power consumption of commercial capacity i in time t.
The objective function is to maximize the overall profit of IVPP.
DR+ Pfix (1)
maintain overall production and balance intermittency problems, if the cast estimation is motioning pall
Pload= Pi,t
i,t
content over a solar ranch.
-
-
Use redundant renewable energy to produce hydrogen and store this gas until it's demanded within thegas network for heat demand.
-
Using trade prices, the control system can produce optimized price schedules which ramp up or down
The objective function is to maximize the overall profit of IVPP.
Cost of interruptible capacity is attained through quantum of resource of commercial capacity and corresponding cost.
.
flexible power consumers( like an commercial pump) which allow for the consumption of electricity when it'scheap and demand is low.
C
i,t
t
DR=iIdr PDR
×c
DR
i,t
(2)
K. DRAWBACKS OF IVPP
-
Although DERs technology is present in numerous nations, yet manufacturers and merchandisers struggle to make it visible. The VPP has still a long way to go, fairly due to the low impact that it has made and frequently incorrect due to myths, rather than the technological systems behind it.
-
FUZZY MODEL OF WIND POWER
In this system forecasting wind power, Forecast values are attained by the Forecast base value, Forecasterror, and Forecast error measure.
wf= Pwf0 + Pwf (3)
Pt t t
Pwf=Pwf0ew (4)
t t t
Utmost wind power production distributions are single peak distribution angles. This composition selects the Gaussian distribution that's the most generally used distribution to express the predictable
t
error of wind power.. In this case ewobeys the normal
Assuming that the electricity trade is fairly mature and accurate, and the number of trade realities is sufficient, the IVPP will share in the electricity trade as a price taker. As shown in illustration, the ideal isto maximize the overall profit of the IVPP. The total
w 2 wf
distribution N (0, (t ) ) and Pt
then obeys the
profit comes from the inflows by dealing fat power in
wf0
wf0
normal distribution N (Pt
,(Pt
w 2.
)
t
the day ahead trade, and the cost considers the
capacity slipping service of controllable loads in the
According to the specific of the normal distribution
and the physical meaning of the class function, the fuzzy number could be used to fit the wind power production. In the dispatch period, the fuzzy parameter of the wind power forecast value can be expressed by a trapezoidal function.
commercial demesne.
Grounded on the commerce with the day-ahead trade, IVPP maintains an energy balancing at each time period during a day. However, the balance constraint of electricity generation and consumption can be expressed as follows,
t
Pwf4
t
Pwf
, Pwf3 Pwf < Pwf4
i,t
iIwind Pwind
k,t
+ kKsolar Psolar
j,t
+ jJbat aj,tPDis +
P
wf4 t
t
Pwf3 t t t
i,t
iIDR PDR
i,t
= iIfix Pfix
+ jJbat(1 aj,t) ×
wf wf2 wf
wf3
Dis+ Pspot (9)
(Pt ) =
1, Pt
wf wf1
< Pt
Pt
(5)
Pj,t t
Pt Pt
, Pwf1 < Pwf Pwf2
wind
solar
t
{Pwf2
t
Pwf1 t t t
Where Pi,t
and Pk,t
are otal quantum of wind
Pt
t t t
0, else
t
andsolar power generations of the IVPP during each
time period t, independently. The capacity is divided
intotwo orders fixed capacity and DR resource, Pi,t
wf=(Pwf1, Pwf2, Pwf3,Pwf4)
wf0
DR
fix represent the quantities of fixed capacity and
= Pt
(r1,t,r2,t,r3,t,r4,t) (6)
and Pi,t
r1,t=(Pt
-3Pt
t )/Pt
(7a)
power generation reduced by DR of commercial
wf0
wf0
wf0 w
wf0 w
wf0
wf0
capacity i at each time t, independently. Pspot
r2,t=(Pt
-Pt
t )/Pt
(7b)
t
indicates the total electricity bought( negative) or
wf0
wf0 w
wf0
r3,t=(Pt
+Pt
t )/Pt
(7c)
vended( positive) by IVPP in the day ahead trade at
wf0
wf0 w
wf0
time period t. indicates the total electricity bought(
r4,t=(Pt
+3Pt
wf
t )/Pt
(7d)
negative) or vended( positive) by IVPP in the day ahead trade at time period t.
Where (Pt
) is a membership function that can
-
Charging and Discharging Regimes
be represented by a four tuple:
P
wf1 t
t
, Pwf2
t
, Pwf3
t
,Pwf4.
To represent the state of the battery, aj,t is introduced
as a variable in the range of 0-1. In this case, the
value of zero indicates that the battery j charges at
-
-
OBJECTIVE FUNCTION
Assuming that the electricity trade is fairly mature and accurate, and the number of trade realities is sufficient, the IVPP will share in the electricity trade as a price taker. As shown in illustration, the ideal isto maximize the overall profit of the IVPP. The total profit comes from the inflows by dealing fat power in the day ahead trade, and the cost considers the capacity slipping service of controllable loads in the commercial demesne.
spot
time t, and the value of one indicate that the battery j
j,t
Pj,t
discharge at time t. When charging, aj,t × PDis will be equal to zero; when discharging, (1 aj,t) × Cha will be zero.
-
Demand response constraints
The DR of controllable guests is paid according to the quantum of their power reduction. Thus,considering the factual situation of each commercial capacity, the DR is constrained as follows,
Max R=24 Pt
× t- CDR (8)
PDR PDR PDR (10)
i=1 t
i,min
i,t
i,max
spot
DR and PDR represents the maximum and
Where Pt
is the amount of electricity sold in
Where Pi,min
i,max
the day ahead trade.
spot
minimum capacities of the DR load i, respectively.
If IVPP buys electricity Pt
spot
is negative. If IVPP
-
Charging and discharging battery constraints
sells electricity Pt
is positive. t is the spot price at
DR
At time t, the energy of battery j can be expressed as
time t during a day, and Ct
response at time t.
is the cost of the load
follows,
Cha –
-
Energy balancing fuzzy constraints of IVPP considering renewable energy uncertainty
j,t
aj,t×PDis j
Ej,t= Ej,t1 + j × (1 aj,t) × Pj,t
j Jbat, t N1. (11)
where j represents the charge and discharge
j,t
effectiveness of battery j PCha
j,t
and PDis
represents the
charging power and discharging power of battery j at
time t respectively. The energy of each energy storehouse system j shall meet the upper and lower limit constraints, considering the parameters of batteries.
Ej_min Ej,t Ej_max (12)
0 P
Cha j,t
Dis
j_max
PCha (13)
Dis (14)
0 Pj,t
Pj_max
where Ej_min and Ej_max represent the upper and lower
limits of the energy level of battery j,
Figure 6: Trapezoidal Memebership Function
(MARCH)
j_max
respectively; PCha
j_max
and PDis represent the upper
limits of the charging and discharging powers of battery j, respectively.
4. CASE STUDIES
It's assumed that in a commercial demesne, there are multiple distributed wind and solar power areas, three reducible commercial loads, and one energy storehouse system. Model optimization is conducted within 24 h using the MATLAB software. Assuming that the electricity trade is mature and the trade structure is reasonable, IVPP earnings gains according to the LMP of the day ahead trade as the price taker. According to the fuzzy model of wind power generation in this study, the prognosticated value of wind power can be expressed by the predicted base value, predicted error and predicted error measure. Two scripts were named in July 2021 (advanced position wind power generation) and March 2021 (medium position wind power generation).
-
Membership Function
Figure 5: Trapezoidal Memebership Function (JULY)
-
Case Study of High Level Generation
(JULY)
Energy savings for transportation and grid operations arbitrage, which involves collecting energy when its affordable and extensively available and dealing itwhen the price rises and it's scarcely available network operation, or managing capacity balance and the power quality of networks upgrade postponement, which involves the detention or elimination of expensive upgrades to electricity structure. In July, the level of wind power generation was high. The battery charge and discharge are demonstrated in the Fig. 9. In this case, the internal power supply of IVPP meets the total load demand. The battery is charged and discharged within one day to meet the changing wind and solar power supply. On a typical day in July, the total power generation of IVPP is as shown in Fig. 7. It can be observed that the reducible load responds several times during the train of the day. At 7:00 and 18:00, owing to the lack of power generation, IVPP purchased a small amount of power from the day-ahead market.
-
Case Study of Medium Level Generation (MARCH)
-
In March, the power supply of IVPP is insufficient due to the decrease of wind power generation in March. As a result, the number of times when IVPP purchases electricity from the power market also increases at 7, 9, 12, and 16. The level of wind power generation was high. The battery charge and discharge are demonstrated in the Fig. 9.
Figure 7: Total Generation in the Day ahead Market in July
Figure 8: Total Generation in the Day ahead Market in March
Figure 9: Daily charging and discharging status of the battery
-
CONCLUSION
-
-
Energy savings for transportation and grid operations arbitrage, which involves collecting energy when its affordable and extensively available and dealing it when the price rises and it's scarcely available network operation, or managing capacity balance and the power quality of networks upgrade postponement, which involves the detention or elimination of expensive upgrades to electricity structure.
-
This study proposed an IVPP optimization model, which integrates the force and demand- side areas of an commercial demesne that participates in the day ahead trade. The proposed model considered renewable generation, different commercial loads, energy storehouse installations, and their constraints.
-
With the increase in battery capacity, the overall trend of power bought from the day ahead trade ison
the rise due to the increase in the quantum of charge and discharge.
-
IVPP uses batteries to charge during the low electricity price period, discharge during the high electricityprice period.
-
On one condition, it can increase the income of IVPP; on the other condition, it promotes carbon redution of the power system. According to its composition, the IVPP forms a type of cooperative business ecosystem with an increased number of relations and interdependencies among IVPP areas.
-
REFERENCES
Gen., Transm., Distrib.,vol. 1, no. 1, pp. 10-16, March 2007
[4] Yaosuo Xue, Bala Venkatesh, Liuchen Chang Bidding wind power in short-term electricity market based on multipleobjective fuzzy optimization. Paper presented at the 2008 Canadian Conference on Electrical and Computer Engineering, Niagara Falls [5] Taner Ustuntas, Ahmet Duran Sahin Wind turbine power curve estimation based on cluster center fuzzy logic modelling Member of Fuzzy Logic and its Technology Club (BUMAT) in Istanbul Technical University Aerodyn. 96 (2008) 611620 [6] Hans Bludszuweit, José Antonio DomÃnguez-Navarro, Member, IEEE, and Andrés Llombart Statistical Analysis of Wind Power Forecast Error IEEE TRANSACTIONS ON POWER SYSTEMS, VOL. 23, NO. 3, AUGUST 2008ElectricalEngineering Department, University of Zaragoza, and also with the CIRCE foundation, associated with it, Zaragoza, Spain 0885- 8950/$25.00 © IEEE
[7] Sherif O. Faried, Senior Member, IEEE, Roy Billinton, Life Fellow, IEEE, and Saleh Aboreshaid, Senior Member, IEEE Probabilistic Evaluation of Transient Stability of a Wind Farm IEEE TRANSACTIONS ON ENERGY CONVERSION, VOL. 24, NO. 3, SEPTEMBER 2009 Paper no. TEC-00268-2008 [8] Wille-Haussmann B, Erge T, Wittwer C. Decentralised optimization of cogeneration in virtual power plants. Solar Energy 2010;84(4):60411. [9] Antonio J.Conejo, Fellow, IEEE, Juan M. Morales, Student Member, IEEE, and Luis Baringo, Student Member, IEEE Real- Time Demand Response Model IEEE TRANSACTIONS ON SMART GRID, VOL. 1, NO. 3, DECEMBER 2010 Junta deComunidades de CastillaLa Mancha under Project PCI-08- 0102 and in part by the Ministry of Science and Technology of Spain through CICYT Project DPI2009-09573. Paper no. TSG- 00124-2010
[10] Salmani MA, Anzalchi A, Salmani S (2010) Virtual power plant: New solution for managing distributed generations in decentralized power systems. Paper presented at the 2010 international conference on management and service science,Wuhan, China, 24-26 Aug. 2010
[11] Kuzle I, Zdrili M, Pandi H (2011) Virtual power plant dispatch optimization using linear programming. Paper presented at the 2011 10th International Conference on Environment and Electrical Engineering, Rome, Italy, 8-11 May 2011 [12] Suresh Munuswamy, Keiko Nakamura, Ajitha Katta Comparing the cost of electricity sourced from a fuel cell-based renewable energy system and the national grid to electrify a rural health centre in India: A case study SRM University, Department of Community Medicine, SRM Nagar, Tamil Nadu 603 203, India Renewable Energy 36 (2011) 2978-2983 [13] E. Mashhour, S. M. Moghaddas-Tafreshi, Bidding Strategy of Virtual Power Plant for Participating in Energy and Spinning Reserve Markets-Part I: Problem Formulation, IEEE Trans.Power Syst., vol. 26, no. 2, pp. 949, May 2011
[14] B.Beigel, P. Andersen, J. Stoustrup, and J. Bendtsen, "Congestion management in a smart grid via shadow prices," 8th Power Plant and Power System Control Symposium, vol. 45, pp. 518-523, 2012. [15] Laura Standardi, Kristian Edlund, Niels Kjølstad Poulsen, John Bagterp Jørgensen A Dantzig-Wolfe Decomposition Algorithm for Linear Economic MPC of a Power Plant Portfolio Published in: The 10th European Workshop on Advanced Control and Diagnosis (ACD 2012) [16] Majid Mokarram, Abdollah Khoei, Khayrollah Hadidi CMOS fuzzy logic controller supporting fractional polynomial membership functions Microelectronics Research Laboratory, Urmia University, Urmia, Iran m3SC+; v 1.191; Prn:22/04/2014; 15:16] P.2 (1-15) [17] Chen W, Ai X, Fan Y (2014) Replicator dynamics algorithm based equilibrium dispatching strategy for distributed energy resources in virtual power plant. Power System Technology 38(03):589-595 [18] Shi L, Sun S, Yao L, Ni Y, Bazargan M (2014) Effects of wind generation intermittency and volatility on power system transient stability. IET Renewable Power Generation 8:509-521 [19] Lorca Ã, Sun X. A. (2015) Adaptive robust optimization with dynamic uncertainty sets for multi-period economic dispatch under significant wind. IEEE Transactions on Power Systems, 30(4):1702-1713 [20] J.S.L. Senanayaka, M.L. Kolhe Integration of Distributed Energy Systems in Micro Grid Architecture for Making a Virtual Power Plant 978-1-4799-1823-2/15/$31.00 ©2015 IEEEEuropean Commission under the FP7 programme with contract number FP7-ICT-2013-11-619560 for project SEMIAH Scalable Energy Management Infrastructure for Aggregation of Households.
[21] A Dolara, F Grimaccia, S Leva, M Mussetta, E Ogliari, A physical hybrid commercial neural network for short term forecasting of pv plant power output, Energies, vol. 8, no. 2, pp. 1138-1153, 2015 [22] Lazaroiu GC, Dumbrava V, Roscia M, Zaninelli D (2015). Energy trading optimization of a virtual power plant on electricity market. Paper presented at the 9th international symposium on advanced topics in electrical engineering, Bucharest, Romania, 7- 9 May 2015 [23] Zamani AG, Zakariazadeh A, Jadid S (2016) Day-ahead resource scheduling of a renewable energy based virtual power plant.Applied Energy 169:324-340
[24] Leo Raju, Kaviya Appaswamy, Janani Vengatraman, Antony Amalraj Morais Advanced Energy Management in Virtual Power Plant using Multi Agent System 2016 3rd International Conference on Electrical Energy Systems 978-1-4673-8262- 5/16/$31.00 c 2016 IEEE [25] Qingli Dong, Yuhuan Sun, Peizhi Li A novel forecasting model based on a hybrid processing strategy and 2 an optimized local linear fuzzy neural network to make wind power 3 forecasting: A case study of wind farms in China National Natural Science Foundation of 739 China (Grant No. 71573034). S0960- 1481(16)30896-5