
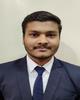
- Open Access
- Authors : Harshal Lonkar , Pranav Khandebharad , Swati Patil , Mayur Limbore, Ashitosh Supekar
- Paper ID : IJERTV10IS040236
- Volume & Issue : Volume 10, Issue 04 (April 2021)
- Published (First Online): 05-05-2021
- ISSN (Online) : 2278-0181
- Publisher Name : IJERT
- License:
This work is licensed under a Creative Commons Attribution 4.0 International License
Human Activity Recogniton using Machine Learning
Swati Patil, Harshal Lonkar, Ashitosh Supekar, Pranav Khandebharad, Mayur Limbore
Jaywantrao Sawant College of Engineering Hadapsar Pune
Abstract Human Activity Recognition is an active field of research and scientific development in which various models have been proposed using different methods for identification and categorization of activities using Machine Learning. The features of image or video data set are extracted using different kinetic models associated with spatial or temporal feature leaning. Also, many deep layer trained models have been successfully used in this field to reach the fundamental goal of this model which is recognition and categorization of activity taking place. These activities can be of different varying nature such as day to day activities like running, jogging, eating, sitting, etc. There can be numerous types of activities in different fields like healthcare, childcare, security or work safety. Human Activity Recognition has a very significant role in different fields like human computer interaction, video surveillance system, robotics, daily monitoring, wildlife observation, etc. With the use of different datasets like UCF-101, HMDB-51, Hollywood2, Sports-1M and training them this task of recognition of activity can be efficiently done. The implementation of Convolutional Neural Network (CNN) model for image recognition with the help of OpenCV helps successful working of this model. Such application of different datasets on activity recognition model has helped in easy categorization of activity based on its nature whether normal or anomalous and suspicious. According to the identified nature an alert is sent through server to the authority concerning the happening of anomalous activity taking place at real time. Due to such application of this model many harmful activities can be avoided or at least negative consequences of such activities can be minimized.
Keywords Human Activity Recognition; Machine Learning; Convolutional Neural Network; OpenCV; video surveillance;
-
INTRODUCTION
With rapid developments in the field of activity recognition and proposition of many new models based on scientific and technological developments immense progress in this field can be seen and observed. The development in deep learning and OpenCV with highly trained datasets have opened a new door of opportunities for upcoming research in this field. Such progress can lead to authentic and useful application of such models in this digitally equipped world for the well-being of all living beings. The use of new and advanced technology in this field by different researchers and developers have resulted in numerous applications of these models.
Due to such highly trained models the activities taking place at real time can be monitored in very effective and optimum manner. Anomalous or suspicious activities can be treated with handy methods ensuring peace and harmony in the society of living beings. This can be also very useful in
creating a smart home environment as well as smart healthcare service with the help of regular monitoring. Many security issues can be handled carefully and the damage to be caused can be minimized. Such effective application of these models in day-to-day life can also ensure the psychological well being of people without concerns of the harm due to such activities.
The human activity recognition model can be implemented with the use of camera module which captures the raw data that serves as an input to the recognition system. By creating different frames of such input data categorization of activity is done after feature extraction. Such activity is then identified as normal or suspicious and immediate alert is sent to the authority.
-
PROBLEM DEFINITION
After surveying the activities taking place in the town, it was found that many types of activities are of unethical nature and are not good for well-being of people. Many other activities were also observed which led an impact with its consequences due to lack of monitoring and proper care. These activities not only cause damage to materialistic things but also leave a psychological impact on human beings. Due to such activities much economic loss is also done.
Due to lack of proper monitoring and care people also have to suffer consequences that can be as disastrous as death of their loved ones especially children and elder people. Due to many such activities the trust on security infrastructure is also compromised.
To overcome all such problems, we have come up with Human Activity Recognition system with the help of machine learning. In this system a camera module has been implemented which helps in capturing the action sequence taking place at real time. This action sequence is further segregated into different frames by segmentation. The feature extraction of input data is done by datasets and the nature of activity is identified. Such activity is classified into normal or suspicious depending on its nature. An immediate alert notification is sent to the authority if the identified activity is of anomalous nature and thus the harmful consequences due to such activity can be minimized. In this study an attempt is made to provide a legitimate solution to such problems occurring in the society. We have concluded this study with possible solutions which is also benefactory for researchers and developers to do further improvisation in this system.
-
LITERATURE SURVEY
-
Action Recognition by Dense Trajectories
(Heng Wang, Liu Cheng-Lin, Alexander Klaser, Cordelia Schmid)
In this paper a very effective and efficient way to extract the dense trajectories is discussed. Using the optical flow fields the densely sampled points can be tracked and then the associated trajectories can be obtained. The scaling of these tracked points is done in much easier manner due to pre computation of denser flow fields. Moreover, the imposition of global smoothness constraints over the points involved in dense optical flow field also results in more robust trajectories unlike matching or tracking of points separately. These dense trajectories are denser and more robust to that of the trajectories of KLT tracker
A very usual problem occurred in this process of tracking is drifting. During the process of tracking the trajectory drifts a bit, usually from its original location. So to avoid the very occurrence of this problem the length of trajectory is limited to L frames. If the trajectory has greater length than L it is excluded from the process of tracking.
These dense trajectories are assesed on standard datasets like Hollywood2, YouTube, KTH and UCF sports. The datasets used are very diverse in nature and can track the activity in different kinds pf scenarios. Also the implementation of KLT tracker is done from OpenCV to compare the dense trajectories with standard KLT tracker.
-
Behaviour Recognition via Sparse Spatio-Temporal Features.
(Piotr Dollar, Garrison Cottrell, Vincent Rabaud, Serge Belongie)
This paper presents the work of doing behavior recognition by characterizing the behavior according to spatiotemporal features. They have presented a new spatio- temporal interest point along with analysis of many cuboid descriptors. Due to the use of these cuboid prototypes, an efficient as well as much robust behavior descriptor is implemented.
Many different types of datasets are compiled together into 3 different datasets namely facial behavior, mouse behavior and human activity. As the differences between behaviors can b very minute or indistinct therefore the optical flow calculation can sometimes be faulty or imperfect. To overcome such defects the datasets are highly trained in recognition of activities having different characteristics and occurrences. The repetition of some activities are also stored as subsets in a dataset.
-
Action Recognition with Improved Trajectories.
(Heng Wang, Cordelia Schmid)
In this paper improvisation of dense trajectories is done by explicitly estimating the camera motion. It is shown that performance can be improvised by removing the background trajectories by estimating approximation in camera motion.
In this model four datasets are used Hollywood2, HDMB51, Olympic Sports and UCF50. These datasets are implemented for effective categorization of activity detection.
In the experimental setup of this model the presentation of implementation details of the features of trajectories is done. Firstly a brief description of dense trajectories is given which are used as baseline in the experiment. The features are encoded using bag of features and Fisher vector.
-
Large-scale Video Classification with Convolutional Neural Networks.
(Andrej Karpathy, Sanketh Shetty, Thomas Leung, Rahul Sukhtankar, George Toderici, Li Fei-Fei)
In this proposed model the study of performance of convolutional neural networks is done in large-scale video classification. As the performance of model is not entirely sensitive to details of architecture, the slow fusion model perform much better than early and late fusion. A mixed resolution architecture is also identified which contains low resolution context and high resolution fovea stream which is very effective in speeding up CNN without any harm to the accuracy.
Videos are very variable in nature due to their temporal extent and therefore requires complex procedure for processing. So in this model each video is treated as a bag of short and fixed sized clips to make the further procedure of categorization and classification more convenient. By doing such a task the spatio-temporal features can be learnt by extending the connectivity of network in time dimension. Here, 3 categories of broad connectivity are used which are Early Fusion, Late Fusion and Slow Fusion. Later a multi resolution architecture is described to address the computational efficiency.
The datasets used in this model are UCF-101 and Sports- 1M.
-
Learning hierarchical invariant spatio-temporal features for action recognition with independent subspace analysis.
(Will Y Zou, Serena Y Yeung, Quoc V Le, Andrew Y Ng)
In this paper they have implemented a method which learns the features from spatiotemporal data with the use of independent subspace analysis. A standard processing pipeline has been used through which the observation has been made that many state-of-the-art methods are outperformed by their simple method. They have used a single method using same parameters across all datasets and have proved to be consistently better than variety of combination of different methods.
Using their method the feature extraction of activity is very fast and efficient as hand designed features. They have also compared the speed of their method with HOG3D algorithm and concluded that using one layer their method is faster than HOG3D but if two layers are used the algorithm is slower. It has also been said that as this method uses matrix vector product and convolutions, its implementation can be done on GPU in an efficient manner.
Various experiments in this model are carried out on datasets such as KTH, Hollywood2, UCF sport action and YouTube in which standard processing pipeline is used.
-
Two-Stream Convolutional Networks for Action Recognition in Videos .
(Andrew Zisserman, Karen Simonyan)
In this system they have proposed a deep video classification model for integration and aggregation of temporal and spatial recognition based on ConvNets. They have also stated that implementation of training on temporal ConvNet during its optical flow is much efficient than training on raw stacked frames. It is also been observed that training on raw stacked frames is much more challenging as it requires many architectural changes. ConvNet assures constancy and smoothness in the flow of feature extraction.
This temporal ConvNet is also useful for training on large video datasets. It has also been observed that temporal ConvNets can outperform spatial ConvNets which also signifies the importance of information of motion for recognition of action taking place.
They have proposed this model in three folds:
-
Firstly, a two-stream ConvNet architecture is used to integrate temporal and spatial networks.
-
Secondly, it is observed that despite having limited training data, ConvNet if trained on multi-frame dense optical flow is able to perform much efficiently.
-
Lastly, it can be seen that performance and amount of training data both can be increased by multi task learning with its application on two different action classification datasets.
In this model the datasets like UCF-101 and HMDB-51 are trained for feature extraction from video frame.
-
-
Beyond Short Snippets: Deep Networks for Video Classification .
(Joe Yeu-Hei Ng, Sudheendra Vijayanarasimhan, Matthew Hausknecht, Rajat Monga, Oriol Vinyals, George Toderici)
In this model they have presented two methods of video classification which can be used for incorporating frame-level CNN outputs into video-level predictions. In this method entire video can be processed in one shot. The training can be done by obtaining large networks by expanding small ones and then by fine-tuning. They have also proposed that to obtain optical flow and take its advantage it is vital to implement more sophisticated sequence processing architecture.
The temporal feature pooling is very effectively used in this model for video classification and its application has been done to representation of bag-of-words. At every time frame images that are based on motion features are computed and quantized and then they are pooled across time.
Several variations that occur are analyzed depending upon the pooling method used and aggregation of features of a particular layer is done. But, both the pooling methods of fully connected layer pooling and average pooling fail due to generation of large number of gradients.
Different datasets like Sports-1M and UCF-101 along with LSTM networks are used for video classification.
-
Long-term Recurrent Convolutional Networks for Visual Recognition and Description .
(Jeff Donahue, Lisa Anne Hendricks, Marcus Rohrbach, Subhashini Venugopalan, Sergio Guadarrama, Kate Saenko, Trevor Darrel)
In this model LRCN has been presented which is a class of models that is deep both spatially as well as temporally and also flexible for its application on variety of vision tasks involving sequential inputs and outputs. These tools are aggregated with much ease into the existing visual recognition pipelines due to which they are considered as instinctive option for perceptual problems as they can easily handle time varying visual input or sequential output with very little input preprocessing as well as no hand-designed features.
The evaluation of this model is done by using the dataset UCF101 which contains various videos which are classified and categorized into different human action classes. Apart from UCF101, other datasets like LSTM, COCO2014 and LRCN have also been used to evaluate this image description model for tasks like retrieval and generation.
-
-
PROPOSED SYSTEM
-
General System
Our system constitutes of the following:
-
Hardware:
The main hardware component used is the Camera Module which has been utilized to capture the real time activities performed. The video captured is basically at minimum of 30-fps which is further stripped further ito different frames that makes 30 frames per second. At a time, a batch of 16 frames is extracted to analyze the activity being performed. Another major component is the processing unit on which the machine learning model runs.
-
Cloud:
The frames extracted from the hardware using Camera module are further processed in the processing unit to check for any anomalous activity. If such an activity is recognized the cloud comes into picture. Cloud is then alerted of the suspicious activity taking place by which it can notify the user or the authority in charge. The authority can be notified in two different ways:
-
Notification through android application
-
SMS by GSM module
The data which is collected on day-to-day basis cannot be fully stored on a physical device so we use cloud as an efficient storage solution. Data collected on cloud can be fetched from any location.
-
-
-
Android Application
The data that is collected on cloud can be seen on the android application. They can be real time data or previously stored data on cloud. Android application can alert the authority or user in different ways like notification alert, vibration or flash lights.
This android application will not only notify the user but also alert the security or police authority in the region in which activity is taking place. Alert can be sent through notification or SMS on the application. Along with alert the location where this suspicious activity is taking place can be provided to the respective authority. The user is also provided with a button which on activation can sound an alarm situated near the camera or surveillance device.
-
-
DIAGRAMS
-
System Architecture
-
-
RESULT ANALYSIS
The camera detects the human in the first place and then the activity that is taking place. The deep learning model used is ResNet which typically uses 2D kernels but to enhance the efficiency of activity recognition 3D kernels are enabled. The activity recognition model is trained on kinetics400 dataset which identifies 400 different types of activities. And to increase the accuracy 400 videos of each activity are present.
The ResNet model for human activity recognition with 2D CNN uses 152 layers for activity identification. This 2D CNN ResNet model performs tasks like detection, segmentation and captioning but our model has additional 3D CNN which processes videos unlike images in 2D CNN which also has 152 layers which increases the chances of success of action recognition. By following tasks like detection, summarization, optical flow, segmentation and captioning.
As a result higher accuracy in human activity recognition is achieved.
-
FUTURE SCOPE
-
Flowchart:
Fig 1. System Architecture
Fig 2. Flowchart
Human Activity Recognition can benefit various applications in fields like smart home monitoring, healthcare services, security surveillance, childcare etc. In future we can update this application by using object activity recognition in which activities performed by objects can also be tracked and analyzed. Application of integrated large datasets can be done to identify the activity taking place as slower rate of time. Even very subtle or minute variations should be recognized by the system. The data of actor performing the anomalous activity can be stored and identification of actor can be done if not caught in the first place. Activities that are of reoccurring manner should be stored to save time and space during recognition process. Implementation of such model can also be done in Government authority section. Much more developments for improvisation in accuracy and dealing with issues related to optical identity and background clutter of image can be done.
-
-
CONCLUSION
We have proposed a Human Activity Recognition system using machine learning which deals with identification of activity based on its nature as normal or suspicious. If such activity of anomalous nature is identified an immediate alert notification is sent to authority due to which further disheartening consequences can be minimized.
-
REFERENCES
-
Kensho Hara, Hirokatsu Kataoka, Yutaka Satoh, (2018 April). Can Spatiotemporal 3D CNNs Retrace the History of 2D CNNs and ImageNet? Retrieved from: https://arxiv.org/abs/1711.09577
-
Du Tran , Jamie Ray , Zheng Shou , Shih-Fu Chang , Manohar Paluri Sabk, (2017 August). ConvNet Architecture Search for Spatiotemporal Feature Learning Retrieved from: https://arxiv.org/abs/1708.05038
-
Moez Baccouche, Franck Mamalet, Christian Wolf, Christophe Garcia, and Atilla Baskurt Asa, (2011). Sequential Deep Learning for Human Action Recognition Retrieved from: https://link.springer.com/content/pdf/10.1007%2F978-3-642-25446- 8_4.pdf
-
Shikhar Sharma, Ryan Kiros & Ruslan Salakhutdinov, (2016 February). Action Recognition using Visual Attention Retrieved from: http://www.shikharsharma.com/publications/pdfs/action- recognition-using-visual-attention-nips2015.pdf
-
Mohamad Hanif Md Saad, Aini Hussain, Liang Xian Loong , Wan Noor Aziezan Baharuddin, Nooritawati Md Tahir, (2011). Event Description From Video Stream For Anomalous Human Activity and Behavior Detection Retrieved from: https://ieeexplore.ieee.org/document/5759931
-
Ishan Agarwal, Alok Kumar Singh Kushwaha, Rajeev Srivastava, (2015). Weighted Fast Dynamic Time Warping Based Multiview Human Activity Recognition Using a RGB-D Sensor Retrieved from: https://ieeexplore.ieee.org/document/7490046
-
Murat Cihan Sorkun, Ahmet Emre Daniman, Özlem Durmaz ncel (2018). Human Activity Recognition With Mobile Phone Sensors: Impact Of Sensors And Window Size Retrieved from: https://ieeexplore.ieee.org/document/8404569
-
Neslihan Käse, Mohammadreza Babaee, Gerhard Rigoll, (2017).
Multi-view human activity recognition using motion frequency
Retrieved from:
https://sigport.org/sites/default/files/docs/ICIP_PaperID1443_Final.p df
-
Hui Huang, Xian Li, Ye Sun, (2016 August). A triboelectric motion sensor in wearable body sensor network for human activity recognition Retrieved from: https://www.researchgate.net/publication/303876425_A_Triboelectri c_Motion_Sensor_in_Wearable_Body_Sensor_Network_for_Human
_Activity_Recognition
-
Shuiwang Ji, Wei Xu, Ming Yang, Kai Yu, (2012). 3D Convolutional Neural Networks for Human Action Recognition Retrieved from: https://ieeexplore.ieee.org/document/6165309
-
Karen Simonyan, Andrew Zisserman, (2014). Two-Stream Convolutional Networks for Action Recognition in Videos Retrieved from: https://arxiv.org/abs/1406.2199
-
Joe Yue-Hei Ng, Matthew Hausknecht, Sudheendra Vijayanarasimhan, Oriol Vinyals, Rajat Monga, George Toderici, (2015 March). Beyond Short Snippets: Deep Networks for Video Classification Retrieved from: https://arxiv.org/abs/1503.08909