
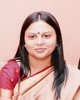
- Open Access
- Authors : Richa Kalpesh Saxena , Dr. Shobha Menon
- Paper ID : IJERTV10IS090119
- Volume & Issue : Volume 10, Issue 09 (September 2021)
- Published (First Online): 21-09-2021
- ISSN (Online) : 2278-0181
- Publisher Name : IJERT
- License:
This work is licensed under a Creative Commons Attribution 4.0 International License
User Perception of Service Quality, Privacy, and Satisfaction for Online Video Streaming Platforms in India
Mrs. Richa Kalpesh Saxena*
Assistant Professor, Marketing and Operations, Narsee Monjee Institute of Management Studies, Mumbai
Dr. Shobha Menon
Principal,
Valia College of Commerce, Mumbai
Abstract – This research paper explored the dimensions of perceived service quality (ePSQ), perceived privacy (ePP), and perceived satisfaction (ePS), and the relationship between these dimensions for online video streaming platforms (OVSPs). The paper started with an extensive literature review in online products identifying the construct dimensions. Next, the data was collected using an online questionnaire from degree college students in Mumbai. Further, the data was analyzed used exploratory and confirmatory factor analysis. Twenty-eight items were identified for Exploratory Factor Analysis. Fifteen valid items were selected for Confirmatory Factor Analysis and Structure Equation Modeling. The study found that the perceived service quality for OVSP is unidimensional, and the significant factors are availability, ease of use, accuracy, navigation, timeliness, speed, and compatibility. Further, perceived privacy is defined by privacy, low risk, and stability. Lastly, perceived satisfaction is defined as value for money, intention to continue using, paying, and recommending others. Both ePSQ and ePP significantly support ePS. The overall model explained 62.5% of the variation.
Keywords: Online Video Streaming Platforms, OTT platforms, OTT service, perceptions, perceived service quality, perceived satisfaction, privacy
-
INTRODUCTION
Worldwide, since the COVID-19 outbreak, there has been a rapid growth in online products [1]. Due to the pandemic, online media has emerged as one of the most thriving businesses when people are forced to stay home [2]. In this scenario, choosing what to watch and where to watch has not been easy [3]. With the increasing demand and competition, online video streaming platforms (OVSPs), such as Netflix, Amazon Prime, and Disney Hotstar, are striving hard to understand the viewers' needs, wants, and preferences [4].
-
NEED FOR THE STUDY
So far, published research in online streaming platforms has focused on exploring the demographic factors to study the usage pattern [5, 6, 7, 8, 9] and the assessment of intention to
use [10, 11, 12, 13]. The research gaps mentioned in the previous studies have highlighted the lack of understanding of the perceived service quality and perceived privacy for OVSPs. This research aims at taking a holistic approach towards customer perceiver service quality (ePSQ) and privacy (ePP). Also, the research aims at understanding their impact on perceived satisfaction (ePS). Therefore, this research aims at the following research questions:
-
What are the indicators of perceived quality of service for OVSPs?
-
How is perceived service quality is related to perceived satisfaction for OVSPs?
-
What are the antecedents and measures of perceived satisfaction for OVSPs?
-
-
LITERATURE REVIEW AND HYPOTHESIS DEVELOPMENT
-
Perceived Service Quality (ePSQ)
The website's visual experience has been a significant factor in e-commerce websites, as visual appeal is the only interaction between the service provider and the customer. The website, therefore, needs to have an up-to-date search engine and a homepage [12]. Further, compatibility with multiple devices has been reported to significantly affecting satisfaction in the context of online videos [13, 11, 10]. Additionally, easy to follow, easy to use, clear and understandable, and easy navigations have supported user-friendliness in online products, such as online videos [10] and internet shopping [14, 15]. It has also positively impacted customer satisfaction in m- commerce [16]. At the same time, speed, information search, and navigation have significantly measured the efficiency dimension in e-commerce service quality [17], and university website quality [18].
Major OVSPs such as Netflix and Amazon Prime are spending heavily on content creation and aggregation [19]. The past literature also verified that updated content accuracy and availability significantly improve the online streaming experience [12, 11]. Besides, appropriate, important, and timely content is reported for many online products [19, 20] and supported system quality [21]. Further, customization has been significant to satisfaction and trust in online videos [12], portal service quality [22], and m-commerce [16, 19]. Additionally, taking account of preferences, speed of downloads and quick problem solving have been significant in assessing service quality for portal service [22] and online products [21]. This research proposes the following hypothesis:
H1: The ePSQ is positively related to ePS for OVSP.
-
Perceived Privacy (ePP)
Despite growing data privacy concerns, the dimensions and impacts of privacy concerns have not yet been studied from an OVSP user's perspective. Online purchase calls for sharing
personal and credit card information. Therefore, the risks involved have been critical to perceived satisfaction [23, 19]. For Instance, accepting various modes of payments has been positively impacting functionality in online travel services [24]. It also has been a significant assurance indicator in e- commerce [25]. Also, the protection of personal information has been a key to assurance/security in m-commerce [19] and e-commerce [25]. Further, company stability, trustworthiness [20], and error-free service were key to customer satisfaction in mobile value-added services [26]. Therefore, this research proposes the following hypothesis:
H2: The ePP is positively related to ePS for OVSPs. H3: The ePP is positively related to ePSQ for OVSPs.
-
Perceived Satisfaction (ePS)
Perceived satisfaction with OVSP also can be drawn on the lines of similar online services. First, recommending others has positively influence satisfaction for travel websites [24], internet shopping [14], and e-tailing [27]. Second, positivity and overall satisfaction have been significant for satisfaction for online videos [10], for adaptation in internet shopping [14], and e-tailing [27]. Third, the intention to continue using has been significant for online services [12, 11]. Fouth, value for money is critical to intention [23]. Finally, preference over other platforms was significant to satisfaction and behavioral intention in mobile value-added services [26].
-
-
RESEARCH METHODOLOGY TABLE 1: QUESTIONNAIRE ITEMS
Variable
Label
Reference
ePSQ
(15 items)
Uptodate, visually
appealing, easy navigation, updated information, easy to use, easy to follow, timely content,
accurate content, content availability, content variety, speed, customization, understanding preferences, solving problems, compatible with other devices
Chen & Kao, 2010; Gupta & Singhania, 2021; Kwon, Park, & Son, 2020; Chi,
2018
ePP (7 items)
Safe transaction, no misuse of information, protection of privacy, low risk,
trustworthiness, mode of payment, error-free transactions
ePS (6 items)
Feel good, recommend to others, continue to use, continue to pay, value for money, overall satisfaction
(Source: The Authors)
-
Questionnaire design
The questionnaire used for the study was designed using the factors discussed in the literature review. Originally there were 33 items in the study. After a small pilot run, five items were
dropped, and a few items were reworded. Twenty-eight items were retained for the study as constructs. Table 1 represents the summary of constructs. Each item measured the respondent's perception of Netflix, Amazon Prime, and Disney Hotstar on a 5-point Likert scale. The items were not grouped under dimensions at this stage.
-
Data Collection
The questionnaire was distributed to degree college students using google forms. A total of 255 forms were received, and
238 complete responses were selected for final analysis. Respondents were asked to rate Netflix, Amazon Prime, and Disney Hotstar separately on the 28 items in each form. In the consolidated analysis, 714 (238X3) responses were taken.
-
Data Screening
The normality of the data was reasonable as the skewness values were between +/- 2.2, though on the negative side and all the kurtosis values were less than 2.2 [28]. The data was further screened for reliability to establish the scale's internal consistency [29]. The common parameter to measure the scale's reliability is Cronbach alpha, which measures the tool's (questionnaire) internal consistency. is calculated using Scale Reliability Analysis on SPSS 25. The value was 0.934, which is excellent for the 28 scale items [28].
Exploratory Factor Analysis (EFA) was conducted using maximum likelihood estimation (ML) as it is recommended for theory development [30]. Thirteen items were dropped due to low extraction value or cross-loadings [27]. Finally, 15 items were taken for further analysis. Confirmatory Factor Analysis (CFA) was conducted for psychometric evaluation of the test constructs for latent constructs for ePSQ, ePP, and ePS [31].
-
Measurement Model of ePS
The Maximum Likelihood extraction method was used on 15 items with varimax rotation. The Kaiser-Meyer-Olkin (KMO) measure was 0.945 showing the adequacy of data [32]. The significant value of Bartlett's test of sphericity 2 (105) = 7230.818, p < 0.001, shows that the data is good for EFA. This has also established structural validity.
An initial analysis was run to obtain eigenvalues, and the three components had eigenvalues over one and explained 70.449% of the variance. Table 2 shows the factor loadings after rotation. The items in each factor suggest that the first component is ePSQ, the second component represents ePS, and the third component is ePP.
The value for each factor shows the predictability of the scale. As shown in Table 2, all factor loadings ranged from
0.596 to 0.829 and were statistically significant. The t -values (shown as CR value in AMOS output) are greater than 1.96. The AVE values are above 0.5, which indicates that each construct was strongly related to its respective indicators. Composite reliability values were also above the recommended value of 0.7. Overall, the measurement model exhibited adequate convergent validity [33]. Nomological validity is also established as all the correlations are positive and significant, as shown in Table 3. Also, all the MSV and ASV values are less than the AVE value. This establishes discriminant validity.
TABLE 2: FACTOR ANALYSIS
Items
Factors
t-value
Composite Reliability
AVE
ePSQ
ePS
ePP
AVAILABILITY
0.737
–
0.839
0.636
EASE OF USE
0.726
23.903
ACCURACY
0.716
21.807
INFORMATION
0.697
21.110
NAVIGATION
0.677
21.390
TIMELINESS
0.652
20.017
SPEED
0.616
21.274
COMPATIBILITY
0.604
20.203
PAY
0.829
22.793
0.922
0.596
CONTINUE
0.765
23.229
VALUE
0.611
–
RECOMMEND
0.596
19.939
LOW RISK
0.826
21.326
0.897
0.688
PRIVACY
0.679
18.994
STABLE
0.613
–
0.921
0.893
0.837
(Source: SPSS Output)
TABLE 3: COVARIANCES AND CORRELATIONS
Covariance
SE.
CR.
P
Correlation
MSV
ASV
ePSQ- ePP
.431
.035
12.378
***
.702
0.493
0.472
ePSQ-ePS
.463
.036
12.852
***
.768
0.590
0.541
ePP-ePS
.418
.035
11.797
***
.672
0.590
0.521
(Source: The Authors)
FIGURE 1. MEASUREMENT MODEL PATH DIAGRAM (Source: AMOS Output)
The CFA model given in Figure 1 shows the measurement model with exogenous constructs of ePSQ, ePP, and ePS. The 2/df =3.377 and the other indices (GFI = 0.951, CFI= 0.973, AGFI = 0.928, NFI = 0.962, TLI=0.965 and RMSEA =0.058)
satisfied the recommended values. Therefore, the fit index result suggests model fitness with the data [33].
The structural relationship is shown in Figure 2. For the SEM, the 2/df =4.265, GFI = 0.9351, CFI= 0.961, AGFI = 0.910,
NFI = 0.950, TLI=0.952 and RMSEA =0.068 satisfied the recommended values. Therefore, the fit index results suggest model fitness with the data [33]. The parsimony fit indices PNFI=0.773 and PCFI = 0.778, also satisfies the parsimony fit criteria [34, p. 284].
-
-
FINDINGS AND CONCLUSIONS
From the Figure 2, ePQS and ePP explained 62.5% variation in endogenous construct ePS. As shown in Table 4, the most important factor for ePS is ePSQ, explaining 59% variation, at p<0.001, thus supporting H1. H2 is also supported at p<0.001 at a 26% variation. The covariance between two exogenous constructs is significant at p<0.001, therefore supporting H3.
The rest of the findings are discussed in the next section.
FIGURE 2. PATH DIAGRAM FOR SEM
(Source: AMOS Output)
Table 4: Structural Parameters and Hypothesis Testing
Estimate
S.E.
t-value
p
Results
ePS
<—
ePSQ
0.590
0.051
11.596
***
H1 supported
ePS
<—
ePP
0.258
0.046
5.649
***
H2 supported
ePSQ
<-->
ePP/p>
0.431
0.035
12.378
***
H3 supported
-
DISCUSSIONS
The most important outcome of this paper is the constructs of ePSQ. Customer satisfaction was expected to be a consequence of SERVQUAL dimensions, but many SERVQUAL factors were eliminated before CFA. There was no representation of tangibles and responsiveness dimensions. Functionality factors of ease of use, speed, and navigation have some impact on service quality. The most important impact came from reliability and accessibility with five variables; information quality, timeliness, availability, accuracy, and compatibility measuring service quality. As expected, safety and security came out as separate factors. The regression model suggests that the quality of service and the safe interactions lead to perceived satisfaction. The most important parameter to assess the quality of service was the accuracy of information regarding movies and shows. For OVSPs, this information acts as a stimulus for deciding which show/movie to watch. OVSP providers, therefore, should emphasize this piece of information. The second important factor was the availability of movies and shows. Due to COVID-19, many new movies were released on OVSPs during the lockdown, thus increasing content availability. The third important factor was ease to use. Since the sampling unit was college-going students, this was expected. The least
(Source: AMOS Output) important factor found out to be the compatibility with multiple devices. This may be because most college-going students use their phones for OVSPs. The construct of privacy
and security had a moderate but significant effect on satisfaction.
A. Limitations and Future Research Directions
The topic of OVSP is not much explored, and therefore the research conducted on various other online services was explored. It would be interesting to find the impact of some new factors, like, no censorship on programs and personalization offered by some platforms. It was expected that SERVQUAL variables would form exogenous constructs, but only a single construct was formed, making this model parsimonious. Future researches may investigate more of these factors. The other concern is the robustness of the model for subscribers and non-subscribers of OVSP and different platforms individually.
Further, the data was collected from college-going students only for Netflix, Amazon Prime, and Disney Hotstar. Other platforms like YouTube, Zee5, and free platforms were not included in the study. Lastly, the OVSP industry is evolving, and new concepts may emerge and be investigated in the future.
-
REFERENCES
-
R. K. Saxena and S. Menon, "Content Wars for Excellence in Online Streaming Platforms in India: A Comparative Analysis of Netflix and Amazon Prime Video," Journal of Education, vol. 23, no. 8, pp. 28-35, 2021.
[1] IANS @ETBrandEquity, "Covid-19: Netflix adds 10 million new paid subscribers as people stay home, Marketing & Advertising News, ET BrandEquity," 2020. [Online]. [Accessed 2020].
[2] WebDesk@IndiaToday, "5 sectors that are booming during the Covid- 19 pandemic – Education Today News," 2020. [Online].
[3] ETBrandEquity, "How 2020 changed the young Indian consumer," 6 Jan 2021. [Online]. Available: https://brandequity.economictimes.indiatimes.com/news/marketing/be- exclusive-how-2020-changed-the-young-indian-consumer/80119029.
[4] R. K. Saxena and S. Menon, "Perceptual Mapping of Online Video Streaming Platforms in India," Asian Journal of Research in Marketing, vol. 10, no. 4, pp. 15-23, 2021.
[5] S. Karim, "A Comparison of the Media Consumption Habits of Gen X, Gen Y and Gen Z," Allana Management Journal of Research, pp. 1-5, 2019.
[6] A. Soni and H. Puthawala, "Factors Affecting Millennials' Preference for Online Video Streaming Apps in Ahmedabad," The IUP Journal of Marketing Management, pp. 23-54, 2020.
[7] S. Poulose, "Study on Customer Satisfaction Towards Cable TV Network and Online Video Streaming Services in Chennai," Journal Of Archaeology Of Egypt/Egyptology, pp. 145-156, 2019.
[8] U. Cebeci, O. Ince and H. Turkcan, "Understanding the Intention to Use Netflix: An Extended Technology Acceptance Model Approach," International Review of Management and Marketing, pp. 152-157, 2019.
[9] D. Madnani, S. Fernandes and N. Madnani, "Analysing the impact of COVID-19 on over-the-top media platforms in India," International Journal of Pervasive Computing and Communication, pp. 457-475, 2020.
[10] G. Gupta and K. Singhania, "Consumption of OTT Media Streaming in COVID-19 Lockdown: Insights from PLS Analysis," Vision: The Journal of Business Perspective, 2021.
[11] Y. Chung and W. Zhang, "Effects of Service Characteristics of a Subscription-based OTT on User Satisfaction and Continuance Intention: Evaluation by Netflix Users," Journal of the Korea Contents Association '20, pp. 123-135, 2020.
[12] Y. Kwon, J. Park and J.-Y. Son, "Accurately or accidentally? Recommendation agent and search experience in over-the-top (OTT) services," Internet Research, 2020.
[13] M.-K. Lee, W.-J. Kim and M.-H. Song, "A Study on the Factors Influencing Continuous Intention to Use of OTT Service Users," Journal of Digital Convergence, pp. 537-546, 2019.
[14] S. Gounaris, S. Dimitriadis and V. Stathakopoulos, "An examination of the effects of service quality and satisfaction on customers' behavioral intentions in eshopping," Journal of Services Marketing, vol. 24, no. 2, pp. 142-156, 2010.
[15] M. Kostrzewska and D. Wrukowska, "Measuring customer satisfaction in the e-service market," Advances in Business-Related Scientific Research Journal, vol. 10, no. 2, pp. 58-69, 2019.
[16] Y. S. Yeh and Y.-M. Li, "Building trust in mcommerce: contributions from quality and satisfaction," Online Information Review, vol. 33, no. 6, pp. 1066-1086, 2009.
[17] A. Parasuraman, V. A. Zeithaml and A. Malhotra, "E-S-QUAL A Multiple Item Scale for Assessing Electronic Service Quality," Journal of Service Research, vol. 7, no. X, pp. 135-139, 2005.
[18] B. Nemati, H. Gazor, S. N. MirAshrafi and K. N. Ameleh, "Analyzing e-service quality in service-based website by E-SERVQUAL," Management Science Letters, vol. 2, no. 2, pp. 727-734, 2012.
[1] IANS @ETBrandEquity, "Covid-19: Netflix adds 10 million new paid subscribers as people stay home, Marketing & Advertising News, ET BrandEquity," 2020. [Online]. [Accessed 2020].
[2] WebDesk@IndiaToday, "5 sectors that are booming during the Covid- 19 pandemic – Education Today News," 2020. [Online].
[3] ETBrandEquity, "How 2020 changed the young Indian consumer," 6 Jan 2021. [Online]. Available: https://brandequity.economictimes.indiatimes.com/news/marketing/be- exclusive-how-2020-changed-the-young-indian-consumer/80119029.
[4] R. K. Saxena and S. Menon, "Perceptual Mapping of Online Video Streaming Platforms in India," Asian Journal of Research in arketing, vol. 10, no. 4, pp. 15-23, 2021.
[5] S. Karim, "A Comparison of the Media Consumption Habits of Gen X, Gen Y and Gen Z," Allana Management Journal of Research, pp. 1-5, 2019.
[6] A. Soni and H. Puthawala, "Factors Affecting Millennials' Preference for Online Video Streaming Apps in Ahmedabad," The IUP Journal of Marketing Management, pp. 23-54, 2020.
[7] S. Poulose, "Study on Customer Satisfaction Towards Cable TV Network and Online Video Streaming Services in Chennai," Journal Of Archaeology Of Egypt/Egyptology, pp. 145-156, 2019.
[8] U. Cebeci, O. Ince and H. Turkcan, "Understanding the Intention to Use Netflix: An Extended Technology Acceptance Model Approach," International Review of Management and Marketing, pp. 152-157, 2019.
[9] D. Madnani, S. Fernandes and N. Madnani, "Analysing the impact of COVID-19 on over-the-top media platforms in India," International Journal of Pervasive Computing and Communication, pp. 457-475, 2020.
[10] G. Gupta and K. Singhania, "Consumption of OTT Media Streaming in COVID-19 Lockdown: Insights from PLS Analysis," Vision: The Journal of Business Perspective, 2021.
[11] Y. Chung and W. Zhang, "Effects of Service Characteristics of a Subscription-based OTT on User Satisfaction and Continuance Intention: Evaluation by Netflix Users," Journal of the Korea Contents Association '20, pp. 123-135, 2020.
[12] Y. Kwon, J. Park and J.-Y. Son, "Accurately or accidentally? Recommendation agent and search experience in over-the-top (OTT) services," Internet Research, 2020.
[13] M.-K. Lee, W.-J. Kim and M.-H. Song, "A Study on the Factors Influencing Continuous Intention to Use of OTT Service Users," Journal of Digital Convergence, pp. 537-546, 2019.
[14] S. Gounaris, S. Dimitriadis and V. Stathakopoulos, "An examination of the effects of service quality and satisfaction on customers' behavioral intentions in eshopping," Journal of Services Marketing, vol. 24, no. 2, pp. 142-156, 2010.
[15] M. Kostrzewska and D. Wrukowska, "Measuring customer satisfaction in the e-service market," Advances in Business-Related Scientific Research Journal, vol. 10, no. 2, pp. 58-69, 2019.
[16] Y. S. Yeh and Y.-M. Li, "Building trust in mcommerce: contributions from quality and satisfaction," Online Information Review, vol. 33, no. 6, pp. 1066-1086, 2009.
[17] A. Parasuraman, V. A. Zeithaml and A. Malhotra, "E-S-QUAL A Multiple Item Scale for Assessing Electronic Service Quality," Journal of Service Research, vol. 7, no. X, pp. 135-139, 2005.
[18] B. Nemati, H. Gazor, S. N. MirAshrafi and K. N. Ameleh, "Analyzing e-service quality in service-based website by E-SERVQUAL," Management Science Letters, vol. 2, no. 2, pp. 727-734, 2012.
-
T. Chi, "Mobile Commerce Website Success: Antecedents of Consumer Satisfaction and Purchase Intention," Journal of Internet Commerce, pp. 189-215, 2018.
-
E. Cristobal, C. Flavian and M. Guinaliu, "Perceived eservice quality (PeSQ) Measurement validation and effects on consumer satisfaction and web site loyalty," Managing Service Quality, vol. 17, no. 3, pp. 317- 340, 2007.
-
J. Xu, I. Benbaset and R. T. Cenfetelli, "Integrating Service Quality with System and Information Quality: An Empirical Test in the E-Service Context," MIS Quarterly, vol. 37, no. 3, pp. 777-794, 2013.
-
T. Kuo, I.-Y. Lu, C.-H. Huang and G.-C. Wu, "Measuring users' perceived portal service quality: An empirical study," Total Quality Management and Business Excellence, vol. 16, no. 3, pp. 309-320, 2005.
-
J.-H. Wu and S.-C. Wang, "What drives mobile commerce?,"
Information & Management, pp. 719-729, 2005.
-
C.-F. Chen and Y.-L. Kao, "Relationships between process quality, outcome quality, satisfaction, and behavioural intentions for online travel agencies – evidence from Taiwan," Service Industries Journal, vol. 30, no. 12, pp. 2081-2092, 2010.
-
N. Kassim and N. A. Abdullah, "The effect of perceived service quality dimensions on customer satisfaction, trust, and loyalty in e-commerce settings: A cross- cultural analysis," Asia Pacific Journal of Marketing and Logistics, vol. 22, no. 3, pp. 351-371, 2010.
-
Y.-F. Kuo, C.-M. Wu and W.-J. Deng, "The relationships among service quality, perceived value, customer satisfaction, and post-purchase intention in mobile value-added services," Computers in Human Behaviour, vol. 25, pp. 887-896, 2009.
-
D. X. Ding, P. J.-H. Hu and O. R. L. Sheng, "E-SELFQUAL: A scale for measuring online self-service quality," Journal of Business Research, vol. 64, no. 5, pp. 508-515, 2011.
-
D. George and P. Mallery, "Reliability Analysis," in IBM SPSS Statistics 25 Step by Step fifteenth edition, New York and London, Routledge Taylor and Francis Group, 2019, pp. 235-245.
-
N. Malhotra and S. Dash, "Measurement and Scaling: Non-comparative scaling Techniques," in Marketing Research: An Applied Orientation, Noida, Pearson India, 2019, pp. 292-295.
-
J. C. Anderson and D. W. Gerbing, "Structural Equation Modeling in Practice: A Review," Psychological Bulletin, vol. 103, no. 3, pp. 411- 423, 1988.
-
T. A. Brown, Confirmatory Factor Analysis for Applied Research, New York: The Guilford Press, 2006.
-
A. Field, Discovering Statistics Using SPSS, Third ed., London: Sage, 2009.
-
J. F. Hair, B. J. Babin, W. C. Black and R. E. Anderson, Multivariate Data Analysis, 8 ed., Delhi: Cengage, 2019.
-
S. A. Sivo, X. Fan, E. L. Witta and J. T. Willse, "The Search for "Optimal" Cutoff Properties: Fit Index Criteria in Structural Equation," The Journal of Experimental Education, vol. 74, no. 3, pp. 267-288, 2006.
-
H. H. Alshibly, "Customer perceived value in social commerce: An exploration of its antecedents and consequences," Journal of Management Research, vol. 7, no. 1, pp. 19-37, 2015.