
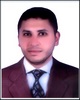
- Open Access
- Authors : Essam Sharaf El Din , Hossam El-Din Fawzy , Samah Abo Ramadan
- Paper ID : IJERTV9IS030013
- Volume & Issue : Volume 09, Issue 03 (March 2020)
- Published (First Online): 09-03-2020
- ISSN (Online) : 2278-0181
- Publisher Name : IJERT
- License:
This work is licensed under a Creative Commons Attribution 4.0 International License
Use of Multivariate Statistical Analysis for Detecting Spatial and Seasonal Attributes of Surface Water Quality
Essam Sharaf El Din
Tanta University, Faculty of Engineering, Public Works Engineering Department, Gharbeya, Egypt
Hossam El-din Fawzy, Samah Abo Ramadan
Kafr El-Sheikh University, Faculty of Engineering, Civil Engineering Department, Kafr El-Sheikh, Egypt
Use of multivariate statistical analysis for detecting spatial and seasonal attributes of surface water quality
The existence of both point and non-point inputs of pollutants raises the cost of water body treatment due to their negative effect on watersheds. Additionally, categorizing the most significant surface water quality parameters (SWQPs) in both spatial and temporal domains is crucial. Thus, to classify the dominant SWQPs and accordingly identify both spatial and temporal aspects of surface water quality, multivariate statistical analysis (MSA), such as principal component analysis/factor analysis, cluster analysis, and discriminant analysis, was used. The obtained results demonstrated that turbidity, total suspended/dissolved solids, chemical oxygen demand, and biochemical oxygen demand are the dominant SWQPs, which contribute to spatial and temporal surface water quality status of the Saint John River, Canada. Moreover, a decrease in the dimensionality of surface water quality data was achieved. To conclude, the use of MSA can lead to effective savings and applicable exploitation of water resources.
Keywords: multivariate statistical analysis (MSA); spatial and seasonal surface water quality; point and non-point inputs of pollutants.
-
INTRODUCTION
Point sources of pollutants establish a fixed polluting source; while, non-point sources represent seasonal circumstances (Singh et al. 2004). These sources of pollution can negatively affect surface water quality of watersheds by raising concentrations of surface water quality parameters (SWQPs) (Carpenter et al. 1998; Qadir et al. 2007). The proper treatment process must be directed to the most significant SWQPs, which contribute to spatial and seasonal changes of surface water quality (Elhatip et al. 2007). By doing this, valuable savings and correct utilization of water resources can be simply achieved (Natural resources 2016).
Thus, multivariate statistical analysis (MSA), such as principal component analysis/factor analysis (PCA/FA), cluster analysis (CA), and discriminant analysis (DA), are used to better understand the updated status of water quality of a specified watershed (Vega et al. 1998; Wunderlin et al. 2001; Reghunath et al. 2002; Simeonov et al. 2003; Shrestha & Kazama 2007; Sharaf El Din & Zhang 2018). In literature, MSA was utilized to calculate correlation among different SWQPs, classify the major SWQPs, monitor seasonal changes of surface water quality, and compress surface water quality data into a few sets of classes (Haag & Westrich 2002; Ouyang et al. 2006; Li et al. 2009; Dong et al. 2010; Huang et al. 2010; Mishra 2010; Salah et al. 2011; Mahapatra & Mitra 2012; Sharaf El Din 2019a; Sharaf El Din et al. 2019b).
The use of MSA is essential due to its potential of clarifying the relationship between various SWQPs, such as total dissolved solids (TDS), total solids (TS), total suspended solids (TSS), turbidity, biochemical oxygen demand (BOD), dissolved oxygen(DO), chemical oxygen demand (COD), electrical conductivity (EC), temperature, and power of hydrogen (pH). Furthermore, it is very difficult to extract obvious conclusions from raw data of surface water quality. Hence, MSA could be employed to detect surface water quality changes and categorize the major SWQPs of water bodies (Reghunath et al. 2002).
Locating the association between water sampling stations, decreasing the complexity of large-scale datasets into clusters with similar characteristics, and recognizing the dominant SWQPs are the main advantages of MSA. Conversely, the occurrence of the same SWQPs in different principal components (PCs) and difficulty in realizing the appropriate number of classified groups are the main drawbacks of MSA (Singh et al. 2004).
The key objectives of our research study are to:
-
categorize the dominant SWQPs of the Saint John River (SJR) using PCA/FA,
-
create multiple levels of clusters using CA, and
-
assess spatio-temporal surface water quality variations of the SJR using DA.
-
-
MATERIALS AND METHODS
-
Study site
The SJR is one of the oldest streams in Canada. It covers an area of 4748 km2. Oromocto, Nashwaak, Keswick, Miramichi, Tobique, Aroostook, and Madawaska feed the SJR. The average width of the SJR is 750 m and the average depth is 3 m (Arseneault 2008). The study area comprises a 130 km long, which covers both the lower and middle basins of the SJR (Fig. 1).
Fig. 1. The study site of the SJR (Google Maps 2016).
-
Analysis of surface water quality parameters (SWQPs)
Sampling stations were gathered from the SJR in June 2015, April 2016, May 2016, July 2016, and August 2016 (Fig. 2). In this research work, 66 water samples were selected over the study site of the SJR. Positions of sampling stations were recorded using GARMIN 76CSx GPS. Measurements of water samples were achieved according to the American Public Health Association (APHA) standards (APHA 2005).
Fig. 2. Water sampling stations.
Optical SWQPs, such as turbidity, TSS, TS, and TDS were analyzed. Additionally, non-optical SWQPs, such as COD, BOD, DO, pH, EC, and temperature were analyzed.
-
Multivariate statistical analysis (MSA)
SWQPs were exposed to MSA to figure out the most important parameters that were responsible for both spatial and seasonal changes in the SJR. The working strategy of MSA, such as PCA/FA, CA, and DA, is provided below.
-
Principal component analysis/factor analysis (PCA/FA)
PCA has been utilized to linearly transform the raw dataset into a new uncorrelated dataset, called principal components (PCs). PCA offers information about the major variables within the utilized dataset (Shrestha & Kazama 2007). PCs can be calculated according to the following equation:
= 1 × 1 + 2 × 2 + + × (1)
where is the score of each PC; is the loading of each PC; is the measured value of each parameter;
is the number of each PC; is the number of the sample; is the total number of parameters.
Following PCA, FA was employed to retain variables with major significance and minimize the influence of variables with negligible significance (Vega et al. 1998; Simeonov et al. 2003). FA can be stated as follows:
= 1 × 1 + 2 × 2 + + × + (2)
where is the measured parameter; is the loading value of each parameter; is the score of each factor;
is the term of errors; is the number of the sample; is the total number of factors.
-
Cluster analysis (CA)
CA classifies entities into discrete clusters (McKenna 2003). Hierarchical agglomerative CA was employed on the utilized surface water quality dataset. A dendrogram is the main visualized result of hierarchical agglomerative CA, which can provide a summary of the obtained clusters with a remarkable decline in dimensionality of the raw dataset (Shrestha & Kazama 2007).
-
Discriminant anaysis (DA)
-
DA establishes relationships between pre-defined clusters according to discriminating variables (Singh et al. 2004). The number of the achieved discriminant functions is either the number of clusters 1, or the number of the parameters, whichever is smaller.
-
-
RESULTS AND DISCUSSION
3.1 Analysis of SWQPs
As shown in Table 1, ten SWQPs, such as Turbidity, TSS, TS, TDS, COD, BOD, DO, pH, EC, and Temperature, were obtained from 66 sampling points according to the APHA standard methods.
Table 1 Statistics of the selected SWQPs
Selected SWQPs
Mean of 66 water samples
Standard deviation
Turbidity (NTU)
4.84
3.73
TSS (mg/l)
3.59
3.10
TS (mg/l)
113.92
42.32
TDS (mg/l)
110.33
39.91
COD (mg/l)
27.55
19.85
BOD (mg/l)
1.75
0.52
DO (mg/l)
9.54
2.64
pH
7.59
0.33
EC (us/cm)
97.09
30.53
Temperature (°C)
15.92
6.97
The range of turbidity concentrations was 1.19 to 13.10 NTU with an average of 4.84 NTU. TSS levels ranged from
0.60 to 11.40 mg/l with a mean value of 3.59 mg/l. TS ranged from 58.00 to 245.00 mg/l, and TDS varied from 52.40 to 233.85 mg/l. While COD ranged from 4.80 to 86.64 mg/l with an average 27.55 mg/l, BOD levels were 1.21 to 3.25 mg/l with an average 1.75 mg/l. Finally, levels of DO, pH, EC, and Temp were 6.71 to 14.14 mg/l, 6.51 to 8.42, 29.50 to 148.90 us/cm, and
5.00 to 23.30 °C, respectively.
In spring, Turbidity and TSS levels were higher than their levels in summer season due to soil erosion from the presence of rainfall and snow melt. Soil erosion could push sediments from forestry into the SJR basins (Sharaf El Din et al. 2017a; Sharaf El Din & Zhang 2017b; Sharaf El Din & Zhang 2017c; Sharaf El Din & Zhang 2017d; Sharaf El Din & Zhang 2017e).
-
Multivariate statistical analysis (MSA)
-
PCA/FA
PCA/FA was employed to categorize the most significant SWQPs in the SJR. It was applied on 66 sampling points using ten SWQPs (i.e., turbidity, TSS, TS, TDS, COD, BOD, DO, EC, pH, and temperature) to classify the dominant parameters contributing to water quality in the selected study site of the SJR.
A set of PCs was generated along with their corresponding eigenvalues, which measure the significance of the extracted PCs, by using PCA. Eigenvalues of 1 are considered significant (Shrestha & Kazama 2007). PC1, PC2, and PC3 have eigenvalues > 1; hence, they are considered as the dominant PCs. As shown in Table 2, PC1, PC2, and PC3 captured 88.126% of the total variation in the data of the SJR. These three PCs explained 49%, 20%, and 19% of the total variance, respectively.
FA was employed by exposing the obtained PCs to varimax rotation in order to improve the interpretation of PCA. By doing this, absolute values of large loadings can be maximized, and absolute values of smaller loadings can be minimized within each PC. The loading values can be subdivided into three principal classes: strong (loading values 0.75), moderate (0.75 > loading values 0.50), and weak (0.50 > loading values 0.40) (Liu et al. 2003).
Table 2 The obtained PCs along with their corresponding eigenvalues
PCs
eigenvalue
Variance %
Cumulative variance %
1
4.907
49.071
49.071
2
2.000
20.005
69.076
3
1.905
19.050
88.126
4
0.741
7.407
95.533
5
0.211
2.116
97.649
6
0.117
1.170
98.819
7
0.067
0.673
99.492
8
0.038
0.375
99.867
9
0.013
0.132
99.999
10
0.001
0.001
100.000
Each SWQP with a loading value of 0.75 or higher was considered as a significant parameter, which may contribute to water quality changes in the SJR. On the other hand, SWQPs with loading values less than 0.40 were considered as insignificant. In Fig. 3, turbidity, TSS, TS, and TDS were loaded as strong with positive values.
In PC1, Turbidity TSS, TS, and TDS are the dominant SWQPs, which contribute to both spatial and seasonal surface water quality changes in the river. Soil erosion is the main cause of increasing concentrations of turbidity and TSS because of the existence of natural and human processes, such as snow melt, rainfall, forestry, and agricultural activities.
PC2 verified that the loading value of EC was considered as strong with positive values; while, pH was loaded as moderate. Hence, EC is the major SWQP responsible for spatio-temporal surface water quality changes in the SJR due to the existence of irrigation purposes.
Fig. 3. The loading value of each SWQP at each component.
PC3 clarified that COD and BOD have strong positive loading values, due to industrial sewage, which may be resulted from food processing and paper production industries along the shoreline of the river.
The above outcomes confirmed that PCA/FA is a cost-effective method in surface water quality research studies owing to its potential of classifying the most significant pollutants in the SJR.
-
CA
Hierarchical agglomerative CA was utilized to identify clusters, which have the same properties of surface water quality. As
shown in Fig. 4, CA created multiple levels of clusters, and a dendrogram that classifyed the collected water sampling points (i.e., 66 samples) into four discrete clusters was produced.
Fig. 4. The generated dendrogram of water sampling points.
As shown in Fig.4, cluster 1 contains 28 water samples, and these samples have higher COD and BOD values because of the existence of food and paper production industries. Cluster 2 includes 15 water samples, and these samples represent the lower basin of the river, which has less industrial and agricultural sewage. Cluster 3 contains 10 water sampling points, and these samples were collected in spring, which means that these 10 samples receive pollution mostly from natural resources, such as rain fall and snow melt in the study site of the SJR. Similar to cluster 3, cluster 4 includes 13 water samples, and these samples were collected in spring. Natural resources were responsible for raising soil erosion, and consequently raise turbidity and TSS concentrations in the SJR.
These findings showed that hierarchical agglomerative CA is essential due to its potential to categorize water samples into separate clusters based on surface water quality characteristics.
-
DA
DA was utilized to further assess spatial changes in surface water quality using clusters, which were produced by hierarchical agglomerative CA. The four clusters represent the dependent variables, while the 10 SWQPs were utilized as the independent variables. As shown in Fig. 5, DA was employed, and three discriminant functions were deeloped. The obtained clusters were obviously distinguished using function 1 and function 2.
Fig. 5. Scatter plot of spatial DA using the four clusters.
Fig. 6. Scatter plot of temporal DA using data from different seasons.
DA was also employed to evaluate seasonal changes in surface water quality. Water samples dataset was subdivided into groups (i.e. early spring (April 2016), late spring (May 2016), early summer (June 2015 and July 2016), and late summer
(August 2016)). The four temporal clusters were used as dependent variables; whereas, the 10 SWQPs were utilized as independent variables. As shown in Fig. 6, the four seasonal clusters were categorized using the first two discriminant functions.
-
-
-
CONCLUSION
To better exploit water resources, it is essential to direct water treatment of waterbodies towards the most significant SWQPs. Hence, MSA was used to classify the key SWQPs in the SJR, diminish the complexity of water quality data, and assess spatio- temporal changes in surface water quality of the study site of the SJR. The key findings of this study are:
-
Turbidity, TSS, COD, BOD, pH, and EC are the main SWQPs in the river.
-
Hierarchicalagglomerative CA gathered 66 water sampling stations into four clusters, which means an obvious decrease in the water quality dataset was accomplished.
-
DA identifies four seasonal groups (early spring, late spring, early summer, and late summer).
ACKNOWLEDGEMENTS
This study is supported by the Egyptian Ministry of Higher Education.
REFERENCES
-
APHA. (2005) Standard Methods for the Examination of Water and Wastewater (21th ed.). American Public Health Association, Washington DC, USA.
-
Arseneault, D. (2008) The Road to Canada – Nomination Document for the St. John River, New Brunswick. The St. John River with the support of the New Brunswick Department of Natural Resources.
-
Carpenter, S. R., Caraco, N. F., Correll, D. L., Howarth, R. W., Sharpley, A. N. and Smith, V. H. (1998) Non-point pollution of surface waters with phosphorus and nitrogen. Ecological Applications, 83, 559568.
-
Dong, J., Zhang, Y., Zhang, S., Wang, Y., Yang, Z. and Wu, M. (2010) Identification of Temporal and Spatial Variations of Water Quality in Sanya Bay, China By Three-Way Principal Component Analysis. Environ. Earth Sci., 60, 1673-1682.
-
Elhatip, H., Hinis, M. A. and Gulgahar, N. (2007) Evaluation of the water quality at Tahtali dam watershed in Izmir, Turkey by means of statistical methodology. Stochastic Environmental Research and Risk Assessment, 22, 391-400.
-
Google Maps (2016) Google Maps [Online]. Available at https://www.google.ca/maps/ [accessed 3 October 2016].
-
Haag, I., and Westrich, B. (2002) Processes Governing River Water Quality Identified By Principal Component Analysis.
Hydrol. Process, 16, 3113-3130.
-
Huang, F., Wang, X., Lou, L., Zhou, Z. and Wu, J. (2010) Spatial Variation and Source Apportionment of Water Pollution in Qiantang River (China) using Statistical. Water Res., 44, 1562-1572.
-
Li, Y., Xu, L. and Li, S. (2009) Water quality analysis of the Songhua River Basin using multivariate techniques. Journal of Water Resource and Protection, 1 (2), 110121.
-
Liu, C. W., Lin, K. H. and Kuo, Y. M. (2003) Application of factor analysis in the assessment of groundwater quality in a Blackfoot disease area in Taiwan. Sci. Total Environ., 313 (1-3), 7789.
-
Mahapatra, S. and Mitra, S. (2012) Managing Land and Water under Changing Climatic Conditions in India: A Critical Perspective. Journal of Environmental Protection, 3 (9), 1054-1062.
-
McKenna, J. E. (2003) An enhanced cluster analysis program with bootstrap significance testing for ecological community analysis. Environmental Modelling & Software, 18 (3), 205-220.
-
Mishra, A. (2010) Assessment of water quality using principal component analysis: A case study of River Ganges. Journal of Water Chemistry and Technology, 32 (4), 227-234.
-
Natural resources (2016) Statistics Canada [Online]. Available at: http://www.statcan.gc.ca/ [accessed 15 October 2016].
-
Ouyang, Y., Nkedi-Kizza, P., Wu, Q. T., Shinde, D. and Huang, C. H. (2006) Assessment of seasonal variations in surface water quality. Water Research, 40, 38003810.
-
Qadir, A., Malik, R. N. and Husain, S. Z. (2007) Spatio-temporal variations in water quality of Nullah Aik-tributary of the river Chenab, Pakistan. Environmental Monitoring and Assessment, 140 (13), 4359.
-
Reghunath, R., Murthy, T. R. and Raghavan, B. R. (2002) The utility of multivariate statistical techniques in hydrogeochemical studies: An example from Karnataka, India. Water Research, 36, 24372442.
-
Salah, E. A., Turki, A. M. and Al-Othman, E. M. (2011) Assessment of water quality of Euphrates River using cluster analysis. Journal of Environmental Protection, 3, 1629-1633.
-
Sharaf El Din, E., Zhang, Y. and Suliman, A. (2017a) Mapping concentrations of surface water quality parameters using a novel remote sensing and artificial intelligence framework. International Journal of Remote Sensing, 38 (4), 1023-1042.
-
Sharaf El Din, E. and Zhang, Y. (2017b) Estimation of both optical and nonoptical surface water quality parameters using Landsat 8 OLI imagery and statistical techniques. J. Appl. Remote Sens., 11 (4), 046008, doi: 10.1117/1.JRS.11.046008.
-
Sharaf El Din, E. and Zhang, Y. (2017c) Improving the accuracy of extracting surface water quality levels (SWQLs) using remote sensing and artificial neural network: a case study in the Saint John River, Canada. Int. Arch. Photogramm. Remote Sens. Spatial Inf. Sci., XLII-4/W4, 245-249.
-
Sharaf El Din, E., and Zhang, Y. (2017d). Statistical estimation of the Saint John River surface water quality using Landsat-
8 multi-spectral data. ASPRS Annual Conference. Proceedings of Imaging & Geospatial Technology Forum (IGTF). Baltimore, US.
-
Sharaf El Din, E., and Zhang, Y. (2017e). Neural network modelling of the Saint John River sediments and dissolved oxygen content from Landsat OLI imagery. ASPRS Annual Conference. Proceedings of Imaging & Geospatial Technology Forum (IGTF). Baltimore, US.
-
Sharaf El Din, E. and Zhang, Y. (2018) Application of multivariate statistical techniques in the assessment of surface water quality in the Saint John River, Canada. UNB Annual Graduate Research Conference (GRC). Fredericton, Canada.
-
Sharaf El Din, E. (2019a). Enhancing the accuracy of retrieving quantities of turbidity and total suspended solids using Landsat-8-based-Principal Component Analysis technique. Journal of Spatial Science, DOI: 10.1080/14498596.2019.1674197.
-
Sharaf El Din, E., Afify, H., and Zhang, Y. (2019b). Statistical Estimation of Turbidity and Total Suspended Solids by Means of Landsat-8 Reflectance and Principal Component Analysis. Canadian Symposium on Remote Sensing and Geomatics Atlantic. New Brunswick, Canada.
-
Shrestha, S. and Kazama, F. (2007) Assessment of surface water quality using multivariate statistical techniques: A case study of the Fuji river basin, Japan. Environmental Modelling & Software, 22, 464475.
-
Simeonov, V., Stratis, J. A., Samara, C., Zachariadis, G., Voutsa, D. and Anthemidis, A. (2003) Assessment of the surface water quality in Northern Greece. Water Research, 37, 41194124.
-
Singh, K. P., Malik, A., Mohan, D. and Sinha, S. (2004) Multivariate statistical techniques for the evaluation of spatial and temporal variations in water quality of Gomti River (India): a case study. Water Research, 38, 3980-3992.
-
Tahir, A. A., Quazi, K. H. and Gopal, A. (2011) A Methodology for Clustering Lakes in Alberta on the basis of Wate Quality Parameters. Clean Soil, Air, Water, 39 (10), 916924.
-
Vega, M., Pardo, R., Barrado, E. and Deban, L. (1998) Assessment of seasonal and polluting effects on the qualityof river water by exploratory data analysis. Water Research, 32, 35813592.
-
Wunderlin, D. A., Diaz, M. P., Ame, M. V., Pesce, S. F., Hued, A. C. and Bistoni, M. A. (2001) Pattern recognition techniques for the evaluation of spatial and temporal variations in water quality. A case study: Suquia river basin (Cordoba, Argentina). Water Research, 35, 28812894.