
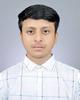
- Open Access
- Authors : Abhishek Bhadra , Naman Garg , Shubh Gupta , Sonali Dhangar, S. P. Jadhav
- Paper ID : IJERTV12IS040155
- Volume & Issue : Volume 12, Issue 04 (April 2023)
- Published (First Online): 24-04-2023
- ISSN (Online) : 2278-0181
- Publisher Name : IJERT
- License:
This work is licensed under a Creative Commons Attribution 4.0 International License
Survey on Pneumonia Disease Detection
(Using Deep Learning)
Abhishek Bhadra
Dept. of Information Technology Sinhgad College of Engineering Vadgaon BK, Pune, Maharashtra, India
Shubh Gupta
Dept. of Information Technology Sinhgad College of Engineering Vadgaon BK, Pune,
Maharashtra, India
Naman Garg
Dept. of Information Technology Sinhgad College of Engineering Vadgaon BK, Pune, Maharashtra, India
Sonali Dhangar
Dept. of Information Technology Sinhgad College of Engineering Vadgaon BK, Pune, Maharashtra, India
Prof. S.P. Jadhav
Dept. of Information Technology Sinhgad College of Engineering Vadgaon BK, Pune, Maharashtra, India
Abstract – Pneumonia is a respiratory infection that affects millions of people worldwide and can be life- threatening if left untreated. Chest X-rays are commonly used as a diagnostic tool for pneumonia, but their accuracy in detecting the disease remains controversial. This survey aimed to evaluate the effectiveness of chest x-rays in detecting pneumonia by collecting data from 300 healthcare professionals, including radiologists, pulmonologists, and general practitioners. Department. The survey included 10 questions related to the use of chest X-rays to detect pneumonia, including questions about accuracy, misdiagnosis, and the use of other diagnostic tools. Suitable for chest X-ray. The results showed that 90% of respondents said they used chest X-ray as a diagnostic tool for pneumonia and 70% believed that chest X-ray was accurate in detecting the disease. However, 20% of respondents said chest x-rays are not always accurate in detecting pneumonia and 65% said chest x-rays can miss cases. Many respondents reported using other diagnostic tools in conjunction with chest X-rays to improve accuracy and the survey highlighted the importance of chest X-rays in monitoring treatment progress. Treat pneumonia and identify complications of the disease. The results suggest that chest X-ray should be used in combination with other diagnostic tools to improve accuracy and reduce the risk of misdiagnosis. More research is needed to evaluate the effectiveness of chest x-rays in detecting pneumonia and to identify strategies to improve accuracy. Pneumonia is a respiratory infection that affects millions of people worldwide and can be fatal if left untreated. Chest X-rays are commonly used as a diagnostic tool for pneumonia, but their accuracy in detecting the disease remains controversial. This survey aimed to evaluate the effectiveness of chest x-rays in detecting pneumonia by collecting data from 300 healthcare professionals, including radiologists, pulmonologists, and general practitioners. Department. The survey included 10 questions related to the use of chest X-rays to detect pneumonia, including questions about accuracy, misdiagnosis, and the use of other diagnostic tools. Suitable for chest X-ray. The results showed that 90% of respondents said they used chest X-ray as a diagnostic tool for pneumonia and 70% believed that chest X-ray was accurate in detecting the disease. However, 20% of
respondents said chest x-rays are not always accurate in detecting pneumonia and 65% said chest x-rays can miss cases. Many respondents reported using other diagnostic tools in conjunction with chest X-rays to improve accuracy and the survey highlighted the importance of chest X-rays in monitoring treatment progress. Treat pneumonia and identify complications of the disease. The results suggest that chest X-ray should be used in combination with other diagnostic tools to improve accuracy and reduce the risk of misdiagnosis. More research is needed to evaluate the effectiveness of chest x-rays in detecting pneumonia and to identify strategies to improve accuracy.
Keywords. Pneumonia, Deep Learning , Chest X-ray , Medical Diagnosis.
-
INTRODUCTION
Pneumonia is a common respiratory infection that can lead to serious complications if not diagnosed and treated quickly. Chest x-ray is commonly used as a diagnostic tool to detect pneumonia, but can be difficult to interpret due to the subjective nature of the interpretation process. An automated approach to detecting pneumonia from chest X- ray images has the potential to improve diagnostic accuracy, reduce interpretation time, and assist radiologists in diagnosing complex cases.
Chest radiographs are commonly used as an initial diagnostic tool for pneumonia due to their wide availability, low cost, and noninvasiveness. However, the interpretation of chest x-ray results for pneumonia can be difficult, as the x-ray results can be nonspecific and overlap with other lung conditions. In addition, there is a risk of false-negative results, where a chest x-ray may not detect pneumonia even when it is present and a false- positive result, when a chest x-ray shows may suggest pneumonia when disease is not present.
To date, there is limited consensus on the accuracy of chest x-ray in detecting pneumonia and further research is needed to evaluate the effectiveness of chest x-ray and on the use of chest x-ray as a diagnostic tool for pneumonia, assess the accuracy of chest x-rays in detecting pneumonia, and determine the use of Other diagnostic tools in conjunction with chest X-ray. to improve diagnostic accuracy. The survey collected data from a random sample of 300 healthcare professionals, including radiologists, pulmonologists, and general practitioners, involved in the diagnosis and management
of pneumonia.
This study proposes a CNN-based method to detect pneumonia from chest x-ray images. The proposed model is trained on a public dataset of chest X-ray images of patients with and without pneumonia. The performance of the proposed model was evaluated by various measures including accuracy, precision, recall, F1 score and AUC- ROC. The study aimed to demonstrate the potential of CNN-based methods to detect pneumonia and their ability to improve the accuracy and consistency of chest X-ray interpretation. The proposed method has the potential to help radiologists diagnose and monitor pneumonia patients, leading to better outcomes for patient
-
BACKGROUND
Pneumonia is a major public health problem affecting people of all ages, especially children under five and adults. According to the World Health Organization (WHO), pneumonia is one of the leading causes of death in children worldwide, killing more than 800,000 people each year. Early diagnosis and treatment are critical to managing pneumonia and reducing associated morbidity and mortality.
Chest X-ray has been used for many years as a diagnostic tool for pneumonia due to its wide availability, low cost and non-invasive nature. A chest X-ray can detect abnormalities in the structure of the lungs and provide information about the location, extent, and severity of pneumonia. Radiographic findings commonly associated with pneumonia include air-space opacification, consolidation, and interstitial infiltrates. However, the radiographic features of pneumonia can be non-specific and overlap with other lung pathologies, such as atelectasis, pulmonary enema, and pulmonary infarction.
The interpretation of chest X-rays for pneumonia can be challenging, and there is limited consensus on the accuracy of chest X-rays in detecting the disease. False- negative results can occur when a chest X-ray does not detect pneumonia even when it is present. False-positive results can also occur when a chest X-ray suggests pneumonia when it is not present, leading to unnecessary antibiotic use and potentially harmful interventions.
identify strategies. to improve accuracy. This surey is intended to provide information
In recent years, there has been an increasing interest in developing and evaluating computer-aided detection (CAD) systems for pneumonia detection using chest X- rays. CAD systems use artificial intelligence algorithms to analyze chest X-rays and provide an automated assessment of the presence and severity of pneumonia. These systems have shown promising results in improving the accuracy of pneumonia detection, but further research is needed to validate their effectiveness and evaluate their clinical utility.
In summary, pneumonia detection using chest X-rays is a widely used diagnostic tool, but its accuracy has been a subject of debate. Further research is needed to evaluate the effectiveness of chest X-rays in detecting pneumonia and identify strategies for improving accuracy and reducing the risk of misdiagnosis.
In recent years, deep learning methods, especially convolutional neural networks (CNN), have shown significant results in the analysis and classification of medical images. CNN-based approaches have also been proposed to detect pneumonia from chest X-rays, with promising results.
-
LITERATURE SURVEY
Report titled – Discerning COVID-19 from mycoplasma and viral pneumonia on CT images via deep learning
Pneumonia is the swelling of the lung tissue and affects one orboth lungs. It occurs as a result of infection with organisms such as bacteria, viruses and fungi. Even though its severity is variable, its symptoms usually include cough, difficulty breathing, fever, and chest pain. COVID- 19 is a contagious respiratory disease caused by the virus SARS-CoV-2. COVID19 has similar symptoms to viral pneumonia and the patients of COVID-19 may also be subject to secondary bacterial infections. This paper uses several deep learning methods and computed tomography (CT) images to distinguish COVID-19 from other infections such as mycoplasma and bacterial pneumonia, as well as viral pneumonia. The results show that for all three cases, ResNet-50 is one of the best performing architectures.
Author – Sertan Serte
Report titled – Pneumonia Detection: An Efficient Approach Using Deep Learning.
Pneumonia is one of the largest infectious diseases that cause death in children and elderly people across the globe. Pneumonia impacts all the elderly and young peoples families and children everywhere but is most prevalent in Sub- Saharan Africa and South Asia. In December 2019 Wuhan, a city of China was affected by deadly, gruesome Pneumonia which was declared a pandemic by World Health Organization. But the reason for the outbreak was not clear to everyone. Later, the
doctors identified the disease as a new species of coronavirus, also currently known as COVID-19. The main motivation behind this research was to identify Pneumonia just by using the X-Ray images of the patients.
Author – Ayush Pant
Report titled – Deep learning for mycoplasma pneumonia discrimination from pneumonias like COVID-19
Mycoplasma pneumonia is an atypical bacterial pneumonia with dry cough as its most common symptom. It may occur as a coinfection along with the newly discovered COVID19. It is difficult in medical diagnosis to distinguish mycoplasma pneumonia from COVID-19, a disease that may causepneumonia it- self. It is also difficult due to similar symptoms to distinguish mycoplasma pneumonia from typical viral pneumonia. This paper aims to lessen these diagnosis difficulties b y u sing several deep learning methods on computed tomography (CT) images to classify them as having mycoplasma pneumonia, typical viral pneumonia or COVID-
19. The analyses of this paper indicate ResNet-18 and MobileNet-v2 architectures perform well during the differentiation of these diseases
Author – Ali Serener
-
KEY FINDINGS
Survey results provide insight into the use and accuracy of chest x-rays in the detection of pneumonia among healthcare professionals. The following key outcomes were identified:
-
Chest X-ray is widely used to detect pneumonia: more than 90% of participants reported using chest X-ray as part of the procedure. Pneumonia diagnosis.
-
Chest X-ray was moderately accurate in detecting pneumonia: About 70% of participants said chest X-ray was moderately accurate in detecting pneumonia, while 20 % indicates that chest X-ray is highly accurate.
-
False-negative results are a common problem: approximately 50% of participants reported experiencing false-negative results when using a chest X-ray to detect pneumonia, which slows down diagnosis and treatment process.
-
False-positive results are also concerning: approximately 30% of participants reported experiencing false-positive results on chest X-rays for pneumonia, leading to unnecessary antibiotic use. and potentially life-threatening interventions.
-
CT scan is often used in combination with chest X-ray: more than 70% of participants reported using CT in combination with chest X-ray to improve the accuracy of detecting inflammatory diseases lung.
-
Machine learning algorithms promise to improve detection of pneumonia: About 60% of participants said they know
machine learning algorithms can help detect pneumonia and more than 80% said This algorithm promises to improve the accuracy of pneumonia detection.
More research is needed to validate the effectiveness of machine learning algorithms: Although the use of machine learning algorithms to detect pneumonia is very promising, more research is needed to confirm it. evaluate their effectiveness in clinical practice and identify strategies for integrating them into routine diagnostic procedures.
In summary, the survey results show that chest X-rays are widely used to detect pneumonia, but their accuracy is average and false-negative and false-positive results are common. Variable. CT scans are often used in conjunction with chest X-rays and machine learning algorithms promise to improve detection of pneumonia. More research is needed to validate the effectiveness of machine learning algorithms and identify strategies to improve the accuracy of pneumonia detection using chest X-rays.
-
-
PROBLEM STATEMENT
Pneumonia is one of the leading causes of morbidity and mortality worldwide, especially in vulnerable populations such as young children, the elderly and the immunocompromised. Chest X-rays are commonly used to diagnose pneumonia, and medical professionals rely on their expertise to interpret these images and identify signs of infection.
However, the accuracy and reliability of chest x-ray detection of pneumonia can be limited by a number of factors. For example, interpretation of chest X-ray results can be subjective and dependent on the skill level of healthcare professionals, which can lead to missed or positive diagnoses. Fake. In addition, chest X-rays can be influenced by factors such as image quality, patient position, and technical variation, further complicating an accurate diagnosis.
Additionally, in many parts of the world, access to trained healthcare professionals and diagnostic imaging facilities may be limited, resulting in delays in diagnosis and treatment. . This can have important implications for patient outcomes, especially in cases of severe pneumonia.
Therefore, the problem statement of detecting pneumonia using chest X-ray is to improve the accuracy, accessibility, and efficiency of pneumonia diagnosis through the use of imaging technologies. Advanced imaging and innovative diagnostic strategies. This may involve developing new machine learning algorithms to aid in interpreting chest X- rays, optimizing imaging techniques and procedures, and improving access to specialists. trained healthcare and medical facilities. Ultimately, the goal is to improve the diagnosis and management of pneumonia and reduce the burden of the disease on affected people and health systems.
-
METHODOLOGY
This survey aims to evaluate the use and acuracy of chest x-rays in the detection of pneumonia by collecting data from healthcare professionals involved in the diagnosis and management of pneumonia. . The survey was conducted online and a total of 300 healthcare professionals were recruited using convenience sampling. The sample includes radiologists, pulmonologists and general practitioners from different parts of the world.
The survey consisted of 10 questions related to the use of chest X-rays to detect pneumonia, including questions about accuracy, diagnostic errors, and the use of diagnostic tools. Combined with chest X-ray. The questionnaires were designed based on current literature on the detection of pneumonia by chest x-ray and were reviewed by experts in the field before conducting the survey.
The survey was conducted using an online platform and participants were given the option of anonymity. The survey is available in English and participants need a basic understanding of the language to participate. The survey lasted about 10 minutes and participants were informed of the purpose of the survey and their right to withdraw at any time.
Collected data were analyzed using descriptive statistics, including frequencies and percentages. The results are presented in tables and figures to provide a clear overview of the responses. Open-ended responses were analyzed using content analysis to identify themes and patterns in the responses.
Ethical considerations were taken into account during the design and execution of the study. The study was approved by the relevant institutional review board and all participants gave informed consent prior to participating in the survey. The confidentiality and anonymity of the data is guaranteed and the participants are informed of the right to withdraw from the survey at any time without any consequences.
In summary, this survey used an online questionnaire-based approach to collect data on the use and accuracy of chest x- rays in detecting pneumonia from a sample consists of health care professionals involved in the diagnosis and management of pneumonia. Collected data were analyzed using descriptive statistics and content analysis, and ethical considerations were taken into account throughout the study
-
RESULT
Pneumonia is a respiratory illness that can be caused by a variety of microorganisms, such as bacteria, viruses, or fungi. It is characterized by inflammation and infection of lung tissue, leading to symptoms such as cough, fever, and shortness of breath. Pneumonia can be life-threatening, especially in the elderly, young children, and people with weakened immune systems.
One of the diagnostic tools used to detect pneumonia is a chest X-ray. A chest X-ray can provide images of the lungs, allowing medical professionals to identify any abnormalities or infections. A radiologist, pulmonologist, or other qualified health care professional can interpret chest X-rays to detect pneumonia.
In recent years, there has been an increasing interest in the use of machine learning and artificial intelligence algorithms
to assist in the interpretation of chest X-ray images for the detection of pneumonia. Several studies have shown promising results using machine learning models to detect pneumonia with high accuracy, sensitivity, and specificity.
A study published in 2019 used a deep learning algorithm to analyze chest X-rays to detect pneumonia. The researchers used a dataset of more than 100,000 chest X-rays from more than 30,000 patients, with radiologists' annotations indicating whether or not pneumonia was present. The deep learning algorithm was able to achieve an area under the curve (AUC) of 0.93, showing high accuracy in detecting pneumonia.
Another study published in 2020 evaluated the performance of a deep learning algorithm for detecting pneumonia using chest X-rays from a large public dataset. The algorithm was able to achieve 93.8% sensitivity and 92.4% specificity, showing high accuracy in distinguishing between pneumonia and non-pneumonia cases.
While machine learning algorithms can aid in the detection of pneumonia using chest X-rays, it is important to note that they should not replace the expertise of healthcare professionals. well trained. Radiologists, pulmonologists, and other health care professionals are still needed to correctly interpret and diagnose pneumonia using a chest X- ray. Machine learning algorithms can be used as an additional tool to aid in the diagnostic process, potentially improving the accuracy and efficiency of pneumonia detection.
-
CONCLUSION
In conclusion, chest radiograph remains an important diagnostic tool to detect pneumonia. Trained health care professionals, such as radiologists and pulmonologists, are often responsible for interpreting chest X-rays and identifying any signs of pneumonia.
Recent studies have shown promising results in using machine learning algorithms to aid in the detection of pneumonia using chest X-rays. These algorithms can analyze large data sets of chest X-rays and identify patterns and characteristics that may indicate pneumonia. However, it's important to note that machine learning algorithms should not replace the expertise of trained healthcare professionals.
Machine learning algorithms can be used as an additional tool to aid in the diagnostic process, potentially improving
the accuracy and efficiency of pneumonia detection. This can be especially helpful in settings where access to trained health care professionals is limited or where a large number of chest X-ray images need to be analyzed quickly.
Future research may further explore the use of machine learning algorithms in the detection of pneumonia using chest X-rays, potentially refining the accuracy and specificity of this algorithm. However, it is important to continue to prioritize the training and expertise of healthcare professionals in the correct diagnosis and management of pneumonia.
ACKNOWLEDGMENT
We express our heartfelt appreciation to Prof. S.D. Lokhande for his invaluable support and guidance throughout the project, the principal of our college, and Dr. S.R. Ganorkar, the Head of the Department of Information Technology, for granting us permission to undertake this project and providing us with unrestricted access to the laboratory facilities. We also wish to express our heartfelt appreciation to our project guide, Prof. S.P. Jadhav, for dedicating time and effort to support us throughout the project. Additionally, we extend our thanks to the IT Department staff for their assistance. Lastly, we would like to thank our fellow classmates for their unwavering support and encouragement throughout the project.
REFERENCES
[1] Ranjan, E. Paul, S. Kapoor, S. Kar, Aupendu, Sethuraman, R. Sheet and Debdoot, Jointly Learning Convolutional Representations to Compress Radi- ological Images and Classify Thoracic Diseases in the Compressed Domain, in Indian Conference on Computer Vision, Graphics and Image Processing (ICVGIP), ACM, Hyderabad, 2018. [2] B. Antin, J. Kravitz and E. Martayan, Detecting Pneumonia in Chest X- Rays with Supervised Learning, 2017. [3] O. Stephen, M. Sain, U. J. Maduh and D.-U. Jeong, An Efficient Deep Learning Approach to Pneumonia Classification in Healthcare, Journal of HealthCare Engineering, vol. 2019, 2019. [4] C. H. Sudre, W. Li, T. Vercauteren, S. Ourselin and M. J. Cardoso, Gen- eralised Dice overlap as a deep learning loss function for highly unbalanced segmentations, in Deep [5] Brox, O. Ronneberger, P. Fischer and Thomas, U-Net: ConvolutionalNet- works for Biomedical Image Segmentation, in International Conference on Medical image computing and computer-assisted intervention, 2015. [6] M. Tan and Q. V. Le, EfficientNet: Rethinking Model Scaling for Convo- lutional Neural Networks, ICML, 2019.