
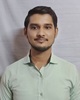
- Open Access
- Authors : Mitesh Chanodiya , Dr. Manish Potey
- Paper ID : IJERTV10IS070275
- Volume & Issue : Volume 10, Issue 07 (July 2021)
- Published (First Online): 31-07-2021
- ISSN (Online) : 2278-0181
- Publisher Name : IJERT
- License:
This work is licensed under a Creative Commons Attribution 4.0 International License
Streamlining Power Consumption in Data Hubs of Distributed Clouds
Mitesh Chanodiya
Department of Computer Engineering,
K.J. Somaiya College of Engineering, Vidyavihar, Mumbai- 400077.
Dr. Manish Potey
Department of Computer Engineering,
-
Somaiya College of Engineering, Vidyavihar, Mumbai-400077.
Abstract: – As per the growing demand for various new technologies, including Internet-of-Things and Cloud Computing; Services end in an unparalleled strength intake for many infrastructures. Flexibility to scale, highly available and cost-effectiveness are concepts that can be understood using this framework of distributed clouds. However, the growth of these information facilities in cloud computing has resulted in uneconomic consumption of power. Infrastructures are overprovisioned in order to determine that the services are reliable, a memory so that data to be store, computation and conditioning to cool hardware. Locus- influenced schedule Algorithm to scale back cloud computation energy also known as LACE will reduce the consumption of the power used in cloud data hubs. This paper also describes an exhaustive study on the usage of IoT which can be used to create a consumer-friendly power monitor and manage utility systems. The solutions for power efficiency and web awareness to allocate resources in cloud environments are described.
Keywords: – Internet-Of-Things, data hubs, LACE, power consumption, energy efficiency.
-
INTRODUCTION
Energy management is more important than ever in this digital age which is important altogether depending on style of living and product categories. If it is household utilities or may be the industry related machinery, power must be optimized. The cloud assets has a group of interconnected as well as heterogeneous or homogeneous systems which have unproven assets like web, computation and disk etc. The most logic for their reputations include utilization, flexible to scale, high availability, tolerate the failure, reliable and economical, and straightforward to usage. Streamlining consumption of energy by cloud assets is among the significant topics survived in both in academia and industry.
The main objective is to make the rule of implementing a self or auto regulating electric meter which will include analysis, management and lift the use of facility and let the use of software application by user to access facility usage to track the service. The client should have control to access through the software application to utilities or computing services which will be connected to the server or cloud that might store required all the data associated with power usage such as categorized by time, date and utilities. The end client should also has control access and whole data to master computer, utilities and application system through RFID & NFC. The connectivity fabric's role in power usage is also addressed.
The era of computing and recent exponential enlargement of cloud services has conclude in energy consumption that is not cost effective, which leads as danger to the atmosphere e.g. of dangerous threat is generation of large carbon footprints of data hubs of cloud services. As per the resources we can say that the typical or particular data hub consumes the maximum energy amount as equivalent to 25,000 households. A mean data hub has production quite one hundred fifty metric million plenty of carbon in a year. Fig. 1 below depicts data hub of Amazon Web Service (AWS) located in Argentina which shows various components such as systems for storage, switches, routers, firewalls and controllers for application delivery. Thus, due to these many number of components power consumed in these data hubs is large.
Fig.1. Cloud Data Hub of AWS in Argentina [1]
Emissions of carbon which are generated through computing of cloud are expected worldwide in 2021 of approximate total as metric million 670 tons. Also, the max use of power consumption at data hubs rise the cost of operation. In US in 2013, data hubs used an predicts kilo watt hours of 91 billion of power[2]. The massive volumes of power consumed at these data hubs increases operational costs. Green computation of cloud goal to save atmosphere from data hubs emissions of carbon by low consume of power.
In IT industry, consumption of energy has never been a goal. 30 years back from 1980s, the sole goal has always been to produce more but also faster; historically, this has been accomplished by cramming everything into a smaller package and operating processor at a better frequencies. Moreover, so as to make sure, infrastructures are overprovisioned in order to determine that the services are reliable. Thus, two metrics were developed as stated:
Energy facility Use Effective (EUE) and Information Hub Infrastructure Efficient (IHIE) in order to streamline power consumption. As a result, effective energy is among the most key measures in deciding running costs and total investments in contemporary cloud computing data hubs, as well as the industry's performance and footprint of carbon.
Section I shows the introduction part, Section II contains the literature survey determining the streamlined power consumption in data hubs, Section III includes hardware and software optimization techniques, Section IV explains the design methodology and different algorithms used to streamline power, Section V shows the results and Section VI focuses on conclusion.
-
LITERATURE SURVEY
In 2018, Cloud computing experts implemented the Collective Self Consumption as shown in Fig. 2 which describes a smart meter which can be the combined form of an electric web with a fanatical infrastructures of control of ICT which grants the previous to extend its flexible nature. Thus, the approaching huge scale deploy nature of those smart meter has grant the development of latest techniques to manage energy which will influence the extend the contribution of renewable sources within the mix of energy[3].
Fig.2. Improved Photovoltaic Self- Consumption
The technique is also named as collective self consumption which is close to appear in many countries. This idea contains the grant to many consumers and energy sources, located during a low geographic place to determine purchase power to be used in that place.
The cost required in data hubs of distributed clouds, which is covered by supported web used tariff, includes both technological and non-technological semi- cost like:
-
Energy loss within distributed generation (mainly wires and cords), or instance, due to its electrical resistivity
-
Aging ancillary appliances
-
Generator management solutions, like metering and so on.
In 2018, cloud experts implemented the Power-effective Schedule Framework by use of Linear Address Theory. The study focuses on scheduling that saves energy, particularly for real-time tasks[4]. They suggested a Linear Address algorithm as depicted in Fig. 3, to encourage the
use of cloud assets, thus lowering the cloud system's energy consumption.
Fig.3. A Learning Automata & its Environment
The Linear Address Thesis has been used to guess utilities use in order to prevent overuse / underuse of PMs, as well as shutting down idle PMs. They submitted a survey on resource allocation that is energy efficient.
Cloud computation is a typical computer environment that stores data over the internet. Their study examines the benefits and drawbacks of various allocation techniques, as well as issues which are open and directions in future in cloud utilities allotmen. They also proposed a cloud-based scheduling architecture that uses the rolling horizon principle to schedule tasks in real time. An awareness in power scheduler will be intended to improve the job's schedulable and resource preservation.
The cloud domain experts investigated multiple utility schedule schemes for cloud Infrastructure as a Utility. Also need to look at different scheduling schemes that support the issue at hand, as well as the metrics used for assessment.
Researchers in cloud computing services domain implemented ARMA Energy Prediction Model which shows optimize web technology which are modeled to scale back consumed power by reducing web traffic in servers[5].
To prevent overlapping paths, the first method produces various disjoint-spanning trees and chooses the reroute how path that uses the least amount of space as described in Fig.
-
The second method moves virtual machines from server which are either under or over utilized by machine that is nearest to them in terms of network distance. The Web Simulation WS2 and the Simulator Cloud Sim are used to analyse both methods.
Fig.4. Network Relay Node System Model
The algorithm aims to consolidate Virtual machines on a limited number of computers by balanced consolidated assets (memories, processors and communication bandwidth) accessed by adoption of cloud computation data centres at the same time. It takes into account a wide range of workloads and different resource consumption features. Because heterogeneous workloads have distinct utilization of resource features, goal is to lower the power spent by increasing assets utilisation. The findings of the experiments demonstrate that multidimensional assets have a well-balanced utilisation and save power when compared to other techniques. The algorithms, on the other hand, are completely centralised and include significant computing overheads.
Also, the cloud domain experts and professors states that they have implemented IoT Power Control System by Management of Power and Control Appliances which describes study on the IoT and how control power services has aided for the development of a more credible infra for energy optimization in home operations[6]. Further research into these area has resulted in the development of highly effective smart sensor systems, providing us the tools to make smart house utilities. As sustainable development initiatives are undertaken to implement a great home which is smart enough, also user compatibility and security take precedence over facility control. The poverty of electric & reuse energy assets in developed countries is limiting urbanization in these regions. Renewable energy sources are also commonly seen in some locations, but their optimization isn't satisfactory.
-
-
HARDWARE AND SOFTWARE OPTIMIZATION According to the report, pressing challenges in green cloud atmosphere is deciding the exact position of new Virtual
Machine requests which came to servers in physical space in order to decrease energy consumption. This issue has spawned a slew of academic initiatives. However, such endeavours are still in their infancy and is divided in three different parts:
-
Hardware,
-
Web,
-
Software optimized techniques initiation.
By using flexibility in hardware which will governs computation of server capability by regulating running server frequency along with voltage, hardware optimization strategies decrease energy consumption levels. The DVFS method is described in the paper as a result of this method. DVFS makes use of a variety of the special CPU which will run in different levels of voltage as well as frequency. To reduce utilization of power altitude without breaking Service Level Agreements endorsed load of work criteria on VM, DVFS chooses acceptable supply voltages and frequencies for processing components. They proposed the Power Awareness Utility Effective work flow Scheduler, which also uses servers named as DVFS which will find the optimized frequency height suitable for task during a science work flow under Deadline constraints (EARES- D)[7]. Under the deadline constraint, the optimized frequency for completing every task is find out by reducing the frequency of processor. Policies such as reuse of VM and ideal time reduction were commonly make into use to increase asset utilisation effectiveness. But, since they require the use of specialised hardware, hardware optimized hardware methods for clouds in green computation tends to be usually expensive also will have limited scalability.
-
-
DESIGN METHODOLOGY AND ALGORITHMS Each of the five research papers had used different strategy and algorithm to make significant use of power and get the most out of cloud data resources. The experts of the respective research papers' real implementation and system design model is as follows: –
-
Smart Grids
-
-
The cost of electricity (in e/kWh) provided to the cloud by another energy provider is factored in to account for grid use costs, which is customary.
AE = AE,p + CE,c
which is a common formula for expressing the energy cost AE, where AE,p and AE,c will be fixed & changing power cost shares AE, respectively[8]. Like wise quantity of energy E, the variable share CE,v is frequently termed as: CE,c = { 0.06 E if E is penetrated into meter
{ 0.15 E if E is bought
Scorpius Algorithm
-
Allocate new VMs;
-
Allocation revision;
-
VMs which are running need to be migrated;
-
for loop start 1 j N do
-
DCj consolidation
-
end of for loop[8]
Fig.5. PV energy produced computation for the time varies t till (t+1)
Table 1: Values at the output after applying Scorpius Algorithm
Term 1
Term 2
Term 3
Term 4
ci (CE / kWh)
4.18
2.81
1.89
1.74
-
Learning Automata
The energy used mostly by VM is categorised: active state energy consumption and idle state energy consumption. When a task is being executed, we suppose that the VM is active. The energy utilized in the idle state is similar to the energy consumption in the active state for one hour. The VM's processing energy is taken into account here as shown in scheduling architecture in Fig. 6. A VM's computation energy is the amount of energy used to perform the tasks that have been allocated to it[9].
Amount of computation (Cj) done by VM vmj is Cj =kji=1 wi.
The power used of VM vmj per MI can be
j = 108 ×(spj) 2 Joules/MI [7].
Final power (j) used by vmj in state which is active is decided can be
j = Cj × j
Power used by vmj in state which is idle is 0.6*j. Final power utilized by cloud assets or environment is
EN = 1.6 ×nj=1 j
where, we can say n is no of VMs availability at the specific instant.
Fig.6. Scheduling Architecture
Task Scheduling Algorithm
Input : Constant at Reward() and constant at Penalty (), it iterator , Max iterator itmax,
List of VM is V , list of task is T. Output: Optimized Task.
1: Initially all probabilities of action prij and set it = 1; 2: Set work task randomly ti to a VM vmj ;
3: while loop it _= itmax do
4: Calculation of time for finish (ij ) of work ti on VM vmj using Equation 2;
5: if ij di then
6: Calculate utilized power (EN) of assignment by use of eqn 4.
7: end of if loop
8: if ENit ENit1 then
9: selected actions need to be rewarded by use of eqn 5. 10 : or else
11 : selected actions need to be penalized by using eqn 5. 12: end of if loop
13: Increment it;
14: Actions with highest probabilities must be selected as required while assignment.
15: end while[10]
Fig.7. Graph : Virtul Machines v/s Energy
-
Automated Power Consume, Appliance Control
IOT experts proposed an approach that can be broadly categorized into three important parts as follows:-
-
Sensor Network
The designed scheme offers a practical solution for regulating home appliances with the help of an AI modulator. This relies output of sensor to function. Sensor web use a combined form of LM35 temp sensors along with humid sensors joint via Zigbee for controlling house appliances if temp falls below a set point.
-
Smart Meter
Our smart meter is the most important component of our model. It's powered by a custom microcontroller that's linked to the facility's power sources. Renewable energy, power system, and finally auxiliary power are used to power the meter as shown in Fig. 8. The smart meter's main aim is the usage of renewable resource to the greatest extent possible before switching to grid power.
Fig.8. Appliance System Control Work flow[11]
-
Cloud Server & Security
The implemented managing energy includes a knowledge managing system of server, just like every managing and optimized work or automatic system. However, in order to develop the effectiveness of IoT system, the design would include a cloud server rather than a central data centre[11].
-
-
Locust Inspired Scheduling Algorithm
A cloud infrastructure data hub mimics to a locust swarm in that both have huge no of server and a huge no of locust. A master is compared to the locust in this instance. A server accepts virtual machines from an arriving VM waiting queue while the locust eats grass (or poorer grasshoppers for an infant locust in the affable process) i.e. invoking of help server or the servers which are LIGHT.
-
Mapped Phase
The primary phase for all master types is mapped phase. A vivid master accepts an arriving Virtual Machine request during this phase (PS or WS). First-Come, First-Served (FCFS) is the method of allocating resources. As a result, the server which is first with good enough utilities receives the request & executes it.
-
Consolidation Phase
Consolidating virtual machines into a small number of PSs is the aim of the consolidation phase. VM will be moved from server which is LIGHT to assist server to do this. Hence, the VM consolidation phase can only be occupied by HELP servers.
-
Migration Phase
The request messages from the HELP master is received by LLS master during a migration phase, indicating the quantity of amount of availability of CAR. Then the LLS
look for VM that will connect to an assistant master by the amount of earning of CAR. The moment LLS receives the required well suited VM, it will send the most important to an assistant first master, then will continue till the moment when there will be no well suited VM more.
After migrating VM all of the available, an LLS will enter the idle state; and then mode of sleep to conserve power. The consolidation phase algorithm have a time complexity as O(m), when m is no of VM on a server. Fig. 9 below shows the energy consumed comparison by number of servers in the particular information hub without and with optimization by LACE Algorithm[12].
Fig.9. Energy consumed in data center consisting of 1000 servers
-
-
Energy Prediction Model using CloudSim & JMetal
-
Modeled a cloud based datacenters with multiple hosts, VMs with varying hardware and power aware configurations using CloudSim library. The aim of the implementation was to optimize an influence aware datacenter by varying decisions of VM allocation, selection algorithms and hardware configurations. So as to seek out solutioning to the optimization problem we used JMetal. Not only aim to undertake and find a solution to multi- objective optimization problem during this domain, but also tried to match the outcomes of those algorithm for the given problem.
The consumption of energy and resources in big data centres is constantly increasing. Major challenges faced are in terms of energy and resource management, which affect computation, storage, and communication resources. Increased resource utilisation allows for the reduction of energy demand. Typically, such large-scale data centres are focused on the following four goals:
-
Enhancement of energy efficiency
-
Reduced execution time
-
Maximum resource utilisation
-
Make task scheduling more efficient.
-
RESULTS
The energy prediction model as explained just above in Section IV: sub-section 5, is implemented using Java Spring Boot Application to get optimized results. The results of energy prediction model without power optimization in Fig. 10 and with power optimization in Fig. 11 which are able to achieve above said four goals using CloudSim Simulator and JMetal are as follows:-
Fig.10. Instances on VM Servers before Power Consumption Optimization
Fig.11. Instances on VM Servers after Power Consumption Optimization
Table 2: Algorithm Ranking and Comparison of above stated Algorithms
-
CONCLUSION
As per the findings and literature survey from above journal papers as well as the implementation of one of the algorithms, the following conclusions are drawn as follows:-
-
Found some interesting answers to the original issue, which was about power consumption in cloud computing data hubs.
-
The best configuration for a cloud of this size indicates that a dual core setup with around 30 hosts and 30 VMs provides the best energy use which leads to streamline the power consumption in cloud data hubs.
-
IQR, LR (allocation policies), and MC (selection policy) were found to be the most power-aware algorithmic policies.
-
The datacenter was able to work with 6-7 kWh under these conditions, with SLA violations of less than 0.1 percent (ideal performance in 99.9 percent ).
-
This is great, and it demonstrates how power- aware systems can be used effectively across cloud infrastructures.
-
With big configurations, however, the results may differ. In terms of enterprise cloud applications, the findings are minor but not negligible.
-
Thus, there can be many algorithms such as self- consumption, LACE, renewable energy sources in green cloud computing, task scheduling algorithms, etc. which can streamline the power consumption leading to efficient utilization of cloud computing data centers.
REFERENCES
-
Camus Benjamin, Blavette Anne, Dufosse Fanny and Orgerie Anne-Cecile, Self-Consumption Optimization of Renewable Energy Production in Distributed Clouds, IEEE International Conference on Cluster Computing, pp. 2168-9253, IEEE, 2018.
-
Sahoo Sampa, Sahoo Bibhudatta and Turuk Ashok Kumar, An Energy-efficient Scheduling Framework for Cloud Using Learning Automata, 9th ICCCNT, July 10-12, 2018, IISC,Bengaluru, India, 2018.
-
Xu1 Pingping, Wu Guilu, Gu Zhifang and Wang Shujun, Joint Relay Selection and Power Allocation for Energy-limited Networks with Cloud Computing, 17th International Symposium on Distributed Computing and Applications for Business Engineering and Science, China, 2018.
-
Hasan Juglul, Haque Tanjim Ul, Hasan Sabab, Cloud-Based Automated Power Consumption Optimization, Power management, and Appliance Control, 1st International Conference on Advances in Science, Engineering and Robotics Technology, Dhaka, Bangladesh, 2019.
-
Kurdi Heba, Alismail Shaden and Hassan Mohammad Mehedi, LACE: A Locust-Inspired Scheduling Algorithm to Reduce Energy Consumption in Cloud Datacenters, King Saud University's Deanship of Scientific Research, IEEE, Volume 6, pp.2169-3536, 2018.
-
Lee, Choon Young, and Zomaya Albert Y., Energy efficient utilization of resources in cloud computing systems, The Journal of Supercomputing, pp. 268280, 2012.
-
Zhiming, Wang, , et al., Energy-aware and revenue-enhancing Combinatorial Scheduling in Virtualized of Cloud Datacenter, JCIT, pp. 62-70, 2012.
-
Yin P. -Y., Yu S. -S., Wang P. -P. and Wang Y. -T., A hybrid particle swarm optimization algorithm for optimal task assignment in distributed systems, Computer Standards & Interfaces, vol. 30, pp. 449-452, 2006.
-
Gao-wei Yan, Zhanju Hao, Novel Atmosphere Clouds Model Optimization Algorithm, International Conference on Computing, Measurement, Control and Sensor Network, ISBN, pp. 989-989, 2012.
-
E. Feller, L. Rilling, C. Morin, Energy-Aware Ant Colony Based Workload Placement in Clouds, 12th International Conference on Grid Computing, pp. 29-32, No. 7 in Grid 10, IEEE Computer Society, 2011.
-
S. Banerjee, I. Mukherjee, P. Mahanti, Cloud Computing initiative using modified ant colony framework, World Academy of Science, Engineering and Technology, pp. 222- 223, WASET, 2009.
-
A Beloglazov, J Abawajy, R Buyya, Energy-aware resource allocation heuristics for efficient management of data centers for cloud computing, Future Generation Computing System, pp.205- 209, vol. 3, 2011.