
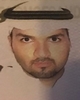
- Open Access
- Authors : Alaa Rashad Sindi , Mohammed Ahmed Balkhair
- Paper ID : IJERTV10IS070141
- Volume & Issue : Volume 10, Issue 07 (July 2021)
- Published (First Online): 29-07-2021
- ISSN (Online) : 2278-0181
- Publisher Name : IJERT
- License:
This work is licensed under a Creative Commons Attribution 4.0 International License
Shopping Trip Model for City Hosting Mega Events Case Study: City of Makkah, Kingdom of Saudi Arabia
Alaa Rashad Sindi
Civil and Environmental Engineering Department King Abdulaziz University
Jeddah, Saudi Arabia
Mohammed Ahmed Balkhair Holy Mosque Expansion Projects Makkah Saudi Arabia
AbstractMakkah city attracts millions of visitors throughout the year and expected to attract 30 million annually in 2030. Some residents take on more jobs during the high- demand seasons, and others avoid the congestion. Visitors also conduct other activities such as shopping and recreational pastimes, which affect the amount and type of activities conducted by the residents and the economical status of the city. Transportation studies tend to model residents travel behavior independently of visitors during various seasons of the year as each group has different constraints and lifestyles. Thus, there is a need to develop a robust transportation demand model that is able to predict future travel conditions to assist in making effective decisions regarding the transportation infrastructure. Developing this model will require years of work. This paper is focused on the first step of the travel demand model, which is trip generation or, more specifically, trip production for shopping activities for the residents of Makkah. In the highly densely populated urban area, shopping activity is one factor that motivates economic development and has a significant impact on the transportation network. Shopping activity is more difficult to model compared to work activity because it varies in demand from one time to another, unlike work trips. A household travel survey conducted by Makkah Municipality in 2010 was used to develop a trip production model for residents from various zones of the city using linear regression method during off peak seasons. Data analysis, carried out using the SPSS software, identified only social factors affect trip production for shopping activities. Data on these factors are collected by the General Authority for Statistics in Saudi Arabia every five years and could be inputted to the model to predict, in simple terms, the residents shopping trips.
KeywordsTrip production, Regression model, Shopping trips, City hosting mega events
-
INTRODUCTION
The four-stage sequential method has been the basis for the analysis of travel demand and travel demand forecasting since the 1950s (Habib, 2007). It consists of trip generation, trip distribution, model split, and traffic assignment. The modeling framework uses individuals sociodemographic information such as household income, employment status, and age. It also uses trips and zoning information such as the area of a zone, travel time, travel distance, and transportation network information. These data are then inputted to the trip generation model to estimate the number of an individuals, households, or vehicles trips. The output of the first stage is inputted into the second, the output of the second stage is inputted into the third, and so on to estimate the number of vehicles in a transportation network. This process is conducted
for various times of the day (peak and off-peak periods), and the total is the daily travel demand of the city. The estimated numbers of vehicles trips during the day or during a specific period are then validated against the actual number of trips to check the accuracy of the model. This results in a tool to predict the travel demand, considering changes in the individuals sociodemographic and infrastructure characteristics. The size of the transportation network and the nature of the city define the complexity of the estimated model (William & Mcguckin, 1998; Garber & Hoel, 2015).
One of the most complex cities around the world in terms of transportation demand modeling and management is the city of Makkah, located in the western part of the Kingdom of Saudi Arabia. The city has various seasons of the year where travel demand varies significantly, which are Ramadan, Umrah, Friday, and Hajj (Sindi, 2017). Annually, millions visit the city with different backgrounds, ethnicities, education levels, languages, and lifestyles, resulting in various combinations of travel demand. Therefore, each group of people during a specific season could be modeled separately to accurately capture their travel behavior. The visitors visit the shopping centers and historic places, and eat at restaurants. As a result, many of shopping places and services are constructed to meet the high demand during the mega-events. The residents adjusts their travel patterns according to the activity of the visitors. Some prefer to stay at home during the high-demand seasons, while others try to volunteer or get an additional paid job. This makes it complicated to model and predict the residents travel demands (Sindi, 2017). During the off-peak season when there are no visitors many shopping places are available to the residents to purchase their merchandizes from. In other words, the shopping supply side is much higher than the demand.
There is a lack of studies related to quantifying and modeling travel demand in the cities holding mega events such as the city of Makkah. The rapid population and economical growth has led to considerable increases in trip generation in the city. The conventional four analytical steps are an adequate method of planning for Makkah. To provide a strong basis for the transportation planning process, this paper is focused on the trip production part of the trip generation stage because this is the first step in the four-stage modeling framework.
Makkah Municipality collected a household travel survey for the residents of Makkah during the off-peak season (the
second and third months of the lunar calendar) in 2010. This data set is adequate to model the residents travel behavior during the off-peak season considering various trip purposes. Researchers around the globe focus on work activities because these are the base activities for modernization. Shopping activity is a sign of economic development in the city. However, it does not gain great attention in many studies in terms of modeling because its travel demand is lower than that for work activity. Most shopping trips conducted in the city of Makkah are causing an evening peak period; thus, important to model. The rapid economical growth of the city as it holds annual mega events provide the residents with variety of shopping options and affect the residents socio economic status.
The aim of this research is to properly divide the city into zones that aid in studying the key factors affecting the trip generation model for shopping activity in a diverse city such as Makkah and to develop trip production models for all zones of the city that enable the planners to predict the shopping trips.
-
LITERATURE REVIEW
The Institute of Transportation Engineers (ITE) trip creation guide is widely used in the United States of America (USA) to predict trip generation. ITE has produced trip generation rates for many land-specific uses based on over three thousand trip generation studies (ITE, 2020). Different cities have different characteristics and need case-specific models. The following are examples of trip generation models developed for various cities.
Taher, and Al-Taei (2006) developed a trip attraction model for the residential area of Dohuk in Iraq in 2006. The study covered twenty residential areas out of the twenty-eight traffic zones located in the urban area of Duhok. The attraction trips were categorized into seven types. Independent variables such as number of mployments and housing units were used to predict the number of trips using SPSS software. The results show that home-based work trips have consistently good R2 value, whereas Home-Based Other and home-based shopping show weak relationships with independent variables such as the number of retail sales in the Central Business District (CBD).
Peska and Venter (2009) examined independent variables that may have affected the generation of residential trips in high- and middle-income areas in 2009. The independent variables selected in the study were:
-
The number of bedrooms or the units size in square meters.
-
The development density (units per hectare).
-
The development size (number of units).
Surveys on trip generation were also conducted in fifty- five locations in Johannesburg and Ekurhuleni. The development density is the key factor that best forecasts trip rates (Peska and Venter, 2009).
Anderson and Malave (2003) explored the possibility of developing a dynamic trip generation model, for a medium- sized urban society, that provides time as a measure in the US-
based trip modeling process to support ITS-based decisions and incident management. The study includes the results of the validation study conducted on the model, with the authors concluding that the models developed in this effort are highly capable of providing dynamic trip generation data.
Broadstock et al., (2010) built a model to generate trips for a cross section of residential developments across the United Kingdom. The pilot model tested whether trip-making patterns for residential developments were independent of vehicle ownership. The result was that trip generation depended on vehicle ownership, economic and social factors, and site- specific characteristics, particularly the type of land area. Nevertheless, the public transport services are not found to have a great correlation with the trip generation.
Golob (1989) developed a model of causal correlation at the family level among car ownership, income, and trip generation. The study was based on data obtained from the Dutch National Navigation Board, which consisted of about 1,800 families. The researcher found that vehicle ownership directly affects public transport journeys with additional income effects.
As shown in the aforementioned studies, a range of demographic and social variables affect trip generation. The basic tool used to create trip creation models is regression analysis. Two kinds of regression are commonly used. The first uses data disaggregated at the household or individual level, with the number of trips by a family or individual as a dependent variable and the household and personal characteristics as explanatory variables. The second uses aggregate data at the district level, with the average number of trips per household in the region as a dependent variable and the average area characteristics as explanatory variables. Both approaches will be tested in this paper.
-
-
DATA
The Makkah Municipality conducted a survey of 5,299 households that targeted the residents of the city (1.5% of total population) in the third and fourth lunar months of 2010. The total number of respondents to the questionnaire was 23,242 persons from the city population (1,549,774 capita). The definition of household did not necessarily mean a family because any number of people living in the same house was called a household. In other words, if a single person lived in a house on their own, they would be called a household. The interviews were carried out with household members who were eight years old of age or older, who also spent at least three nights a week in that house. Upon the initiation of the interviews, the interviewer sought permission to conduct the interview from the head of the household (usually the oldest male in the house). After that, the interviewer explained the survey to the participants and identified the household by address. Next, all eligible participants were interviewed according to a fixed set of questions asked in a certain preset order. Some careful measures were taken by the interviewer in terms of assuring the completion and maximum clarity of the answers before leaving the household. At the end, an identification number was given to each eligible member of
the family for organizational and analytical purposes (Sindi, 2017).
Three topics were covered by the interview questions: household, members, and trips. The household questions were concerned with socioeconomic status and other general information (e.g., number of household members, number of cars owned, and the salaries of working members). The household members-related questions targeted areas such as their age, occupation, and place of work and whether they owned a drivers license. The trip-related questions sought information concerning the chain of trips carried out by each member of the household a day before the interview (e.g., start and end time of each trip in the chain, origin and destination, mode of transportation used, number of people in the car, and purpose of the trip). The origin of the second trip in the trip chain was defined as the destination of the first. The GIS model of the city contains zone sizes, population, and the facilities in each zone (Sindi et al,. 2016, 2017 and 2018).
The percentage of males represented 53.7% and the percentage of females represented 46.3% of the study sample. The number of family members was classified into eight categories. Most numbers of households contained four members, accounting for 17.7% of the total sample. The age of family members was classified into six categories. The younger age groups (under twenty years), accounting for 39%, were the majority of the population of all age groups, and age groups regularly decreased as age increased. Most of the sample was at the secondary level, meaning that the model was expected to be reflective of this education-level group (24.9% were in secondary school, 23.5% were not educated, 19.1% were in preschool, 16% were in primary school, 15.5% were studying at university, and 1% had a master or PhD degree). Writers accounted for 10.3% of the sample (meaning people who work in the educational sector), followed by 5.9% working in services, and 2.9% who were specialists in science, art, and humanity studies. The rest were divided between traders, businesspeople, drivers, and other professions. We found that for most HHS, their income was between 3000 and 7000 SAR, which represented 42% of the sample size. Then, 33% of households had incomes of less than 3000 SAR, 22% had incomes of more than 7000 SAR, and 3% gave no response.
Categorical variables in this study included household income, gender, nationality, education level, work status, acquisition of a drivers license, and profession (see Table I). The category of household income was that received per month in Saudi Riyal (Table II). Age was recategorized into six groups (Table III).
TABLE I. CATEGORICAL VARIABLES FROM MAKKAH DATASET
TABLE II. HOUSEHOLD INCOME CATEGORIES
TABLE III. AGE CATEGORIES
-
RESULTS
The center of the city has the oldest landmark of the city which is the Al-Haram. The City then formed its transportation system around the Al-Haram such that its circular roads around it and radial roads from it. The city has 949 TAZ; however, the study area was rearranged and divided into twenty-three TAZ based on the intersection of the circular and radial roads as some zones has limited number of data points (i.e. not enough data to estimate the model). Based on the GIS model, Table IV shows sample size, population, and area of study. The sum of zone areas was 1,111.26 km², which means the population density is 1,394.61 residents/km². Most of the population are located in the center of the city and decrease as located away from the center of the city.
TABLE IV. ZONE AREA, POPULATION SIZE, SAMPLE SIZES, AND POPULATION DENSITY PER TRAFFIC ANALYSIS ZONE FOR STUDY AREA
p>Fig. 1. Traffic Analysis Zones (TAZ) of MakkahZoning merge
Samples were randomly selected from each zone. The sample size for each TAZ is shown in Table V and Figure 2.
TABLE V. NUMBER OF HHS SAMPLE PER TAZ
Fig. 2. Percentages of population for each zone
The questionnaire used by Makkah Municipality was aimed at collecting individuals and families characteristics. These variables were used to construct trip production models, which will be examined statistically and logically to adopt the most appropriate model that can predict the trips produced by the TAZ.
The questionnaire contains two tables. The first table gives details about the socioeconomic variables of households and their individuals. These details include: household member education level, age, possession of drivers license, ownership of private cars, type of house (house or apartment), and family income. The explanatory variables considered for potential use in the modeling process are summarized in Table VI.
TABLE VI. HOUSEHOLDS (HHS) EXPLANATORY VARIABLES
The second part of the survey was to gather trip data. Table VII calculates the total number of trips undertaken by the family and the number of trips per type (work trips, education trips, shopping trips, social trips, leisure trips, and other trips such as hospital, barbershop, or campus trips), as well as the number of trips for a specific time period. These trips are divided into seven groups, which were developed for model testing. The first group includes trips from 12:00 a.m. to 6:00 a.m. The second group includes trips between 6:00
a.m. and 8:00 a.m., which is usually the morning peak period when most students and some employees make their trips. The third and fourth periods include trips between 8:00 a.m. and 12:00 p.m., which is outside the peak period, and 12:00 p.m. and 2:00 p.m., respectively. Group 5 includes trips occurring from 2:00 p.m. and 6:00 p.m., which is the evening peak period. Groups 6 and 7 are from 6:00 p.m. to 9:00 p.m. and 9:00 p.m. to 12:00 a.m., respectively. Usually, most shopping, social, and leisure trips are made after 6:00 p.m.. All these trips are summarized as shown in Table VII.
TABLE VII. TRIPS DATA
Models of multiple linear regression of trip generation were developed using the SPSS software version 25. The best and most commonly utilized approach to allocating parameters of the prediction model is the stepwise approach (Al-Hasani, 2010). A confidence level of 95% was considered when deciding to accept or reject the independent variables. Un like disaggregate model, aggregate model variables were statistically insignificant. The structure of a household does not affect shopping trips. In other words, households depend on members of the household in making shopping trips. Only males of 18 years and older are allowed to drive; thus, females depend on male to conduct shopping trips.
Total trips made by all households selected were 44,156 trips for all purposes, and only 2464 trips made were shopping trips. In other words, 5.58% of all-purpose trips made were shopping trips. 2089 of them were made during weekdays and the rest of 375 trips were made in the weekend days. In other words, 85% of shopping trips were accomplished during weekdays. This observation brings up a question to define what a shopping trip in Makkah really is, and how the situation of shopping in Makkah 2010 looked like. Based on the result observed from data conducted, people living in the city had different schedules and priorities in the weekends than shopping. shopping trips were, prioritized number four after work, education, and Haram visits. The residents tended
to go to Haram or visiting families or traveling to nearby cities (Jeddah, Taif) during the weekends instead of shopping. Regression model were estimated for shopping during the weekends, but the variables were statistically in significant which comply with the data. As a result, trips data used to develop the model were confined only for shopping trips during weekdays.
This paper computes regression models for shopping trips produced from all zones of the city. The forward regression models are provided in Table VIII. The area of zones, population size in each zone and population density were not significant. The top three repeated variables among various models of all 23 zones were age (4049), education (secondary), and profession (writer). Therefore, these variables were selected as key variables among the others (see Table VIII). Secondary students are at the age when they are starting to drive and own vehicles. Usually, they do all the driving tasks for the family as it is a new experience for them. Those aged between forty and forty-nine make fewer shopping trips compared to other groups. Usually, this age group are the head of the family who work to support the family and have limited time for other activities. The head of the family distribute other shores to family other members such as secondary level students members who conduct more shopping activities. The residents who work in the education sector conduct more shopping trips than any other profession such that they provide material for their class during the terms. In addition, residents who work in education sector are the highest in number compared to other sectors in the city.
Zone one in particular has a constant value of 1.55, which is the highest among other. This indicates that most of shopping trips were generated from this zone, where includes the Al-Haram, Ajyad, and Jarwal districts which are the city center. This brings a potential of having multi-purpose trips. For example, Haram trip could be extended to include shopping activities. This situation, however, is identify using the traditional travel demand model. The farther zone from the center the more shopping trips generated by writers where the new and big shopping centres are located. Secondary-level students make the highest shopping trips in zone 17 were the large shopping malls located. Residents of age 40-49 in zone 19 engage less in shopping trips than others. They live far away from the city center and central business district and expected to spend more time in working and commuting. As results, less time spent for shopping. Zone 22 is reserved for the mega event (i.e. Hajj) and has no residential area; thus, no shopping trips are generated from this zone.
TABLE VIII. FORWARD REGRESSION RESULTS FOR SHOPPING TRIPS YS
X1: Age (4049)
X2: Secondary-level student
X3: Writer (works in education sector)
Model verification tests the ability of the model to predict future behavior. Of the data, 90% are used to estimate the model, and 10% are used for verification because there is no other set of data. The results show that the estimated model accurately predicts shopping trips (see Table IX). In average, the model predictability is 81% which is considered good given the nature of the inconsistent shopping trips.
TABLE IX. HBS WEEKDAY TRIP GENERATION MODEL VERIFICATION
-
CONCLUSION
Makkah city attracts millions annually and is expected to host thirty million pilgrims by 2030 as it is the vision of the Kingdome of Saudi Arabia. Thus, there is a need for a travel demand model that is capable of predicting travel demand accurately during various seasons of the year that aid in
planning of the city. The basis of the analysis of travel demand is the four-stage sequential model. This paper contributes to the city travel demand model by developing a trip generation model for shopping trips that explains the residents behavior in conducting this type of activity. The city of Makkah collected a household travel survey for residents in the second and third months of the lunar calendar in 2010. It contains individuals, household, and trip information. This data set was cleaned and organized to model shopping trips. The data were analyzed using SPSS software. The analysis provided an overview of the demographical distribution of the resident. The data presented that the majority of the population is of the young generation and that most families range between three and four per household. The city has 949 TAZ. The number of data points in some zones is not adequate to develop a regression model, and the number of data points available is sensitive in the model estimation process. Therefore, these 949 zones were merged to twenty-three based on the intersection of the major radial and circular roads of the city. This zoning system will help in estimating travel demand on the major roads of the city.
The household-level shopping trips were insignificant; thus, individual-level shopping trips were tested. Individual- level shopping trips resulted in a better model in terms of coefficients of determination. Individual information is always statistically significant, unlike household information. Various models were tested using various market segmentation, including working-days, weekends, and all-days models. The model that resulted in the highest goodness of fit was the working-days model. This agrees with the high number of shopping trips generated during the working days compared to the weekends. During the process of model development, all variables were tested. The results show that the three variables that were repeated regularly in most of the models developed for each zone were age, profession, and education level; thus, the final model included only these three variables. The coefficients sign and the statistical test were sound. These variables are collected every five years by the state of Saudi Arabia, which makes the model easy to use in the future (i.e., no data collection effort by individuals is needed). Zone 1 is in a high-density mixed land-use development next to the city center. This zone, which includes Haram, Ajyad, and Jarwal, has the highest constant value. This indicates that most shopping trips are conducted in these areas. Zone 22 does not generate trips because it is the Al-Masheer zone (i.e. Mega- events are), which does not have any residential areas during the off seasons. The model was developed based on 90% of the data, while 10% was used for validation. Results show that the model predictability accuracy was, on average, 81%, which is considered good for shopping trips. Unlike other trip generation models, this model depends on individual information to predict shopping trips. This reflects the unique travel behavior of the residents of Makkah such as generated shopping trips are based on individuals characteristics. Additional set of data of various years could be used to better validate the model and to develop a model that takes into account the annual increase in travel demand. The model was developed for the nonseason period and thus could be used in other Saudi cities of similar size that share the same zone characteristics such as Al-Madinah city. In 2010, females
depended on males to conduct trips, but more recently, females have been allowed to drive. Gender was not one of the key factors, and neither was the number of drivers licenses, although females depended on males to make shopping trips. Thus, gender and acquisition of driver licenses are not affecting the number of shopping trips in the city of Makkah. This research is the first stage in developing the traditional four-stage travel demand model. Similar models could be developed for other sets of activities such as work, and school trips.
REFERENCES
-
Al-Hasani S.S.F., (2010). Modeling Household Trip Generation at Descret Zone Baghdad City. MSc. thesis, Engineering College, University of Baghdad.
-
Anderson, M. D., & Malave, D. N. (2003). Dynamic trip generation for a medium-sized urban community. Transportation research record, 1858(1), 118-123.
-
Broadstock, D. C., Collins, A., & Hunt, L. C. (2010). Modelling car trip generations for UK residential developments using data from TRICS. Transportation planning and technology, 33(8), 671-678.
-
Garber, N. J., & Hoel, L. A. (2009). Transportation Planning. In Traffic and Highway Engineering (4th ed., pp. 551-620). Toronto, ON: Cengage Learning.
-
Golob, T. F. (1989). The causal influences of income and car ownership on trip generation by mode. Journal of Transport Economics and Policy, 141-162.
-
Habib, K. (2007). Modelling activity generation processes. Ph.D. dissertation University of Toronto, Toronto. 2007.
-
Institute of Transportation Engineers (ITE) (2020). Trip Generation, 10th Edition. https://www.ite.org/technical-resources/topics/trip-and- parking-generation/trip-generation-10th-edition-formats/
-
Peska, P., & Venter, C. J. (2009). A relook at residential trip generation variables. SATC 2009.
-
Rahman, R. A. (2009). Modelling of Trip Generation Based on School Attraction. Universiti Teknologi Malaysia.
-
Sindi, A., Easa, S., Abd El Halim, A., (2016). Time expenditure differences among Weekdays, Weekends and Fridays for the Residents of Makkah, Kingdom of Saudi Arabia. Presented at the Canadian Society for Civil Engineering (CSCE), London, Ontario, Canada, June 1-4, 2016.
-
Sindi, A., Abd El Halim, A., & Easa, S. (2017). Evaluation of Store Closing Policy for the City of Makkah, Kingdom of Saudi Arabia. International Journal of Service Science, Management and Engineering. Vol. 4, No. 4, 2017, pp. 31-37.
-
Sindi, A. (2017). Improved Activity-Based Model for Mega-Events. Ph.D. dissertation Carleton university, Ottawa, Ontario.
-
Sindi, A., Easa, A., & Abd El Halim, A. (2018). Improved multiple discreet-continuous extreme value model. Canadian Journal of Civil Engineering, 2018, 45(12): 1040-1052, https://doi.org/10.1139/cjce- 2017-0186
-
Taher, A. M., & Al-Taei, A. K. (2006). Trip attraction development statistical model in dohuk city residential area. AL Rafdain Engineering Journal, 14(2), 12-23.
-
Martin, W. A., & Nancy A. M. (1998). Travel Estimation Techniques for Urban Planning (Report 365). NATIONAL COOPERATIVE HIGHWAY RESEARCH PROGRAM.