
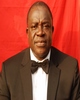
- Open Access
- Authors : Idachaba Alexander Ogacheko , Idoniboyeobu Dikio
- Paper ID : IJERTV10IS030181
- Volume & Issue : Volume 10, Issue 03 (March 2021)
- Published (First Online): 27-03-2021
- ISSN (Online) : 2278-0181
- Publisher Name : IJERT
- License:
This work is licensed under a Creative Commons Attribution 4.0 International License
Sensitivity Analysis of A Predictive Quadratic Line Voltage Stability Index for Power Systems Network Voltage Collapse Studies
Idachaba Alexander Ogacheko1, Idoniboyeobu Dikio2
1(Department of Electrical Engineering/ Rivers State University, Port Harcourt, Rivers State, Nigeria)
2(Department of Electrical Engineering/ Rivers State University, Port Harcourt, Rivers State, Nigeria)
Abstract : Voltage Stability Margins (VSM) plays a crucial role in modern power systems by ensuring that power system networks do not operate too close to their stability limits. In this paper, we study the sensitivity of three parameters of a Predictive Quadratic Line Voltage Stability Index (pq-LVSI), the collapse detection threshold (cdt) factor, the degree of Confidence (doC), and alpha parameters. Sensitivity studies are tools applied to evaluate the possible predicted states of the pq-LVSI and determine the extent of their influence over these predicted states.
Keywords LVSI, Predictions, Power System Network, Voltage Collapse, Transmission Lines
-
INTRODUCTION
Nigeria and the world's developing economies are often confronted with the challenge of ensuring the supply of adequate and reliable electricity to a teeming populace comprising industrial and domestic consumers, particularly in the urban areas. However, exponential urban growth due to situation of rural-urban migration and the evolution of new lines of power- hungry businesses due to a diversification of electrical equipment or loads continue to rise at an alarming rate; this has further led to the situation of voltage instability evidence by voltage dips and more commonly frequent blackouts which is due to the phenomenon generally referred to as voltage collapse.
Voltage collapse can hamper power system operations by denying the consumers a constant and reliable source of electricity (Idoniyeobu et al., 2018; Idachaba, 2018).
To study this effect, the Voltage Stability Index (VSI) in (Ratra et al., 2018), Line Collapse Proximity Index (LCPI) in Tiwari et al. (2012), power line stability index (L-index) in equivalent node voltage collapse index (ENVCI) in Wang et al. (2009), Voltage Margin Index (VMI) based on Local Impedance Matching (LMI) technique as proposed in (Toban V et al., 2014), reactive power loss index (RPLI) in Moger & Dhadbanjan (2015).
Furthermore, in a scenario-based negotiation via Tap Agents, an index was developed to eliminate bus voltage violations that may occur amongst Bus Agents (BA) in a power system network through a priority based allocation of Reactive Agents (RA) across the BA's (Nassaj & Shahrtash, 2018). In agent processing, Tap Agent and BA's are part of a larger agent system with intelligent and reactive capabilities.
The use of approximate determinants for computing voltage stability index in power distribution networks was proposed in (Aolaritei et al., 2017). Also, a statistical method for online voltage collapse proximity index (VCPI) estimates has been proposed in (Sanz et al., 2016). In this VCPI model, a database is used for storing a history of power system loading and contingencies. Their model was applied to the IEEE 14-bus system and an equivalent Mexican 190-bus system.
Further works in this area of voltage collapse indexing can be found in the review by Modarresi et al. (2016) and in Idachaba (2018).
In this paper, we present once again the performance of a novel approach called the Predictive Quadratic Line Voltage Stability Index (pq-LVSI) that was proposed earlier in (Idoniyeobu et al., 2018; Idachaba, 2018) and used to estimate voltage collapse prone regions in the Nigerian 330kV 30-bus power system transmission network. We also study through sensitivity analysis the influence of certain associated system parameters on the prediction performance of pq-LVSI in a simulation-based environment.
-
METHODOLOGY
-
Parameters for Sensitivity Analysis
The technique for sensitivity analysis of the proposed predictive quadratic Line Voltage Stability Index (pq-LVSI) proposed in (Idoniyeobu et al., 2018) includes a determination of influential (primary) system parameters that affect pq-LVSI performing, including the computed LVSI and its corresponding moving average predictions for the buses under consideration. The primary parameters have to be identified from the set of LVSI parameters and after a number of trial runs. This is made possible by running a load flow program on a given dataset, then using the generated bus voltage-phase-angle as input to a pq-LVSI program.
The parameters for sensitivity studies are defined in the sub-sections that follow, while the default values are given in Table 1.
-
Collapse detection threshold (cdt)
This parameter is used to estimate the index of the computed LVSI probabilities by conditioning to a pre-specified value prior to confidence testing for each bus and for the specified number of trial runs. When the LVSI index exceeds this value, it signals a Boolean value of 1 otherwise, a Boolean value of 0 is signaled.
-
Degree of Confidence (doC)
This parameter is used for confidence testing and for estimating the mean state of the conditioned LVSI's by conditioning to a pre-specified value for each bus and for the specified number of trial runs. Using the doC, it is possible to finalize the desired expectations from the initial process of probabilistic reasoning for both the standard LVSI estimate and the moving average LVSI estimates.
-
alpha
This parameter is used to fine-tune moving averages using different processing functions or operations such as simple, square- root, exponential, etc. It has two associated parameters: the lead, which defines the number of steps to look-ahead, and a lag that defines the number of steps to make a look-back during moving average estimates. In this way, moving averages become predictors.
TABLE 1. Default values of the pq-LVSI
Parameter
Default value used
Collapse detection threshold (cdt)
1.5
Degree of Confidence (doC)
0.7
alpha
1
-
-
Load flow Analysis
Load flow analysis (LFA) is a necessary requirement to solve most power system bus networks. For the LVSI processing, its operation is as shown in the flow diagram of Fig 1. The steps are generally set out as follows:
-
First, the system bus parameters such as line impedances, bus generation real and reactive (complex) powers, initial bus voltage/angle guesses, and the NR parameters such as the number of simulation runs and stopping criterion are all specified and entered into a simulation file.
-
Then a simulation operation is performed for a stipulated number of trial runs
-
Finally, the actual bus voltage, phase angles, and line flows are computed and stored once the simulation completes.
Fig.1: Flow diagram for LVSI calculation/prediction scheme (Source: Ratra et al., 2018).
-
-
Predictive Quadratic Line Voltage Stability Index (pq-VLSI)
Predictive Quadratic Line Voltage Stability Index (pq-LVSI) is a Voltage Collapse Metric (VCM) developed earlier in (Idachaba 2018). It uses the ABCD transmission line parameters for estimating the voltage stability margins (VSMs) of a power system network (Ratra et al., 2018).
A pq-LVSI may be computed by following the steps below (Idoniyeobu et al., 2018; Idachba, 2018):
[Step].1 Define the required bus voltages (sending and receiving end voltages) and the corresponding phase angles; also define the transmission line parameter, including the ABCD model descriptors for the considered lines. [Step].2 Model a phase angle as a difference between a sending and receiving end voltage of a given line. [Step].3 Model the receiving end active power in terms of the ABCD parameters. [Step].4 Form a quadratic equation from the computed model in Step 3. [Step].5 Compute the sensitivity of the receiving end voltage as a differential change with respect to received power. [Step].6 Estimate voltage stability requirements. [Step].7 Estimate the voltage collapse avoidance satisfiability criterion [Step].8 Compute the LVSI from Steps 6 & 7. [Step].9 Compute LVSI probabilities. [Step].10 Compute the degree of Confidence (doC) voltage collapse estimate. -
-
EXPERIMENTAL DETAILS AND RESULTS
We have performed the sensitivity studies using code developed in the MATLAB programming language and using Voltage- Phase angle data after a Newton-Raphson (NR) load flow of the Nigerian 330-kV power system bus and transmission line; the data can be obtained from the National Control Centre (NCC) Osogbo, Nigeria.
-
Sensitivity Analysis Task
The task is to determine the most influential parameter and predictor of the proposed pq-LVSI. We vary the associated LVSI parameter default values in the same incremental order to avoid bias. The solution values used (bus voltages and angles) after an NR-load flow is as shown in Table 2.
TABLE 2. Voltage and phase angles for considered lines
Bus no.
Bus Name
Voltage (p.u)
Angle (rad)
Description
1
Egbin-GS
1.0000
0.0000
Gen bus
5
AFAM-GS
1.0000
0.3043
Gen bus
8
SHIRORO-PS
1.0000
-0.5057
Gen bus
12
IKEJA-WEST
0.9930
-0.0961
Load bus
26
ALAOJI
0.9564
0.2707
Load bus
29
KADUNA
0.8738
-0.6941
Load bus
-
Experimental Results of the Sensitivity analysis by varying the cdt parameter
The results of adjusting the doC parameter are as shown in Table4. In these experiments, the other two parameters are kept at default values while the cdt parameter is incrementally adjusted from a value of 1.4 to 1.7 in steps of 0.1 units; then, the pq- LVSI code is simulated for bus line sequence b1-b12, b5-b26, and b8-b29.
TABLE 3. Voltage and phase angles for considered lines
cdt = 1.4
cdt = 1.5
cdt = 1.6
cdt = 1.7
Line Sequence
pq_n
pq_sh
pq_lg
pq_n
pq_sh
pq_lg
pq_n
pq_sh
pq_lg
pq_n
pq_sh
pq_lg
b1-b12
1
1
1
1
1
1
1
1
1
1
1
1
b5-b26
1
1
1
1
1
1
1
1
0
1
1
0
b8-b29
1
1
1
1
1
0
1
1
0
1
1
0
-
Experimental Results of the Sensitivity analysis by varying the doC parameter
The results of adjusting the doC parameter are as shown in Table4. In these experiments, the other two parameters are kept at default values while the doC parameter is incrementally adjusted from a value of 0.5 to 0.8 in steps of 0.1 units; then, the pq- LVSI code is simulated for bus line sequence b1-b12, b5-b26, and b8-b29.
TABLE 4. Voltage Collapse Prediction for Varying doC Values
doC = 0.5
doC = 0.6
doC = 0.7
doC = 0.8
Line Sequence
pq_n
pq_sh
pq_lg
pq_n
pq_sh
pq_lg
pq_n
pq_sh
pq_lg
pq_n
pq_sh
pq_lg
b1-b12
1
1
1
1
1
1
1
1
1
1
0
0
b5-b26
1
1
1
1
1
1
1
1
1
1
0
0
b8-b29
1
1
1
1
1
0
1
1
0
1
0
0
-
Experimental Results of the Sensitivity analysis by varying the alpha parameter
The results of adjusting the doC parameter are as shown in Table5. In these experiments, the other two parameters are kept at default values while the alpha parameter is incrementally adjusted from a value of 0.7 to 1.0 in steps of 0.1 units; then, the pq- LVSI code is simulated for bus line sequence b1-b12, b5-b26, and b8-b29.
TABLE 5. Voltage Collapse Prediction for Varying alpha Values
alpha = 0.7
alpha = 0.8
alpha = 0.9
alpha = 1.0
Line Sequence
pq_n
pq_sh
pq_lg
pq_n
pq_sh
pq_lg
pq_n
pq_sh
pq_lg
pq_n
pq_sh
pq_lg
b1-b12
1
1
1
1
1
1
1
1
1
1
1
1
b5-b26
1
1
1
1
1
1
1
1
1
1
1
1
b8-b29
1
1
0
1
1
0
1
1
0
1
1
0
-
-
DISCUSSIONS
The results of simulation experiments show that both moving average long (mov-avg-long) and moving average short (mov- avg-short) predictions are sensitive to a higher cdt and doC values than default value; however, the cdt is most sensitive with at most 3 indications in 3 cdt simulation trial values and having two of its mov-avg-long predictions indexing a collapse (0) signal at the 3rd cdt trial value. The results also show that the moving average long (mov-avg-long) prediction is most sensitive to wide variations of the considered pq-LVSI parameters. In particular, the pq-LVSI technique with moving average filter prediction- long is indicative of the true predictive state of a moving average based pq-LVSI.
-
CONCLUSIONS AND FUTURE WORK
In this paper, we have performed sensitivity analysis using several parameters of the proposed pq-LVSI technique. We have shown through several simulations that the key influential predictor is the moving average long (mov-avg-long) and the influential parameter. Further simulations may be necessary to identify any subtle change in stability index signals. Also, this model will be more useful if it is verified using different power system network benchmarks such as the IEEE-power system bus networks. Future work should focus on adapting more predictive but simple neural-inspired intelligent agents to the proposed statistical predictive model for increased reliability.
-
REFERENCES
-
Aolaritei, L., Bolognani, S., & Dörfler, F. A distributed voltage stability margin for power distribution networks. IFAC-Papers On-Line, 50(1), 2017, 13240-13245.
-
Idachaba, A. O. Voltage Collapse Evaluation and Prediction in the Nigeria 30 Bus 330kV Power Network (Moving Average Method) MSc.
Dissertation, Rivers State University, Port Harcourt. 2018
-
Idoniboyeobu, D., Braide, S. L., & Idachaba, A. O. Analysis of Voltage Collapse in the Nigeria 30 Bus 330kv Power Network. IOSR Journal of Electrical and Electronics Engineering 13(4), 2018, 42-50
-
Modarresi, J., Gholipour, E., & Khodabakhshian, A. A comprehensive review of the voltage stability indices. Renewable and Sustainable Energy Reviews, 63, 2016, 1-12.
-
Moger, T., & Dhadbanjan, T. A novel index for identification of weak nodes for reactive compensation to improve voltage stability. IET Generation, Transmission & Distribution, 9(14), 2015, 1826-1834.
-
Nassaj, A., & Shahrtash, S. M. A predictive agent-based scheme for post-disturbance voltage control. International Journal of Electrical Power & Energy Systems, 98, 2018, 189-198.
-
Ratra, S., Tiwari, R., & Niazi, K. R. Voltage stability assessment in power systems using line voltage stability index. Computers & Electrical Engineering, 70, 2018, 199-211.
-
Sanz, F. A., Ramirez, J. M., & Posada, J. Statistical method for online voltage collapse proximity estimation. International Journal of Electrical Power & Energy Systems, 82, 2016, 392-399.
-
TOBON, J. E., CORREA GUTIERREZ, R. E., & RAMIREZ, J. M. Voltage collapse detection based on local measurements. Electric power systems research, 107, 2014, 77-84.
-
Wang, Y., Li, W., & Lu, J. A new node voltage stability index based on local voltage phasors. Electric Power Systems Research, 79(1), 2009, 265- 271.