
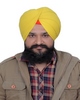
- Open Access
- Authors : Gurmail Singh , Amit Dutt
- Paper ID : IJERTV9IS090421
- Volume & Issue : Volume 09, Issue 09 (September 2020)
- Published (First Online): 29-09-2020
- ISSN (Online) : 2278-0181
- Publisher Name : IJERT
- License:
This work is licensed under a Creative Commons Attribution 4.0 International License
Relationship Between Various Key Performance Indicators of Supply Chain and Net Sales
Gurmail Singh
Phd scholar, Mittal School of Business, Lovely Professional University, Phagwara, India.
Amit Dutt
Professor and Associate Dean , Lovely Professional University, Phagwara,
India .
Abstract: Since 1990, extensive studies and analyses have conducted in the area of supply chain performance management. Cost and non-cost viewpoints are various supply chain overview measures; political, tactical or operational focus; business process opportunities, and economic point of view standardized measuring systems used as a tool for efficient supply chains to accomplish their functional goals. An empirical study has performed with some of the most commonly discussed organizational performance mechanisms, and it demonstrates that several of the evaluation system 's validity must also be examined. Because of the sophistication of such processes, the method of determining effective supply chain performance metrics is challenging. The resource skills required are, therefore, often challenging for individual organizations to acquire and maintain, so sound decision-making is crucial in supply chain management. This regression analysis helps consumers to predict and fulfill potential needs and standards. The paper explores the connection between the main determinants of performance and total income in the supply chain. It also explores the connection between logistics spending and net sales, which provide a forum for successful decision- making. The findings presented in the paper are based on data from 4 separate companies. Multiple regressions were then performed to research the relationship between the identified variables. The findings support the proposed approach and give credibility to present decision-making strategies that may impact the actual outcome of the company, which is Net sales
KeywordsRegression, key performance indicators, Supply chain , net sales.
INTRODUCTION
Corporate companies have to focus on supply chain (SC) skills and expertise, with the correct products or service characteristics and the overall best value, to deliver goods and services to the market at the lowest potential cost. For SC 's productivity, performance metrics are critical. Business activities can not be streamlined any longer, except for the activities of its vendors and consumers (Lu.Dawei, 1998). Supply Chain Performance Measures reflect just how the SC program operates. Measurement of SC success will boost SC 's understanding and efficiency overall. The success assessment of the SC of which a corporation is a partner an emerging necessity. In the past 20 years, interest in measuring performance has increased significantly. Companies also recognized that it is essential to track and recognize company output to succeed in rapidly evolving environments. Measuring was recognized as a critical factor in enhancing business efficiency(Chan, 2011). Specific output metrics to measure the productivity of SC are in operation. Cost and not cost viewpoint; strategic, tactical or
market approach; viewpoint on the market process and financial outcomes are specific viewpoints of supply chain performance measures (SCPM). The previous emphasis was on the monetary perspective, which is slowly shifting into non-financial viewpoints. Some models have undergone other empirical tests, and others have only evolved theoretically(Borgström, 2005). The literature reviewed for the practical formulation and application of the supply chain measurement device offers minimal direction.
OBJECTIVES OF SUPPLY CHAIN
The Performance Measurement System is a stable and useful process, which allows decision-taking systems to be assisted by the collection of data, elaboration, and analysis. A commentary further expanded the description on the idea of "alignment," and "effective " 'Balance' means the need to use specific methods and perspectives which give the business a comprehensive view (Rienkhemaniyom & Pazhani, 2015). The definition of effectiveness relates, however, to the need to build a framework that tracks and assesses goals and targets continually in internally and externally contexts. Supply Chain performance Measure System is a monitoring process that provides workers with input on the effects of action (Song & Sun, 2016) .
Therefore, SCPM 's aim must promote and improve SCM's productivity and effectiveness. The principal objective of SCPM theories and approaches is to assist researchers by enabling them to assess market success, evaluate and improve product quality through improved decision-making processes (Kurien & Qureshi, 2011). An efficient, integrated, and balanced SCPMS should involve the progress evaluation framework as a tool for institutional change. SCPM should promote SC members' cross-understanding and incorporation. It makes an invaluable contribution to SCM decision-making, particularly in redesigning business priorities and objectives and redesigning procedures.
KEY PERFORMANCE INDICATORS FOR SUSTAINABILITY
Since government bodies were the key driver behind the Strategic Decision, the first initiatives for the implementation of key performance metrics centered on global and local levels. The most massive global structure at the regional level is the United Nations Commission on Sustainable Development, although several other programs exist (Singh et al., 2019). New Primary Performance Indicator Sets were produced at the corporate level in the mid-1990s.
Nonetheless, corporations' prominent position in adopting SD has attracted growing attention. The largest international project is the Global Reporting Project. Nonetheless, several other institutions and research systems (Amrina & Yusof, 2011). Although key performance metrics for strategic management is a valuable tool for reporting on SD results, it has two significant drawbacks. First, large corporate initiatives will result in small overall changes, as SD success is linked to the entire supply chain. Therefore, businesses are primarily drawn to resolve specific effects they are responsible for the outcome of the company. The global consequences of all supply chain firms can be left aside. Sustainable supply chain management concept thus arose, based mainly on Life Cycle Assessment methods. trAll efficient supply chain systems are product-oriented and strategic decision metrics for all product stages of life. In our literature review, we differentiated a fourth reporting standard based on sector research (Tavassoli et al., 2014). Key sector- level process metrics are not a product- or business-oriented but interaction-oriented. An example of this kind of framework in the Supply chain sector is used in regression.
As stated in the success assessment, specific measures often mean. Those recommendations will profoundly impact the proposed metrics. We discern three primary literature-related success measures. Therefore, we recommend the following three overlapping strategic choices influencing the suggested Key Performance Indicators. The first issue is about establishing a consistent Key Performance Indicators system for stabilization.
The discussion stays available in the research; some authors argue that measures must be defined on a case-by-case basis; others argue that a standardized set of measures is feasible. From our viewpoint, the Key Performance Indicators help us to anticipate standardization as this global guideline is being adopted by many more businesses from various sizes and sectors worldwide (Aramyan et al., 207). Therefore, in this study, we propose to establish a consistent set of main performance measures and to analyze their relationship with Net sales with the help of the Multiple Regression.
KEY PERFORMANCE INDICATORS GOALS AND CHARACTERISTICS
The key performance indicator objectives must be extracted from the strategic direction chosen. The development of a standard set of key performance indicators permits time comparison and assessment of two significant Key Performance Indicators across the different supply chains. It is also a precondition for communicating effectively both centrally (information and employee motivation) and publicly (SD reports) (Gestionale et al., 2019). The second strategy formulation includes the isolation of each impact criteria. This quality is required to obtain practical technical assistance for SD management practices. Also, these requirements can be used as a basis for achieving and pursuing sustainable targets. A quantitative set of key performance indicators will be our last strategic analysis. Key Performance Indicators can highlight the adsorption mechanism and assess specific win-win situations. These eight key performance indicators are consistent with our strategic guidelines (Part, 2010). The features of key
performance indicators are well debated in the literature. In our opinion, the main performance metrics can be divided into two groups. One contains fundamental and useful characteristics for the key performance indicators, such as being straightforward yet essential, given the available and accurate statistics, useful to stakeholder information requirement, and with a controllable variety of metrics(Angappa Gunasekaran & Kobu, 2007). The two types of key performance indicators must be derived from the proposed management chosen. In our scenario, the main performance metrics should be equivalent to a particular metric over time. The uniformity also means that key performance measures are not technology-oriented. Our second strategic approach involves a collection of effect- oriented measures instead of a single composite index.
KEY PERFORMANCE INDICATORS /VARIABLES OF SUPPLY CHAIN USED AS INDEPENDENT VARIABLES IN THE STUDY.
-
Average Collection Period (Kurien & Qureshi, 2011)(Huang et al., 2019)(Huan et al., 2004)(Farris & Hutchison, 2002)
-
Inventory Conversion Period (Saudi et al., 2019)(Banomyong & Supatn, 2011)(Chen, 2011)(Chae, 2009)
-
Inventory Turnover Ratio (Raob, 2009)(Ruth Banomyong, 2005)
-
Inventory ratio: Degree of Inventory (P, 2017)(Longinidis & Georgiadis, 2011)(Moss & Stine, 1993)
-
Working capital Effectiveness Ratio(Peng & Zhou, 2019)(Gelsomino et al., 2019)(Zhu et al., 2017)
-
Return on investments.(Sambrani & Pol, 2017)(Aramyan et al., 2007)
-
Debtors turnover ratio(Longinidis & Georgiadis, 2011)(Angappa Gunasekaran & Kobu, 2007)
-
Transportation (Kumari, 2017)(MR. Deepak Bhimrao Magar, 2016)(Baymout, 2015) (Pasutham, 2012)
-
Net Sales(Siddhey, 2015)(A. Gunasekaran et al., 2001)(Beamon, 1999)(Cooper, 1998)
REGRESSION ANALYSIS
Linear multiple regression was used to test the association among predicted factors and explanatory factors. They were using the regression model to predict market expectations. Second, a multiple layer perception is being used to predict the relationship between these independent and dependent
variable set. This analysis is given by equation a common- sense study of causality among variables, in which, due to chance, any association may only be a transient phenomenon. Regression methods are used to predict the short and mid- term. These are important as product affects variables on which data are traditionally and for the projected era.
Simple linear regression consists of a dependent variable and an independent variable, whereas multiple linear regression analysis requires two or more independent variables.
Obtaining accurate and reliable estimates of regression through regular tedious steps that can disrupt at any time of the study, and evaluating them (the effects of the regression) is often a problem. The studies have identified in the article the care every researcher must take. Individuals must try to achieve their right findings and analysis, the comprehensiveness, and the detailed level of analysis. The aspect the explanation has to protect for any rational representation of reconstruction findings (both numerical and substantive) while composing a regression analysis
(especially multiple regression) outcomes This paper is meant to be a simple and easy-to-follow description of 4 companies for the understanding of regression analysis results. Conversely, this article's focus is restricted to shed some light just on the fundamental insights that the regression output provides, based on multiple regression output with SPSS tools.
In this article, we would like to widen scholars' eyes wide from which multiple linear regression results can be interpreted effectively. The topic as "analysis of statistics in multiple regression is the same as in multivariate regression, except for in multiple regression, the results of independent variables frequently overlap in their interaction with the dependent variable.
Model |
Unstandardized Coefficients |
Standardized Coefficients |
t |
Sig. |
Collinearity Statistics |
||
B |
Std. Error |
Beta |
Tolerance |
VIF |
|||
(Constant) |
-1088.9 |
456.799 |
-2.384 |
0.097 |
|||
Transport |
4542.6 |
970.642 |
0.804 |
4.68 |
0.018 |
0.597 |
1.67 |
Debtors Turnover Ratio |
-53.917 |
46.214 |
-0.532 |
-1.167 |
0.049 |
0.085 |
9.78 |
Degree of Inventory |
550.501 |
570.703 |
0.328 |
0.965 |
0.04 |
0.153 |
6.54 |
Inventory Turnover Ratio |
4.496 |
54.811 |
0.022 |
0.082 |
0.94 |
0.246 |
4.06 |
Return on Investment |
-112.57 |
367.65 |
-0.056 |
-0.306 |
0.779 |
0.521 |
1.92 |
Working Capital Turnover Ratio |
10.295 |
20.036 |
0.213 |
0.514 |
0.043 |
0.102 |
9.78 |
Model |
Unstandardized Coefficients |
Standardized Coefficients |
t |
Sig. |
Collinearity Statistics |
||
B |
Std. Error |
Beta |
Tolerance |
VIF |
|||
(Constant) |
-1088.9 |
456.799 |
-2.384 |
0.097 |
|||
Transport |
4542.6 |
970.642 |
0.804 |
4.68 |
0.018 |
0.597 |
1.67 |
Debtors Turnover Ratio |
-53.917 |
46.214 |
-0.532 |
-1.167 |
0.049 |
0.085 |
9.78 |
Degree of Inventory |
550.501 |
570.703 |
0.328 |
0.965 |
0.04 |
0.153 |
6.54 |
Inventory Turnover Ratio |
4.496 |
54.811 |
0.022 |
0.082 |
0.94 |
0.246 |
4.06 |
Return on Investment |
-112.57 |
367.65 |
-0.056 |
-0.306 |
0.779 |
0.521 |
1.92 |
Working Capital Turnover Ratio |
10.295 |
20.036 |
0.213 |
0.514 |
0.043 |
0.102 |
9.78 |
a. Dependent Variable: Net Sales
COMPANY 1
ANALYSIS
Correlation |
Annova |
|||||
Sr no |
r |
r square |
Adj r square |
df |
f value |
sig value |
company 1 |
.973a |
.947 |
.841 |
6,3 |
8.942 |
.049b |
company 2 |
.962a |
.926 |
.834 |
5,4 |
10.040 |
.022b |
Table1
The result of the regression in Table 1 shows the R-value, which denotes the measure of the quality of the prediction of the dependent variable (net sales). The outcome shows that the quality of the analysis is useful as the value of the r is 0.973and 0.962 of company 1 and company 2, respectively.
R square also called the coefficient of determination, which is the proportion of variance in the dependent variable that can be explained by the independent variables. The analysis shows that 94.7 % of company 1 and 92.6 % of company 2 independent variables are available for the analysis of the variability of our dependent variable.
Along with this, "Adjusted R Square" is another critical factor. The High discrepancy between the values of R- squared and Adjusted R Square indicates a poor fit of the model. The adjusted R2 tells how well the data points fit a regression line showing the percentage of variation explained only by the independent variables that affect the dependent variable. Also, an example of interpreting and applying a multiple regression model (n.d.)reveals that the "adjusted R²" is intended to "control for" overestimates of the population R² resulting from small samples, high collinearity or small subject/variable ratios. Its perceived utility varies greatly across research areas and time, and it shows that 84.1% and 83.4% accurately report data of companies 1 and 2, respectively.
The F-ratio in the ANOVA tests whether the overall regression model is a good fit for the data. The table shows that the independent variables of company 1 statistically significantly predict the dependent variable. The table shows that the independent variables statistically significantly predict the dependent variable, F(6,3) = 8.942, p(0.049) < .05 (i.e., the regression model is a good fit of the data). In company 2 , F(6,3) = 13.727 , p(0.028),in Company 3 F(6,3)
= 9.411 , p(0.047) , In company 4 F(5,4) = 13.727 , p(0.022)
which shows that the regression model is fit for all the companies.
Ho: There is no significant impact of the Variables on net sales.
H1: There is a significant impact of the variables on the net sales.
The variable name average collection period and Inventory conversion period have the high VIF, so it is excluded.The other values depicts that Transport p(.018)<0.05,Debtors Turnover Ratio p (0.049)<0.05, Degree of Inventory p(0.040)<0.05, ,Working Capital Turnover Ratio p(0.043)<0.05. So we reject the null hypothesis, and it can be said that variables have a significant impact on the Net sales, but Inventory Turnover Ration p(0.940)>0.050 and Return on investment p(0.779)>0..05 which is not significant. It means that the explanatory variable Inventory Turnover ratio and Return on investment is no significant impact on the dependent variable.
Constant -1088.930, is the predicted value for the dependent variable which is net sales , if all independent variables Average Collection Period =0 ,Debtors Turnover Ratio =0, Degree of Inventory =0, Inventory Turnover Ratio =0
,Return on Investment =0, Working Capital Turnover Ratio
=0 and Inventory Conversion Period =0 .
The general form of the equation to predict Net sales from Average Collection Period ,Debtors Turnover Ratio , Degree of Inventory , Inventory Conversion Period, Inventory Turnover Ratio, Return on Investment , and Working Capital Turnover Ratio is: predicted Net Sales = – 1088.930+ (4542.956 x Transport ) + ( -53.917 x Debtors Turnover Ratio) + ( 550.501 x Degree of Inventory) + ( 4.496 x Inventory Turnover Ratio) + ( -112.568 x Return on Investment) + ( 10.295 x Working Capital Turnover Ratio ) .
Unstandardized coefficients indicate how much the dependent variable varies with an independent variable when all other independent variables are held constant. The unstandardized coefficient, B1, for Transport, is equal to 4542.956. It means that increase in the average collection period; there is also an increase in net sales of 4542.956 units. The degree of Inventory is equal to 550.501. It means that increase in the Degree of Inventory; there is also an increase in net sales of 550.501 units. Inventory Turnover Ratio is equal to 4.496. It means that increase in Inventory Turnover Ratio; there is also an increase in net sales of 4.496 units. Working Capital Turnover Ratio is equal to 10.295. It means
that increase in Inventory Turnover Ratio; there is also an increase in net sales of 10.295 units. However, Debtors turnover Ratio and Return on Investment show the inverse effect, which means that increase in up said variables there is a decrease in net sales of 53.917 and 112.568 units, respectively.
Table 2
As shown in the table 2, if all the variable increases by one unite, each the net sales increases from -1088.930 units to 3852.473 units.
CONCLUSION
This work has been carried to evaluate the relationship on the operational and overall efficiency of SC collaboration using several linear regression models of the key performance indicators and company-specific output variable Net Sales. Reliable results from the Regression study point to several conclusions. Firstly, many firm-specific variables tend to be linked to a company's understanding of supply chain collaboration value.
Four different equation with four different firms accurately portrays the effect of multiple key performance metrics on net sales. Various variables have high multi-collinearity values represented from the high VIF, the meaning is omitted from the study, and the particular variables did not impact the equation.
Company 2
Model |
Unstandardized Coefficients |
Standardized Coefficients |
t |
Sig. |
Collinearity Statistics |
||
B |
Std. Error |
Beta |
Tolerance |
VIF |
|||
(Constant) |
534.588 |
133.521 |
4.004 |
0.016 |
|||
Transport |
42.158 |
17.285 |
0.468 |
2.439 |
0.041 |
0.501 |
1.996 |
Debtors Turnover Ratio |
-35.937 |
14.663 |
-0.871 |
– 2.451 |
0.04 |
0.146 |
6.847 |
Inventory Turnover Ratio |
-9.012 |
5.21 |
-0.34 |
-1.73 |
0.159 |
0.477 |
2.099 |
Return on Investment |
249.593 |
550.972 |
1.321 |
4.526 |
0.011 |
0.216 |
4.619 |
Working Capital Turnover Ratio |
-4.366 |
3.885 |
-0.315 |
– 1.124 |
0.324 |
0.235 |
4.249 |
Ho: There is no significant impact of the Variables on net sales.
H1: There is a significant impact of the variables on the net sales.
The variable name average collection period, degree of Inventory, and Inventory conversion period have the high VIF, so it is excluded.The other values depicts that Transport p(.041)<0.05,Debtors Turnover Ratio p (0.040)<0.05,return on Investment p(.011). So we reject the null hypothesis, and it can be said that variables have a significant impact on the Net sales, but Inventory Turnover ratio p(0.159)>0.050 and Working Capital Turnover Ratio p(0.324)>0..05 which is not significant. It means that the explanatory variable Working Capital Turnover ratio and Return on investment is no significant impact on the dependent variable.
Constant 534.588, is the predicted value for the dependent variable which is net sales , if all independent variables Average Collection Period =0 ,Debtors Turnover Ratio =0, Degree of Inventory =0, Inventory Turnover Ratio =0
,Return on Investment =0, Working Capital Turnover Ratio
=0 and Inventory Conversion Period =0 .
Table 3
As shown in the table 2, if all the variable increases by one unite, each the net sales increases from 534.588 units to
-
units.
The other variables display the positive and negative effect on the constant, the association between the dependent variable and independent variables are shown, and the net sales were seen in the value. If the equation runs in a mathematical style, it easily depicts how many units a unit or two per variable the business requires to raise their net profits or other dependent variables. The equation description implies that the company's management should plan differently for different variables that should affect or render a stable relationship between the dependent and independent variables. Using the equation, all stakeholder variables responsible for net sales (Dependent variable) available for review can quickly reflect net sales.
Large companies are calculated by net sales income in the case of operating efficiency and the number of workers for total performance, and companies that cooperate widely seem to regard collaborative performance more strongly. Surprisingly, we could not establish a correlation between perceived efficiency and length of familiarity with successful procurement efforts. After altering for substantial firm- specific impacts, our findings indicate that cooperative exchange of information on factors and using information systems tend to assess the value of the relationship. Finally, target congruity has been the only conceptual variable we could relate to supply chain efficiency and effectiveness.
REFERENCE
-
Amrina, E., & Yusof, S. M. (2011). Key performance indicators for sustainable manufacturing evaluation in automotive companies. IEEE International Conference on Industrial Engineering and Engineering Management, 10931097.
https://doi.org/10.1109/IEEM.2011.6118084
-
Aramyan, L. H., Lansink, A. G. J. M. O., Van Der Vorst, J. G. A. J., & Kooten, O. Van. (2007). Performance measurement in agri-food supply chains: A case study. Supply Chain Management, 12(4), 304 315. https://doi.org/10.1108/13598540710759826
-
Banomyong, R., & Supatn, N. (2011). Developing a supply chain performance tool for SMEs in Thailand. Supply Chain Management, 16(1), 2031. https://doi.org/10.1108/13598541111103476
-
Baymout, M. (2015). Supply-Chain-Management-for-Small-and- Medium-Size-Enterprises. International Journal of Advancements in Research & Technology, 4(5), 2034.
-
Beamon, B. M. (1999). Measuring supply chain performance. In International Journal of Operations and Production Management (Vol. 19, Issue 3, pp. 275292). https://doi.org/10.1108/01443579910249714
-
Borgström, B. (2005). Exploring efficiency and effectiveness in the supply chain: A conceptual analysis. Proceedings from the 21st IMP Conference, 113. http://impgroup.org/uploads/papers/4670.pdf
-
Chae, B. (2009). Developing key performance indicators for supply chain: An industry perspective. Supply Chain Management, 14(6), 422428. https://doi.org/10.1108/13598540910995192
-
Chan, C. K. (2011). Successful Strategies in Supply Chain Management. In Successful Strategies in Supply Chain Management. https://doi.org/10.4018/978-1-59140-303-6
-
Chen, H. L. (2011). An empirical examination of project contractors supply-chain cash flow performance and owners payment patterns. International Journal of Project Management, 29(5), 604614. https://doi.org/10.1016/j.ijproman.2010.04.001
-
Cooper, J. D. P. A. M. C. (1998). Supply Chain Postponment and Speculation Stretegies How to Choose the right strategy. Journal of Business Logistics.
-
Farris, M. T., & Hutchison, P. D. (2002). Cash-to-cash: The new supply chain management metric. International Journal of Physical Distribution and Logistics Management, 32(4), 288298. https://doi.org/10.1108/09600030210430651
-
Gelsomino, L. M., de Boer, R., Steeman, M., & Perego, A. (2019). An optimisation strategy for concurrent Supply Chain Finance schemes. Journal of Purchasing and Supply Management, 25(2), 185196. https://doi.org/10.1016/j.pursup.2018.07.004
-
Gestionale, I., Magistrale, L., & Augusto, D. (2019). Key Performance Indicators of Supply Chain Seica S.P.A. Thesis, December.
-
Gunasekaran, A., Patel, C., & Tirtiroglu, E. (2001). Performance measures and metrics in a supply chain environment. International Journal of Operations and Production Management, 21(12), 71 87. https://doi.org/10.1108/01443570110358468
-
Gunasekaran, Angappa, & Kobu, B. (2007). Performance measures and metrics in logistics and supply chain management: A review of recent literature (1995-2004) for research and applications. International Journal of Production Research, 45(12), 28192840. https://doi.org/10.1080/00207540600806513
-
Huan, S. H., Sheoran, S. K., & Wan, G. (2004). A review and analysis of supply chain operations reference (SCOR) model. Supply Chain Management, 9(1), 2329.
https://doi.org/10.1108/13598540410517557
-
Huang, H., Li, S., & Yu, Y. (2019). Evaluation of the allocation performance in a fashion retail chain using data envelopment analysis. Journal of the Textile Institute, 110(6), 901910.
https://doi.org/10.1080/00405000.2018.1532376
-
Kumari, C. P. A. (2017). AN EFFECTIVE WAY TO OPTIMIZE THE KEY PERFORMANCE FACTORS OF SUPPLY CHAIN MANAGEMENT (SCM). Thesis, Jain Unive(December).
-
Kurien, G. P., & Qureshi, M. N. (2011). Study of performance measurement practices in supply chain management. In International Journal of Business, Management and Social Sciences (Vol. 2, Issue 4). www.ijbmss-ng.com
-
Longinidis, P., & Georgiadis, M. C. (2011). Integration of financial statement analysis in the optimal design of supply chain networks under demand uncertainty. International Journal of Production Economics, 129(2), 262276.
https://doi.org/10.1016/j.ijpe.2010.10.018
-
Lu.Dawei. (1998). Fundamentals of Supply Chain Management. In Journal of the Royal Society of Medicine (Vol. 91, Issue 10). https://doi.org/10.1177/014107689809101016
-
Moss, J. D., & Stine, B. (1993). CASH CONVERSION CYCLE AND FIRM SIZE: A STUDY OF RETAIL FIRMS. Managerial
Finance, 19(8), 2534. https://doi.org/101108/eb013739
-
MR. Deepak Bhimrao Magar. (2016). COMPARATIVE STUDY OF SUPPLY CHAIN MANAGEMENT IN RETAIL AND MANUFACTURING INDUSTRIES IN AURANGABAD DISTRICT. In Ph.D. Thesis (Issue December).
-
P, R. R. (2017). A STUDY OF THE RELATIONSHIP AMONG SUPPLY CHAIN PERFORMANCE , MANAGEMENT FACTORS AND ORGANIZATIONAL PERFORMANCE OF MANUFACTURING INDUSTRIES Submitted by Department of Management Studies. Thesis, 600095(May).
-
Part, R. C. (2010). Measuring retail supply chain performance: theoretical model using key performance indicators (KPIs). Supply Chain Management: An International Journal, Unit 07, 15.
-
Pasutham, A. (2012). SUPPLY CHAIN PERFORMANCE MEASUREMENT FRAMEWORK: CASE STUDIES OF THAI MANUFACTURERS.
-
Peng, J., & Zhou, Z. (2019). Working capital optimization in a supply chain perspective. European Journal of Operational Research, 277(3), 846856.
https://doi.org/10.1016/j.ejor.2019.03.022
-
Raob, C. M. R. and K. P. (2009). INVENTORY TURNOVER RATIO AS A SUPPLY CHAIN PERFORMANCE MEASURE. Serbian Journal of Management, 4150.
-
Rienkhemaniyom, K., & Pazhani, S. (2015). A Supply Chain Network Design Considering Network Density. https://doi.org/10.1007/978-3-319-19006-8_1
-
Ruth Banomyong. (2005). Measuring the Cash Conversion Cycle in an International Supply Chain. Annual Logistics Research Network (LRN) Conference Proceedings 2005.
-
Sambrani, V. N., & Pol, N. B. (2017). Supply Chain Management Impact of Distributor ROI Towards Sales Enhancement in FMCG Sector. SSRN Electronic Journal. https://doi.org/10.2139/ssrn.2770481
-
Saudi, M. H. M., Wedi Rusmawan Kusumah, R., Adjeng Mariana, R., & Zainudin, Z. (2019). Effect of working capital management on supply chain performance and company growth in Malaysian manufacturing industry. International Journal of Supply Chain Management, 8(1), 256265.
-
Siddhey, P. K. (2015). A STUDY OF THE EFFICACY OF SUPPLY CHAIN MANAGEMENT IN MANUFACTURING SECTORS. PHD Thesis.
-
Singh, C. S., Soni, G., & Badhotiya, G. K. (2019). Performance indicators for supply chain resilience: review and conceptual framework. Journal of Industrial Engineering International, 15(s1), 105117. https://doi.org/10.1007/s40092-019-00322-2
-
Song, G., & Sun, L. (2016). Evaluation of factors affecting strategic supply chain network design. International Journal of Logistics: Research and Applications, 0(0), 121.
https://doi.org/10.1080/13675567.2016.1267125
-
Tavassoli, M., Faramarzi, G. R., & Saen, R. F. (2014). A joint measurement of efficiency and effectiveness for the best supplier selection using integrated data envelopment analysis approach. International Journal of Mathematics in Operational Research, 6(1), 7083. https://doi.org/10.1504/IJMOR.2014.057861
-
Zhu, Y., Xie, C., Wang, G. J., & Yan, X. G. (2017). Comparison of individual, ensemble and integrated ensemble machine learning methods to predict Chinas SME credit risk in supply chain finance. Neural Computing and Applications, 28, 4150.
-
https://doi.org/10.1007/s00521-016-2304-x