
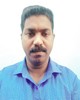
- Open Access
- Authors : Sajithkumar S K
- Paper ID : IJERTV12IS030129
- Volume & Issue : Volume 12, Issue 03 (March 2023)
- Published (First Online): 29-03-2023
- ISSN (Online) : 2278-0181
- Publisher Name : IJERT
- License:
This work is licensed under a Creative Commons Attribution 4.0 International License
Regression Model to Predict Road Accidents in India: Identifying Relevent Factors and Parameters for Improved Road Safety Policies
Sajithkumar S. K.
Lecturer in Automobile Engineering department Government Polytechnic College, Attingal Kerala, India
AbstractRoad accidents are significant issue globally, but they are particularly severe in India due to tremendous growth in road networks and traffic. Road safety involves implementing procedures and methods to prevent accidents by controlling various parameters such as road characteristics, traffic volume, driver behavior, vehicle condition and weather conditions. This paper aims to identify the relevant factors and parameters that contribute to road accidents and develop a model to predict the number of accidents. The number of accidents is a variable that depends on various independent parameters. To estimate the relationship between the identified parameters and the number of accidents, regression analysis is utilized.
KeywordsRoad accident, road safety, accident prediction.
-
INTRODUCTION
Transportation is vital both to the economic success and to the quality of life in urban and rural areas. However, the rapid growth of city populations and corresponding vehicle travel, commerce, and transportation infrastructure has generated negative effects such as congestion, deterioration of air quality, noise, and motor vehicle crashes. An accident is an unpleasant, undesirable or damage that happens unexpectedly or by chance.
Road transport is the backbone of modern society and economy. Tremendous growth of road network and road traffic in India brought the problem of road accidents resulting in injury and fatalities to road users. Although road transport safety is a worldwide issue but it is more severe in India. [1]Increase in vehicle population and limited expansion of roads will ultimately leads to road accidents. Accidents causes severe mess to society in terms of human costs, economic costs, property costs and medical costs. Understanding the various factors that affect accident occurrence is of particular concern to decision makers.
[2] Road accidents has been a treat to the safety of family members and are associated with numerous problems each of which needed to be addressed separately; human, vehicle and environmental factors play roles, before, during and after traumatic event. Now road accidents are becoming very common and are robbing the nation of its valuable human resources, and the implications of these lead to both social and economic trauma. The measures identified and implemented to reduce road accidents are named as road safety measures. Preventing road accidents is a complex and multifaceted issue that involves various sectors and dimensions of safety. This includes systematic planning andmanagement of road development, ensuring the availability of safer vehicles, and implementing a comprehensive response to accidents. Achieving road safety also requires the use of modern traffic management systems and practices, providing driver training programs, improving safety standards for road design, construction, operation and maintenance, as well as producing and maintaining safer vehicles. Ultimately, road safety measures are aimed at reducing the occurrence of accidents on the roads. Management, road authorities, road designers and road safety practitioners need prediction tools, commonly known as Accident Prediction Models (APMs), [3] allowing them to analyze the potential safety issues, to identify safety improvements and to estimate the potential effect of these improvements in terms of crash reduction. Controlling of the road accident factors reduces the number of accidents.
-
LITERATURE REVIEW
In past years numerous studies have been carried in road accident prediction using different statistical methods under different road traffic conditions. Some of the major models are listed below.
-
Smeeds Model
[4] In this model the number of fatalities, the number of vehicles and the population are connected through an equation, D/N =0.0003(N/P)-0.67. D, N and P are number of deaths, Number of vehicles and Population respectively. This model does not fit to the available Indian data. -
Andreassen Equation
Smeeds analysis was heavily criticized by Andreassen for model accuracy. He argued that the Smeeds formula cannot be applied universally to all countries. The generalized relationship Andreassen [5] produced in the year 1985 is of the form,
D=e13.47xN0.613xP-0.63.
(1)
The failure of Smeeds model to predict fatalities in many developed and developing countries motivated Andreassen to modify the same by including other variables like road length to improve its predictability.
-
Accident Prediction Model (APM) with Crash Modification Factors (CMFs)
Accident Prediction Model (APM) model with a unique set of Crash Modification Factors (CMFs) is developed in
transnational accident prediction modelling which predicts the average crash frequency for a specific site [3].
Np = Nspf x (CMF1x CMF2.. CMFm)x C
(2)
Np is predicted average crash frequency for a specific site, Nspf is predicted average crash frequency determined for the base conditions of the safety performance function, CMF1 is crash modification factor and C is the calibration factor.
Crash modification factors and crash modification functions the indicators that quantify the crash reductions that result from interventions a are the basis for evidence-based safety policies. Specifically, CMFs are fundamental to identifying the most effective road safety countermeasures. Furthermore, they are a useful tool for achieving optimal use of resources as they allow for calculating safety benefits in economic analyses of safety policies. Through a crash modification function (CMF) it is possible to combine different evaluation results and consequently better comprehend and implement effective safety measures
-
Generalized linear Regression modeling
Generalized linear model developed by using GLIM computer package is used to predict accidents in freeways [6]
E(P) = aTb
(3)
where P, the accident potential per kilometer per unit of time, T traffic volume per unit of time, a and b are model parameters estimated by GLIM. This package gives the flexibility of a nonlinear accident traffic relationship with user specified error and empirical Bayesian procedure for improving the accuracy of model.
-
The Empirical Bayes (EB) approach
[7] The empirical Bayes (EB) approach to road safety analysis combines local accident history with an expected accident frequency, estimated with accident prediction model. The general form of accident prediction model isP = 0. AADT 1. exp (2×2 + 3×3 + ….) (4)
where P is accident frequency rate, AADT is the average annual daily traffic and xi , i are the regression parameters.
-
Expected Crash Frequency modified EB approach
Np/x = Npw + X/Y (1 w)
(5)
w = (1 + KNpY /L ) 1
(6)
where Np/x =expected number of crashes at subject location given that X were reported, crashes per year
Np = expected number of accidents calculated using EB approach, Y number of years w weight given to Np/x and K is the dispersion parameter
-
-
METHODS AND MEASURES
India currently has only one mechanism of collecting road accident data and that is from the olice accident investigations. Secondary data collected from the government website for the period of 13 years from 2003. The collected data comprised state wise listing of total accidents, injured, fatalities happened in accidents, reason for occurrence of
accidents, and the type of vehicle involved in the accident. Accident fatality rate is used as a measure of fatality, which is the product of vehicles per person and fatalities per vehicle. Accident prediction model for fatalities will be useful for forecasting. These forecasting will alert policy makers to take counter measures.
The factors which lead to accidents are generally grouped under following heads Road design, Road maintenance, Traffic control, Vehicle Design and protective device, Vehicle and garage inspection, Driver training and regulation of professional driver, public education and information. The predominant factors lead to road accidents, are Road condition, Fault of driver, Condition of vehicle, Weather condition [9].
Driver related factors include driver training and testing, driver behavior, overs speeding, driver errors, drugs and alcohol consumption, impaired drivers etc. Road condition includes dangerous road locations, lack of pedestrian walkways, lack of illumination and poor rescue system. Vehicle related factors includes defects in vehicle and environment around road.
The relevant parameters are identified for developing a model for the prediction of number of accidents are number of registered vehicles, population and length of road. Accidents are reported by police departments and Hospitals. Accidents recorded by police department are taken for the analysis to obtain statistical regression model. Accident injury severity are classified in to fatal injuries, series injuries, slight injuries, very slight injuries, property damage only. Injury severity refers to the outcome of accidents in terms of injury to people or damage to property. The probability of occurrence is affected by large number of risk factors related to the elements of traffic system, infrastructure and traffic control devices, vehicles and road users. Regression analysis is done to find the relationship between the parameter.
Regression analysis is a statistical method used to estimate the relationships between the variables. It encompasses several techniques for modelling and analyzing multiple variables, with a particular emphasis on the relation ship between a dependent variable and one or more independent variables. Regression analysis is frequently employed for prediction purposes.
Collected secondary data analysis done with the use of MS-Excel software. In the analysis, total number of accidents that occurred along the national highways is taken as dependent variable while the parameters such as number of vehicles, population and length of road comprises of independent parameters.
-
Fatality Prediction Model
Regression analysis done with the use of MS-Excel software to predict the fatality by road accidents in national highways. The independent parameter identified for the analysis is the number of registered vehicles, total population and length of road during the period from 2003 to 2015. From the regression analysis the equation obtained is
F = 207409 + 0.00050V 0.00012P 1.356L
(6)
where V is the number of registered vehicles, P is the total population, L is the length of highway in kms and F is the total fatalities
-
Accident Prediction Model
MS-Excel software is used for regression analysis to find out the mathematical model for the prediction of road accidents in highways. the regression equation obtained is
A = 452839 + 0.00073V 0.00022P 2.23461L
A is the number of accidents, V is the total number of vehicles registered, P is the total population and L is the length of road in kms.
-
-
RESULTS AND DISCUSSION
In fatality prediction model p value obtained for the explanatory variables number of vehicles(V) and total population(P) are below the level of significance and for length of road(L) is which is above significance level. So, the variables V and P are significant in fatality prediction model. The adjusted R square value of 0.84 indicates that 84% of the variance in fatality can be attributed to the selected variables. Significance value for F is less than 0.05, therefore at 5% level of significance at least one explanatory variable has significant linear relationship with response variable and the fitted linear model is valid.
According to accident prediction model, the p-values for the explanatory variables number of vehicles(V) and total population (P) are below the significance level, indicating that they have significant impact on the model. However the p- value for the length of road(L) is above the significance level, indicating that it does not have a significant impact on the model. The adjusted R square value of 0.46 indicates that 46% of the variance in the accident can be attributed to selected variables. The significance value obtained for F is less than 0.05, suggesting that at least one explanatory variable has a significant linear relationship with the response variable, and the fitted linear model is valid at 5% level of significance.
-
CONCLUSION
In the models formulated by the study it is found that at least one selected variable in linear relation with the
independent variable by the inference from F-test. So, two mathematical models namely fatality prediction model and accident prediction model are valid with R square value of
0.84 and 0.46 respectively. Parameters like average annual daily traffic, road width, width of carriageway, width of median and number of junctions are assumed to be constant. Weather condition, number of minor crossing and exits, type of shoulder, footpath present or not, service road availability, type of road condition are not taken in to consideration. These are the limitations of the model. A more accurate model can be formulated by taking all the parameters as independent variables, which is possible only over a fixed length of road.
REFERENCES
[1] M. Yeole, R. K. Jain, and R. Menon, Prediction of Road Accident Using Artificial Neural Network, IJETT, vol. 70, no. 2, pp. 143150, Feb. 2022, doi: 10.14445/22315381/IJETT-V70I2P217. [2] J. G. Beck and S. F. Coffey, Group cognitive behavioral treatment for PTSD: Treatment of motor vehicle accident survivors, Cognitive and Behavioral Practice, vol. 12, no. 3, pp. 267277, Jun. 2005, doi: 10.1016/S1077-7229(05)80049-5. [3] F. La Torre et al., Development of a Transnational Accident Prediction Model, Transportation Research Procedia, vol. 14, pp. 17721781, Jan. 2016, doi: 10.1016/j.trpro.2016.05.143. [4] R. J. Smeed, Some Statistical Aspects of Road Safety Research, Journal of the Royal Statistical Society. Series A (General), vol. 112, no. 1, pp. 134, 1949, doi: 10.2307/2984177. [5] D. Andreassen, Population and registered vehicle data vs. road deaths, Accident Analysis & Prevention, vol. 23, no. 5, pp. 343351, Oct. 1991, doi: 10.1016/0001-4575(91)90055-A. [6] National Research Council, Ed., Highway and traffic safety and accident research, management and issues. Washington, DC: National Academy Press, 1993. [7] J. Ambros and J. SedonÃk, A Feasibility Study for Developing a Transferable Accident Prediction Model for Czech Regions, Transportation Research Procedia, vol. 14, pp. 20542063, Jan. 2016, doi: 10.1016/j.trpro.2016.05.103. [8] J. Bonneson, Role and Application of Accident Modification Factors in the Highway Design Process. [9] B. Dadashova, B. A. RamÃrez, J. M. M. McWilliams, and F. A. Izquierdo, Dynamic Statistical Model Selection: Application to Traffic Accident Analysis in Spain, Procedia – Social and Behavioral Sciences, vol. 48, pp. 642652, Jan. 2012, doi: 10.1016/j.sbspro.2012.06.1042.