
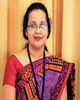
- Open Access
- Authors : Anvitha A , Chandana P R , Syeda Nousheen Fathima , Dr. Ayesha Taranum
- Paper ID : IJERTV11IS060242
- Volume & Issue : Volume 11, Issue 06 (June 2022)
- Published (First Online): 16-07-2022
- ISSN (Online) : 2278-0181
- Publisher Name : IJERT
- License:
This work is licensed under a Creative Commons Attribution 4.0 International License
Pharmaceutic Inflammation Prognosis Based on Neighborhood Information Aggregation in Neural Networks
Dr. Ayesha Taranum Assistant professorDept. of ISE, GSSSIETW,
Mysuru, India
Anvitha A
Dept. of ISE,GSSSIETW,
Mysuru,india
Chandana P R
Dept. of ISE, GSSSIETW,
Mysuru, India
Syeda Nousheen Fathima
Dept. of ISE,GSSSIETW,
Mysuru, India
Abstract: Online health fraternities are rapidly expanding their medical information resources for patients, medical practitioners, and system administrators. We collect real- time health posts from reputable websites in this system, where cases include their examinations, including their body goods, and emprise with medicinals used by them. We want to run a summary of stoner postings per cure and move forward with appropriate cures for the medical community as well as the persistent community in general. Similarly, we intend to dispose of addicts based on their unreserved mental state.We will also undertake cognizance discovery from stoner postings, using Association Rule Mining to generate valuable configurations about the triad medicinals- symptoms-medicinal.
Keywords: sentiment analysis, opinion mining, conclusionsfor medical fraternity, Pattern prediction, summarization
-
INTRODUCTION
With the vast accumulation in ambush, electronic information is likewise accelerating in colossal quantum, which, while advantageous with felicitations to the Information Age, generates an outflow of consistency and slack. Furthermore, information comprehension and consequential knowledge continue to be key concerns. On data gathered from the health tribunal point health- boards.com, we propose to execute individual powerful applications such as Association Rule Mining, Summarization, and Sentiment Analysis for knowledge mining of the health posts. Electronic information is also accelerating in colossal quantum with the massive accumulation in ambush, which, although beneficial with felicitations to the Information Age, causes an outflow of consistency and slack. Additionally, information comprehension and consequential knowledge remain major challenges. We propose to perform individual powerful applications such as Association Rule Mining, Summarization, and Sentiment Analysis for knowledge mining of the health posts using data acquired from the health tribunal point health-boards.com. The next step is to create an abstract, which entails revitalising rooted content. Machine literacy task of association rule mining is well-known and long-standing. It's employed in a well- known database to identify interesting correlations
between variables. The arrangement RHS (Right Hand Side) = LHS (Left Hand Side) According to the rule, RHS is likely to occur anytime the LHS set appears. Sentiment analysis (SA) or
opinion mining is the process of modifying attitudes from a textbook (OM). Individual opinions, television channels, and feelings about reality are all examples of these attitudes. Reality can be used to portray people, events, or circumstances. These are the motifs that are most likely to be safeguarded by user evaluations. WalaaMedhat considered sentiment analysis to be a bracket operation. The bracket situations that were evaluated were document position, judgement position, and respect position. The power characteristics are preferably taken from the textbook while performing SA, and bracketing is done using the suitable classifier.
-
LITERATURE SURVEY
[1] A summary is given below. For summarization, a simplified Lesk algorithm was used in this study. After weighting, the sentences are arranged in decreasing order, and summarising is performed using the percentage of summarization as input. The output is evaluated using precision, recall, and the f-measure. This is a simple algorithm that evaluates each sentence separately, making it useful for summarising user comments. [2] A summary is given below. For summarization, a simplified Lesk algorithm was used in this study. After weighting, the sentences are arranged in decreasing order, and summarising is performed using the percentage of summarization as input. The output is evaluated using precision, recall, and the f-measure. This is a simple algorithm that evaluates each sentence separately, making it useful for summarising user comments. A hybrid model of lexicon-based and rule-based techniques was used on unstructured and informal medical literature. Information at the sentence level is not considered, which could improve the result. Amedical ontology was created in that leverages Me SH to provide an interface for navigating across discussions. One of the main disadvantages is thatmedical terminology with only one word is considered.
[3] Association rule mining was used on UCI. Data on heart illness. After receiving the rules, they evaluated them in regard to gender and significant risk factors. They used apriori, predictive apriori, and tertius algorithms to create rules. Their findings show how computational intelligence can be used to identify crucial disease-causing components. The PCMIE system, developed by Yi Chen et al., links medical data and offers findings in terms of keyword search and co-occurrence analysis for ADR detection. It is unable to determine whether the linked word is "cause" or "ADR." [4] The analysis of user posts from health communities for knowledge discovery is an attractive research issue that we discuss in this work. This study will explore for connections between different medications, diseases, and symptoms. Doctors will benefit from learning about the adverse effects of different medications in order to prescribe better drugs to people with similar diseases. [5] A Comparative Approach to Sentiment Analysis from Summarized User Health Posts A number of online health communities provide a plethora of medical information supplied by users. The health post includes a patient's perspective, opinion, or experience with a specific medicine. We proposed in this paper to collect real-time posts from websites such as askapatient.com, healthboards.com, and others. We proposed utilising a simple Lesk algorithm to summarise user posts. The importance of each sentence is determined using an online lexicon called Word Net when summarising. This research focuses solely on diabetes and its associated patterns. The goal of this research is to compare and contrast different sentiment analysis approaches that are used to process patients' opinions. Doesn't anticipate the link between symptoms, diseases, and medications. [6] Online health communities provide a wealth of medical information that could be accessed by anyone. As with other data- intensive activities, this data contains interesting patterns that, if examined, analysed, and understood, might be extremely beneficial to directors, medical practitioners, and patients alike. There are websites where patients can share their symptoms or pharmaceutical side effects. We employed data mining to uncover polychromatic likely connections between similar real-time health content from respected websites in this study. We'll also use stoner posts to undertake knowledge discovery, identifying useful' patterns' in groupings like disorder to disease, fever to treatment, and pharmaceutical to symptom. It is revealed that there is a treatment for every ailment. [7] This research presents a new approach of pattern analysis in medical data sets utilising SOM for medical treatment services in a ubiquitous computing environment where real-time accessibility and agility are required. In this paper, we must classify disease patterns in the medical historical record to join the information of the patient, using SOM neural network with input vectors of different features, disease code, and input factors to take the medicaltreatment service in medical data sets, to reduce patients' search effort for diagnosis information and to improve the rate of accuracy. We conduct trials with data acquired in the medical centre to validate better performance.
[8] Much of the current research in the area of sentiment analysis is focused on the sheer volume of opinion rich web resources such as discussion forums, review sites, blogs, and news corpora available in digital form due to the sheer volume of opinion rich web resources such as discussion forums, review sites, blogs, and news corpora available in digital form. People are expected to create a system that can recognise and classify sentiment or opinion expressed in an electronic text. We could extract thoughts from the internet and anticipate online client preferences using an accurate method for predicting sentiments, which may be useful for economic or marketing studies. Until date, this research community has focused on a few key issues, including sentiment classification, feature-based classification, and negation handling. This paper gives a summary of sentiment analysis methodologies and approaches, as well as issues that have arisen in the field. [9] Pattern recognition is a challenging topic in a wide range of engineering and scientific disciplines. Medical imaging for diagnostic purposes is the most common operation in the drug industry. Medical records mining is the subject of this research, which is a different form of pattern recognition operation. The analysis of treatment pathways (in which characteristic and healingprocesses are organised on a timetable) as a means of providing standardised, high-quality patient care has been one of the most important problems in the field of medical informatics in the recent decade. Because there is a need for scalable computational tools for pattern disquisition, we propose an abstract frame, tailored to Poland's environment, for describing data mining jobs to find knowledge about instances' and providers' parallels with multiple temporal and process granularities. [10] As massive amounts of data are generated at a rapid rate, effective information access becomes a critical issue. Effective recapture of brief and significant information is frequently requested, particularly in critical subjects as as medicine and finance. The Unified Medical Language System, an ontology knowledge source from the National Library of Medicine, is used in this research to suggest a new doper query-predicated primer summarization approach. When we compare our system to a keyword-only strategy, we see that ontology-based methods outperform keyword-only techniques. Our design also shows that it may be applied to other types of data storage. [11] We shared our current work on an addict query- predicated summarising system as well as our own experience with it in this publication. Ontology knowledge has been shown to be a useful tool to go beyond keyword- based information retrieval techniques. We believe that ontological knowledge can be used in different disciplinesof broad information processing and knowledge discovery as a result of our essay. When statistical data of judgments in the abstract is available, use thresholds for handpicking judgments in the summary. In our strategies, we use some natural language processing techniques such as parsing and syntax analysis.
[12] Based on a survey of the present state of the art as well as existing medical document formats and summary applications, this investigation looked into the possibilities of summarising technology in the medical profession. The problem that the summary inquiry must solve is the requirement to be viewed through the prism of the specific field's requirements. The creation of informative summaries using more advanced language and knowledge engineering strategies, the creation of manifested summaries, portability to new sub-domains, the design of evaluation scripts that model real-world situations, and the integration of summarization technology in practical operations such as the clinical workflow are just a few of the challenges. [13] In this publication, we discussed our present work on an addict query-predicted summarising system as well as our own experience with it. Beyond keyword-based information retrieval approaches, ontology knowledge has been proved to be a beneficial tool. As a result of our essay, we believe that ontological knowledge can be used to several fields of large information processing and knowledge discovery. When statistical data on abstract judgments is available, employ thresholds to handpick summary judgments. We use natural language processing techniques like parsing and syntax analysis in our. [14] Based This inquiry looked into the possibilities of summarising technology in the medical profession based on a survey of the current state of the art as well as existing medical document formats and summary apps. The requirement to be examined through the prism of the specific field's requirements is the challenge that the summary inquiry must address. The creation of informative summaries using advanced language and knowledge engineering strategies, manifested summaries, portability to new sub-domains, the design of evaluation scripts that model real-world situations, and the integration of summarization technology into practical operations such as the clinical workflow are just a few of the challenges. [15] Abstractive summarization systems generate a large amount of conformable data and a small amount of extraneous summary. Because of the intricacy of natural language processing, abstractive summarization is a difficult task in general. In the field of abstractive summarization, multiplex manufacturing is being carried out, particularly through the application of ontology in kaleidoscopic departments. Now is the time to think about how we can combine all of these unambiguous baronies into a single platform to create a robust, extensible summarization system that will allow us to generate an abstractive summary from various demesnes. This research looks at a variety of ontology-based abstractive summarization techniques and their impact on variousparts. There are also other strategies for valuing ontology that are specified. Without a doubt, this study may be revised in such a way that fresh researchers in the field can benefit from it. A deeper grasp of ontology-based approaches can be gained through hand summarization.
-
MOTIVATION
Computerized systems may now perceive, understand, and act intelligently like humans thanks to the development of artificial intelligence (AI) . A transdisciplinary field, AI ML, Computer Vision, Deep Learning, and Natural LanguageProcessing of language . ML algorithms use a variety of optimization, learning from statistical and probabilistic methods data that was produced based on prior experiences,and use it in making decisions . These algorithms wereconsidered to be used. in a variety of fields, including network intrusion detection of consumer purchasing patterns, industrial processes detection of credit card fraud, illness management, and optimization. Several of these apps were made utilizing the method of supervised learning. Using this strategy, datasets models for prediction are induced using labels that are known.Examples without labels. This outlines the theory that Clinicians can use supervised learning as a potent tool to tool to better effectively perform illness diagnostics .
-
METHODOLOGY
We employ the "Equivalence Class Clustering and bottom- up Lattice Traversal algorithm" in the project to detect relationships between medical items like symptoms, illnesses, and medications. The Eclat algorithm is a fast data processing algorithm.This technique handles both small and big data sets with ease.A system's structure, behaviour, and other aspects are all defined by its conceptual model, or system architecture. A formal description and representation of a system, arranged to facilitate analysis of its structures and behaviours, is known as an architectural description. Both patients and doctors can benefit from the knowledge available in online health forums. However, these user-generated resources are frequently rife with errors.Include false information In this paper, we offer a technique for automated verification of user-generated content's reliability medical claims, as well as the reliability of their writers using verbal clues and monitoring from a distance, reputable sources. Important user-related aspects online publishing of their opinions. The practise of gathering consumer feedback online for products, social and political issues, and other topics is growing in popularity every day. When making choices, the general public and stakeholders can benefit from user views. Opinion mining is a technique for gathering data from social media, blogs, and search engines. The sheer volume of reviews that are unstructured language makes it hard to manually summarise the data. Therefore, extracting and summarising the reviews from corpora and web articles requires effective computational techniques. The purpose of this paper is to give a thorough literature evaluation of computational methods, models, and
algorithms for extracting opinion elements from unstructured reviews.
Fig 1: Proposed System Architecture
Login Module
The administrator logs into the application using their login id and password in this module. One administrator controls the entire application, and the login id and password are stored in the database.
Manage Medical Practitioners
Based on the hospital, the administrator adds medical practitioners to the server. During this process, the administrator assigns each medical practitioner a unique id and password..
Manage Symptoms Data-set
The administrator uploads the symptoms onto the server in this module. Admins have access to edit, update, view, and delete symptoms on and off the server.
Manage Diseases Data-set
The administrator uploads the disorders onto the server in this module. Admins can add, edit, update, view, and remove illnesses from the server.
Manage Medicine Data-set
The administrator uploads the drug information to the server in this module. Admin has access to edit, update, read, and remove medication information on the server.
-
FRAMEWORK AND METHOD FOR DETERMINING LATENT MEDICATION PATTERNS
illustrates the typical four stages required to find treatment trends in EMR material. Data extraction, data pre- processing for classication, medication data grouping, and pharmaceutical pattern detection are some of these the English language are separated by spaces. The accuracy of
the data collected from EMRs depends on how well words are segmented. The collected data ischanged into a format that retrieved medications into several groupings in the third stage. The fourth stage, however, identifies the clinical significance in order to extract it from the clustered data.
-
BASIC PROCESSING STEPS
The fundamental processing phase that kicks off the mining process is data segmentation and extraction. Although differs somewhat from English in an NLP analysis, extracting drug usage scenarios from EMR free text is simpler than extracting other aspects (such as the description of sickness). This is due to the drug's steady and reliable name. We utilized a medicine dictionary given by our cooperating hospital to extract the names of medications from the EMR text in order to obtain Chinese drug treatment material. In EMR text, usage instructions, such as the precise medication consumption time and duration, are listed after the names of the pharmaceuticals. We automatically extracted this data using keywords.
-
PATTERN DISCOVERY WITHOUT TIME SEQUENCE
We utilised a two-dimensional matrix to describe the medication process for each patient based on the data we retrieved from the EMRs. The matrix's columns denote the hospital treatment day, and each line denotes a medicine.
-
THE JACCARD SIMILARITY COEFFICIENT TO DETERMINE HOW SIMILAR PRESCRIBED MEDS ARE TO OTHER MEDICATIONS.
Similarity is a crucial characteristic that helps group individuals who are receiving comparable drug therapies. Indicator Jaccard is used to determine how comparable the drug is.patients' experiences with various LoSs. a Jaccard J Represents
when sample sets A and B are used. The definition may be expressed as the intersection's size divided by the sample sets' union's size. Sets A and B's similarity may be determined
-
USE SPECTRAL CLUSTERING TO CLUSTER THE PATIENTS.
We may construct a graph, PG, based on the similarity measurement, where all of the patients are connected to one another using different edge weights. The similarity between any two patients is measured by their weight. Now, by splitting the network into numerous sub-graphs,
the difficulty of grouping these patients may be resolved. Each of the sub-graphs indicates a group of individuals who most likely receive comparable medical care. While the weights of the connections inside the sub-graphs are large, the goal of cutting during patient clustering is to decrease the weights of the link between two sub-graphs. As a result, we developed a unique clustering approach known as Normalized Cut (N-cut) . An N-cut (Ncut) can be created by taking into account the relationship between the relative densities of each group.
-
PERFORMANCE EVOLUTION
Using five-fold cross-validation, we assessed DivePred's efficacy in identifying possible drug-disease connections. Four of the five equal subsets of the known drug-disease links were utilised to train our model, and the fifth set conduct the test.
Area under ROC curve values of 8 drugs using DivePred and other methods Table 1.
Area under precision-recall curve values of 9 drugs using DrivePred and other methods Table 2.
Results of Wilcoxon test on DrivePred and four others contrast methods for 562 drugs. Table 3.
RESULT AND DISCUSSIONS
To infer the probable relationships between medications and illnesses, the non-negative matrix factorization technique DivePred was created. DivePred records a wide range of data on each drug, including four main types of
drug characteristics and particular features connected to certain elements of the drugs. In the meanwhile, it also records drug-disease connections and disease-disease similarities. To improve the inference of drug-disease connections, the projection of various types of drug attributes, together with the drugs and illnesses neighbour information, was fully integrated. To prioritise which diseases to treat with each treatment, an iterative method was created to assess drug-disease association scores. DivePredperforms better in AUPRs and AUCs than other approaches. DivePred is particularly helpful for biologists since its top candidate featured more actual drug-disease connections.
Figure 2 Two types of curves for evaluating the predicting performance of DivePred and other methods. (a) receiver operating characteristic (ROC) curves; (b) precisionrecall (PR) curves.
CONCLUSION
In this study, we gather real-time health postings from purported websites, apply data mining to identify various correlations from these posts, perform knowledge discovery from user posts, and identify relevant 'patterns' concerning groupings like illness to disease, disease to drug, and drug to symptom. To do this, the Association rules algorithm is employed. Thiswill help doctors identify the negative effects of various medicines, enabling them to recommend better medications to other patients wh have conditions that are similar to those they are treating. Pharmaceutical companies may respond to a range of requests for medications and discover which medicines are popularand should be produced. Additionally, this will let patients learn about the experiences of previous users, enabling them to choose the right medication.
REFERENCES
[1] S.Anami, Categorized Text Document Summarization in the Kannada Language by Sentence Ranking, 12th International Conference on Intelligent Systems Design and Applications (ISDA), pp 776-781, 2020. [2] AlokRanjan Pal, DigantaSaha, An Approach to Automatic Text Summarization using WordNet, IEEE International Advance Computing Conference (IACC), 2019 [3] C. Lakshmi Devasenal and M. Hemalatha, Automatic Text Categorization and Summarization using Rule Reduction, IEEE- International Conference On Advances In Engineering, Science And Management (ICAESM -2012), pp 594-598, 2018 [4] Yi Chen, Yunzhong Liu, Connecting the Dots: Knowledge Discovery in Online HealthcareForums, ICEC14 August 05 – 06 2018, ACM [5] Subhabrata Mukherjee, Gerhard Weikum, CristianDanescu- Niculescu-Mizil, People on Drugs: Credibility of User Statements in Health Communities, KDD 14, August 24 – 27 2014, New York, ACM, 2018 [6] SaeedMohajeri,AfsanehEsteki, Osmar R. Zaiane and DavoodRafiei, Innovative Navigation of Health Discussion Forums based on Relationship Extraction and Medical Ontologies,IEEE International Conference on Bioinformatics and Biomedicine, pp 13-14 ,2018 [7] Unstructured Medical Text, Proc.Of the 2014 9th International Conference on System ofSystems Engineering (SOSE), Adelaide,Australia- June 9-13, pp 85-90, 2018
[8] Yi-Ping Phoebe Chen, Association rule mining to detect factors which contribute to heart disease in males and females, J. Nahar et al. / Expert Systems with Applications 40 (2013) 1086 1093, [9] Elsevier,2017 [10] Lakshmi K.S, G. Santhosh Kumar, Association Rule Extraction from Medical Transcripts of Diabetic Patients,IEEE,2017. [11] Khairullah Khan, BaharumBaharudin, Aurnagzeb Khan, Ashraf Ullah, Mining opinion components from unstructured reviews: A review, Journal of King Saud University Computer and Information Sciences (2014), Elsevier, 2017.