
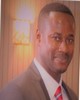
- Open Access
- Authors : Issa Diop , Georges Abdul-Nour , Dragan Komljenovic
- Paper ID : IJERTV10IS120059
- Volume & Issue : Volume 10, Issue 12 (December 2021)
- Published (First Online): 14-12-2021
- ISSN (Online) : 2278-0181
- Publisher Name : IJERT
- License:
This work is licensed under a Creative Commons Attribution 4.0 International License
Overview of Strategic Approach to Asset Management and Decision-Making
Issa Diop1, Georges Abdul-Nour1, Dragan Komljenovic2
1Department of Industrial Engineering, University of Quebec in Trois-Rivieres (UQTR), Trois-Rivieres, QC G8Z 4M3, Canada
2Hydro-Quebec Research Institute (IREQ), Varennes, QC J3X 1S1, Canada
AbstractThis article focuses on identifying and analyzing elements of strategic asset management (SAM). It reviews physical asset management (AM) models defined as the coordinated activity of an organization to realize value from assets, along with SAM which focuses on aligning AM strategies with various levels of organizational strategy (corporate, business, and functional-level strategy). The analysis of recent scientific papers devoted to AM with a special emphasis on SAM aspects and decision-making models as well as risk management help provide a starting point for new research directions specifically the influence of risk-informed decision-making (RIDM) process and emerging industry 4.0 technologies, as well as resilience engineering on SAM. For instance, applying Maintenance 4.0 using technological solutions such as industrial internet of things (IIOT), cloud computing, cyber-physical systems (CPS), and big data analytics could decrease the challenges encountered by top management such as managerial, technological, and methodological challenges. The outcomes will contribute to position and validate the link between SAM and RIDM, as well as Maintenance 4.0 and resilience engineering inside the vast discipline of AM and the alignment with various levels of organizational strategy.
KeywordsAsset management strategy; risk management; resilience engineering; maintenance 4.0; industry 4.0; decision- making.
-
INTRODUCTION
ISO 55000 standard defines Asset Management (AM) as the coordinated activity of an organization to realize value from assets [1]. AM strategies should align with various levels of organizational strategy (corporate, business, and functional-level strategy). Managing assets has become more complex and demanding due to harsh international competition, unpredictability, volatility, as well as insecurity. These businesses face significant uncertainties and dreaded risks of all kinds. These include the strategic, operational, organizational, and financial, as well as technological and technical concerns that seriously influence all the company's business processes. Hence, organizations have serious demands to reduce harmful and unexpected equipment failures inducing high degree expectations from maintenance [2]. They are increasingly thriving to foresee all potential futures in the challenging and dynamic market characterized by escalating resource shortage, environment impairment liability, climate change, etc. [3]. For instance, Komljenovic [4] point out that power utilities should manage the replacement of huge parts of their assets as they reach the end of their lifecycle, become obsolete due to technological changes or because of transition to more efficient and carbon-free power alternatives.
AM is not a novelty; in practice, asset-intensive businesses such as aviation and electrical power compagnies have been managing assets since the dawn of time [5]. Both AM decision
makers and stakeholders in various industries are frequently obliged to make more at lower price. Asset management system (AMS) must establish clear and effective connectivity of the strategic asset management plan (SAMP) and the activities of the various departments and stakeholders of the organization (human resources, operations, maintenance, engineering, etc.) [6]. AM supports business leaders in understanding the value of assets and their role in achieving organizational goals [1].
This review contributes to the current literature on physical asset management and decision-making by attempting through a holistic approach to bring together information about strategic approach to AM and decision-making models with special emphasis on risk management. A focus is given on risk management approach to improving implementation and governance of AM with respect to risk identification and characterization, as well as risk treatment of extreme and rare events which are research components of major interest in the scientific community.
The remainder of this paper is structured as follows: PART 2 presents the methodology of the review to identify papers relevant for this topic. PART 3 conveys a comprehensive review on the theoretical background of the range of decision- making models and techniques used in AM with respect to their usefulness and contribution to the harmonisation and coordination, as well as alignment with various levels of organizational strategy. PART 4 provides a broad overview of emerging aspects of SAM and maintenance 4.0. Finally, PART 5 concludes the review and highlights gaps and provides new research directions in the existing literature as a starting point for future targets for this research.
-
METHODOLOGY FOR THE SYSTEMATIC LITERATURE REVIEW
To have a mapping of the state of the art relevant to this topic, a systematic and critical literature search has been conducted to quantify the scientific production and measure its quality and impact. The search methodology used entailed the following steps: (i) identifying appropriate and relevant keywords employed in the search of databases, (ii) searching the online database Scopus, then (iii) gathering relevant articles, (iv) identifying whether the paper is relevant or not,
-
performing snowball approach to identify more relevant papers, (vi) performing inverse search with the help of the Web of Science (WoS) core collection and Google scholars. The subsequent paragraphs described each step.
-
Keywords definition, online database search and paper selection
First and foremost, keywords relevant to the subject matter were defined as follows:
-
"strategic asset management" or "strategic approach to asset management" or "asset management strategy" or "asset management model" or "physical assets management model" or "physical assets management framework",
-
"risk-informed decision-making" or "risk-informed asset management",
-
"industry 4.0" and maintenance or "intelligent maintenance" or "predictive maintenance" or "condition-based maintenance",
-
"resilience engineering" or "system resilience" or "resilience modeling"
To retrieve further relevant papers, keywords were generated by combining keywords from (a), (b), (c) and (d). Then the combined keywords were used to investigate the databases. The online database Scopus was used to systematically retrieve recent scientific analysis of studies that included either (a), (b), (c), or (d) in the article title and published between 2010 and 2021.
Moreover, further analysis was needed to identify whether the paper is relevant or not. Therefore, each article thus found was subjected to a further investigation of their title, abstract, keywords and, if it appeared to be pertinent, the article was selected to be entirely read and analyze.
Furthermore, snowball approach was conducted by verifying the bibliographies of the previously selected articles to mine more data for this review to be analyzed. Finally, an inverse search was performed with the help of the online databases WoS and Google scholars to gather scholarly relevant research papers which cited the selectedarticles.
The initial search retrieves 96 bibliographic references for the string (a), 103 for (b), 122 for (c), 650 for (d). In the subsequent section, we performes the bibliometrix analysis of the research field, its structure and evolution as well as its dynamical aspects. This analysis gives a mapping of the field growth, the leading productive authors, papers, countries and most relevant keywords.
-
-
Results
-
-
Descriptive Analysis and conceptual structure for (a) keywords
Table 2 Co-word Analysis through Keyword co-
occurrences for string (a)
Rank
Keyword
Occurences
1
Asset management
52
2
Asset management strategy
25
3
Strategic asset managements
20
4
Maintenance
17
5
Risk assessment
12
6
Decision making
10
7
Life cycle
9
8
Investments
9
9
Budget control
7
10
Decision support systems
6
Table 2 Co-word Analysis through Keyword co-
occurrences for string (a)
Rank
Keyword
Occurences
1
Asset management
52
2
Asset management strategy
25
3
Strategic asset managements
20
4
Maintenance
17
5
Risk assessment
12
6
Decision making
10
7
Life cycle
9
8
Investments
9
9
Budget control
7
10
Decision support systems
6
The following figures provide a visualization of the scientific landscape of the subject-matter using the (a) keywords in the search of databases: "Strategic asset management" or "strategic approach to asset management" or "asset management strategy" or "asset management model" or
Figure 1 depicts the the annual total references published between 2010 and 2021 and the annual growth of publications.The number of references decreased in significantly in 2012 then fluctuated from 2013 to 2019 before a high peak was observed in 2020 followed by a decline in 2021. These metrics imply that the annual amount of references published during the survey period was meaningfully erratic. This signals that this research area was unstable in the matter of amount of publications [7, 8].
"physical assets management model" or "physical assets management framework".
Information in Table 1 beolow shows that most of the publications was issued as conference paper (51.50%), journal article (39.00%) and book chapter (3.90%).
Table 1 Document Type for string (a)
Rank
Document Type
# Documents ~ %
1
Article
49~51.00%
2
Conference Paper
41~42.70%
3
Book
2~2.10%
4
Review
2~2.10%
5
Review
8~2.0%
6
Book Chapter
1~1.00%
8
Note
1~1.00%
Figure 1 the number of annual references for string (a)
Information in the chart (Table 2) depicts the most frequent and relevant keyword co-occurrences on the intellectual domain and as well provide hotspots in the field of asset management. The value of authors keyword co-occurrences, and their usefulness within a particular area of research were shown in prior investigations [8-10].
The co-word analysis through keyword co-occurrences point out clusters of keywords. For more details on co-word analysis, the reader is referred to the paper by Cobo [11] and their bibliographic references. Five clusters was found, clustered into four areas of research as shown in Table 3.
Table 3 Top Keyword clustered into 4 areas of research for string (a)
Cluseter 1
(4 items)
Cluseter 2
(8 items)
Cluseter 3
(6 items)
Cluseter 4
(3 items)
Cluseter 5
(1 items)
Management practice
Costs
Budget control
Asset management
Risk management
Strategic asset management
Decision making
Electrical utilities
Asset management strategy
Strategic approach
Decision support systems
Human resource management
Project management
Strategic planning
Investment
Maintenance
Life cycle
Reliability
Reliability analysis
Repair
Risk
Risk assessment
Table 4 Top 10 Authors for string (a)
Most Productive-Authors
Most Cited-Author References
Rank
Author
Documents
Author
Citations
1
Alegre H.
3
Velasquez-Contreras [13]
42
2
Vlok, P.J.
3
Roshani [14]
28
3
Brito , R.S.
2
Caradot [15]
22
4
Brown, K .
2
Joseph [23]
21
5
Dray, A.
2
Morrison [26]
13
6
Hamilto n, M.
2
Ten Veldhuis [27]
13
7
Keast, R.
2
Alegre [16]
13
8
Laue, M .
2
Vreeburg [28]
9
9
Mahmood, M.N .
2
Haynes [30]
8
10
Rødseth, H.
2
Clements [33]
7
Table 4 depicts the most productive-authors as well as the most cited-author references. Afuye [8] remark that the number of papers published in a research area is typically deemed an valuable system of measurement to quantify the impact of an author in a specific area of research. In the same vein, Wang
[12] mentioned that the volume of papers published by an author reveals his nfluence as well as the usefulness of his research. Alegre H. and Vlok, P.J. ranked first in term of amount of publications with 3 papers. In term of amount of citations, Velasquez-Contreras [13] ranked first with 42 citations followed by Roshani [14] ranking second (28 citations) and Caradot [15] (22 citations).The citation analysis depicts the structure of the field through the linkages between nodes Co-citation network illustrates interactions among cited-reference works, cited- journals. Aria [34] indicates that the useful dimensions to comment the co-citation networks are: (i) centrality and peripherality of nodes, (ii) their proximity and distance, (iii) strength of ties, (iv) clusters, (iiv) bridging contributions. Information in Table 5 illustrates the top 10 countries list from the perspective of amount of papers published and amount of citations per country. Within the survey period, the United states ranked first with the largest document number followed by the United kingdom, then Australia. The country collaboration networks depicts how a country interact to others in a particular area of study.
Information Table 6 shows the performance in international collaboration between countries clustered into 5 research groups. The density visualization map shows regular research groups in this area of research and their relations [11].
-
Descriptive Analysis and conceptual structure for (b) keywords
The following figures provide a visualization of the scientific landscape of the subject-matter using the (b) keywords in the search of databases: "Risk-informed decision- making" or "risk-informed asset management".
Information in Table 7 shows that most of the manuscripts was published as conference paper (69.90%) and journal article (26.20%). Figure 2 depicts the the annual total references
Table 5 Top 10 Most Productive Country for string (a)
Rank
Country
Documents
Citations
1
United states
23
22
2
United kingdom
16
65
3
Australia
11
32
4
Netherlands
7
33
5
Norway
4
2
6
South Africa
4
6
7
Canada
4
29
8
Portugal
4
13
9
Colombia
3
2
10
France
3
26
Table 6 Country collaborations clustered into 5 research groups for string (a)
Cluseter 1
(6 countries)
Cluseter 2
(3 countries)
Cluseter 3
(2 countries)
Canada
Australia
France
Iran
United states
Germany
Netherlands
Colombia
Norway
South Africa
United kingdom
published between 2010 and 2021 and the annual growth of publications. The number of references decreased drastically arount 2013 and 2014 before a high peak was observed in 2019. These metrics infer that the annual amount of references published during the survey period was significantly unstable. This indicates that this research area was unsteady in the matter of amount of publications [7, 8].
Table 7 Document Type for string (b)
Rank
Document type
# Documents ~ %
1
Conference Paper
72~69.90%
2
Article
27~26.20%
3
Book Chapter
1~1.00%
4
Erratum
1~1.00%
5
Review
1~1.00%
6
Short Survey
1~1.00%
Figure 2 the number of annual references for string (b)
IJERTV10IS120059
www.ijert.org 66
Table 8 Co-word Analysis through Keyword co-occurrences for string (b)
Rank
Keyword
Occurences
1
Decision making
72
2
Risk-informed decision making
70
3
Risk assessment
56
4
Safety engineering
42
5
Nuclear power plants
31
6
Risk management
27
7
Probabilistic risk assessment
19
8
Uncertainty analysis
19
9
Nuclear fuels
15
10
Nuclear energy
13
Information in Table 8 illustrates the most frequent and relevant keyword co-occurrences on the intellectual domain and as well provide hotspots in this subject-matter. The reader is referred to Zhang [9], Chen [10] and Afuye [8] and their cited references for more details on the value of authors keyword co-occurrences, and their usefulness within a
Table 9 Top Keyword clustered into 5 areas of research for string (b)
particular area of research.
The co-word analysis through keyword co-occurrences point out clusters of keywords. Five clusters was found, clustered into five areas of research as shown in Table 9.
Table 10 shows the most productive-authors as well as the most cited-author references. Narumiya y. ranked first in term of amount of publications with 7 papers, followed by Parry g. ranking second with 6 papers. However, Dubois [35] ranked first in term of amount of citations with 45 citations, followed by Vaurio [36] with 41 citations and Volkanovski [37] with 27 citations.
Information in the Table 11 illustrates the most productive countries from the perspective of amount of papers published and amount of citations per country. Within the survey period, the United states ranked first with the largest document number and citations, followed by Canada, then Japan.
-
Descriptive Analysis and conceptual structure for (c) keywords
The following figures provide a visualization of the scientific landscape of the subject-matter using the (c) keywords in the search of databases: "Industry 4.0" and maintenance or "intelligent maintenance" or "predictive maintenance" or "condition-based maintenance".
Information in Table 12 shows that most of the documents was published as conference paper (55.20%), journal article (35.10%) and book chapter (3.7%).
Rank
Document type
# Documents ~ %
1
Conference Paper
73~59.80%
2
Article
41~33.60%
3
Book Chapter
5~4.10%
4
Review
2~1.60%
5
Note
1~0.80%
Rank
Document type
# Documents ~ %
1
Conference Paper
73~59.80%
2
Article
41~33.60%
3
Book Chapter
5~4.10%
4
Review
2~1.60%
5
Note
1~0.80%
Table 12 Document Type for string (c)
Table 10 Top 10 Authors for string (b)
Most Productive-Authors
Most Cited-Author References
Rank
Author
Documents
Author
Citations
1
Narumiya y.
7
Dubois [35]
45
2
Parry g.
6
Vaurio [36]
41
3
Ferrante f.
5
Volkanovski [37]
27
4
Cho n.
4
Ham [54]
20
5
Dube d.
4
Talarico [55]
16
6
Jang d.
4
Aven [56]
13
7
Lewis s .
4
Fumagalli [58]
11
8
Yamaguchi a.
4
Morales-Torres [62]
8
9
Bujor a .
3
Alipour [66]
8
10
Carlos, S.
3
Gjorgiev [69]
8
Table 11 Top 10 Most Productive Country for string (b)
Figure 3 illustrates the the annual total references published between 2010 and 2021 and the annual growth of publications. The number of references increased considerably. These metrics infer that the annual amount of references published during the survey period was significantly high and stable. This implies that this research area was steady in the matter of amount of publications [7, 8].
Cluseter 1
(9 items)
Cluseter 2
(9 items)
Cluseter 3
(6 items)
Dams
Accident prevention
Bayesian network
Failure (mechanical)
Decision making
Maintenance
Hazards
Decision making process
Nuclear industry
Risk analysis
Nuclear energy
Risk-informed decision making
Risk assessment
Nuclear power plants
Technical specifications
Risk perception
Probabilistic safety assessment
Uncertainty
Risk-informed decision making
PSA
Safety engineering
Regulatory bodies
Uncertainty analysis
Risk management
Cluseter 4
(5 items)
Cluseter 5
(3 items)
Decision makers
Epistemic uncertainties
Integrated risks
Informed decision
Nuclear fuels
Reliability
Probabilistic risk assessment
Risk information
Cluseter 1
(9 items)
Cluseter 2
(9 items)
Cluseter 3
(6 items)
Dams
Accident prevention
Bayesian network
Failure (mechanical)
Decision making
Maintenance
Hazards
Decision making process
Nuclear industry
Risk analysis
Nuclear energy
Risk-informed decision making
Risk assessment
Nuclear power plants
Technical specifications
Risk perception
Probabilistic safety assessment
Uncertainty
Risk-informed decision making
PSA
Safety engineering
Regulatory bodies
Uncertainty analysis
Risk management
Cluseter 4
(5 items)
Cluseter 5
(3 items)
Decision makers
Epistemic uncertainties
Integrated risks
Informed decision
Nuclear fuels
Reliability
Probabilistic risk assessment
Risk information
Figure 3 the number of annual references for string (c)
Rank
Country
Documents
Citations
1
United states
42
49
2
Canada
12
14 Information in Table 13 illustrates the most frequent and
3
Japan
9
1 relevant keyword co-occurrences on the intellectual domain
4
South korea
6
0 and as well provide hotspots in this subject-matter.
5
Spain
4
18
6
Slovenia
3
37
7
Norway
3
18
8
Italy
3
14
9
Germany
3
4
IJE10RT
10IS120C0pin9a
3
0 www.ijert.org 67
Rank
Country
Documents
Citations
1
United states
42
49
2
Canada
12
14 Information in Table 13 illustrates the most frequent and
3
Japan
9
1 relevant keyword co-occurrences on the intellectual domain
4
South korea
6
0 and as well provide hotspots in this subject-matter.
5
Spain
4
18
6
Slovenia
3
37
7
Norway
3
18
8
Italy
3
14
9
Germany
3
4
IJE10RT
10IS120C0pin9a
3
0 www.ijert.org 67
V
Four clusters was found, clustered into four areas of research as shown in Table 14.
Table 13 Co-word Analysis through Keyword co-occurrences for string (c)
Rank
Keyword
Occurences
1
Industry 4.0
102
2
Maintenance
61
3
Predictive maintenance
55
4
Embedded systems
15
5
Machine learning
14
6
Internet of things
14
7
Learning systems
11
8
Artificial intelligence
11
9
Augmented reality
11
10
Decision making
10
Table 14 Top keyword clustered into 4 areas of research for string (c)
Cluseter 1
(11 items)
Cluseter 2
(8 items)
Cluseter 3
(7 items)
Cluseter 4
(7 items)
Advanced analytics
Artificial intelligence
Asset management
Big data
Cyber physical system
Decsion support systems
Augmented reality
Costs
Data analytics
Industrial maintenance
Industry 4.0
Decision making
Deep learning
Industrial research
Life cycle
Internet of things
Embedded systems
Industrial revolutions
Maintenance
Manufacturing process
Engineering education
Machine learning
Maintenance management
Preventive maintenance
Learning systems
Predictive analytics
Maintenance process
Machinery
Predictive maintenance
Maintenance strategies
Manufacturing industries
Smart manufacturing
Table 15 shows the most productive-authors as well as the most cited-author references. El-Thalji, I. and Mentzas, G. ranked first in term of amount of publications with 4 articles. However, Yan [70] ranked first in term of amount of citations with 155 citations, followed by Masoni [71] with 101 citations and Sahal [72] with 84 citations.
Table 15 Top 10 Authors for string (c)
Most Productive-Authors
Most Cited-Author References
Rank
Author
Documents
Author
Citations
1
El-Thalji, I.
4
Yan [70]
155
2
Mentzas, G.
4
Masoni [71]
101
3
Bousdekis, A.
3
Sahal [72]
84
4
Fiorentino, M.
3
Li [84]
79
5
Frontoni, E.
3
Ceruti [88]
76
6
Gattullo, M.
3
Franciosi [89]
46
7
Nordal, H.
3
Scurati [83]
42
8
Paolanti, M.
3
Sipsas [90]
40
9
Uva, A.E.
3
Zonta [91]
39
10
Apostolou, D.
2
Alqahtani [92]
39
Information in the Table 16 shows the most productive countries from the perspective of amount of papers published and amount of citations per country. Within the survey period, Italy ranked first with the largest document number and citations, followed by Germany, then Greece.
Table 16 Top 10 Most Productive Country for string (c)
Rank
Country
Documents
Citations
1
Italy
24
432
2
Germany
14
20
3
Greece
10
101
4
Norway
9
91
5
Brazil
8
70
6
United Kingdom
8
115
7
Spain
7
68
8
France
6
91
9
India
6
20
10
United States
6
113
Information in Table 17 shows the performance in international collaboration between countries clustered into 4 research groups.
Table 17 Country collaborations clustered into 4 research groups for string (c)
Cluseter 1
(3 countries)
Cluseter 2
(3 countries)
Cluseter 3
(3 countries)
Cluseter 4
(2 countries)
Germany
India
France
Brazil
Greece
Norway
Spain
United states
Poland
United kingdom
Italy
-
Descriptive analysis and conceptual structure for (d) keywords
Table 18 Document Type for string (d)
Rank
Document type
# Documents ~ %
1
Article
320~ 49.20%
2
Conference Paper
225~ 34.60%
3
Book Chapter
37~ 5.70%
4
Review
33~5.10%
5
Book
9~ 1.40%
6
Editorial
9~ 1.40%
7
Note
6~ 0.90%
8
Other
8~ 1.80%
Figure 4 the number of annual references for string (d)
The following figures provide a visualization of the scientific landscape of the subject-matter using the (d) keywords in the search of databases: "resilience engineering" or "system resilience" or "resilience modeling".
Information in Table 18 shows that most of the manuscripts was published as journal article (50.00%), conference paper (33.30%) and book chapter (6.70%).
(d)
Rank
Keyword
Occurences
1
Resilience
192
2
Resilience engineerings
127
3
System resiliences
108
4
Resilience engineerings
98
5
Human
71
6
Safety engineering
63
7
Disasters
53
8
Risk assessment
46
9
Reliability
41
10
Decision making
41
11
Uncertainty analysis
38
12
Electric power transmission networks
34
13
Climate change
33
14
Risk management
32
15
Accident prevention
31
(d)
Rank
Keyword
Occurences
1
Resilience
192
2
Resilience engineerings
127
3
System resiliences
108
4
Resilience engineerings
98
5
Human
71
6
Safety engineering
63
7
Disasters
53
8
Risk assessment
46
9
Reliability
41
10
Decision making
41
11
Uncertainty analysis
38
12
Electric power transmission networks
34
13
Climate change
33
14
Risk management
32
15
Accident prevention
31
Table 19 Co-word Analysis through Keyword co-occurrences for string
Table 20 Top keyword clustered into 5 areas of research for string (d)
Cluseter 1
Cluseter 2
Cluseter 3
(25 items) (23 items) (17 items) Distributed energy resources System resilience Risk
Distribution systems System engineering Risk assessment
Electric load flow Resilience metrics Risk management
Electric power systems Resilience model Safety
Electric power transmissions Adaptative capacity Safety engineering
Electric power transmissions networks
Uncertainty analysis Safety management
Electric utilities Recovery Sociotechnical systems
Energy resources Reliability Health
Disasters Sensitivity analysis Accident prevention
Natural disaster Benchmarking Resilience engineering
Extremes events Decision making Resilience systems
Extreme weather Critical
infrastructure
Advanced traffic management systems
Extreme weather events Complex networks Air traffic control
Hurricanes Complex systems Dada envelopment analysis
Storms Bayesian networks Questionnaire
Power system resilience Behavioral research Surveys
System resilience Large scale systems Priority journal
Restoration Information systems
Intelligent systems Infrastructure systems
Investments Stochastic systems
Microgrids Transportation
system
Smart power grid Water supply
Optimization Public works
Monte Carlo methods
Integer programming
Cluseter 4 Cluseter 5
Figure 4 describes the the annual total references published between 2010 and 2021 and the annual growth of publications. The number of references increased considerably. These metrics infer that the annual amount of references published during the survey period was significantly high and stable. A high peak was observed in 2021 with a total of 114 references published. This indicates that this research area was steady in the matter of amount of publications.
Information in Table 19 illustrates the most frequent and relevant keyword co-occurrences on the intellectual domain and as well provide hotspots in this subject-matter. Five clusters was found, clustered into five areas of research as shown in Table 20.
Table 21 shows the most productive-authors as well as the most cited-author references. Azadeh [93] ranked first in term of amount of publications with 22 articles published followed by Hollnagel [94] with 12 articles published . In term of amount of citations, Hollnagel [94] ranked first with 1395 citations, followed by Hosseini [95] with 682 citations.
Information in the Table 22 shows the most productive countries from the perspective of amount of papers published and amount of citations per country. Within the survey period, the United States ranked first with the largest document number (212) and the largest references cited (5825) , followed by the United Kingdom with 65 references cited 1691 times.
Information in Table 23 shows the performance in international collaboration between countries clustered into 6 research groups.
(11 items) (15 items)
Climate change Conceptual framework
Covid-19 Controlled study
Ecosystem resilience Government programs
Resilience Organization
Soial-ecological systems Economics
Sustainability Health care
Sustainability development Health systems
Vulnerability Health care policy
Floods Delivery of health
care
Flood security Health care Delivery
Reliability analysis Patient safety Human Interview Learning
Review
Table 1 Top 10 Authors for string (d)
Most Productive-Authors
Most Cited-Author References
Rank
Author
Documents
Author
Citations
1
Azadeh [93]
22
Hollnagel [94]
1395
2
Hollnagel [94]
12
Hosseini [95]
682
3
Azadeh [93]
12
Henry [96]
456
4
Righi [97]
11
Woods [98]
310
5
Bie [99]
7
Panteli [100]
309
6
Panteli [100]
7
Ayyub [101]
289
7
Ranasinghe [102]
7
Hollnagel [103]
289
8
Woods [98]
7
Li [104]
251
9
Patriarca [105]
6
Bie [99]
231
10
Azadeh [106]
5
Farzin [107]
224
Table 22 Top 10 Most Productive Country for string (d)
Rank
Country
Documents
Citations
1
United States
212
5825
2
United Kingdom
65
1691
3
Iran
64
1386
4
China
54
422
5
Australia
40
789
6
France
38
766
7
Germany
37
285
8
Italy
34
340
9
Canada
31
364
10
Brazil
27
544
Table 23 Country collaborations clustered into 6 research groups for
string (d)
Cluseter 1
(8 countries)
Cluseter 2
(8 countries)
Cluseter 3
(5 countries)
Cluseter 4
(3 countries)
Cluseter 5
(3 countries)
Cluseter 6
(3 countries)
China
Belgium
Australia
Brazil
United States
France
Germany
Denmark
Canada
Norway
Bangladesh
Japan
Netherlands
Finland
India
Sweden
South Korea
Morroco
New Zeland
Iran
Philippines
Nigeria
Italy
Poland
Singapore
Portugal
Switzerland
South Africa
United Kingdom
Spain
It was then necessary to select according to the relevance of the content of the paper in relation to the research. Precisely, papers were integrated in the analysis if they were written in English, they were a journal or conference papers or written by a subject matter expert and their summary specified that the document focuses broadly on strategic approach to asset management and/or decision making. Further analysis was done to identify whether the paper is appropriate or not. Each article content was read to identify whether its subject matter is relevant to our review or not. The selected documents were then exported to the reference management software Endnote. However, all articles were not discussed in depth to keep the length of this paper within acceptable boundaries. Only those articles that are most appropriate and relevant to the review were discussed in detail.
-
-
ASSET MANAGEMENT MODELS
The new normal of the environment of industrial systems characterized by complexity as well as the wide range of industrial automation applications compel organizations to optimize their strategic management economic models for physical assets management. AM decision makers and practitioners have developed numerous tactics and processes to support their competitiveness at the worldwide market. For instance, Baglee [2] points out that a system-wide communication approach is essential to accurately manage the complexity from data gathering, analysis, visualisation and sharing to sustain the development of useful maintenance strategy. These days, executives rely on the explosion of industry 4.0 tools such as IIoT, AI, CPS and Cloud Computing to manage data and make optimal decisions.
AM plays a determining role allowing assets a long-term survival to cope with factors such as the escalating competition, and markets deregulation, as well as technological innovation and risks from other events such as terrorist attacks, natural disasters, major geomagnetic disturbances and cyberattacks [108, 109]. Likewise, a rapid move has been noticed on the range of this research area from managing unfussy repair operations to optimising multiple units which are more complex systems leading to the rise of the concept of multi-unit system [110]. The reader is referred is referred to Petchrompo [110] and their bibliographic references for more details on the insights into the recent trends in multi-asset maintenance. The purpose of this review section is to provide the reader with an overview of key relevant AM models. The leading models of AM lying in the field of interest are summarized in Table 24.
-
The ISO range of standards
The ISO range of standards (55000, 55001 & 55002) builds the basic concepts of a holistic approach to AM with all its implications and purpose. The family of standards in question and the manual for international infrastructure management succeed the PAS 55 guide. Thusly, even if the PAS 55 has become obsolete as a formal specification of the British Standard Institute (BSI) since 2015, organizations may note the existence of a value of this guide as educational material. ISO 55000 standard provides an overview, principles and terminology of AM and Asset Management System and the context for the ISO 5500x series[1]. ISO 55001 specifies requirements for an Asset Management System (AMS) within the context of the organization. It identifies the requirements for the establishment, implementation, maintenance and improvement of AMS [114]. Guidelines relating to the requirements for the application of ISO 55001 are formulated in the ISO 55002 standard [115, 116].
-
The GFMAM: Asset Management Landscape
Subjects
The GFMAM: Asset Management Landscape Subjects: In 2011 a gathering of various experts, academics, and other professionals in the field of AM and Maintenance founded the Global Forum on Maintenance and Asset Management (GFMAM). This landscape contrasts from ISOs themes. The latter do not cover the entire scope of AM knowledge as GFMAM does [117]. The GFMAM published a document bringing together 39 subjects of AM in 6 groupings (themes) describing a section of the body of knowledge which are detailed in the following paragraphs [118]:
-
Strategy and planning theme
Strategy and planning theme seeks to align AM activities with the organization's mission and organizational goals. It deals with: (i) the development of a policy framing the practice of AM within the organization, (ii) the translation of the expectations of the various stakeholders into AM objectives and strategies, (iii) as well as the planning to coordinate activities throughout the asset lifecycle. The Strategy and Planning theme is made up of five subjects (see Table 25):
IJERTV10IS120059
www.ijert.org 70
Acronym
Designation
Main goal
Source
PAS 55
Publicly Available Specification for the optimal management of physical assets
explicitly focussed on the optimal management of physical assets
British Standard Institute (BSI) (2002, 2004, 2008)
ISO 55000
Asset management – Overview, principles and terminology
specifies basic concepts of a holistic approach to AM
International Organization for Standardization, ISO (2014)
ISO 55001
Asset management – Management systems – Requirements
is a standard for ANY asset type; it defines the requirements for an asset management system within the context of the organization
International Organization for Standardization, ISO (2014)
ISO 55002
Asset management – Management systems – Guidelines for the application of ISO 55001
provides interpretation and implementation guidance for such a management system.
International Organization for Standardization, ISO (2018)
ISO 55010
Guidance on the alignment of financial and
non-financial functions in asset management
enables better understanding why and how alignment between financial and non-financial functins is crucial in realizing value from assets.
International Organization for Standardization, ISO (2019)
The GFMAM
Asset Management Landscape
The Global Forum on Maintenance & Asset Management, GFMAM Landscape of subjects
promote a common global approach to Asset Management and Maintenance professions by collaborating on knowledge, standards and practices. 39 AM Subjects and Principles and a framework for describing best practices, maturity and standards
The Global Forum on Maintenance & Asset Management, GFMAM (2011)
The IAM Conceptual AM model
The IAM Asset Management – An Anatomy
is a framework for assessing maturity in AM. This framework is aligned with the principles of ISO 55000 series of standards. It is made up of 39 topics that detail the AM activities within an organization.
The Institute of Asset Management (IAM) (2015)
CIGRE
conceptual decision-making process for AM
International Council on Large Electric Systems (Conseil international des grands réseaux électriques, CIGRÉ) decision making decision making processus
is the Asset Management of Transmission and Distribution business operating in an electricity market involves the central key decision making for the network business to maximize long term prots, whilst delivering high service levels to customers, with acceptable and manageable risks [111, 112].
International Council on Large Electric Systems (Conseil international des grands réseaux électriques, CIGRÉ) (1921)
AMBoK
Asset Management Body of Knowledge (AMBoK)
provides a collection of models (strategic, operational, tactical, maturity) and definitions forming a comprehensive framework of AM practices
Australian Asset Management Council (AM-Council) (2014)
EFNMS-Bok
European Federation of National Maintenance Societies (EFNMS) Conceptual Model
is a conceptual model of AM based on continuous improvement approach PDCA (Plan Do Check Act); it provide the body of knowledge (Bok) describing the maintenance landscape and the knowledge required
The European Asset Management Committee, EAMC (2018)
RIDM
The Risk-Informed Decision-Making (RIDM) process
These are structured processes which assist decision- makers when faced with high impact, complex decisions involving multiple objectives and the presence of uncertainty. They aim to ensure that decisions between competing alternatives are taken with an awareness of the risks associated with each option, and that all attributes of a decision are
considered in an integrated manner [113].
The National Aeronautics and Space Administration , NASA and the US Nuclear Regulatory Commission (2008, (2010)
Acronym
Designation
Main goal
Source
PAS 55
Publicly Available Specification for the optimal management of physical assets
explicitly focussed on the optimal management of physical assets
British Standard Institute (BSI) (2002, 2004, 2008)
ISO 55000
Asset management – Overview, principles and terminology
specifies basic concepts of a holistic approach to AM
International Organization for Standardization, ISO (2014)
ISO 55001
Asset management – Management systems – Requirements
is a standard for ANY asset type; it defines the requirements for an asset management system within the context of the organization
International Organization for Standardization, ISO (2014)
ISO 55002
Asset management – Management systems – Guidelines for the application of ISO 55001
provides interpretation and implementation guidance for such a management system.
International Organization for Standardization, ISO (2018)
ISO 55010
Guidance on the alignment of financial and
non-financial functions in asset management
enables better understanding why and how alignment between financial and non-financial functions is crucial in realizing value from assets.
International Organization for Standardization, ISO (2019)
The GFMAM
Asset Management Landscape
The Global Forum on Maintenance & Asset Management, GFMAM Landscape of subjects
promote a common global approach to Asset Management and Maintenance professions by collaborating on knowledge, standards and practices. 39 AM Subjects and Principles and a framework for describing best practices, maturity and standards
The Global Forum on Maintenance & Asset Management, GFMAM (2011)
The IAM Conceptual AM model
The IAM Asset Management – An Anatomy
is a framework for assessing maturity in AM. This framework is aligned with the principles of ISO 55000 series of standards. It is made up of 39 topics that detail the AM activities within an organization.
The Institute of Asset Management (IAM) (2015)
CIGRE
conceptual decision-making process for AM
International Council on Large Electric Systems (Conseil international des grands réseaux électriques, CIGRÉ) decision making decision making processus
is the Asset Management of Transmission and Distribution business operating in an electricity market involves the central key decision making for the network business to maximize long term prots, whilst delivering high service levels to customers, with acceptable and manageable risks [111, 112].
International Council on Large Electric Systems (Conseil international des grands réseaux électriques, CIGRÉ) (1921)
AMBoK
Asset Management Body of Knowledge (AMBoK)
provides a collection of models (strategic, operational, tactical, maturity) and definitions forming a comprehensive framework of AM practices
Australian Asset Management Council (AM-Council) (2014)
EFNMS-Bok
European Federation of National Maintenance Societies (EFNMS) Conceptual Model
is a conceptual model of AM based on continuous improvement approach PDCA (Plan Do Check Act); it provide the body of knowledge (Bok) describing the maintenance landscape and the knowledge required
The European Asset Management Committee, EAMC (2018)
RIDM
The Risk-Informed Decision-Making (RIDM) process
These are structured processes which assist decision- makers when faced with high impact, complex decisions involving multiple objectives and the presence of uncertainty. They aim to ensure that decisions between competing alternatives are taken with an awareness of the risks associated with each option, and that all attributes of a decision are
considered in an integrated manner [113].
The National Aeronautics and Space Administration , NASA and the US Nuclear Regulatory Commission (2008, (2010)
Table 24 summary of relevant models developed in physical asset management (Source: Own representation)
Table 25 Strategy and planning
Asset Management Policy subject analyzes the principles and requirements that flow from the Strategic Asset Management Plan (SAMP) and that guide the organization's AM activities. This topic analyzes the organization's AM policy, which ensures management commitment and provides a framework for the development and iplementation of AM objectives and strategies. Politics is a key component of the AM approach. It serves as a catalyst for a change in culture and in the way of doing things and as a starting point to lead the development of the AM strategy. If the policy is not communicated or understood throughout the organization, it is difficult to align asset management activities with organizational objectives and to achieve the expected results of the process.
Asset Management Strategy and Objectives subject deals with the SAMP which directs the organization's AM activities and describes the long-term planning approach that will be taken. It analyzes current and future AM capabilities and supports the alignment between organizational goals and AM objectives and describes the processes and strategies to be adopted to achieve them.
Demand Analysis subject analyzes the procedures used to assess the demand for assets and the organization level of service. This subject looks at the historical and future demand for the service as well as its evolution over time. It analyzes demand requirements and their impact on the asset portfolio and its capacity.
Strategic Planning subject discusses the procedures used by the organization to implement the SAMP. It assesses the development of a strategic planning framework and process that describes how demand analysis and required service
Table 26 Asset management decision-making
IJERTV10IS120059
www.ijert.org 71
levels are factored into and modeled in business development.
Asset Management Planning subject assesses activities aimed at developing Asset Management Plans (AMPs) that describe the condition of assets and specify the tasks, timelines, responsibilities, and resources required to achieve AM objectives. It also examines the financial strategy and the lifecycle management strategy as well as the risks associated with the planning and implementation of activities.
Lifecycle Delivery theme characterizes how the organization carries out the selected projects, operates and maintains the assets to ensure the production of an expected level of service. It first establishes the strategies developed and AMP and then frames the activities and risks associated with the asset lifecycle (acquisition, operation, maintenance, and disposal). Lifecycle Delivery theme consists of 11 subjects as shown in Table 27.
-
Asset management decision-making theme
the
Technical Standards and Legislation subject encompasses procedures used in an organization to ensure that its AM
Asset management decision-making theme helps identify processes for informed decision making that maximize value in different phases of the asset
lifecycle. It examines the processes that assess the impact and criticality of the various choices of acquisition, operation, maintenance, and disposal of assets in terms of performance, risks, and associated costs. This
theme involves five subjects as shown in Table 26.
Capital Investment Decision Making subject deals with the procedures and decisions to assess and analyze the organization's capital investment scenarios. These procedures and decisions may relate to new assets acquisition or replacements of end-of-life assets.
Operation and Maintenance Decision Making subject covers the management activities and procedures that go into determining the requirements for operation and maintenance decision-making to support AM objectives. This subject assesses the various maintenance strategies (predictive, preventive, corrective, etc.) and their correlation with the level of criticality of the assets and analyzes the alignment of these strategies with the optimal delivery of the service. It would be relevant to align O&M decision making with the objectives of AM. Maintenance strategies should be reviewed considering their impact on service delivery.
Lifecycle Value Realisation subject focuses on the activities performed by an organization to assess the various intervention options required to upgrade assets during their lifecycle (maintenance, replacement, decommissioning, etc.). This subject analyzes assessment models that are based on a comparison of the cost and benefit ratio of options and a comparison of the total lifecycle value.
Resourcing Strategy subject examines the processes and activities that an organization must implement to optimize the use of human resources, facilities, tools, and materials to achieve AM goals. It analyzes the strategy for acquiring resources and their integration across the organization to implement Asset Management Plans (AMP).
Shutdowns and Outage Strategy subject assesses activities carried out by the organization to develop a strategy to manage breakdowns and various types of asset shutdowns (for e.g.: periodic maintenance shutdowns). It involves procedures and requirements needed to ensure a robust agreement between all concerned players. This allows to reduce the impact on the service level of the organization (reduce wasted time, downtime, etc.).
-
Lifecycle Delivery theme
activities comply with applicable legislation and technical standards.
Table 27 Lifecycle Delivery
Asset Creation and Acquisition subject assesses the organizational processes that frame the first phase of the life cycle, that is, the definition of requirements, acquisition, installation, and commissioning of assets. It ensures that the choices and selection of new options for operability, maintainability and resource sustainability are based on the value of the entire life cycle of the asset.
Systems Engineering subject analyzes engineering processes so that they follow an interdisciplinary and collaborative approach to analyze needs, design, and evaluate assets. It refers to the policies and procedures for requirements analysis, design, and assessment of assets.
Configuration Management subject covers configuration management procedures and asset functionality review and monitoring processes. It examines how to adapt equipment to achieve better functional consistency and optimal performance throughout the life cycle.
Maintenance Delivery subject deals with the management of AM activities including methodologies for managing inspections and preventive and corrective maintenance carried out on assets.
Reliability Engineering subject frames studies to ensure that an asset will operate in accordance with a specified standard, for a given duration and in a defined environment. It investigates the organization's reliability plans and requirements and performance requirement compliance processes.
Asset Operations subject describes the procedures an organization uses to leverage its assets to achieve business objectives. It ensures that operator instructions are in accordance with defined parameters for design, maintenance, and operation.
Resource Management subject assesses the implementation of organizational strategies aimed at managing the use of funds, labor, facilities, tools, and materials in the performance of AM activities. It also covers the efficiency of the resource management process, its coordination and integration within the organization.
Shutdown and Outage Management subject deals with the application of the processes that an organization uses to
IJERTV10IS120059
www.ijert.org 72
identify, plan, schedule, execute and control work related to the different types of asset shutdowns.
Fault and Incident Response subject analyzes the systematic response to failures and incidents including incident detection and identification, failure analysis, temporary and permanent repairs, etc.
Asset Decommissioning and Disposal subject discusses the procedures an organization uses to decommission and retire assets due to aging or changes in performance and capacity requirements. It ensures that asset retirement is planned as part of the life cycle.
-
Asset Information theme
Asset Information theme aims to develop an in-depth knowledge of the asset portfolio (condition, criticality, capacity, etc.) which serves as an input and a key element in various AM processes. It studies the availability, reliability, and accessibility of relevant information on which the quality of decisions in operation, maintenance, financing, and risk management is based. The theme consists of 4 subjects as shown in Table 28.
Table 28 Asset Information:
Asset Information Strategy subject deals with the necessary strategic approach to defining, collecting, managing, communicating and general governance of asset information to support the implementation of the objectives and the AM strategy. It checks the alignment of the information management strategy with the Strategic Asset Management Plan (SAMP) and analyzes the information needs and requirements related to assets.
Asset Information Standards subject assesses the establishment of a coherent structure and format intended to collect and store information relating to assets and report on its quality and accuracy. It examines the standards developed to adopt an approach to recording and classifying information aligned with the asset reporting strategy.
Asset Information Systems subject focuses on the information systems an organization puts in place to support AM activities and decision-making processes in accordance with the asset information strategy. It validates whether the architecture in place processes and supports information relating to the life cycle of assets and whether it ensures the centralization of data and its accessibility to the various stakeholders.
Data and Information Management subject analyzes data and information held by an organization within asset information systems and the management and governance procedures. It examines the various processes and plans that frame the management and coordination of the information lifecycle.
-
Organization and People theme
Organization and People theme looks at the organizational structure and culture, roles, responsibilities, and key skills
related to AM. It examines the adoption of AM thinking among senior management and employees, as well as the alignment of their efforts and behaviors with best practices. Organization and People theme consists of five subjects as shown in Table 29.
Table 29 Organization and People
Procurement and Supply Chain Management subject deals with the procedures used by an organization to ensure that all outsourced AM activities are aligned with AM objectives and to monitor the results of these activities. It reviews the activities required to create, manage, and maintain relationships with service providers, suppliers, and contractors.
Asset Management Leadership subject assesses the leadership required in an organization to promote a lifecycle approach to AM with the goal of maximizing value and serving the purpose of the organization.
Organizational Structure subject verifies whether the organizational structure promotes the achievement of the organization's mission and AM objectives, within the framework of a multidisciplinary collaborative approach. It analyzes the roles and responsibilities, interdependencies and information flows between different departments and functions.
Organizational Culture subject discusses the culture of an organization in relation to its ability to achieve organizational goals. It assesses the values shared in the organization, behaviors, perceptions, rules, and societal influences.
Competence Management subject discusses the processes an organization uses to consistently develop and maintain a skilled workforce and board of directors who are motivated to achieve AM goals to meet current and future needs. This subject assesses training and evaluation programs for operational skills, thinking and analysis skills, programs for developing knowledge in AM and understanding of the activities to be carried out through the. life cycle.
-
Risk and Review theme
Risk and Review theme is an important influencer of themes that cover strategic planning and decision making in AM. It deals with the organization's sensitivity to asset failures and the level of resilience of the various business sectors associated with them. It addresses several basic activities including the identification of risk management, determination of effective feedback and review mechanisms to provide assurance that objectives are achieved, knowledge of the physical condition and performance of assets, continuous improvement of AM activities. Furthermore, this theme covers the performance and reliability of the Asset Management System (AMS) ensured by the feedback and monitoring processes. The Risk and Review theme consists of nine subjects as shown in Table 30.
Table 30 Risk and Review
IJERTV10IS120059
www.ijert.org 73
Risk Assessment and Management subject covers the policies and procedures for identifying, quantifying, and mitigating risks and exploiting opportunities. Risk Assessment and Management is common to all the themes of the AM conceptual model. This subject validates its integration in strategy, in AM Planning as well as in the implementation of the various phases of the life cycle.
Contingency Planning and Resilience Analysis subject assesses the procedures and systems put in place by an organization to ensure, in the event of unforeseen events, the continuity of operation of assets to provide the required level of service. It validates whether in critical moments the organization is prepared to respond well and make appropriate decisions based on contingency plans and proven scenarios.
Sustainable Development subject analyzes the multidisciplinary and collaborative processes that an organization uses to ensure a sustainable and balanced approach to its economic activity, its environmental responsibility, and its social progress, with a view to ensuring that all its activities are sustainable in the long run.
Management of Change subject deals with an organization's procedures for identifying, evaluating, implementing, and communicating changes affecting people, processes, and assets. It addresses the management of risks and opportunities associated with changes that may affect the achievement of AM objectives and measures to mitigate their impact.
Assets Performance and Health Monitoring subject inspects the processes and metrics an organization uses to measure the condition and performance of its assets. It analyzes the compliance of asset lifecycle assessment and monitoring programs against performance goals.
Asset Management System Monitoring subject covers the procedures and measures an organization uses to assess the performance of its AMS. It analyzes the monitoring program of this system and evaluates its performance.
Management Review, Audit and Assurance subject discusses an organization's processes that review and audit the effectiveness of its procedures and AMS. It examines the internal audit, control policies and plans as well as the reports of non-conformities and the resulting corrective measures.
Asset Costing and Valuation subject covers the processes used to define and capture the costs of building, maintaining, and renewing assets. Also included are the methods used to assess the value and depreciation of assets. This topic analyzes the framework that defines the composition of all costs associated with managing the lifecycle of assets and estimating and forecasting their value, considering changes in costs over their useful life.
Stakeholder Engagement subject assesses the methods an organization uses to foster stakeholder engagement in AM. It reviews policies and procedures for mapping stakeholders and their expectations as well as communicating and interacting with them.
-
-
The IAM Conceptual AM model
The institute of asset management (IAM) has developed a conceptual model for AM including the six groups of themes covering the 39 above-mentioned themes of AM initially
published by the GFMAM [6, 118]. The IAM Asset Management – An Anatomy is a framework for assessing maturity in AM. This framework is aligned with the principles of ISO 55000 series of standards. It is made up of 39 topics that detail the AM activities within an organization. These 39 subjects are grouped into 6 themes. This demonstrates the scope of AM, the interrelationship between multiple activities and the need to integrate them, as well as the importance of alignment with the objectives and strategy of the organization. Figure 5 depicts the IAM model for the management of physical asset including the 6 subjects groups.
Figure 5 Conceptual AM model [6]
The maturity levels used to qualify the organization's level of progress in the management of physical assets are based on definitions adopted by IAM. Figure 6 shows the path through excellent AM practices. The maturity rating is measured on a scale of 0 to 5, whereby level 3 corresponds to the qualified degree of "Competence". The latter corresponds to the certification requirements of the ISO 55001 standard [114]. This level means that processes are in place for AM activities, that they are well documented, communicated and controlled across the organization, and that they are delivering the expected results.
Figure 6 IAM Asset Management Maturity Scale 5 (Source: The Institute of Asset Management (IAM))
It is common to observe a level of maturity of "Awareness" among organizations that perform an initial assessment of their AM practices. This step is often a starting point on the organization's journey towards best AM practices,
which is a continuous and evolving process based on continuous improvement.
-
Asset Management Body of Knowledge (AMBoK)
In 2014, the australian asset management council (AM- Council) developed a compilation of best practices known by the acronym AMBoK constituting a comprehensive AM framework [119]. AMBoK provides a collection of models (strategic, operational, tactical, maturity) and definitions forming a comprehensive framework of AM practices. Figure 7 illustrates the different models developed and their levels of involvement in AM.
Figure 7 Asset Management Body of Knowledge collection of models (AMBoK)
(Source: Own representation based on Asset Management Council [119])
CIGRE conceptual decision-making process for AM
The international council on large electric systems, CIGRÉ (Conseil international des grands réseaux électriques, CIGRÉ) is a world-wide nonprofit professional organization in the field of high voltage electricity initiated in 1921 in France. Thusly, in Electrical Utilities, CIGRE characterize AM as follows:The Asset Management of Transmission and Distribution business operating in an electricity market involves the central key decision making for the network business to maximize long term prots, whilst delivering high service levels to customers, with acceptable and manageable risks [111, 112].
CIGRÉ has developed a conceptual model of decision- making for AM (Asset Management Decision Process) comprising three levels of information requirements: Corporate Level, Network Level, and Component Level. Figure 8 illustrates the conceptual decision-making process for AM in the power sector according to CIGRE [120, 121]. Indeed, the process shows the different levels of information requirements for decision making in a Risk Management Regime.
Figure 8 Conceptual Model of Decision-Making for Asset Management (Source: [122])
* Note: S3 stands for Safety, Social, and System Oriented Risks.
-
-
RISK MANAGEMENT AND DECISION-MAKING PROCESS IN ASSET MANAGEMENT
This section provides the reader with an overview of key relevant background aspects of the concept of a research component of major interest in the scientific community namely the concept of risk management (RM) and decision- making process in asset management at all levels such as risk- informed decision making (RIDM) and risk-informed asset management (RIAM).
-
Risk and risk assessment
International Organization for Standardization [123], International Organization for Standardization [124] standards define risk management (RM) as coordinated activities to direct and control an organization with regard to risk. Furthermore, Hubbard [125] describes RM as the identification and evaluation as well as prioritization of risks in addition to the coordination to either increase the fulfilment of opportunities or reduce the likelihood or effect of tragic events. Risk is characterized as an effect of uncertainty on objectives[123, 124]. An effect is a deviation from the expected. It can be positive, negative or both, and can address, create, or result in opportunities and threats. Risk is frequently stated in the matter of risk sources, potential events, their consequences and their likelihood [123, 124]. To rephrase it, risk can be characterized as the likelihood that an event will happen that unfavorably disturbs the realization of an organizational goal. Operationally, NASA procedural requirement NPR 8000.4 expresses risk as a set of three components: (i) the scenario(s), (ii) the likelihood(s), and (iii) the consequence(s) as shown in Figure 9 [126]. The latter authors mention that identifying risk in this manner helps RM since: (i) it distinguishes high-probability, low-consequence outcomes from low-probability, highconsequence outcomes,
-
it points the way to proactive risk management controls, for example by supporting identification of risk drivers and the screening of low-probability, low-consequence outcomes; and
-
It can point the way to areas where investment is warranted to reduce uncertainty.
Figure 9 risk defined as a set of triplets
(Source: Own representation based on NASA/SP-2010-576 International Organization for Standardization [123])
In other words, it can be characterized as the resultant of an organization setting of objectives and priorities as well as actions to be undertaken against uncertainties of the environment such as uncertainty in worldwide markets deregulation, legal liabilities, technological innovation and risks from other events such as terrorist attacks, natural disasters, major geomagnetic disturbances and cyberattacks [108, 109].
Accordingly, uncertainties are a key aspect of risk, they are integrated in the assessment of likelihoods and
Figure 10 Global RM process in AM
(Source: Own representation based on International Organization for Standardization [123])
consequences. The uncertainty occurs from various sources including internal and external factors and impacts that can be sources of failure or delay attaining the corporate objectives [123, 124, 127, 128]. RM should include these subsequent
principles (Figure 10).
Thusly, ISO 31000 standard points out that managing risk is iterative and assists organizations in setting strategy, achieving objectives and making informed decisions. Studies show that RM is gradually considered as a means of enhancing the probability of achievement in strategic asset management and should be an integral component of all activities of an organization and its stakeholders [126, 129, 130]. The outcomes of the risk assessment and uncertainty analysis provide asset managers and practitioners with an unmistakably informed picture of the matter and helpful assistance when taking decisions to reason and deliberate in an assured manner [4, 108, 113, 131-135]. One can utilize risk acceptance criteria (RAC) methodology to assess whether the risk level is tolerable or not, through risk analysis and risk evaluation, as well as risk reduction and risk management, particularly while the consequence has a meaningful societal impact. The reader is referred to Marhavilas [136] and their bibliographic references for more details on RAC.
The following section addresses the risk-informed decision-making process (RIDM) which is an esential component of RM.
-
-
Risk-Informed Decision-Making process
The concept of risk-informed decision-making (RIDM) was established by the United States nuclear engineering industry in connection with safety concerns that come with nuclear power, and the Aerospace industry (the National Aeronautics and Space Administration (NASA) and the US Nuclear Regulatory Commission(USNRC)) [137].
Conventionally, risk management (RM) was deemed equivalent to continuous risk management (CRM). Traditionally, to perform Risk Assessment and Uncertainty Analysis, asset practitioners has used probabilistic analysis (known as Probabilistic Risk Assessment, PRA, or Quantitative Risk Assessment, QRA). Making decision involves numerous inputs which are very diverse in nature (qualitative inputs as the expert judgment, and quantitative as the risk assessment). Today, RM is labeled as encompassing both CRM process, and risk-informed decision-making (RIDM) process [126, 138, 139]. Proactive RM should combine RIDM (which focuses on the risk informed selection
of decision alternatives to ensure successful methods to attaining objectives) and CRM (which tackles the execution of the chosen alternative to ensure that requirements are encountered). RIDM and CRM are complementary processes and should be combine into a single framework to enables effective and useful RM. Now, the trend is moving from a risk-based toward a risk-informed safety management approach wherein the perceptions given by the PRA (or QRA) and the deterministic method is treated as fundamental element of a decision-making process [4, 108, 113, 140, 141]. This approach is denoted risk informed decision-making (RIDM) when it is utilized for decisions-making process in relation to safety concerns [138]. In the same vein, Zio [113] remark that quantitative outcomes of risk assessment are only one component of the decision-making process, being combined with other criteria (such as social preferences, political concerns and budgetary constraints). Dezfuli [126] draw attention to the fact that technical information cannot be the only basis for decision making. The latter authors point out that human judgment plays a key role in decisions. Likewise, Komljenovic [4] point out that Risk Assessment is just a portion of the complete RIDM process. Indeed, to be fruitful, RIDM embraces analyses in a broader scale such as engineering, risk, economic, societal, environment, regulatory.
NASA risk management procedural requirements NPR 8000.4 encompasses three parts broken down into six various process steps that constitutes the risk-informed decision- making (RIDM) process (NASA/SP-2010-576) as depicted in the following chart (Figure 11).
Figure 11 Global RIDM process
(Source: Own representation based on NASA/SP-2010-576 International Organization for Standardization [123])
Part 1 identifies decision alternatives (recognizing opportunities) in the context of objectives. Part 2 performs risk analysis (integrated perspective) and development of the technical basis for deliberation. Part 3 deliberates and selects an alternative and associated performance commitments informed by (not solely based on) risk analysis.
Zio [113] conducted a study overviewing and describing in detail, including an assessment of commonalities and
differences in methodology, the RIDM processes developed by the NASA and the USNRC. This analysis provides the overall concepts, denitions and issues associated with the application of RIDM methods. It also provides reasons for the use of these methods as a complement to more conventional and established deterministic approaches to risk assessment. According to these authors, these are a structured processes which assist decision-makers when faced with high impact, complex decisions involving multiple objectives and the presence of uncertainty. They aim to ensure that decisions between competing alternatives are taken with an awareness of the risks associated with each option, and that all attributes of a decision are considered in an integrated manner. Komljenovic [132], Komljenovic [133] suggested an approach to conduct RIDM process suitable for immense projects
Figure 12 Global RIDM process in AM
(Source: Own representation based on Komljenovic [133])
dealing with Strategic Asset Management aspects, such as long-term performance and sustainability as follow and shown in Figure 12: (i) establish the decision-making framework, (ii) perform detailed analyses, and (iii) deliberate and make final decisions. However, the authors mentioned that this process is not useful for a day-to-day decision-making.
Today, this tool applies to other industries, such as aerospace and infrastructure safety such as aerospace and dam safety and provide useful decision support to decision-makers [108, 126, 129].
-
Risk-Informed Asset Management (RIAM) process
The concept of risk-informed asset management process (RIAM) is a risk-based decision analysis tool, initially developed for nuclear power stations, suitable for use in a market-driven industry. Simply put, the RIAM process provides plant executives with an instrument from planning
Figure 13 Risk-Informed Asset Management process (RIAM) (Source: [142])
project priorities and lifecycle management to developing long-term maintenance plans, budgeting, and awareness of economic risks, as well as the reliability and availability of the systems. Figure 13 depicts at a high-level, the conceptual model of RIAM resting upon the technical report of the development plan of the Electric Power Research Institute (EPRI) [142]. RIAM method is an additional method to the Nuclear Asset Management (NAM) method of managing nuclear assets. The latter uses risk management and defines business objectives to support investment decision-making in a long-term planning horizon throughout the life cycle of assets [142].
The RIAM process establishes several profitability models and performance metrics or decision support metrics (cost, safety, revenue, scheduling, etc.) to assist decision-
makers in resources allocation and optimization. Hence, the RIAM process is as well a performance-based concept [143, 144].
Furthermore, Komljenovic [145] introduced the concept of a holistic risk-informed, performance-based asset management in mining (RIPBAMM) as a
complementary tool to the current strategic mining activities. It is worth emphasizing that the latter author was the first to recommend the RIPBAMM
process in further expanding the RIDM process in the mining sector.
Figure 14 risk-informed, performance-based asset management in mining (RIPBAMM)
(Source Komljenovic [145])
The concept should provide key performance indicators to help asset management practitioners in key decision-making processes by using a combination of mathematical modelling and probabilistic quantification. The benefits of RIPBAMM process lies on the long-term profitability through continuous improvement on decision-making which contribute to enhance
the net present value (NPV) of the mining industry. Figure 14 depicts the 12 modules at a high-level flow diagram of the RIPBAMM process proposed by the latter author including the key mine activities and performance models
-
-
RESILIENCE ENGENEERING
-
General concept of resilience
Asset management plays a key role for assets to cope with
Figure 15 hierarchy of organizational types of ecosystems (Source: Own representation based on Komljenovic [146])
Table 31 definitions of resilience (Source: Own representation)
the external and internal organizational environment such as the ever-increasing competition, and markets deregulation, as well as technological innovation and risks from other events such as terrorist attacks, natural disasters, political environment, major geomagnetic disturbances and cyberattacks [108, 109]. Figure 15 depicts the hierarchy of these types of environments. The external environment rmains neither correctly foreseeable nor accurately controllable or influenceable due to its complexity and profound uncertainty [146].
To cope with this kind of environment, resilience management is a well-known approach suggested in RM and uncertainties analysis. A broad panoply of definitions of resilience arises from the scientific literature [95].
Table 31 depicts a no exhaustive directory of those definitions of resilience found in the scientific literature in relevant sectors. This variety in defining the resilience concept can lead to complexities in interpreting and measuring resilience [96]. The several distinctions and understandings of the concept of resilience are determined by which aspect of the subject matter of a resilient system is under study [162- 164]. Vugrin [152] mentioned a broad range of interrelated domains for assessing system resilience: (i) technical, (ii) organizational, (iii) social, (iv) economic, (v) ecological and
(vi) environmental. For e.g., Resilience engineering is a paradigm which has been promoted as a modern safety management concept to cope with complex socio-technical
Discipline
Authors / Paper
Definition
Resilience engineering
Association [147] / Resilience
Resilience Engineering is a trans-disciplinary perspective that focuses on developing on theories and practices that enable the continuity of operations and societal activities to deliver essential services in the face of ever-growing dynamics and uncertainty. It addresses complexity, non-linearity, inter-dependencies, emergence, formal and informal social structures, threats and opportunities.
Fairbanks Fairbanks [148] / Resilience and resilience engineering in healthcare
Resilience engineering is the deliberate design and construction of systems that have the capacity of resilience
Hollnagel [149] / Resilience engineering in practice
The intrinsic ability of a system to adjust its functioning prior to, during, or following changes and disturbances, so that it can sustain required operations under both expected and unexpected conditions.
Woods [150] / Creating foresight: how resilience engineering can transform NASAs approach to risky decision making.
Resilience engineering uses the insights from research on failures in complex systems, including organizational contributors to risk, and the factors that affect human performance to provide systems engineering tools to manage risks proactively
Resilience in infrastructure systems
Resilience [151] / The National Infrastructure Advisory Council, Resilience, Critical Infrastructure.
The ability to reduce the magnitude, impact, or duration of a disruption.
Vugrin [152]/ A framework for assessing the resilience of infrastructure and economic systems.
Given the occurrence of a particular disruptive event (or set of events), the resilience of a system to that event (or events) is the ability to efficiently reduce both the magnitude and duration of the deviation from targeted system performance levels.
Resilience in organisational systems
International Organization for Standardization [153]
The ability of an organization to absorb and adapt in a changing environment to enable it to deliver its objectives and to survive and prosper.
[154] / Defining resilience. Resilience describes the characteristic of managing the organisation's activities to anticipate and circumvent threats to its existence and primary goals.
Denyer [155] / Organizational resilience: a summary of academic evidence, business insights and new thinking
The ability of an organisation to anticipate, prepare for, respond, and adapt to incremental change and sudden disruptions in order to survive and prosper
Lexicon [156] / U.S. Department of Homeland Security Risk Steering Committee
The ability to resist, absorb, recover from or successfully adapt to adversity or a change in conditions:
OrtizdeMandojana [157] / The long-term benefits of organizational resilience through sustainable business practices.
The ability of organizations to anticipate, avoid, and adjust to shocks in their environment.
Home III [158]./ Assessing Behaviors that Create Resilient Organizations.
The ability of a system to withstand stresses of environmental loading
Resilience in socio- ecological systems
Holling [159] / Resilience and stability of ecological systems
A measure of the persistence of systems and of their ability to absorb change and disturbance and still maintain the same relationships between populations or state variables
Walker [160]/ Resilience,
adaptability and transformability in social- ecological systems.
The capacity of a system to absorb disturbance and reorganize while undergoing change so as to still retain essentially the same function, structure, identity, and feedbacks
Resilience in
environmental systems
Tierney [161] / Conceptualizing and Measuring
Resilience
Both the inherent strength and ability to be flexible and adaptable after environmental shocks and disruptive events.
-
ability of systems, infrastructures, government, business, and citizenry to resist, absorb recover from, or adapt to an adverse occurrence that may cause harm, destruction, or loss of national significance
-
capacity of an organization to recognize threats and hazards and make adjustments that will improve future protection efforts and risk reduction measures
systems [165, 166]. It is associated with the integrity and reliability of physical infrastructure systems. In contrast, even though there exists some common ground with Resilience engineering, Socio-ecological resilience can be referred to as environmental and ecological concerns, security concerns, disaster response, business continuity, OHS, etc. [164]. Four common principles emerge from the literature review as shown in the following chart (Figure 16). These characteristics involves surviving change, either by lowering the effect of the disruption or adapting to it or recovering from it. It is worth emphasizing that the speed of the recovery is an important aspect of the resilience concept. Successful organizations are more resilient to predict and react to threats and opportunities in a complex and shifting environment.
Figure 16 four common principles of resilience (Source: Own representation)
It is worth emphasizing that resilience as an approach to deal with complexity and uncertainties should not be considered at the component level, nevertheless, should be view as an attribute at the system level to withstand severe conditions.
The general concept of resilience is depicted in the following chart (Figure 17).
Figure 17 General concept of resilience (Source: Komljenovic [167], Kurth [168])
It implies four phases: (i) Planning this phase prepares and anticipates the arrival of an adverse event, (ii) Absorption this phase implies the amount of loss of performance while an adverse event occurs, (iii) Recovery this phase regains the performance after an adverse event occurs. The length of time to fully recovery following an adverse event is much longer if the magnitude of performance loss is larger or the required resources to recover the damage are unavailable. According to these, several recovery shapes are possible. (iv) Adaptation this phae gathers information and acquires experiences on the adverse event and continue to improve the system resilience.
Resilience entails a persistent continuous improvement and adaption as many aftermath performance levels are likely after recovery.
This general concept of resilience entails four main properties: (i) Robustness (the capacity to absorb a shock, to withstand critical functions, to survive after an adverse event: namely absorbing and adapting), (ii) Redundancy, (iii) Resourcefulness (the capacity to plan for and make ready to withstand a disruption), and (iv) Rapid recovery (the capacity to quickly return to operation and efficiently following an accident) [164, 167].
To cope with these complexity and uncertainties challenges, for e.g., Komljenovic [167] has proposed a holistic methodology in managing the closure and abandonment of
Figure 18 holistic approach in managing the closure and abandonment of trailing ponds
(Source: Komljenovic [167])
trailing ponds as shown in Figure 18.
The proposed approach combines four blocks of functions and activities as shown in Figure 18 namely: (i) operation management; maintenance, monitoring and inspection management, (ii) General management, risk, and emergency management, return of experience and lessons learnt, (iii) establishing an adequate equipment and system design criteria; R&D and innovation, (iv) the fourth element relates to the legal and regulatory framework, which are appropriate to the four phases of the resilience idea. This study fosters a culture of anticipatory and preventive actions by recommending maintaining in all these activities an overall good performance to withstand severe conditions.
Finally, resilience as an approach to cope with complexity and uncertainties is a newly coined word and concept that has been established in the last decades to tackle mainly complexity of industrial systems and critical infrastructures involving the subsequent dimensions of resilience: organizational, technical/technological, operational, social, economic, financial, reputational, and business model [98, 167, 169, 170].
-
-
-
STRATEGIC ASPECTS OF ASSET MANAGEMENT AND
MAINTENANCE 4.0
The purpose of this literature review section is to provide the reader with an overview of key relevant background aspects of Strategic Asset Management (SAM) and Industry
4.0 digital technology with a special emphasis on maintenance
4.0 tools.
SAM aims on aligning AM strategies with various levels of organizational strategy: corporate level strategy, business level strategy, and functional-level strategy. Figure 19 depicts the three levels of strategy pyramid:
Corporate level strategy: as a whole, this level of organisation establishes the strategic objectives for the organization. It gives direction to the business level strategy, and the
Figure 19 three levels of strategy pyramid
(Source: Own representation based on the literature)
functional-level strategy for development endeavors.
Business level strategy establishes the strategic objectives for the business unit. This level of organisation is informed by the corporate objectives.
Functional-level strategy establishes the strategic objectives to deliver on the organizational objectives and to keep on improving the functional-level strategy. This level of organisation is informed by the corporate objectives and the business strategic plans to establish a strategy to respond to those demands.
Asset management professionals will have to operate systems and infrastructures as well as decision-making processes at all levels of organizational strategy made up of a panoply of complex and uncertain technological objects including capital investment, definition of requirements, acquisition, installation, and commissioning and decommissioning of assets (O&M), shutdown and outage strategies, life cycle value realisation, etc. These systems and infrastructures are mostly complex, critical, and characterised by unpredictability relating the subsequent dimensions of resilience: organizational, technical/technological, operational, social, economic, financial, reputational, and business model [98, 167, 169-172]. Faced with this situation, AM constitutes a research element of major interest in the scientific community who is increasingly interested in SAM. The latter starts to be recognized as a basis of viable competitive advantages in the uncertain environment of enterprises.
In a wide variety of sectors, integrated risk management is gaining in importance. For instance, in the electrical power industry designing and operation, such as transmission and distribution, AM plays a determining role in the performance of assets to cope with power market deregulation, competition among players and risks from other events such as terrorist attacks, natural disasters, major geomagnetic disturbances and cyberattacks [4, 112, 133]. Recently, we have been observing
an evolution from Risk-based to Risk-informed safety management methodologies since the quantitative outcomes of risk assessment are simply a portion of the complete Risk- Informed Decision-making process and should be combined with other criteria such as social preferences, political concerns, and budgetary constraints, etc.[4, 108, 113].
Furthermore, industry 4.0 tools have been affecting organizations on local and world-wide level, as well as AM economic models in which maintenance performs a starring role. Industry 4.0 has substantially evolved in terms of application domains and enabling technologies such as Intelligent Maintenance (Maintenance 4.0) in the form of self- learning and intelligent devices that predicts breakdown, analyzes behavioral patterns and trends through data, makes judgment, and prompts maintenance decisions [173, 174]. Applying Maintenance 4.0 technological solutions such as IIOT, Cloud, CPS, and Big Data Analytics to AM could decrease the challenges encountered by top management such as managerial, technological, and methodological challenges. Nevertheless, acquiring accurate data and knowledge from the systems as well as understanding the patterns associated to these data and maintenance decisions are the requirements in Maintenance 4.0 [175]. In the meantime, few research has comprehensively assessed the associated advantages and effects of SAM and Intelligent Maintenance (Maintenance 4.0) on the overall performance and limitations of physical assets. Gavrikova [109] conducted a study pointing out that current studies are generally case-specific in interpreting SAM and fall back on some theoretical concepts and approaches. Therefore, it is crucial to carry out scientific research to determining theoretical and methodological coherence and consistency in understanding the different aspect of SAM in recent developments and the future research directions. This will help to align SAM within the field of AM and its various levels of organizational strategy. For e.g., enormous pieces replacement while assets reach their lifespans or as technologies turn into obsolescence is a major challenge for Electrical Power Centers (EPC) [176]. Besides, Komljenovic
[133] point out that globalization, free-market capitalism, and fierce competition make the operational and commercial environment of Electrical Power Services more and more uncertain and demanding in investments. These authors remark that High-impact, low-frequency (HILF) events such as extreme weather/natural disasters, major geomagnetic disturbances and cyberattacks have become significant factors.Moreover, modern industries are characterized by smart and networking systems, connected units bringing new business opportunities such as reconfiguration and flexibility of systems [71, 175, 177]. These will be strongly influenced by technological advances such as the ubiquity of sensors in all sectors of activity at affordable costs (cheaper, ubiquitous sensors), the computing power (computational speeds), the capacity to store dta and the power to move them quickly, as well as the contribution of Artificial Intelligence (AI) [178]. Today and tomorrow, the issue of dependability integrating reliability, maintainability, availability, and safety (RAMS) of production systems is the weak link in the management of physical assets. To face these sizeable challenges for the industry, radical changes to AM practices, especially Maintenance Management, are essential. The driving technologies of Industry 4.0, especially those of Intelligent
Maintenance (Maintenance 4.0), hold promise for SAM. It was shown in a recent survey led by ReliabilityWeb.com from 2014 to 2019 the top five benefits of asset management 4.0 that companies were most dedicated on: (i) Lifecycle cost reduction, (ii) Identifying and managing risk, (iii) Extracting data to support evidence-based asset management decisions,
(iv) Determining the optimum maintenance approaches for assets, (v) Changing company culture.
Industry players are daily confronted with decision- making at all levels involving Big Data Analytics as well as the customization and reconfiguration of processes. In the era of the Smart Factory, with the advent of new Industry 4.0 technologies in recent years, assets are becoming increasingly critical and complex to ensure dependability [178-180]. Gradually, the maintenance function gained ground and evolved from a reactive approach under the denomination of Maintenance 1.0 to a preventive approach under the designation of Maintenance 2.0, then to a Maintenance 3.0 based on the condition of the asset, and finally to a predictive and normative maintenance under the name of Maintenance
4.0 based on technologies of industry 4.0 [179]. Indeed, the major shifts in Information and Communication Technologies (ICTs) capabilities has resulted in a promising move from conventional maintenance approach to a preventive and predictive approach namely e-maintenance whereby assets are remotely monitored and managed through the Internet, and intelligent maintenance over technologies such as IoT sensors on strategic equipment [181, 182].
AM has evolved from Maintenance 1.0 using printed paperwork and considered as a "Necessary Evil" to Maintenance 4.0 corresponding to the present position where AM activities are fundamental functions of SAM [183]. Table 32 depicts the evolution of AM and the understanding of the field by top management.
Table 32 Evolution of AM and corporate thinking (Source: Own representation based on IBM, 2007)
Maintenance must play a key role to meet the challenges of Industry 4.0 and in turn, to minimize the effects of unforeseen failures as well as risks and disruptions in a context of digitized production [180]. Thusly, the ability to predict asset maintenance needs is one of the main issues and challenges of maintenance function and production operations. The aim of the maintenance function is to guarantee the productivity and sustainability as well as the dependability of assets, integrating reliability, maintainability, availability, and safety (RAMS). Maintenance performs a key role in AM as well as within industry 4.0 [175]. A recent portrait of the state of the art in the body of knowledge of Industry 4.0 positions the contribution of predictive maintenance (Pd.M.) to improving
the dependability of assets, and to optimization costs as well as productivity and product quality [91]. In the same vein, Maleti [184] carried out surveys within Slovenian companies with the purpose of exploring the capacity to assimilate the complexity of new technologies of Industry 4.0. They note that most players are aware of the positive benefits provided by the digital transformation of companies. However, they remarque an absence of a roadmap as well as a clear vision for the implementation of digital solutions in connection with predictive maintenance. In the same vein, Sahal [72] signalled a set of prerequisites and conditions for e.g., in in the fields of transportation and energy. The latter authors observe a technological gap between the needs of digital technologies applications and the capacities of existing big data analytics opensource technologies for Pd.M. Moreover, Zonta [185] observe a lack of publications about predictive maintenance in connection with Industry 4.0. Indeed, most publications in the scientific literature are exclusively interested in the analysis of data and artificial intelligence machine learning methods for the optimization of processes and procedures. The use of new technologies from Industry 4.0 makes it possible to move from the old way of doing things to optimal Intelligent Maintenance called Maintenance4.0 [70]. For instance, IoT sensors on strategic equipment on a production line make it possible to monitor the condition of assets and their performance and prevent the arrival of failures or problems that could cause production stoppage or poor product quality. As a result, connected objects as well as their conditions and performance can be monitored and followed in real time through dashboards which centralize information from the equipment.
Table 33 Maintenance 4.0 Smart Technologies
Sensors
Data (acquisition, storage, and processing units)
Artificial Intelligence
Output Unit
Actuators
Temperature
SCADA
Expert systems
AR devices
Pumps
Pressure
Hard drives
Machine Learning
Interfaces
Valves
Cameras
Cloud
Numerical Methods
D/A converter
Relays
Humidity
DAQ cards
Physical models
Screens
Hydraulic pistons
Acceleration
CPU
Web services
Servomechanisms
Rotation
MCU
Phone apps
Piezoelectric actuators
GPS
Electric motors
In the same idea, Campos [181] conducted a study on the performance measurement in AM regarding the ICTs capabilities. These authors signal the contribution of innovative technology concepts such as Big Data Analytics and Data Mining in achieving the performance measurements in AM. Table 33 shows a list of smart technologies of Maintenance 4.0 also called Intelligent Maintenance [186].
The advent of Industry 4.0 affects all industrial spheres and integrates new modern and advanced technologies. These technologies capture, optimize and deploy Big Data. We are witnessing the emergence of the "Smart Factory" concept, which is an important link in Industry 4.0. Technologies such as IoT, AI, CPS and Cloud Computing communicate, interact, and adapt continuously [187]. the most popular technologies in the scientific literature are discussed below
Cyber-physical system: CPS is a computer system in which a mechanism is controlled or monitored by computer algorithms [188, 189]]. Khaitan [190], mention that CPS are a new class of engineering systems offering a close interaction between cyber and physical components. The software and hardware of these systems are interrelated and capable of functioning at different spatiotemporal scales and can interact
with each other to change according to the context [190, 191]. In other words, CPS portray themselves as the convergence of the Physical and Digital Worlds by establishing Global Networks for the integration of manufacturing systems, machines, and warehousing systems [191, 192]. Examples of CPS include smart grids, industrial control systems, robotic systems and airplane autopilot, self-driving cars, medical surveillance. Indeed, the sensors nd actuators of these systems and machines are controlled by microcontrollers of physical cyber systems exchanging information and data through on-board computer terminals or wireless applications or clouds [193]. CPS systems have been deployed over three generations of technologies[192]: (i) technologies such as RFID allowing the unique identification of objects using bar codes with centralized data management (storage and analysis), (ii) technologies equipped with sensors and actuators with a limited set of functions, (iii) technologies equipped with networked sensors and actuators capable of storing and analyzing data.
Internet of Things: The concept of the Internet of Things (IoT) describes the network of technological physical objects integrated with technologies such as sensors and software whose purpose is to connect and exchange data with other systems via the internet [194, 195]. IoT systems rely on cloud computing infrastructures and CPS. In other words, the concept of IoT refers to a system of correlated computing devices, mechanical and digital machines, objects, humans, or animals with Unique Identifiers (UIDs) with the ability transfer data over a network without requiring human interaction (H2H Human-to-Human interaction or H2C Human-to-Computer interaction) . IoT is also a category of software application programs for process control, real-time data collection from remote sites to control equipment and conditions, spontaneous extension of Supervisory Control and Data Acquisition (SCADA). The SCADA integrates hardware components that collect and feed the data into a computer to which the SCADA software is installed where it is then processed and presented in a timely manner [196]. Flexible Manufacturing Systems (FMS) and Reconfigurable Manufacturing Systems (RMS) are increasingly equipped with intelligence allowing communication between machines (Machine to Machine, M2M), the system and the human operator (Machine to Human, M2H / System to System, S2S). M2M refers to the connection of a device to the cloud (Cloud), its management and data collection [196, 197]. The concept of the IoT has evolved from M2M communication, that is, machines connecting to each other through a network without human interaction. IoT derives its success from its power to acquire and identify data for their use while ensuring confidentiality and security [198].
Cloud Computing: The term cloud computing or on- demand availability of computer system resources is a technology usually associated with the accessibility of computer services by many users through the cloud from a provider. The cloud mainly designates computing power and cloud storage (data storage) without direct active administration by the user [199, 200]. Three standard services models of cloud computing solutions are offered by cloud- computing providers [199]: (i) Software-as-a-Service (SaaS) such as integrated management software, Enterprise Resource Planning (ERP), Customer Relationship Management (CRM), Email, virtual desktop, communication, games, etc., available
on the internet rather than installing it locally on a single PC. The consumers utilize the providers applications operating on a cloud infrastructure. (ii) Platform-as-a-Service (PaaS) which allows customers to access applications in the cloud: execution runtime, database, web server, development tools, etc. The consumer manages and controls the implemented applications, for instance, configuration settings. However, the provider endorses the cloud infrastructure comprising network, servers, operating systems, or storage. (iii) Infrastructure-as-a-Service (IaaS) for data storage, virtual machines, servers, networks, and other vital computing resources. The consumer can install and run software featuring operating systems and applications. The consumer manages and controls the implemented applications, operating systems, storage, etc. The underlying cloud infrastructure is under the control of the cloud computing provider. Let us mention some market giants in terms of availability on demand of IT system resources [201]: Amazon Web Services (AWS) providing Platforms and Application Programming Interface (APIs) on demand on a pay-per-use basis, Google with Google Drive, Microsoft with Windows Azure, and IBM with Blue-Cloud. Nowadays, it is common to store data to the cloud with the explosion of cloud providers to support administer the data- driven and technology-leveraging decision making.
Cognitive Computing / Artificial Intelligence: Cognitive computing (CC) involves the reproduction of human thought process in a computerized model in complex situations. In other words, CC systems involve technological platforms based on AI and signal processing. It encompasses self- learning systems that use data mining, pattern recognition and natural language processing, to mimic the human brain [197, 202]. Indeed, machine learning algorithms perpetually acquire knowledge from data collected from systems based on rigorous statistical and mathematical formulas (Data Mining). The purpose of these technologies is to create automated systems with the ability of extracting information, performing tasks, and solving problems without requiring human intervention.
Figure 20 Digital Twin cube (Source: IoT-Analytics [203])
Simulation Digital Twins (SDT): a digital twin (DT) is a virtual version that performs as the real-time digital equivalent of a physical thing. SDT provides asset practitioners to respond to disruptions with sound decision in real time and increase visibility on the effect of their decisions for a more resilient future. IoT-Analytics [203] defines DT as a digital abstraction or representation of a physical systems attributes and/or behavior on 3 distinct dimensions: (i) the hierarchical
level on which it is structured, Lifecycle phases in which it is applied, and (iii) its use and input data type in practice. The various DT available in the market are framed in Figure 20: Digital Twin cube including the 3 dimensions as follows [203].
SDT mainly encompasses three advances to making optimal decisions: (i) 360° holistic view (replicate an entire ecosystem), (ii) dynamic simulation (predict an organization growth in the future), (iii) opportunity to connect and synchronize in real-time (IoT can provide data into the DT, then decision making can be automated)[204].
-
DISCUSSION
The volatile economic context and the rapid advance of technology are forcing industry players to adapt their economic AM models to cope with the challenges inflicted by the fierce competition of international economies. These organizations face significant uncertainties and dreaded risks of all kinds. These include the strategic, operational, organizational, and financial, as well as technological and technical issues that seriously influence all the company's business processes. Many researchers have been interested in AM models and the organizational transformation of the increasingly digitally focused business environment and its numerous tools for the development of business models to help practitioners in various industries (for e.g., electrical power centers).
The new era of the industry is shaping the future of organizations and will induce profound changes in workforce planning. The digital shift is inevitably accompanied by a host of new challenges related to the connectivity of digital technologies, cybersecurity, the standardization and reengineering of business processes, the redefinition of processes, products, and services, and labor (acquisition, maintenance, and training). It brings new opportunities, new challenges, and a new organization of work. Likewise, given the current uncertain business context caused by the coronavirus disease pandemic (COVID-19), the challenges will be of nature, among many other aspects, strategic, operational, regulatory, financial as well as health and safety. In a wide variety of sectors, integrated risk management (RM) is gaining in importance. Indeed, asset managers will have to operate nfrastructures and decision-making processes made up of a panoply of complex and uncertain technological objects resulting from Industry 4.0. Furthermore, in the context of aging assets, organizations will face dependability challenges (Reliability, Availability, Maintainability and Safety – RAMS).
Face with this context of aging assets, the myriad of new technologies of Industry 4.0 such as simulation digital twins (SDT), artificial intelligence (AI), big data (BD), Internet of Things (IoT), Cyber-physical system (CPS), and cloud computing can help to cope with these challenges. For instance, in electrical power industries, SDT can support to enhance asset breakdown detection or prediction, manage the replacement of assets when they attain their life span, become obsolete or when transitioning to more economical and carbon-free power alternatives. Also, it can make easier to prioritize predictive maintenance as well as preventive maintenance and enhance asset replacement and optimize resource allocation. Concretely, for e.g., Velasquez-Contreras
[205] have developed a broad AM mock-up for power transformers (general asset management model for an electric utility, GAMMEU). This platform enables data integration and provides systems with intelligence for maintenance planning and scheduling, breakdown detection and diagnosis, as well as reliability analysis by means of simulations and prediction models based on industry 4.0 technologies (SDT, AI, IoT, CPS, etc.). Figure 21 shows the block diagram of GAMMEU model encompassing the following module: (i) information technology platform for data integration, (ii) intelligent system for detection and diagnosis, (iii) failure rate estimation model,-
reliability analysis, (v) optimisation model for maintenance scheduling.
Figure 1 Block diagram of GAMMEU model (Source: Velasquez-Contreras [205])
Recent studies in the Quebec manufacturing sector conclude that there is a significant delay in integrating the concept of Industry 4.0 and the technologies that accompany it despite the will of decision-makers [206]. The organizational transition and the digital context require substantial investments in new technologies and in the fight against computer crime (cyber crime) and network security. Indeed, remote management and data exchange through IoT and the panoply of opportunities provided by digital transformation require constant mobilization and vigilance to deal with any eventuality.
Thus, each organization will do better to reinvent its own business model and its way of making profits in the digital age by identifying possible changes that can disrupt its capacity [207, 208]. In other words, organizational, political, economic, and social challenges risk becoming a significant stumbling block compromising certain investment projects of organizations on the path to digital transformation [178, 209- 212].
Ultimately, all these challenges are inherent in the acquisition and storage of massive data (big data), digital governance, as well as equipment connectivity, automation and robotization through IoT and CPS. All in all, to achieve digital maturity, organizations must ensure information excellence and digital governance as well as the challenges of talents acquisition and their retention in the workplace in addition to new communication technologies 4.0. Companies that fully understand and grasp the value of the benefits that emanate from digital maturity including industry 4.0 technologies will be best positioned to meet the challenges of the future. In addition, a survey by the firm KPMG indicates
the priority of automation and digital governance in various industries. The results of the report reveal the emergence of new trends such as artificial intelligence, machine learning, the digitization of robotic processes and their positive influences on the company's value chain [213]. The report draws attention to the management of strategic, organizational, and operational issues, as well as the acquisition of talent and their retention in the workplace to improve the activities induced by the digital transformation.
In the same vein, Asset Risk Management practices (ARM) should be an integral part of physical asset management processes (AM) and strongly deemed to enable an effective AM as shown in a recent survey conducted in industrial manufacturing companies investigating the relationship and interdependency between ARM practices and their influences on maintenance performance outcomes [130]. Likewise, to cope with this kind of environment, resilience management is a well-established approach advocated in RM and uncertainties analysis. It has been promoted as a modern safety management concept to cope with complex socio- technical systems.
-
-
CONCLUSION AND FUTURE RESEARCH Both practitioners and scholars have been interested in
AM models and its numerous tools for the development of new economic models with the aim of optimizing the company's value chain. While making decision, it is crucial to come up with the proper balance between various challenging considerations for instance costs and benefits as well as opportunities and risks. Therefore, it is imperative to look thoroughly at this subject matter to grasp all the implications and identify the real demands that call for an answer at strategic level. Hence, the future target for this research is:
-
to conduct a case study highlighting RIDM's contribution to Strategic Asset Management (SAM) for the management of physical assets that allow the achievement of the desired performance of electrical utilities such as transmission and distribution which are considered as capital- intensive assets and complex adaptive system of systems.
-
to conduct a case study highlighting the influence of industry 4.0 (Maintenance 4.0) in resilience engineering.
-
to conduct a case study highlighting the influence of Resilience engineering in SAM.
These case-studies would enable to identify approaches to potential policies and strategies for SAM. The outcomes will contribute to position and validate the link between SAM and RIDM, as well as Maintenance 4.0 and Resilience engineering inside the vast discipline of AM and the alignment with various levels of organizational strategy.
REFERENCES
-
International Organization for Standardization, I., ISO-55000: Asset managementOverview, principles and terminology. 2014, International-Organization-for-Standardization, Technical Committee : ISO/TC 251 Asset management Geneva, Switzerland. p. 19.
-
Baglee, D., M. Knowles, S.-K. Kinnunen, and D. Galar, A proposed maintenance strategy for a wind turbine gearbox using condition monitoring techniques. International Journal of Process Management and Benchmarking, 2016. 6(3): p. 386-403.
-
Brown, K., M. Laue, J. Tafur, M.N. Mahmood, P. Scherrer, and R. Keast, An integrated approach to strategic asset management, in Infranomics. 2014, Springer. p. 57-74.
-
Komljenovic, D., Prise de décision en gestion des actifs industriels en tenant compte des risques. 2018, Université du Québec à Trois- Rivières.
-
Bulita, H., Fundamentals of Real Property Administration. BOMI Institute: Arnold, MD, USA, 1994.
-
IAM, I.-o.-A.-M., Asset Management – An anatomy V3. 2015.
-
Ellegaard, O. and J.A. Wallin, The bibliometric analysis of scholarly production: How great is the impact? Scientometrics, 2015. 105(3): p. 1809-1831.
-
Afuye, G.A., A.M. Kalumba, E.T. Busayo, and I.R. Orimoloye, A bibliometric review of vegetation response to climate change. Environmental Science and Pollution Research, 2021: p. 1-13.
-
Zhang, Y., Y. Zhang, K. Shi, and X. Yao, Research development, current hotspots, and future directions of water research based on MODIS images: A critical review with a bibliometric analysis. Environmental Science and Pollution Research, 2017. 24(18): p. 15226-15239.
-
Chen, K., Y. Zhang, and X. Fu, International research collaboration: An emerging domain of innovation studies? Research Policy, 2019. 48(1): p. 149-168.
-
Cobo, M.J., A.G. López-Herrera, E. Herrera-Viedma, and F. Herrera, An approach for detecting, quantifying, and visualizing the evolution of a research field: A practical application to the fuzzy sets theory field. Journal of informetrics, 2011. 5(1): p. 146- 166.
-
Wang, Z., Y. Zhao, and B. Wang, A bibliometric analysis of climate change adaptation based on massive research literature data. Journal of cleaner production, 2018. 199: p. 1072-1082.
-
Velasquez-Contreras, J.L., M.A. Sanz-Bobi, and S. Galceran Arellano, General asset management model in the context of an electric utility: Application to power transformers. Electric Power Systems Research, 2011. 81(11): p. 2015-2037.
-
Roshani, E. and Y.R. Filion, Event-based approach to optimize the timing of water main rehabilitation with asset management strategies. Journal of Water Resources Planning and Management, 2014. 140(6).
-
Caradot, N., H. Sonnenberg, I. Kropp, A. Ringe, S. Denhez, A. Hartmann, and P. Rouault, The relevance of sewer deterioration modelling to support asset management strategies. Urban Water Journal, 2017. 14(10): p. 1007-1015.
-
Alegre, H., Is strategic asset management applicable to small and medium utilities? Water Science and Technology, 2010. 62(9): p. 2051-2058.
-
Alegre, H., R. Amaral, R.S. Brito, and J.M. Baptista, Public policies as strategic asset management enablers: The case of Portugal. H2Open Journal, 2020. 3(1): p. 428-436.
-
Alegre, H., R.S. Brito, and P. Machado, Strategic asset management approach for planning investment in a large-scale irrigation system. Water Science and Technology: Water Supply, 2020. 20(6): p. 2165-2174.
-
Campher, C.A. and P.J. Vlok, Building a scenario based active mapping investment tool within a physical asset management framework. South African Journal of Economic and Management Sciences, 2014. 17(2): p. 194-206.
-
Baum, J. and P.J. Vlok, Mapping primary constraints in physical asset management strategy execution, using social network analysis. South African Journal of Industrial Engineering, 2013. 24(2): p. 47-58.
-
Stimie, J.E. and P.J. Vlok, A mechanism for the early detection and management of physical asset management strategy execution failure. South African Journal of Industrial Engineering, 2016. 27(3SpecialIssue): p. 158-173.
-
Laue, M., K. Brown, P. Scherrer, and R. Keast, Integrated strategic asset management: Frameworks and dimensions, in Topics in Safety, Risk, Reliability and Quality. 2014, Springer Netherlands. p. 75-87.
-
Joseph, T., C.E. Ugalde-Loo, J. Liang, and P.F. Coventry, Asset management strategies for power electronic converters in transmission networks: Application to Hvdc and FACTS devices. IEEE Access, 2018. 6: p. 21084-21102.
-
Stratford, D., T. Stevens, M. Hamilton, A. Dray, M. Carter, and M. Sultan, Discussion: Strategic asset management modelling of
infrastructure assets. Proceedings of the Institution of Civil Engineers: Engineering and Computational Mechanics, 2012. 165(3): p. 217-218.
-
Stratford, D., T. Stevens, M. Hamilton, and A. Dray, Strategic asset management modelling of infrastructure assets. Proceedings of the Institution of Civil Engineers: Engineering and Computational Mechanics, 2010. 163(2): p. 111-122.
-
Morrison, N., Meeting the Decent Homes Standard: London Housing Associations' Asset Management Strategies. Urban Studies, 2013. 50(12): p. 2569-2587.
-
Ten Veldhuis, J.A.E. and F.H.L.R. Clemens, The efficiency of asset management strategies to reduce urban flood risk. Water Science and Technology, 2011. 64(6): p. 1317-1324.
-
Vreeburg, J.H.G., I.N. Vloerbergh, P. Van Thienen, and R. De Bont, Shared failure data for strategic asset management. Water Science and Technology: Water Supply, 2013. 13(4): p. 1154- 1160.
-
Shah, S.W.A., M.N. Mahmood, and N. Das. Strategic asset management framework for the improvement of large scale PV power plants in Australia. in 2016 Australasian Universities Power Engineering Conference, AUPEC 2016. 2016. Institute of Electrical and Electronics Engineers Inc.
-
Haynes, B.P., N. Nunnington, and T. Eccles, Corporate real estate asset management: Strategy and implementation. Corporate Real Estate Asset Management: Strategy and Implementation. 2017: Taylor and Francis. 1-458.
-
Rødseth, H., A. Wilson, and P. Schjølberg, Integrated Production and Maintenance Planning for Successful Asset Management Strategy Implementation, in 13th World Congress on Engineering Asset Management, WCEAM 2018, J.P. Liyanage, J. Amadi- Echendu, and J. Mathew, Editors. 2020, Springer Science and Business Media Deutschland GmbH. p. 610-617.
-
Rødseth, H. and R.J. Eleftheriadis, Successful Asset Management Strategy Implementation of Cyber-Physical Systems, in 13th World Congress on Engineering Asset Management, WCEAM 2018, J.P. Liyanage, J. Amadi-Echendu, and J. Mathew, Editors. 2020, Springer Science and Business Media Deutschland GmbH. p. 15- 22.
-
Clements, D. and P. Mancarella, Systemic modelling and integrated assessment of asset management strategies and staff constraints on distribution network reliability. Electric Power Systems Research, 2018. 155: p. 164-171.
-
Aria, M. and C. Cuccurullo, bibliometrix: An R-tool for comprehensive science mapping analysis. Journal of informetrics, 2017. 11(4): p. 959-975.
-
Dubois, D. and D. Guyonnet, Risk-informed decision-making in the presence of epistemic uncertainty. International Journal of General Systems, 2011. 40(2): p. 145-167.
-
Vaurio, J.K., Importance measures in risk-informed decision making: Ranking, optimisation and configuration control. Reliability Engineering and System Safety, 2011. 96(11): p. 1426- 1436.
-
Volkanovski, A. and M. epin, Implication of PSA uncertainties on risk-informed decision making. Nuclear Engineering and Design, 2011. 241(4): p. 1108-1113.
-
Kuramoto, T., Y. Narumiya, S. Ueno, and K. Murakami. Establishment of the implementation standard concerning integrated risk-informed decision making for the safety improvement in nuclear power plants. in 30th European Safety and Reliability Conference, ESREL 2020 and 15th Probabilistic Safety Assessment and Management Conference, PSAM 2020. 2020. Research Publishing Services.
-
Narumiya, Y., K. Hayashi, and T. Kuramoto. Challenges of evolution for the risk-informed decision making standard. in 2017 25th International Conference on Nuclear Engineering, ICONE 2017. 2017. American Society of Mechanical Engineers (ASME).
-
Narumiya, Y., M. Yasuda, A. Yamaguchi, and M. Hirano. Development of a standard for risk-informed decision making. in International Topical Meeting on Probabilistic Safety Assessment and Analysis 2011, PSA 2011. 2011. Wilmington, NC.
-
Yamaguchi, A., Y. Narumiya, and M. Hirano. Technical overview of Japan's standards for risk-informed decision making. in International Topical Meeting on Probabilistic Safety Assessment and Analysis 2011, PSA 2011. 2011. Wilmington, NC.
-
Narumiya, Y. Methodology of selecting useful activities for risk- informed decision making. in 10th International Conference on Probabilistic Safety Assessment and Management 2010, PSAM 2010. 2010. Seattle, WA.
-
Narumiya, Y., M. Hirano, and M. Hirano. A technical overview of the Japan's standards for risk-informed decision making. in International Conference on Nuclear Engineering, Proceedings, ICONE. 2010. American Society of Mechanical Engineers (ASME).
-
Ferrante, F., S. Lewis, G. Parry, D. Dube, and J. Chapman, Use of Risk Insights in the Practical Implementation of an Integrated Risk-Informed Decision-Making Framework. Nclear Technology, 2021. 207(3): p. 452-459.
-
Ferrante, F., S. Lewis, G. Parry, D. Dube, D. True, and J. Chapman. Use of risk insights in the practical implementation of integrated risk-informed decision-making framework. in 16th International Topical Meeting on Probabilistic Safety Assessment and Analysis, PSA 2019. 2019. American Nuclear Society.
-
Dube, D., G. Parry, S. Lewis, D. True, F. Ferrante, and J. Chapman. Enhanced guidance on integrated risk-informed decision-making. in 2017 International Topical Meeting on Probabilistic Safety Assessment and Analysis, PSA 2017. 2017. American Nuclear Society.
-
True, D. and G. Parry. Establishing a balance between the principles of risk-informed decision- Making related to making changes to the licensing basis. in 11th International Probabilistic Safety Assessment and Management Conference and the Annual European Safety and Reliability Conference 2012, PSAM11 ESREL 2012. 2012. Helsinki.
-
Drouin, M., G. Parry, J. Lehner, J. LaChance, and T. Wheeler. Treatment of uncertainties associated with PRAs in risk-informed decision making (NUREG-1855). in 2009 Risk Management Embedded Topical Meetings. 2011. Washington, DC.
-
Wood, J., N. Siu, G. Parry, D. Dube, A. Mosleh, and M. Modarres. Model uncertainty: Conceptual and practical issues in the context of risk-informed decision making. in 2009 Risk Management Embedded Topical Meetings. 2011. Washington, DC.
-
Ferrante, F., A. Maioli, K. Kiper, A.A. Sivori, and C. Trull. Consideration of multi-unit risk aspects within an integrated risk- informed decision-making framework. in 16th International Topical Meeting on Probabilistic Safety Assessment and Analysis, PSA 2019. 2019. American Nuclear Society.
-
Ferrante, F. External flooding in regulatory risk-informed decision-making for operating nuclear reactors in the United States. in 2015 International Topical Meeting on Probabilistic Safety Assessment and Analysis, PSA 2015. 2015. American Nuclear Society.
-
Jang, D., N. Cho, and S.C. Kim. Development of domestic risk informed decision making framework. in 2018 Transactions of the American Nuclear Society, ANS 2018 and Embedded Topical Meeting Nuclear Fuels and Structural Materials. 2018. American Nuclear Society.
-
Kim, B.G., D. Jang, N. Cho, S.C. Kim, Y. Lee, and Y. Cho. Development of safety significance analysis system for risk- informed decision making. in 2018 Transactions of the American Nuclear Society, ANS 2018 and Embedded Topical Meeting Nuclear Fuels and Structural Materials. 2018. American Nuclear Society.
-
Ham, Y. and J. Kim, Participatory Sensing and Digital Twin City: Updating Virtual City Models for Enhanced Risk-Informed Decision-Making. Journal of Management in Engineering, 2020. 36(3).
-
Talarico, L. and G. Reniers, Risk-informed decision making of safety investments by using the disproportion factor. Process Safety and Environmental Protection, 2016. 100: p. 117-130.
-
Aven, T. and V. Kristensen, How the distinction between general knowledge and specific knowledge can improve the foundation and practice of risk assessment and risk-informed decision-making. Reliability Engineering and System Safety, 2019. 191.
-
Parry, G.W., D.E. Vanover, D.E. True, S. Lewis, and M. Presley. Risk-informed decision-making: Addressing very large PRA uncertainties. in International Topical Meeting on Probabilistic Safety Assessment and Analysis 2013, PSA 2013. 2013. Columbia, SC: American Nuclear Society.
-
Fumagalli, L., L. Cattaneo, I. Roda, M. Macchi, and M. Rondi, Data-driven CBM tool for risk-informed decision-making in an electric arc furnace. International Journal of Advanced Manufacturing Technology, 2019. 105(1-4): p. 595-608.
-
Nezamian, A. and M. Kartsounis. Risk-informed asset management framework for aged port facilities against potential external hazards. in 29th International Ocean and Polar Engineering Conference, ISOPE 2019. 2019. International Society of Offshore and Polar Engineers.
-
Tan, T.G., S. Jang, and A. Yamaguchi, A novel method for risk- informed decision-making under non-ideal Instrumentation and Control conditions through the application of Bayes Theorem. Reliability Engineering and System Safety, 2019. 188: p. 463-472.
-
Tan, T.G., S. Jang, and A. Yamaguchi. Application of Bayes theorem for risk-informed decision-making at the decommissioning of Fukushima Daiichi nuclear power plant. in 14th Probabilistic Safety Assessment and Management, PSAM 2018. 2018. International Association for Probablistic Safety Assessment and Management (IAPSAM).
-
Morales-Torres, A., I. Escuder-Bueno, A. Serrano-Lombillo, and
J.T. Castillo RodrÃguez, Dealing with epistemic uncertainty in risk- informed decision making for dam safety management. Reliability Engineering and System Safety, 2019. 191.
-
Bujor, A., R. Gheorghe, M. El-Hawary, A. El-Jaby, J. Szymanski, and P. Wan. Application of the Risk-Informed Decision Making process to the moderator subcooling margins issue in a Pressurized Heavy Water Reactor (PHWR). in European Safety and Reliability Conference, ESREL 2013. 2014. Amsterdam: shers.
-
Bujor, A. Risk Informed Decision Making-approach for consideration of time-at-risk. in European Safety and Reliability Annual Conference: Reliability, Risk and Safety: Back to the Future, ESREL 2010. 2010. Rhodes.
-
Bujor, A. and R. Gheorghe. Risk informed decision making- specific aspects for risk-informing decisions in a regulatory environment. in European Safety and Reliability Annual Conference: Reliability, Risk and Safety: Back to the Future, ESREL 2010. 2010. Rhodes.
-
Alipour, M.H., Risk-Informed Decision Making Framework for Operating a Multi-Purpose Hydropower Reservoir During Flooding and High Inflow Events, Case Study: Cheakamus River System. Water Resources Management, 2015. 29(3): p. 801-815.
-
Martorell, S., M. Villamizar, I. Martón, S. Carlos, and A.I. Sánchez. Overall treatment of uncertainties in the context of risk- informed decision-making on Completion Time changes. in European Safety and Reliability Conference, ESREL 2013. 2014. Amsterdam: shers.
-
Martorell, S., M. Villamizar, J.F. Villanueva, S. Carlos, and A.I. Sánchez. Importance analysis in risk-informed decision-making of changes to Allowed Outage Times addressing uncertainties. in European Safety and Reliability Conference: Advances in Safety, Reliability and Risk Management, ESREL 2011. 2012. Troyes.
-
Gjorgiev, B., D. Kanev, and M. epin, Risk-informed decision making in the nuclear industry: Application and effectiveness comparison of different genetic algorithm techniques. Nuclear Engineering and Design, 2012. 250: p. 701-712.
-
Yan, J., Y. Meng, L. Lu, and L. Li, Industrial Big Data in an Industry 4.0 Environment: Challenges, Schemes, and Applications for Predictive Maintenance. IEEE Access, 2017. 5: p. 23484- 23491.
-
Masoni, R., F. Ferrise, M. Bordegoni, M. Gattullo, A.E. Uva, M. Fiorentino, E. Carrabba, and M. Di Donato, Supporting remote maintenance in industry 4.0 through augmented reality. Procedia manufacturing, 2017. 11: p. 1296-1302.
-
Sahal, R., J.G. Breslin, and M.I. Ali, Big data and stream processing platforms for Industry 4.0 requirements mapping for a predictive maintenance use case. Journal of Manufacturing Systems, 2020. 54: p. 138-151.
-
Nordal, H. and I. El-Thalji, Modeling a predictive maintenance management architecture to meet industry 4.0 requirements: A case study. Systems Engineering, 2021. 24(1): p. 34-50.
-
Duque, S.E. and I. El-Thalji, Intelligent Maintenance Maturity of Offshore Oil and Gas Platform: A Customized Assessment Model Complies with Industry 4.0 Vision, in 13th World Congress on Engineering Asset Management, WCEAM 2018, J.P. Liyanage, J.
Amadi-Echendu, and J. Mathew, Editors. 2020, Springer Scienceand Business Media Deutschland GmbH. p. 653-663.
-
El-Thalji, I., S.E. Duque, and H. Nordal, Design for Intelligent Maintenance: A Potential Reference Standard Complies with Industry 4.0 Requirements, in 13th World Congress on Engineering Asset Management, WCEAM 2018, J.P. Liyanage, J. Amadi-Echendu, and J. Mathew, Editors. 2020, Springer Science and Business Media Deutschland GmbH. p. 500-510.
-
Nordal, H. and I. El-Thalji, Intelligent Maintenance Practices Within Norwegian Continental Shelf Toward Industry 4.0 Vision: An Overview, in 13th World Congress on Engineering Asset Management, WCEAM 2018, J.P. Liyanage, J. Amadi-Echendu, and J. Mathew, Editors. 2020, Springer Science and Business Media Deutschland GmbH. p. 636-645.
-
Bousdekis, A., K. Lepenioti, D. Apostolou, and G. Mentzas, A review of data-driven decision-making methods for industry 4.0 maintenance applications. Electronics (Switzerland), 2021. 10(7).
-
Bousdekis, A., K. Lepenioti, D. Apostolou, and G. Mentzas. Decision making in predictive maintenance: Literature review and research agenda for industry 4.0. in 9th IFAC Conference on Manufacturing Modelling, Management and Control, MIM 2019. 2019. Elsevier B.V.
-
Mentzas, G., K. Hribernik, K.D. Thoben, D. Kiritsis, and A. Mousavi, Part 6 Summary: Predictive maintenance in industry 4.0
– methodologies, tools and interoperable applications. Enterprise Interoperability: Smart Services and Business Impact of Enterprise Interoperability, 2018: p. 235-237.
-
Bousdekis, A. and G. Mentzas, Condition-Based Predictive Maintenance in the Frame of Industry 4.0, in IFIP WG 5.7 International Conference on Advances in Production Management Systems, APMS 2017, R. Riedel, et al., Editors. 2017, Springer New York LLC. p. 399-406.
-
Marcucci, G., S. Antomarioni, F.E. Ciarapica, and M. Bevilacqua, The impact of Operations and IT-related Industry 4.0 key technologies on organizational resilience. Production Planning and Control, 2021.
-
Gattullo, M., A. Evangelista, A.E. Uva, M. Fiorentino, A. Boccaccio, and V.M. Manghisi, Exploiting Augmented Reality to Enhance Piping and Instrumentation Diagrams for Information Retrieval Tasks in Industry 4.0 Maintenance, in 16th International Conference on Virtual Reality and Augmented Reality, EuroVR 2019, P. Bourdot, et al., Editors. 2019, Springer. p. 170-180.
-
Scurati, G.W., M. Gattullo, M. Fiorentino, F. Ferrise, M. Bordegoni, and A.E. Uva, Converting maintenance actions into standard symbols for Augmented Reality applications in Industry
4.0. Computers in Industry, 2018. 98: p. 68-79.
-
Li, Z., Y. Wang, and K.S. Wang, Intelligent predictive maintenance for fault diagnosis and prognosis in machine centers: Industry 4.0 scenario. Advances in Manufacturing, 2017. 5(4): p. 377-387.
-
Calabrese, M., M. Cimmino, F. Fiume, M. Manfrin, L. Romeo, S. Ceccacci, M. Paolanti, G. Toscano, G. Ciandrini, A. Carrotta, M. Mengoni, E. Frontoni, and D. Kapetis, SOPHIA: An event-based IoT and machine learning architecture for predictive maintenance in industry 4.0. Information (Switzerland), 2020. 11(4).
-
Calabrese, M., M. Cimmino, M. Manfrin, F. Fiume, D. Kapetis, M. Mengoni, S. Ceccacci, E. Frontoni, M. Paolanti, A. Carrotta, and
G. Toscano. An event based machine learning framework for predictive maintenance in industry 4.0. in ASME 2019 International Design Engineering Technical Conferences and Computers and Information in Engineering Conference, IDETC- CIE 2019. 2019. American Society of Mechanical Engineers (ASME).
-
Paolanti, M., L. Romeo, A. Felicetti, A. Mancini, E. Frontoni, and
J. Loncarski. Machine Learning approach for Predictive Maintenance in Industry 4.0. in 14th IEEE/ASME International Conference on Mechatronic and Embedded Systems and Applications, MESA 2018. 2018. Institute of Electrical and Electronics Engineers Inc.
-
Ceruti, A., P. Marzocca, A. Liverani, and C. Bil, Maintenance in aeronautics in an Industry 4.0 context: The role of Augmented Reality and Additive Manufacturing. Journal of Computational Design and Engineering, 2019. 6(4): p. 516-526.
-
Franciosi, C., B. Iung, S. Miranda, and S. Riemma. Maintenance for Sustainability in the Industry 4.0 context: a Scoping Literature Review. 2018. Elsevier B.V.
-
Sipsas, K., K. Alexopoulos, V. Xanthakis, and G. Chryssolouris. Collaborative Maintenance in flow-line Manufacturing Environments: An Industry 4.0 Approach. in 5th CIRP Global Web Conference Research and Innovation for Future Production, 2016. 2016. Elsevier B.V.
-
Zonta, T., C.A. da Costa, R. da Rosa Righi, M.J. de Lima, E.S. da Trindade, and G.P. Li, Predictive maintenance in the Industry 4.0: A systematic literature review.. Computers & Industrial Engineering, 2020: p. 106889.
-
Alqahtani, A.Y., S.M. Gupta, and K. Nakashima, Warranty and maintenance analysis of sensor embedded products using internet of things in industry 4.0. International Journal of Production Economics, 2019. 208: p. 483-499.
-
Azadeh, A., V. Salehi, M. Arvan, and M. Dolatkhah, Assessment of resilience engineering factors in high-risk environments by fuzzy cognitive maps: A petrochemical plant. Safety Science, 2014. 68: p. 99-107.
-
Hollnagel, E., D.D. Woods, and N. Leveson, Resilience engineering: Concepts and precepts. Resilience Engineering: Concepts and Precepts. 2012: Ashgate Publishing Ltd. 1-397.
-
Hosseini, S., K. Barker, and J.E. Ramirez-Marquez, A review of definitions and measures of system resilience. Reliability Engineering and System Safety, 2016. 145: p. 47-61.
-
Henry, D. and J.E. Ramirez-Marquez, Generic metrics and quantitative approaches for system resilience as a function of time. Reliability Engineering and System Safety, 2012. 99: p. 114-122.
-
Righi, A.W., T.A. Saurin, and P. Wachs, A systematic literature review of resilience engineering: Research areas and a research agenda proposal. Reliability Engineering and System Safety, 2015. 141: p. 142-152.
-
Woods, D.D., Four concepts for resilience and the implications for the future of resilience engineering. Reliability Engineering and System Safety, 2015. 141: p. 5-9.
-
Bie, Z., Y. Lin, G. Li, and F. Li, Battling the Extreme: A Study on the Power System Resilience. Proceedings of the IEEE, 2017. 105(7): p. 1253-1266.
-
Panteli, M. and P. Mancarella, The grid: Stronger, bigger, smarter?: Presenting a conceptual framework of power system resilience. IEEE Power and Energy Magazine, 2015. 13(3): p. 58- 66.
-
Ayyub, B.M., Systems resilience for multihazard environments: Definition, metrics, and valuation for decision making. Risk Analysis, 2014. 34(2): p. 340-355.
-
Ranasinghe, U., M. Jefferies, P. Davis, and M. Pillay, Resilience Engineering Indicators and Safety Management: A Systematic Review. Safety and Health at Work, 2020. 11(2): p. 127-135.
-
Hollnagel, E., J. Pariès, D. Woods, and J. Wreathall, Resilience engineering in practice: A guidebook. Resilience Engineering in Practice: A Guidebook. 2011: Ashgate Publishing Ltd. 1-322.
-
Li, Z., M. Shahidehpour, F. Aminifar, A. Alabdulwahab, and Y. Al-Turki, Networked Microgrids for Enhancing the Power System Resilience. Proceedings of the IEEE, 2017. 105(7): p. 1289-1310.
-
Patriarca, R., J. Bergström, G. Di Gravio, and F. Costantino, Resilience engineering: Current status of the research and future challenges. Safety Science, 2018. 102: p. 79-100.
-
Azadeh, A., S.M. Asadzadeh, and M. Tanhaeean, A consensus- based AHP for improved assessment of resilience engineering in maintenance organizations. Journal of Loss Prevention in the Process Industries, 2017. 47: p. 151-160.
-
Farzin, H., M. Fotuhi-Firuzabad, and M. Moeini-Aghtaie, Enhancing Power System Resilience Through Hierarchical Outage Management in Multi-Microgrids. IEEE Transactions on Smart Grid, 2016. 7(6): p. 2869-2879.
-
Komljenovic, D., M. Gaha, G. Abdul-Nour, C. Langheit, and M. Bourgeois, Risks of extreme and rare events in Asset Management. Safety science, 2016. 88: p. 129-145.
-
Gavrikova, E., I. Volkova, and Y. Burda, Strategic aspects of asset management: An overview of current research. Sustainability, 2020. 12(15): p. 5955.
-
Petchrompo, S. and A.K. Parlikad, A review of asset management literature on multi-asset systems. Reliability Engineering & System Safety, 2019. 181: p. 181-201.
-
Bartlett, S., Asset Management in a de-regulated environment on behalf of the CIGRE Joint Task Force 23.18 and Australian Working Groups. CIGRE Paris, 2002.
-
Khuntia, S.R., J.L. Rueda, S. Bouwman, and M.A. van der Meijden, A literature survey on asset management in electrical power [transmission and distribution] system. International Transactions on Electrical Energy Systems, 2016. 26(10): p. 2123- 2133.
-
Zio, E. and N. Pedroni, Overview of risk-informed decision-making processes. 2012: FonCSI.
-
International Organization for Standardization, I., ISO-55001: Asset Management – Management Systems – Requirements. 2014, International-Organization-for-Standardization, Technical Committee : ISO/TC 251 Asset management: Geneva, Switzerland. p. 14.
-
International Organization for Standardization, I., ISO 55002:2014: Asset management Management systems Guidelines for the application of ISO 55001. 2014, International- Organization-for-Standardization, Technical Committee : ISO/TC 251 Asset management: Geneva, Switzerland. p. 32.
-
International Organization for Standardization, I., ISO 55002:2018: Asset management Management systems Guidelines for the application of ISO 55001. 2018, International Organization for Standardization, Technical Committee : ISO/TC 251 Asset management: Geneva, Switzerland. p. 72.
-
Campbell, J.D., J.V. Reyes-Picknell, and H.S. Kim, Uptime: Strategies for excellence in maintenance management. 2015: CRC Press.
-
GFMAM, G.-F.-o.-M.a.A.-M., The Asset Management Landscape. 2014, GFMAM: Zürich.
-
Asset Management Council, A., Asset Management Body of Knowledge (AMBOK) : Framework for Asset Management. 2014, Asset Management Council Melbourne.
-
CIGRÉ, C.-I.-d.-G.-R.-É., IT Strategie for Asset Management of Substations – General Principles., W.G.B.T. 05, Editor. 2014, International Council on Large Electric Systems: Paris.
-
CIGRÉ, C.-I.-d.-G.-R.-É., Asset management decision making using different risk assessment methodologies, W.G. C1.25, Editor. 2013, International Council on Large Electric Systems: Paris.
-
Conseil-International-des-Grands-Réseaux-Électriques, CIGRÉ: Asset management decision making using different risk assessment methodologies. 2013, CIGRÉ: Conseil-International-des-Grands- Réseaux-Électriques.
-
International Organization for Standardization, I., ISO 31000:2018 Risk management Guidelines. 2018, International Organization for Standardization ISO Geneva, Switzerland, Technical Committee : ISO/TC 262 Risk management. p. 16.
-
International Organization for Standardization, I., ISO-31000: Risk Management-Principles and guidelines. 2009, International Organization for Standardization ISO Geneva, Switzerland.
-
Hubbard, D.W., The failure of risk management: Why it's broken and how to fix it. 2020: John Wiley & Sons.
-
Dezfuli, H., M. Stamatelatos, G. Maggio, C. Everett, R. Youngblood, P. Rutledge, A. Benjamin, R. Williams, C. Smith, and S. Guarro, NASA risk-informed decision making handbook. 2010.
-
International Electrotechnical Commission, I., ISO 31010Risk ManagementRisk Assessment Techniques. 2008, International Electrotechnical Commission: Geneva.
-
Purdy, G., ISO 31000: 2009setting a new standard for risk management. Risk Analysis: An International Journal, 2010. 30(6): p. 881-886.
-
Olechowski, A., J. Oehmen, W. Seering, and M. Ben-Daya, The professionalization of risk management: What role can the ISO 31000 risk management principles play? International Journal of Project Management, 2016. 34(8): p. 1568-1578.
-
Maleti, D., H. Paaiová, A. Nagyová, and M. Maleti, The link between asset risk management and maintenance performance: A study of industrial manufacturing companies. Quality Innovation Prosperity, 2020. 24(3): p. 50-69.
-
Aven, T., Some reflections on uncertainty analysis and management. Reliability Engineering & System Safety, 2010. 95(3): p. 195-201.
-
Komljenovic, D., G. Abdul-Nour, and J.-F. Boudreau, Risk- informed decision-making in asset management as a complex
adaptive system of systems. International Journal of Strategic Engineering Asset Management, 2019. 3(3): p. 198-238.
-
Komljenovic, D., M. Darragi, L. Pierre, C. Steve, and C. Ramzi, Risk-Informed Decision-Making in Asset Management of Electrical Utilities in CIGRE Canada Conference, September 16-19, 2019,
C. Canada, Editor. 2019, CIGRE Canad: Montréal, Québec.
-
Zio, E., Reliability engineering: Old problems and new challenges.
Reliability engineering & system safety, 2009. 94(2): p. 125-141.
-
Aven, T. and E. Zio, Some considerations on the treatment of uncertainties in risk assessment for practical decision making. Reliability Engineering & System Safety, 2011. 96(1): p. 64-74.
-
Marhavilas, P.K. and D.E. Koulouriotis, Risk-Acceptance Criteria in Occupational Health and Safety Risk-AssessmentThe State-of- the-Art through a Systematic Literature Review. Safety, 2021. 7(4): p. 77.
-
Travers, W., Staff RequirementsSECY-98-14White Paper on Risk-Informed and Performance-Based Regulation. US Nuclear Regulatory Commission(NRC)< http://pbadupws. nrc. gov/docs/ML0037/ML003753601. pdf>(accessed 24 December, 2015), 1999.
-
Dezfuli, H., M. Stamatelatos, G. Maggio, and C. Everett. Risk- informed decision making in the context of NASA risk management. in 10th International Conference on Probabilistic Safety Assessment and Management 2010, PSAM 2010. 2010. Seattle, WA.
-
Dezfuli, H., G. Maggio, and C. Everett. Risk-informed decision making application to technology development alternative selection. in 4th IAASS Conference 'Making Safety Matter'. 2010. Huntsville, AL.
-
Aven, T., Foundations of risk analysis. 2012: John Wiley & Sons.
-
National Aeronautics and Space Administration, N., Probabilistic risk assessment procedures guide for NASA managers and practitioners. Technical report, NASA. Washington, DC: NASA, 2002.
-
Electrical-Power-Research-Institute(EPRI), Risk-Informed Asset Management (RIAM) Development Plan. 2002, EPRI: Palo Alto.
-
Liming, K., Risk-informed asset management for electric power plants. Energy pulse RIAM paper, 2002.
-
Komljenovic, D., J. Paraszczak, and V. Kecojevic, Possibilities for optimization of reliability and maintenance in mining operations based on experience in nuclear industry. Mine Planning and Equipment Selection 2005, 2005: p. 632-645.
-
Komljenovic, D., Development of risk-informed, performance- based asset management in mining. International Journal of Mining, Reclamation and Environment, 2008. 22(2): p. 146-153.
-
Komljenovic, D., G. Abdul-Nour, and N. Popovic, An approach for strategic planning and asset management in the mining industry in the context of business and operational complexity. International Journal of Mining and Mineral Engineering, 2015. 6(4): p. 338-360.
-
Association, R.E. Resilience. 2021 [cited 2021 November 2021]; Available from: http://www.resilience-engineering-association.org.
-
Fairbanks, R.J., R.L. Wears, D.D. Woods, E. Hollnagel, P. Plsek, and R.I. Cook, Resilience and resilience engineering in health care Joint Commission journal on quality and patient safety, 2014. 40(8): p. 376-383.
-
Hollnagel, E., Resilience engineering in practice: A guidebook. 2013: Ashgate Publishing, Ltd.
-
Woods, D.D., Creating foresight: How resilience engineering can transform NASAs approach to risky decision making. Work, 2003. 4(2): p. 137-144.
-
Resilience, C.I., The National Infrastructure Advisory Council . Resilience, Critical Infrastructure. 2009.
-
Vugrin, E.D., D.E. Warren, M.A. Ehlen, and R.C. Camphouse, A framework for assessing the resilience of infrastructure and economic systems, in Sustainable and resilient critical infrastructure systems. 2010, Springer. p. 77-116.
-
International Organization for Standardization, I., ISO-22316: Security and resilience – Organizational resilience – Principles and attributes. , in
2017, International Organization for Standardization, Technical Committee : ISO/TC 292 Security and resilience: ISO Geneva, Switzerland. p. 10.
-
Hale, A. and T. Heijer, Defining resilience, in Resilience engineering. 2017, CRC Press. p. 35-40.
-
Denyer, D., Organizational Resilience: A summary of academic evidence, business insights and new thinking. BSI and Cranfield School of Management, 2017: p. 8-25.
-
Lexicon, D.R. and R.S. Committee, US Department of Homeland Security. 2008, September.
-
OrtizdeMandojana, N. and P. Bansal, The longterm benefits of organizational resilience through sustainable business practices. Strategic Management Journal, 2016. 37(8): p. 1615-1631.
-
Home III, J.F. and J.E. Orr, Assessing behaviors that create resilient organizations. Employment relations today, 1997. 24(4): p. 29-39.
-
Holling, C.S., Resilience and stability of ecological systems.
Annual review of ecology and systematics, 1973. 4(1): p. 1-23.
-
Walker, B., C.S. Holling, S.R. Carpenter, and A. Kinzig, Resilience, adaptability and transformability in socialecological systems. Ecology and society, 2004. 9(2).
-
Tierney, K. and M. Bruneau, Conceptualizing and measuring resilience: A key to disaster loss reduction. TR news, 2007(250).
-
Lofquist, E.A., Jousting with dragons: a resilience engineering approach to managing SMS in the transport sector. 2017.
-
Sikula, N.R., J.W. Mancillas, I. Linkov, and J.A. McDonagh, Risk management is not enough: a conceptual model for resilience and adaptation-based vulnerability assessments. Environment Systems and Decisions, 2015. 35(2): p. 219-228.
-
Hickford, A.J., S.P. Blainey, A.O. Hortelano, and R. Pant, Resilience engineering: theory and practice in interdependent infrastructure systems. Environment Systems and Decisions, 2018. 38(3): p. 278-291.
-
Hollnagel, E. and D.D. Woods, Epilogue: Resilience engineering precepts, in Resilience engineering. 2017, CRC Press. p. 347-358.
-
Righi, A.W., T.A. Saurin, and P. Wachs, A systematic literature review of resilience engineering: Research areas and a research agenda proposal. Reliability Engineering & System Safety, 2015. 141: p. 142-152.
-
Komljenovic, D., L. Stojanovic, V. Malbasic, and A. Lukic, A resilience-based approach in managing the closure and abandonment of large mine tailing ponds. International Journal of Mining Science and Technology, 2020. 30(5): p. 737-746.
-
Kurth, M.H., J.M. Keenan, M. Sasani, and I. Linkov, Defining resilience for the US building industry. Building Research & Information, 2019. 47(4): p. 480-492.
-
Albino, D.K., K. Friedman, Y. Bar-Yam, and W.G. Glenney IV, Military strategy in a complex world. arXiv preprint arXiv:1602.05670, 2016.
-
Zio, E., Challenges in the vulnerability and risk analysis of critical infrastructures. Reliability Engineering & System Safety, 2016. 152: p. 137-150.
-
Roshani, E. and Y. Filion, Event-based approach to optimize the timing of water main rehabilitation with asset management strategies. Journal of Water Resources Planning and Management, 2014. 140(6): p. 04014004.
-
Alegre, H., R. Amaral, R. Brito, and J. Baptista, Public policies as strategic asset management enablers: the case of Portugal. H2Open Journal, 2020. 3(1): p. 428-436.
-
Lee, J., H.-A. Kao, and S. Yang, Service innovation and smart analytics for industry 4.0 and big data environment. Procedia Cirp, 2014. 16: p. 3-8.
-
Guillén, A., V. González-Prida, J. Gómez, A. Crespo, G. Turconi, and G. Ventola. Maintenance 4.0. review of maintenance role in the industry 4.0 revolution. in 27th European Safety and Reliability Conference, ESREL 2017. 2017. CRC Press/Balkema.
-
Galar, D. and M. Kans. The impact of maintenance 4.0 and big data analytics within strategic asset management. in Maintenance Performance and Measurement and Management 2016 (MPMM 2016). November 28, Luleå, Sweden. 2017. Luleå tekniska universitet.
-
Biard, G. and G.A. Nour, Industry 4.0 Contribution to Asset Management in the Electrical Industry. Sustainability, 2021. 13(18): p. 10369.
-
Kans, M. and A. Ingwald, Business model development towards service management 4.0. Procedia Cirp, 2016. 47: p. 489-494.
-
Gershwin, S.B.S.S.B., The future of manufacturing systems engineering.. International Journal of Production Research, 2018. 56(1-2): p. 224-237.
179. |
Jasiulewicz-Kaczmarek, M., S. Legutko, and P. Kluk, Maintenance 4.0 technologiesnew opportunities for sustainability driven |
197. Rouse, M. Cognitive Computing.. 2016 2016/02/01; Available from: https://whatis.techtarget.com/fr/definition/Informatique- |
maintenance.. Management and Production Engineering Review, |
cognitive#:~:text=Les%20syst%C3%A8mes%20d'informatique%2 |
|
180. |
2020. 11. Jasiulewicz-Kaczmarek et al., G., Maintenance 4.0 Technologies |
0cognitive,pour%20en%20tirer%20des%20informations. 198. ITU-T-Y.4000, Overview of the Internet of things.. in Edition 1.0 . |
for Sustainable Manufacturing-an Overview. IFAC-PapersOnLine, |
Study, R.Y.Y. (06/12), Editor. 2016, The International |
|
181. |
2019. 52(10): p. 91-96. Campos, J., P. Sharma, E. Jantunen, D. Baglee, and L. Fumagalli, |
Telecommunication Union ( ITU) 199. Mell, P. and T. Grance, The NIST definition of cloud computing.. |
Business performance measurements in asset management with the |
2011, The National Institute of Standards and Technology. |
|
support of big data technologies. Management Systems in Production Engineering, 2017. |
200. Montazerolghaem, A., M.H. Yaghmaee, and A. Leon-Garcia, Green Cloud Multimedia Networking: NFV/SDN based Energy- |
|
182. |
Iung, B., E. Levrat, A.C. Marquez, and H. Erbe, E-maintenance: |
efficient Resource Allocation.. IEEE Transactions on Green |
principles, review and conceptual framework. IFAC Proceedings Volumes, 2007. 40(19): p. 18-29. |
Communications and Networking, 2020. 201. Haug, K.C., T. Kretschmer, and T. Strobel, Cloud adaptiveness |
|
183. |
Konstantakos, P., P. Chountalas, and A. Magoutas, The |
within industry sectorsMeasurement and observations.. |
contemporary landscape of asset management systems. Quality- Access to Success, 2019. 20(169): p. 10-17. |
Telecommunications policy, 2016. 40(4): p. 291-306. 202. Kelly III, J., Computing, Cognition, And the Future of Knowing: |
|
184. |
Maleti, D., N. Almeida, D. Komljenovic, V. Lovreni, and M. |
How Humans and Macines Are Forging a New Age of |
Maleti, Digitalizing Predictive Maintenance to Improve Asset Management: Are We Ready.. 2020. |
Understanding.[online] Computing Research News.. 2016. 203. IoT-Analytics, Digital Twin Insights Report 2020. 2020. p. 90. |
|
185. |
Zonta, T., C.A. da Costa, R. da Rosa Righi, M.J. de Lima, E.S. da |
204. Bantel, H.d. White Paper: Simulation Digital Twins, IoT and the |
Trindade, and G.P. Li, Predictive maintenance in the Industry 4.0: A systematic literature review. Computers and Industrial |
Cloud: The New Nexus of Value Creation: Welcome to the new normal: its volatile, its uncertain and its complex. 2021 [cited |
|
Engineering, 2020. 150(106889). |
2021; Available from: |
|
186. |
Arranz, M.C., Introduction and Need for Maintenance in Transportation: A Way Towards Smart Maintenance, in |
https://cosmotech.com/resources/ebooks/white-paper-iot-cloud- simulation-digital-twins/. |
187. |
Transportation Systems. 2019, Springer. p. 95-111. Boston-Consulting-Group. Putting Industry4.0 to Work.. 2020 |
205. Velasquez-Contreras, J.L., M.A. Sanz-Bobi, and S.G. Arellano, General asset management model in the context of an electric |
[cited 2020 01.01.2021]; Available from: https://www.bcg.com/fr- |
utility: Application to power transformers. Electric Power Systems |
|
ca/capabilities/operations/embracing-industry-4.0-rediscovering- growth |
Research, 2011. 81(11): p. 2015-2037. 206. Gamache, S., G. Abdul-Nour, and C. Baril, Evaluation of the |
|
188. |
Bartodziej, C.J., The concept industry 4.0.. in The concept industry |
influence parameters of Industry 4.0 and their impact on the |
189. |
4.0. 2017, Springer. p. 27-50. The National Sanitation Foundation, N., Cyber-Physical Systems |
Quebec manufacturing SMEs: The first findings. Cogent Engineering, 2020. 7(1): p. 1771818. |
(CPS).. 2020, The National Sanitation Foundation (NSF) US |
207. Mettling, B., Transformation numérique et vie au travail, Rapport |
|
190. |
National Science Foundation. Khaitan, S.K. and J.D. McCalley, Design techniques and |
à lattention du Ministre du Travail, de lEmploi, de la Formation professionnelle et du Dialogue social. 2015. |
applications of cyberphysical systems: A survey.. IEEE Systems |
208. European-Asset-Management-Committee, Vers lusine du futur – |
|
191. |
Journal, 2014. 9(2): p. 350-365. Shafiq, S.I., C. Sanin, C. Toro, and E. Szczerbicki, Virtual |
White Paper. EFICIO, lefficience des CIO et lefficacité des processus transformés.. 2017. |
engineering object (VEO): Toward experience-based design and |
209. Lorenz, M., M. Rüßmann, R. Strack, K.L. Lueth, and M. Bolle, |
|
manufacturing for industry 4.0.. Cybernetics and Systems, 2015. 46(1-2): p. 35-50. |
Man and machine in industry 4.0: How will technology transform the industrial workforce through 2025.. The Boston Consulting |
|
192. |
Wang, S., J. Wan, D. Zhang, D. Li, and C. Zhang, Towards smart |
Group, 2015. 2. |
factory for industry 4.0: a self-organized multi-agent system with big data based feedback and coordination.. Computer Networks, |
210. Koch, V., S. Kuge, R. Geissbauer, and S. Schrauf, Industry 4.0: Opportunities and challenges of the industrial internet.. Strategy & |
|
2016. 101: p. 158-168. |
PwC, 2014. |
|
193. |
Lu, Y., Industry 4.0: A survey on technologies, applications and open research issues.. Journal of industrial information integration, |
211. Birkel, H.S.H.H.S., Impact of IoT challenges and risks for SCM.. Supply Chain Management, 2019. 24(1): p. 39-61. |
2017. 6: p. 1-10. |
212. Wyrwicka, M. and B. Mrugalska, Industry 4.0towards |
|
194. |
Erboz, G. How to define Industry 4. 0: The Main Pillars of Industry 4. 0.. in 7th International Conference on Management |
opportunities and challenges of implementation.. DEStech Transactions on Engineering and Technology Research, |
(ICoM 2017), At Nitra, Slovakia. 2017. |
2017(icpr). |
|
195. |
Wang, K., Intelligent predictive maintenance (IPdM) system Industry 4.0 scenario. WIT Transactions on Engineering Sciences, |
213. KPMG, Adoption of intelligent automation does not equal success. 4Q 2017 KPMG Global Insights. Pulse Survey Report. 2018: USA. |
2016. 113: p. 259-268. |
||
196. |
Rouse, M. Internet of things (IoT).. 2020 01.02.2020 [cited 2021 01.01.2021]; Available from: |
https://internetofthingsagenda.techtarget.com/definition/Internet- of-Things-IoT