
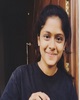
- Open Access
- Authors : Neha J , Vikash D , Akash R M , Paramesh R
- Paper ID : IJERTV11IS060178
- Volume & Issue : Volume 11, Issue 06 (June 2022)
- Published (First Online): 22-06-2022
- ISSN (Online) : 2278-0181
- Publisher Name : IJERT
- License:
This work is licensed under a Creative Commons Attribution 4.0 International License
Mean Variance Optimization and Momentum Investing
1 Neha J, 2 Akash R M, 3 Vikash D, Paramesh R
Global Academy of Technology
Abstract:- The aim of the paper is to understand the limitations of MVO, alternate approaches that may be more appropriate for practical use and to build a portfolio optimizer based on factor based investing. Some of the factors for consideration include Size, Value premium, Income and Yield, Momentum and Volatility. In particular, the intent is to study the momentum factor as applicable to Indian Capital markets and extend this into the broader portfolio construction process. With the evolution of capital markets over time, the basic principles on which some of the fundamentals of investing were built are being revisited. The classical Mean Variance Optimization (MVO) approach and the addendums to it have been questioned. Investor behaviour is now being deemed as an important input to the portfolio construction process.
-
INTRODUCTION
Mean-variance optimization is a key element of the data-based investing. It is the process of measuring an assets risk against its likely return and investing based on that risk/return ratio .A mean-variance analysis is a tool, that investors use to help spread risk in their portfolio. In it the investors measure an assets risk, expressed as the variance, then compares that with assets likely return.
The goal of the mean-variance optimization is to maximize an investments reward based on its risk. Once you calculate variance and the expected reward, the goal is to invest rationally based on your appetite for the risk. All the things being equal, when two securities have same expected return you should select the one with the lower variance. Likewise, when two assets have same variance, you should select the one with higher expected return.
-
HISTORICAL STUDY
Portfolio theory development took place through three historical phase: Traditional portfolio theory (TPT), Modern portfolio theory (MPT) and Post-modern portfolio theory (PMPT).TPT relied on analysis of individual securities. On the other hand, MPT relied on analysis of portfolio characteristics. It improved financial theory and investment practice and through an objective system-based approach, enabled the optimization of relationship between the expected return and the assumed risk. An even stronger and more precise framework for designing optimal portfolio came from PMPT, which avoids known MPT errors.
PMPT is an extended and advanced MPT. PMPT avoids the known MPT errors and provides a stronger and more precise framework for constructing optimal portfolios..
MPT defines risk as total return volatility around the mean value and is measured by variance or by standard deviation of return.
The Fischer black and Robert Litterman Model authored by Fisher Black and Robert Litterman published at Goldman Sachs , dated on June 20, 2014.They have included methodologies like Integrated and mixed-sleeve multi-factor portfolios. Advantage of this paper is it considers multiple factors and their impact. Disadvantage of this paper is Lack of standards in factor definitions. A Golden Combination authored by Markowitz published at VU University, Amsterdam , dated on May 16, 2015.They have included methodologies like Mean Variance optimization. Advantage of this paper is Conceptually superior ,easy to understand from first principles. Disadvantage of this paper is allows short selling and apply poorly specified time horizons
Rise of Factor Investing Asset Prices, Informational Efficiency, and Security Design authored by Lin William Cong, Douglas Xu published at Cornell University, USA , dated on November 28, 2016.They have included methodologies like Trading transparency distinction between bundles and derivatives. Advantage of this paper are it enhance returns, diversification and reduce the expected volatility within the equity portfolio. Disadvantage of this paper are Opportunity cost and Liquidity risk.
The Characteristics of Factor Investing authored by David Blitz,Milan Vidojevic published at VU University, Amsterdam,dated on July 2, 2018 .They have included methodologies like Integrated and mixed-sleeve multi-factor portfolios. Advantage of this paper is it considers multiple factors and their impact. Disadvantage of this project is lack of standards in factor definitions.
A Step-By-Step Guide to the Black-Litterman Model Incorporating User-specified Confidence Levels authored by Thomas M Idzorek published at Goldman Sachs, dated on November 3, 2019.They have included methodologies like Black-Litterman Model. Advantage of this paper is Realistic portfolio weights. Disadvantage of this project are Formulation of Views and degree of Uncertainty is subject to interpretation.
Mean Variance Optimization and Momentum Investing Momentum Investment Strategy Using a Hidden Markov Model authored by Markowitz published at Yonsei University, Seoul ,dated on August 28, 2020.They have included methodologies like Hidden Markov model. Advantage of this paper is Noticeable outperformance during particular periods of time. Disadvantage of this paper are high portfolio churn and high portfolio rebalance frequency.
-
Investment Pool
-
-
CONCEPT AND APPLICATION
The investment pool consists of ETFs from the 8 different countries from Asia and the benchmark ETF is from China. For testing purposes it is assumed that there are corresponding ETFs that will perfectly tract the performance of the countrys market index. Thus, the corresponding market historical index of 8 countries and benchmark where obtained and treated as the ETFs
-
Portfolio Selection Model
As for the portfolio selection model, mean-variance theory is applied in selecting for the optimal portfolio considering 8 other ETFs aside from China as the investment pool. The concept of mean-variance theory is that the investors will invest based on the mean return and the risk involve such that given similar returns (risk) with different risk (returns) rational investors will select the investment with the smallest risk (highest return). Considering investors have the different return and risk tradeoffs (), 5 sets of and 1- are used for back-test.
-
Performance Evaluation
The performance of the resulting portfolios are analyzed and compared to the benchmark. Basic statistics of mean and the standard deviations are analyzed. In addition, return distributions and the cumulative returns are also studied. Then, for a more accurate and rigorous comparisons pair-return difference T test is also applied. The null hypothesis of the test is that there is no significant difference between the returns of the MV portfolio and the benchmark. The alternative hypothesis of the test is that the return difference of MV portfolio and the benchmark is greater than 0. Note that outliers are first removed before performing the pair-return difference T tests.
-
-
CURRENT STATE
Before we can talk strategy and future real estate needs, its critical to evaluate your existing portfolio. A current state assessment helps identify all the supportive, unsupportive, and missing (gaps) assets in your CRE portfolio, while also taking into account the people, processes, and technology that impact your real estate. In order for real estate to align with business goals, we must understand your existing assetswhich are supporting your business and which no longer add value?
An effective current state assessment should have two goals in mind: understand your assets, liabilities, and oportunities within your CRE portfolio, and establish the baseline for all status quo costs. Projected cost savings will be compared against the status quo.
During the current state assessment phase, partner with a trusted real estate advisor to review all of the owned and leased properties within your portfolio. As you review, categorize the assets as supportive (add value to your business), unsupportive (do not support your goals), or missing (assets that should be added to your portfolio).
As part of this review, consider completing the following tasks: Conduct a lease and asset valuation for your real estate portfolio. Identify existing policies and internal processes.
Understand the tools and technologies you currently use.
When done correctly, you should walk away from the first phase of portfolio optimization with clear performance benchmarks, a utilization model of your CRE portfolio, and a baseline financial model.
Having a clear understanding of your commercial real estate portfolio is an essential step in optimizing your overall business strategy. By knowing which properties in your portfolio are no longer advantageous, you can shed those unnecessary investments and hone in on the ones that move your business forward.
-
HYPOTHESIS
Modern portfolio theory is refined portfolio management approach, and it offers how to choose portfolio with superior feasible expected returns. The mean-variance model is a cornerstone of the modern portfolio theory, in which risk is defined as possible variation of expected portfolio returns Then, absolute deviation portfolio optimization model , semi-absolute deviation portfolio optimization model mean-var model , mean-cvar model , multi-period semi-variance model, and nonlinear futures hedging model were been proposed based on this model .
In terms of inventory and the increased assets, Chi Guotaiset up a decision making model of loans optimization based on the total portfolio risk minimization of inventory and incremental loans. Hong Zhongcheng built a loan decision-making optimal on the objective of maximizing the total return of the inventory portfolio and the incremental portfolio. Yu Chao set up a optimal decision- making model for the incremental portfolio based on the maximum return probability. Yu Chao proposed optimal conditions to manage interest rates of risk immunization for all asset and liability portfolios can be achieved by establishing function relationships between abanks net value changes, incremental asset and liability, and stock asset-liability duration.
Hong Bowen et al. constructed a general model of the dynamic multi-objective optimal dispatch, and applied it in the day-ahead optimal dispatch of a typical PV- wind-battery-diesel microgrid. Tomás et al. proposed the application of Markowitzs portfolio
theory to the selection of the digital communication channel.
Existing literatures had made considerable progress, but there were still many problems that cannot get a better solution, the common problem was that ,it only reflected the risk control and optimization of the incremental assets, did not reflect the risk of all the assets.
Though a considerable number of research papers has been published for the portfolio selection problem, none has considered the optimization model with a defined investment ratio based on the inventory and increased assets. The paper is organized as follows, we will give a short discussion on the optimization principle of inventory and incremental assets. We will introduce decision- making models based on the inventory and increased assets. We will introduce portfolio models with a defined investment ratio based on the inventory and increased assets.
-
DESIGN AND IMPLEMENTATION
The design of the project consists of the following: The classical Mean Variance Optimization (MVO) approach and the addendums to it have been questioned. Investor behaviour is now being deemed as an important input to the portfolio construction process. Some of the factors for consideration include Size, Value premium, Income and Yield, Momentum and Volatility. In particular, the intent is to study the momentum factor as applicable to Indian Capital markets and extend this into the broader portfolio construction process.
Online analytical processing, or OLAP, is an approach to the answer multi-dimensional analytical queries swiftly in computing. OLAP is the part of broader category of business intelligence, which also encompasses relational databases.
The overview of this project is to understand the limitations of MVO, alternate approaches that may be more appropriate for practical use and to build a portfolio optimizer based on factor based investing.
To take bipartite weighted graph and compute modules by applying Newman's modularity measure in a bipartite weighted version to it and re-runs the algorithm several times, returning the most modular result, to stabilize modularity computation.
To build a portfolio optimizer based on Factor based investing and To explore the possibility of extending Momentum principles into the classical Mean Variance Optimization in Equity investing.
Figure 1: System Architecture
This figure 1 conveys the system architecture of Mean variance Optimization. In this figure we have user workstation which includes the user interface with the transaction linked to the computing engine and high performance server which has Online processing Database which computes the price, volume, company and the index.
Figure 2: Use Case Diagram
This figure 2 conveys use case diagram of the Mean Variance Optimization . The use case diagram has the factor selection, run back stimulation, details of the export portfolio and run portfolio performance attribution.\
-
RESULT AND DISCUSSION
The results obtained are discussed below:
-
Data Description
The closing market indexes of the 9 countries in Asia are collected from yahoo finance and investing.com. Wherein the return rates of each index are calculated which are assumed as return rates of corresponding ETF that exactly tracks the performance of underlying index. The collected data is from September 1, 2015 to August 20, 2018.
0.2000
0.1500
0.1000
0.0500
0.0000
-0.0500
Figure 1: Daily Return Rates
0.2000
0.1500
0.1000
0.0500
0.0000
-0.0500
Figure 2: Cumulative Return Rates
-
-
CONCLUSION
Mean-Variance optimization is a great concept but not very practical. Bayesian approach-based methods produce higher accuracy, but present challenges with transforming qualitative information. Factor-based approach seems rational; should not induce additional risks. The goal of the project is to build a portfolio optimizer based on Factor based investing while controlling portfolio risks. A mean-variance analysis is tool that investors use to help spread risk in their portfolio. In it the investors measure an assets risk, expressed as the variance, then compares that with the asset's likely return. The goal of the mean-variance optimization is to maximize an investment's reward based on its risk. It is the process of weighing risk, expressed as variance, against expected return. Mean-variance analysis allows the investors to find the biggest reward at a given level of risk or the least risk at a given level of return.
-
.REFERENCES
7031. 10.3390/su12177031.
[2] David Blitz 1, Milan Vidojevic 2, The Characteristics of Factor Investing, July 2, 2018 [3] Thomas M. Idzorek, A Step-By-Step Guide to the Black-Litter man Model Incorporating User-specified Confidence Levels, November 3, 2019 [4] Lin William Cong & Douglas Xu, Rise of Factor Investing: Asset Prices, Informational Effiiency, and Security Design, November 28, 2016 [5] Jay Walters, CFA, The Black-Litter man Model in Detail, June 20, 2014 [6] Wouter J. Keller, Adam Butler & Ilya Kipnis, Momentum and Markowitz: A Golden Combination, May 16, 2015