
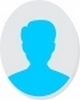
- Open Access
- Authors : Thedieudonne Kabeya Nahum, Allen Tam, Gopi Chattopadhyay, Leonard Kabeya Mukeba
- Paper ID : IJERTV13IS010043
- Volume & Issue : Volume 13, Issue 01 (January 2024)
- Published (First Online): 24-01-2024
- ISSN (Online) : 2278-0181
- Publisher Name : IJERT
- License:
This work is licensed under a Creative Commons Attribution 4.0 International License
Maintenance Strategy Optimization in Mineral Processing Multi-component Systems: A Case Study of Slurry Filtration Plant
Dieudonne Kabeya Nahum, Allen Tam, Gopi Chattopadhyay
Institute of Innovation, Science and Sustainability Federation University Australia
Victoria, Australia
Abstract Development of Maintenance Strategy in Mineral Processing Multi-Component Systems is a complex process, and its optimization helps in reducing costs and risks and enhancing performance of assets. A slurry filtration plant is considered as case study. Individual components failure rate and MTBF are determined to facilitate the development of a cost-effective maintenance plan. A cost function for predictive and preventive maintenance are proposed for the overall system, considering multi-component inter-dependence. The reliability of critical components is analyzed with Weibull model. Single-component age-specific maintenance-based algorithm is used to determine the preventive replacement times of all components reducing the total cost by reducing the total downtime of the system. This paper presents a general approach for the optimization of a mineral processing multi-component system in a context of economic dependence based on maintenance execution strategy. Illustrative example analyses MTBF for decisions on when the preventive maintenance can take place based on interactive data acquisition from SCADA on asset performance.
KeywordsModelling, optimization, multi-component system; economic dependence; maintenance strategy
-
INTRODUCTION
Engineering systems are generally subject to deterioration with age and/or use. For some systems (power plant, transport, etc.), the concern for safety makes it extremely important to make every effort to avoid failure in service to reduce the loss of production and/or profit. Maintenance of systems improves reliability and enhances safety. An optimal maintenance strategy is developed based on required availability and equipment integrity at the lowest possible costs (Wang H. 2002). A well-developed single- component maintenance plan facilitates the multi- component systems reliability management. In a multi-component asset, inter-dependence between components can be due to economic dependence, structural dependence, and stochastic dependence and/or failure interactions (Thomas, 1986), (Dekker, R., et al., 1997). Economic dependence means the costs can be reduced when multiple components are maintained in a coordinated manner for economies of scheduling scale (R. Laggoune, 2007). If several components are structurally linked, structural dependence applies for maintaining one component along with others linked components. Stochastic dependence occurs when the condition of one component influences the lifespan distribution of other components (Dekker, R., et al., 1997) or
Leonard Kabeya Mukeba
Institut Supérieur de Techniques Appliquées,
ESU-ISTA, Kinshasa, DR Congo
when external forces cause failures of more than one component at the same time (R. Dekker and R. Wildeman, 1997). The literature research shows majority of researchers work with systems by simplifying the problem with assumptions to keep mathematical modelling less complicated. Markov's analysis, based on the state space grows exponentially with the number of system components (R. Laggoune, 2007). Hence, heuristic models are developed for systems with several components. These models address unique cases (structure, constant failure rate, …). This paper presents a general method for the optimization of a multi- component system in series by developing an optimal maintenance plan as shown in figure 2. Equations (12) and
(13) provide preventive and corrective maintenance cost. The preventive replacement intervals for each component are calculated to reduce the overall cost whilst taking the system's total downtime into account, as expressed in equation (14)
-
MODELLING OF THE MULTI-COMPONENT SYSTEM
Let the system consist of components subjected to stresses. The given strengths of the components 1, 2, , , (+1) with parameters , = 1, , . Each component is subjected to a given stresses 1, 2, , , (+1) with components parameters , = 1, , . Stresses and strengths are assumed to be independent and are given as , . In this application
strengths and stresses refer to components design-
performance and operation solicitations causing failure respectively. The reliability of both systems, parallel and series arrangements of the components can be determined using the below theories.
-
Reliability of the parallel systems
For parallel multi-components, the reliability of the system is given by:
1 = [(1, 2, , , (+1) )
> (1, 2, , , (+1))] = [ > ]
= () ()
0 (1)
where = (1, 2, , , (+1)), = max (1, 2, , , (+1)) and the probability function. The
(0+1 ++)
expression of parallel system reliability in equation (1) takes
0
1
With 11<< (+ +
++
the system strength ratio
)
into consideration the sub-component stresses and strengths
where strengths remain higher over the system life. The
survival function under strength condition given in equation (2) is defined for [0, ].
considering sub-components life cycle under strength conditions. If the variable time is considered, the reliability of
such a system at a given time can be expressed in a contracted
form as:
() = [ > ] =
(1)+1 1 << ( >
, 2 > , , > )
=1
,=1
1 1
() = 1 () =
()
(9)
(2)
() =
(1)+1 exp(( +
+ +
With Rsys(t) the reliability of the system, Fsys(t) the probability
function of system failure and Ri(t) the reliability of component i.
=1
11<<
0 1
)) (3)
Survival functions given in equations (2) and (3) apply in the
determination of the multi-component systems efficiency under cumulative stress distribution.
Serial system with components
Figure 1:
Considering , the cumulative distribution
1, 2, , , (+1) of given in equation (4) is expressed
in terms of systems strengths over the operation life:
The below figure illustrates a preventive maintenance plan for
a serial system with components.
=1
() = 1
1
(1)+1 1 << exp((0
+ 1 +
Figure
+ )) (4)
Substituting with (3) and (4) into (1), the reliability of a
multi-component system can be expressed with consideration stresses and strengths from commissioning to operation in its entirety as given in equation (5).
2:
Preventive Maintenance strategy
The times t1, t2, t3, , are calculated between preventive
1 =
(1)+1 1 << (0 +
+ +
replacements of components 1, 2, 3, …, (Laggoune, R. et al.
=1
1 1
+1
2009). The decision when to shutdown such a system to pre-
) {=1(1)
1
1 + + )
× 11<<(0 + 1 + + + 0 +
} (5)
emptively replace other components, is based on the decrease in reliability and the increase of risk of failure incurred before the next scheduled time. During a corrective replaement of an
With 0 design-parameter independent of components run time and the stresses parameters at an initial state, i.e.,
commissioning phase.
-
Reliability of the series systems
component, the opportunity is seized to anticipate the replacement of other components. The total maintenance cost
per unit of time is obtained by using (12) and (13) into
equation (11):
++ )()+( + )()
For series multi-components, the reliability of the system is:
2 = (min(1, , ) > ) = ( > ) =
(0
() =
=1
0
0 ()
=1
(10)
0 () ()
(6)
This equation is the total maintenance cost of the system per
unit of time before streamlining the repair time. This
where = (1, , ) and exponentially distributed with . The survival function of is expressed in equation
(7).
() = exp () (7)
This equation is applied to determine components life under
conditions. From (7) and (4) in (6) the reliability of series system can be written as
(0+1 ++)
mathematical approach does not consider strengths and
stresses under operation conditions but instead the failure time.
indicates the component that fails first: = min ( ) ( = 1, 2, ). The opportunistic policy proposes grouping
replacements so as not to penalize the total availability of the system, this grouping is obtained by rearranging the times of
replacement by: = . where an integer; = min ();
= 1, 2, …, (Figure 2). The expression of the total cost then
becomes:
2 =
(1)+1 1 << (8)
=1
1 (+0+1++)
(, 1, 2, , ) =
=1
=1((0 + + =1 ),()+(0 + )())
()
=1
0 (11)
where , is the probability of system failure due to component . In comparison of equation (10), the expression
given in equation (11) is the total maintenance cost of the
system relative to components stresses.
-
-
MAINTENANCE COST STRUCTURE OF MULTI-COMPONENT SYSTEM
The maintenance cost consists of the fixed part relating to the
0
0
system and the variable part for each component. With : fixed cost induced by a corrective maintenance operation, : fixed cost induced by a preventive maintenance operation,
cost of the patch for component and
cost of preventive
care relating to component . The cost of preventive replacement of system components is given by:
When component i fails at time ti, the opportunity to replace component i fails at time ti, the opportunity to component j as
= 0 +
(12)
preventive maintenance is based on the analysis of its
=1
cost/profit balance according to the decision criteria:
p c c
The cost of preventive replacement expressed in equation (12)
is applied to determine individual components cost. The cost
of the system patch following the failure of component , including the cost of the preventive replacement of the
components during the opportunity is:
Cj (Rj(ti) Rj((k + 1))) < (C0 + Cj )Fj((k + 1)) . This
condition indicates the opportunity to inspect and/or replace
component j. The opposite condition restricts the component
to stay in place until next scheduled replacement.
V. INDUSTRIAL CASE STUDY
= + +
(13)
, 0
=1
The application of the proposed filtration process is found in upstream concentrate mineral processing wet plant. Filtration plant is a multi-component system in which the technology
-
MAINTENANCE STRATEGY OPTIMIZATION
The proposed model is based on the age-specific maintenance strategy for a single-component system and used in the algorithm process (Piresa C.R., 2019) to determine ages for each components preventive maintenance. The total expected replacement cost rate is expressed in equation (14).
( )+ [1 ( ) ]
mainly incorporates fluid and electric power to enable the separation of minerals in fine form from water through a porous polyethylene cloth used as filtering agent by pressing and blowing slurry feed in cake chamber. From metallurgy perspective, five filtration process steps can be considered as indicators to determine sub-components performance: (a) cake formation, (b) moisture reduction, (c) cake washing, (d) cake discharge, and (e) medium washing (B. Wills, et al., 2016). Based on the theory of Darcy and Poiseuille, the basic
() =
0 ()
(14)
filtration equation can be written as (Dahlstrom, 2003): n =
1 =
where the element of slurry volume is function
With () + [1 ()] the expected preventive
()
replacement cost per equipment cycle and 0 ()
the
of run time and the variation of blowing pressure is taken from
minimal to maximal pressure input. This mathematical
expected length of a failure cycle, T the age of preventive replacement, Cp the cost of preventive replacement, Cd the cost of failure and R(t) = 1 F(t) the reliability function
(Bassem S. et al., 2006), (Scarf P. A., Deara M., 1998). In this
approach, the optimization consists of determining the ti
replacement times that would minimize the total cost rate. The
resolution is obtained numerically by the Monte Carlo simulation according to the iterative algorithm shown in figure 3.
expression is basically a process approach for filters sizing and is applicable for filtration plant reliability in design phase. In addition, the mechanical deterioration of sub-components can be deducted from pressure and slurry flow aspects. The filtration plant considered in this case study has recorded several downtime affecting daily production key performance indicators as shown in Table 1. An asset management system to capture all downtime and main causes was implemented to facilitate the development of an effective maintenance strategy. Since this filter is multi-components system, a 6- month observation were conducted for failure rate and MTBF
determination. A process operation investigation was also conducted to examine the root-cause of those failures. However, the operational analysis results are Considered negligible in this approach. Relation (9) was applied to determine components failure and MTBF.
Fig. 4(a): Failure and MTBF for sub-components
Fig. 4(b): Overall failure in a period of 135 days
Figure 4 illustrate the filtration plant performance and Weibull distribution over a period of 135 days before maintenance strategy optimization. The numerical data given in table 1 were analysed in MS Excel.
Figure 5: Actual preventive and correct maintenance costs over a period of 6 months
The costs of corrective and preventive maintenance are calculated by relation (14) considering downtime, labour, parts, and other relative costs. Filter clothes and clothes bar sensors preventive maintenance cost reveal to be higher than the corrective maintenance cost, whilst the poly plates both costs look slightly the same. The below figures show the established ratio between the two costs for every single component. The application of multi-component systems maintenance plan in figure 2 allows to develop the horizontal pressure filter serial components maintenance plan and schedule based on their failure and MTBF; Table 2.
Figure 6: Corrective and preventive maintenance costs ratio
Figure 7: Saved cost on initial and actual preventive maintenance cost.
Figure 8: Components failure and TBF trend per scheduling group.
The initial preventive maintenance cost and the actual cost are differentiated to determine the saving cost per scheduled component group and the overall business cost saved; Figure
8. Approximately 34% of preventive maintenance cost is saved per scheduled group-components with an overall cost saving of 29%.
VI. CONCLUSION
The application of reliability improvement in a slurry pressure filter proved to be cost effective for interdependent serial components. The Weibull graph of Figure 4(b) plotted based on real data illustrates the continuous probability distribution of approximately 52% possible premature downtime within the first 100 operation days. The implementation of the
preventive maintenance plan for serial systems with
components shown in Table 2, demonstrates the effective
strategy with a cost saving of $40165 in five months. The algorithm here presented can provide a framework to guide future maintenance optimization. In future root cause analysis of component failures can be combined for enhanced life- cycle management of systems.
REFERENCE
[1] Wang, H. (2002). A Survey of Maintenance Policies of Deteriorating Systems. European Journal of Operational Research, 139, 469-489. http://dx.doi.org/10.1016/S0377-2217(01)00197-7 [2] Dekker, R. et al. (1997). Review of Multi-Component Maintenance Models with Economic Dependence. Mathematical Methods of Operations Research (1997) 45:411-435 [3] Thomas, L. (1986). A survey of maintenance and replacement models for maintainability and reliability of multi-item systems. Reliability Engineering 16:297309. [4] Laggoune, R. and Aissani, D. (2007). Optimisation du plan de maintenance dun système multi-composant. Laboratoire de Modélisation et dOptimisation des Systèmes. Séminaire sur les Techniques et Management de la Maintenance, STMM 2007 [5] Barry A. Wills and James A. Finch, (2016). Wills mineral processing technology. An introduction to the practical aspects of ore treatment and mineral recovery. 8th Edition, Elsvier, Canada. [6] Pires C.R. et. al., (2019). Mathematical model for maintenance planning of machine tools. 29th International Conference on Flexible Automation and Intelligent Manufacturing (FAIM2019), 2019, Limerick, Ireland. [7] Bassem S. et al. (2006). A suitable inspection policy definition for system with two modes of degradation. IFAC Proceedings Volumes. Elsevier, Volume 39, Issue 13, Pages 789-794 [8] Scarf P. A., DEARA M. (1998). On the development and application of maintenance policies for a two-component system with failure dependence, IMA Journal of Management Mathematics, Volume 9, Issue 2, March 1998, Pages91107, https://doi.org/10.1093/imaman/9.2.91
[9] Khairy a. H., D. N. Prabhakar D.N. Complex System Maintenance Handbook – Reliability Engineering Springer. [10] Laggoune R. et al. (2009). Opportunistic policy for optimal preventive maintenance of a multi-component system in continuous operating units. Computers & Chemical Engineering, Volume 33, Issue 9. [11] Alain Ratle et al. (2013). Availability Optimization for Series/ Parallel Systems using Evolutionary Algorithm, Inter-universityResearch Centre on Enterprise Networks, Logistics and Transportation (CIRRELT) and Department of Mechanical Engineering, 1065, Quebec, Canada.