
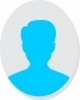
- Open Access
- Authors : Allois Kioko Luvai , John. P.O. Obiero , Christian T. Omuto
- Paper ID : IJERTV10IS080179
- Volume & Issue : Volume 10, Issue 08 (August 2021)
- Published (First Online): 30-08-2021
- ISSN (Online) : 2278-0181
- Publisher Name : IJERT
- License:
This work is licensed under a Creative Commons Attribution 4.0 International License
Land Use and Land Cover Change Assessment for Management of a Dam Reservoir (A Case Study of Maruba Dam, Machakos)
Allois Kioko Luvai Department of Crop Sciences, Pwani University, Kilifi, Kenya
John. P.O. Obiero
Department of Environmental and Biosystems Engineering University of Nairobi, Nairobi, Kenya
Christian T. Omuto
Department of Environmental and Biosystems Engineering University of Nairobi, Nairobi, Kenya
AbstractDam catchments are undergoing rapid deterioration due to land use and land cover dynamics. Land use and land cover (LULC) change is therefore an important factor that helps planners, designers and managers in fast-tracking sustenance of dam catchments. Therefore, an up to date spatio- temporal data can provide a perfect basis for developing plans and conservation programmes for enhancement of catchment areas. The objective of this study was to analyze the land use and land cover changes in Maruba dam catchment using Landsat satellite images for 1999, 2010 and 2020. Land use and land cover change was detected using supervised classification based on the maximum likelihood classifier algorithm in Idrisi Selva software. The catchment was classified into seven major LULC classes viz. water bodies, bare land, built-up areas, croplands, dense forest, sparse forest, rocks and sand. Temporal comparison of different quantities of LULC class conversions was achieved after analyzing change detection. The study revealed that LULC classes experienced either positive or negative change for the considered period of time. These changes were attributed to climatic factors and human activities within the catchment area. Dam catchments must therefore be subjected to LULC mapping on frequent basis as one way of gathering the catchment position for planning and management actions.
KeywordsLand use/land cover, catchment, remote sensing, change, detection)
-
INTRODUCTION
Land use refers to the manner in which land and its resources are utilized for sustenance of humankind. Land cover on the other hand refers to the vegetation type which appears on the land surface for instance crop lands, vegetation etc. [1]. Therefore, land use and land cover change (LULCC) refers to the modification of the earth's terrestrial surface due human influence, [1, 2]. Globally, noticeable land use and land cover changes have occurred [3]. These changes have resulted in fragmented ecosystems [4]. Land use and land cover changes are brought about by the combined effect of the complex socio-economic as well as environmental factors [4, 5]. The changes are influenced by the interplay between several factors and not a single one is responsible for such changes. However, the interactions between these factors vary from region to region which translates to different land use
and land cover types. Population growth has been considered as the most significant driver of LULC especially in developing countries [3]. Land use and land cover changes have brought about grave environmental concerns, most of which affect humankind. In this regard, LULC change has emerged as an important environmental issue whose effect cannot be ignored and it constitutes an important area of research [1]. Hence, research has endeavored to address the negative issues associated with LULC through monitoring and mediation while enhancing sustainable development of essential resources.
The demand for fostering sustainable management of many vulnerable landscapes has grown tremendously. The disruptions on the ecosystem brought about by LULC changes necessitate the need to incorporate such changes in addressing sustainability challenges associated with land management, food security, climate change mitigation and biodiversity loss. Reference [6] noted that LULC change is a product of management interventions (reforestation, soil erosion control, wildlife conservation and restoration of soil) which again trigger some land use decisions as well as responses. In many third world countries, development strategies which target to address vital landscape-related issues notably mitigating climate change, conserving biodiversity, reducing deforestation and improving living conditions for the rural population have gained momentum especially in dam catchment areas. Catchments are quite sensitive to land use and land dynamics. Changes in land cover for instance influence river flows and sediment generation within catchments. Further, a catchment's hydrological response and that of water in rivers basins is mostly influenced by land use changes. Therefore, catchment based assessment of land use and land cover change is critical for planning of catchment based integrated management approaches [7].
Maruba dam is the only source of water for Machakos town and its environs. Although the dam was rehabilitated in 2010, its reservoir is threatened by sedimentation. The sustainability of the dam reservoir is compromised by sedimentation. Little is still known with about to sediment discharge and sediment transport dynamics in the dam catchment. Surface runoff and soil erosion within the
catchment area and subsequent deposition in the dam reservoir are things to worry about. However, these processes cannot be understood without a clear picture of the land use and land cover dynamics in the catchment area. This study sought to characterize the current trend in land use and land cover change between 2000 and 2020 in Maruba dam catchment using remote sensing. Remote sensing has served as an important tool for classifying, identifying and analyzing land use and land cover using different techniques and various data sets [2]. Satellite image processing and use of radar information provides a basis for identifying and verifying changes that occur in land use at given spatio-temporal scales [2].
B. Data Sources
The study relied on three important datasets: digital elevation model (DEM) and satellite images (Table 1). Digital elevation models for Kenya at a spatial resolution of 30 m were obtained from the Shuttle Radar Topography Mission (SRTM) database available at http://opendata.rcmrd.org/datasets/kenya-srtm-dem-30meters. Landsat 8 OLI/TIR for 2000, 2010 and 2020 with a resolution of 30 m were downloaded from USGS website (https://earthexplorer.usgs.gov).
Table 1: Study datasets and their source
-
MATERIALS AND METHODS
A. Study Site
Maruba dam is located in the neighborhood of Machakos town, Machakos County, Kenya (fig. 1). The dam supplies water to Machakos town and its environs. The astronomic position of the dam catchment extends from 1o 26' 0'' S to 1o 32' 0'' S latitude and from 37o12' 0'' to 37o18' 0''E longitude. The elevation ranges between 1576 m and 2119 m above mean sea level. The catchment has an area of 4900 hectares and it serves as an important hydrological watershed in Machakos County. Topographically, the catchment lies between a slope gradient of between 0 and 50 % (fig. 2).
Figure 1: Study site
Figure 2: Topographic variation
-
Catchment Delineation
Raster based analysis was performed on the terrain data of the digital elevation model (DEM) with a resolution of 30 m by 30 m. Thereafter, the catchment boundary was then delineated using the Arc-Hydro extension tools embedded in ArcGIS software version 10.5.
-
LULC Classification Image Pre-processing
Maruba dam catchment was interpreted using remote captured data onboard TM and OLI sensors [8]. Multi- spectral satellite images (2000, 2010 and 2020) of the study area were imported into Idrisi Selva mage processing software. Pre-processing included geo-referencing, mosaicking and creation of image subsets based on the area of interest [9, 10].
Image Classification
Images are classified in order to convert them to thematic form [10]. The objective of image classification methodology is to categorize all the image pixels into LULC classes in an automatic manner. The outcome of image classification is transforming image data into thematic information [10]. Here, different spectral signatures obtained from Landsat datasets were assigned to different land use land cover types [11]. This was enabled by the reflectance characteristics from different land use and land cover types [10]. Images were classified using both supervised and unsupervised classification techniques [9, 11]. Prior information regarding a particular area is an important consideration when carrying out supervised classification because it furnishes the software with some unique training classes [3, 10]. It therefore the prerogative of the user to clearly define the original pixels that have similar spectral classes corresponding to some land cover classes. A global position system (GPS) was used to mark points deemed as representative of the different types of land cover during field work [8]. Consequently, supervised classification was executed in Idrisi Selva software based on the maximum likelihood classification [3, 11].
Accuracy Assessment
Classified land use and land cover data captured using remote sensing tools should be subjected to accuracy assessment before change detection analysis is done because it checks the how well the reference samples march with classified images [3, 8, 9, 10]. Accuracy assessment for each individual classification must be carried out to measure the usefulness of classification data [12]. In this study, accuracy assessment for 2020 satellite image was validated using ground truth information [9]. The overall accuracy was obtained by dividing the total sum of the sample units which had been correctly classified by the entire number of the sample units, [9, 10].
-
-
RESULTS AND DISCUSSIONS
The land use and land cover classification changes in 2000, 2010 and 2020 are presented in table 2 and figures 2, 3 and 4 for illustration and interpretation of the different land use and land cover classes.
The catchment was classified into seven land use and land cover classes viz. water bodies, bare land, built-up areas, croplands, dense forest, sparse forest, rocks and sand, (fig. 3 – 5). Bare lands, dense forest, grass land and sparse forest recorded negative changes between 2000 and 2020 (table 2).
These changes corresponded to 21 to 18 %, 10 to 4 %, 26 to 23 % and 18 to 10 % respectively. On the other hand, built-up areas, rainfed and irrigated agricultural lands, rocks and sands and water bodies recorded positive changes which ranged from 0.6 to 3.3 %, 23 to 38 %, 0.9 to 1 %, 1.2 to 1.8 % and
0.25 to 0.29 % respectively.
Table 2: Land use and Land cover Changes between 2000 and 2020
Figure 3: LULC map for 2000
Figure 4: LULC map for 2010
Figure 5: LULC map for 2020
Negative land use and land cover changes were an indication that the catchment experiencing various forms of degradation. Bare lands are dominant in the upper part of the catchment. This region is characterized by high slopes and as such barrenness is attributed to soil erosion. However, the negative change affecting bare lands would be an indication that some soil and water management aspects have been put into place. The negative LULC changes in dense and sparse forests and in grass lands indicate that such areas are replaced by agricultural lands. Similar trends in LULC change were also reported by [11]. This kind of change translates to loss of canopy cover and vegetation cover hence the soil is such areas are vulnerable soil erosion.
The positive LULC changes are noted in built-up lands, agricultural activities, rocks and sands and water bodies. These changes indicate the socio-economic influence by human kind. Increased area of built-up areas is an indication of the growing human population within the catchment. Hence, buildings have been put up to accommodate the rising population. Consequently, rise in human population means increased pressure on the available natural resources within the catchment. Such resources include soil, water, grasslands and forests among others. This explains why forest lands and grasslands have declined in size to accommodate such pressures. This is evident again from the increased area of cultivated lands [11]. It has also been noted that the area occupied rocks and sands has increased significantly. Rock outcrops are indicative of shallow soils within the catchment. This confirmed by a soil survey carried out in the area. Shallow soils are very vulnerable to soil erosion and the situation gets worse in areas that lack canopy and vegetation cover.
A very interesting trend is noted with increased area occupied by water bodies. Maruba dam reservoir is the only significant water body in the catchment. The study shows that
in the year 2000, the catchment area was about 12 hectares in size. However, after rehabilitation works on the dam reservoir in 2010, the area increased to 20 hectares. Between 2010 and 2020, the size of the dam reduced from 20 hectares to 14 hectares. This is an indication that the dam had lost 6 hectares in about 10 years. This loss in area was attributed to soil erosion which results from the numerous socio-economic activities within the catchment.
-
CONCLUSIONS
The study sought to understand the spatial and temporal magnitude of land use and land cover changes in Maruba dam catchment. LULC change was studied based on multi-spectral Landsat satellite image data for 2000, 2010 and 2020. Satellite image data analyses showed that significant LULC changes have occurred in Maruba dam catchment between 2000 and 2020. The study reported both negative and positive land use and land cover changes for considered period of time. The findings of the study showed that for the last two decades, vegetation cover (grasslands, dense and sparse forests) experienced a declining trend. The change detection analysis established that grasslands, dense and sparse forests (26 % to 23 %, 10 % to 4 % and 18 % to 10 % respectively) had lost credible size of area to agricultural activities (both rainfed and irrigated at 23 % to 38 % and 0.9 % to 1 % respectively). Notable negative change in water bodies was noted between 2010 and 2020 (0.42 % to 0.29 %). These were attributed socio-economic activities within the catchment which were reported to be on the rise due to population increase. The study concludes that socio-economic activities have significant contribution in catchment degradation.
The study proved that multi-spectral satellite images are quite successful in detecting comprehensive changes associated with land use and land cover changes in a changing environment can be detected. The study further proved that supervised classification of multi-spectral images is an important tool through which land use and land cover changes can be detected. This set of information serves as an important input in soil and water management plans.
-
RECOMMENDATIONS
The study recommends carrying out a detailed analysis based on primary and secondary data in order to identify the driving forces behind the socio-economic aspects and consequences which result from land use and land cover changes. The study should suggest alternative options which are possible in establishing sustainable practices for better management of resources in Maruba dam catchment.
REFERENCES
-
V.N.Mishra , P.K. Rai, and K. Mohan, "Prediction of Land use changes based on land change modeler (LCM) using Remote Sensing: A case study of Muzaffarpur (Bihar), India", J. Geogr. Inst. Cvijic. vol 64,
Issu. 1, pp. 111-127. 2014
-
A.Z.A. Razad, S.H. Samsudin, A. Setu, N.A. Abbas, L. M. Sidek, and
H. Basri, "Investigating the Impact of Land Use Change on Sediment Yield for Hydropower Reservoirs through GIS Application," IOP Conf.
Ser.: Earth Environ. Sci. 540 012037. 2020
-
A.J. Jande, N.G. Nsofor, and A. Abdulkadir (2019), "Assessment of Land Use and Lan Cover Changes and Urban Expansion using Remote Sensing and GIS in Gboko, Benue State, Nigeria," Journal of Research in Forestry, Wildlife and Environment, Vol 11, issu. 3, pp. 201 – 214. 2019.
[4] |
J.L.Kouassi, A.Gyau, L. Diby, Y. Bene and C. Kouame, "Assessing Land Use and Land Cover Change and Farmers Perceptions of Deforestation and Land Degradation in South-West Côte dIvoire, West |
[9] |
M.C. Cheruto, M.K. Kauti, P.D. Kisangau and P. Kariuki, "Assessment of Land Use and Land Cover Change Using GIS and Remote Sensing Techniques: A Case Study of Makueni County, Kenya", Journal of |
[5] |
Africa", Land 10, 429. 2021 B. Addae and N. Oppelt, "Land-Use/Land-Cover Change Analysis and |
[10] |
Remote Sensing & GIS, Vol. 5, issu. 4., pp. 1 – 6. 2016 A.A. Beyene, "Soil Erosion Risk Assessment in Nashe Dam Reservoir |
Urban Growth Modelling in the Greater Accra Metropolitan Area |
Using Remote Sensing, GIS and RUSLE Model Techniques in Horro |
||
[6] |
(GAMA), Ghana", Urban Science, Vol. 3, issu. 26. 2019. R.W. Kariuki, L.K. Munishi, C.J.C. Mustaphi, C. Capitani, A. |
Guduru Wollega Zone, Oromia Region, Ethiopia", Journal of Civil, Construction and Environmental Engineering, Vol. 4. issu. 1, pp. 1 – |
|
Shoemaker, P.J. Lane et al, "Integrating stakeholders perspectives and |
18. 2019 |
||
spatial modelling to develop scenarios of future land use and land cover change in northern Tanzania", . PLoS ONE, Vol. 16, issu. 2: |
[11] |
M.J. Syombua, "Land use and land cover changes and their implications for human-wildlife conflicts in the semi arid rangelands |
|
e0245516I.S. |
of southern Kenya. Journal of Geography and Regional Planning, Vol. |
||
[7] |
H. Hayicho, M. Alemu and H. Kedir, "Dam in Melka Wakena Catchment of Sub-Upper Wabe-Shebelle Watershed, South Eastern |
[12] |
6, issu. 5, pp, 193 – 199. 2013 E. Boakye, N.S. Odai, A.K. Adjei and F. Annor, "Landsat images for |
Ethiopia", Agricultural Sciences, Vol. 10, pp. 819 – 840. 2019 |
assessment of the impact of land use and land cover changes on the |
||
[8] |
G.W. Woldemariam and A.E. Harka, "Effect of Land Use and Land Cover Change on Soil Erosion in Erer Sub-Basin, Northeast Wabi |
Barekese catchment in Ghana. European Journal of Scientific Research, Vol. 22, issu. 2, pp. 269-278. 2008 |
|
Shebelle Basin, Ethiopia," Land, Vol. 9, issu. 111. 2020 |