
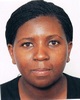
- Open Access
- Authors : Damaris Kerubo Oyaro , Zablon Isaboke Oonge , Patts Meshack Odira
- Paper ID : IJERTV10IS030012
- Volume & Issue : Volume 10, Issue 03 (March 2021)
- Published (First Online): 16-03-2021
- ISSN (Online) : 2278-0181
- Publisher Name : IJERT
- License:
This work is licensed under a Creative Commons Attribution 4.0 International License
Kinetic Modelling of Methane Production from Anaerobic Digestion of Banana Wastes
Damaris Kerubo Oyaro
Department of Civil and Construction Engineering, University of Nairobi, Nairobi City,
Kenya
Zablon Isaboke Oonge
Department of Civil and Construction Engineering, University of Nairobi, Nairobi City,
Kenya
Patts Meshack Odira
Department of Civil and Construction Engineering, University of Nairobi, Nairobi City,
Kenya
Abstract Energy is required to achieve interrelated economic, social and environmental aims of human development. Anaerobic digestion of agricultural wastes provides a solution to some of the environmental and health problems, which include production of renewable energy, waste management, and reduction of greenhouse gases. Agriculture is one of the main economic activities for Kenyans and among the fruits grown is the banana. Kenya produces about one million tonnes of bananas yearly, mostly for local consumption, of which the banana herb produces the fruits once in a lifetime. The waste from the banana plant includes leaves, stems, peduncles, peels and rejected fruits. This study aims to establish the methane produced from banana waste substrates, which are the leaf, stem and peduncle. Anaerobic batch digestion test conducted in triplicate batch systems, at mesophilic (37C) conditions at a period of 51 days using digested sludge from a wastewater treatment plant as the seeding sludge. The yields were 0.125, 0.132 and 0.062, m3CH4/kg oDM for the stem, peduncle and leaf, respectively. From the results of the batch fermentation, kinetic modelling was done using Fist Order Kinetic, Modified Gompertz and Logistic models was conducted, to determine the model that bests describes the degradation of complex substrates containing lignocellulosic. The study found out that the Modified Gompertz model predicted data had the best fit with the Experimental data.
Keywords Anaerobic batch digestion, methane, Fist Order Kinetic model , Modified Gompertz model and Logistic model.
-
INTRODUCTION
Global energy crisis as a consequence of declining quantity of fossil fuels coupled with the unprecedented rising crude oil prices. The crisis demands greater attention to alternative energy sources and revision of existing technologies. The burning of fossil fuels also emits carbon dioxide to the atmosphere which is a greenhouse gas associated with the global warming. There is also a rapid population increase especially in the developing countries, thus putting more pressure on the available fossil fuels, since the demand increases with increase in population. Hence, it is critical now not only to focus on sustained economic use of the existing limited resources but to identify new technologies and renewable resources that have the potential to cater for the increasing energy demand in addition to possessing other positive attributes such as being sustainable, globally available and easy to exploit (Nzila, Jo, Henri, Kiriamiti, & Herman,
2019). These are some of the main reasons that most countries are shifting towards green energy production, which includes wind energy, solar energy, hydro power, bio-fuel and biomass. For economical purposes, countries will opt for renewable energy that suits them most, depending on the geographical location, climate condition and availability of renewable sources. Biomass is a source of renewable energy, this energy source if developed sustainably it can be the answer to the environmental issues and definitely a potential solution to climate change. Biomass takes in carbon dioxide from the atmosphere during their growth stages to compensate for the carbon produced when it is burnt. With this potential zero carbon emission, the use of banana waste biomass as one of the sustainable energy mixes has great potential benefits (Jing Yan Tock, 2010).
In the rural areas of Kenya, whose population constitutes about two-thirds of the national population the main source of energy is traditional biomass in the form of fuel wood and charcoal. This fact is a cause for concern in view of the depletion of forests and consequent environmental 1 degradation arising from cutting of trees for fuel wood and burning of charcoal. Most of the people in the rural areas depend on agriculture as the main economic activity. To help reduce dependency on wood fuel, agricultural wastes could be used as a source of energy in the form of biogas, biodiesel and bioethanol. Agricultural wastes are the residues left behind after harvesting the food part of the crop, they include, stalks, stems, roots, leaves, cobs, and straws. The common practices of handling these wastes in Kenya are burning them before planting again new crops, feeding to livestock or leaving them on the land, thus, converting these wastes to bio-fuels will assist in environmental protection and also proper waste management.
Banana occupies a distinctive place in Kenyas national as well as household economy. It occupies 7.44% of the gross cropped area, but its share in total fruit area of Kenya is quite substantial at 55%. After harvesting of the banana fruit, the banana waste comprising of pseudo stem, peduncle, leaves, corms, rachis and waste fruits is mainly fed to livestock or left on the farm to decompose to form organic fertilizer. From various literatures, organic wastes have been seen to be good substrates for anaerobic digestion for the production of biogas.
Anaerobic Digestion is a robust technology for Biogas production and is a very attractive route to utilize certain
categories of biomass for meeting partial energy needs, mostly for domestic needs of heating and lighting. In fact, proper functioning of biogas system can provide multiple benefits to the users and the community resulting in resource conservation and environmental protection.
Anaerobic digestion is a complex process involving various bacterial populations and substrates. Usually, such processes contain a particular step, the so-called rate-limiting or rate-determining step, which, being the slowest, limits the rate of the overall process. A limiting step "that step which will cause process failure to occur under imposed conditions of kinetic stress (G.Lyberatos, 1999). Though the anaerobic process for production of biogas is a natural process that has been known for many years, there is a demand to comprehend and improve the process. Mathematical modelling comes in as a tool to aid in understanding the complex process of conversion of organic substances to biogas and other gases through various bacteria groups. With the current trends in advanced computer applications modelling comes in as an easy tool to demonstrate various complex processes. The whole modeling process (selecting a model structure, identifying the parameter values, and planning the experimental measurements) should be in coherence with the objective pursued. In general, the three most common objectives of using a model are: understanding the systems behavior and interaction of components; quantitatively expressing or verifying our hypothesis and predicting the behavior of the system in the future or under other similar circumstances (Andres Donoso-Bravo J. M.-L., 2011).
Various models are designed to meet specific objectives, they can be designed depending on the process understanding, dynamics of simulation, optimization and controls. Models contain unknown parameters, e.g., initial conditions, stoichiometry, and kinetic parameters which have to be estimated from experimental data. Parameter identication is a delicate task due the potentially large number of parameters an the scarcity of informative experimental data. Anaerobic digesters often exhibit significant stability problems that may be avoided only through appropriate control strategies. Such strategies generally require the development of appropriate mathematical models, which adequately represent the key processes that take place. The Mathematical Kinetic models used in Anaerobic Digestion helps in optimizing, predicting, simulating and monitoring process performance under different situations (Sagor Kumar Pramanik, 2019). The three Kinetic Models used in the study are the First Order kinetic Model, Modified Gompertz Model and Logistic Model.
This study is aimed at evaluating the anaerobic degradability of the three components of banana waste (leaf, peduncle and stem) in a batch digester with the help of an inoculum (untreated digested sludge from a waste water treatment plant) at mesophilic temperature range of 37C ± 2
C, in accordance with (VDI-4630, 2006). The results from the batch anaerobic digestion will be used for evaluating the best suitable Kinetic models (First Order kinetic model, Gompertz Model and Logistic Model) to explain the degradability of complex lignocellulosic substrates that is the leaves, stem and peduncle parts of banana wastes.
-
MATERIALS AND METHODOLOGY
-
Materials
The substrates used in this study were banana leaf (midrib and leaf blade), banana stem (leaf sheath) and banana peduncle after collection from the farm they were stored frozen. The inoculum was untreated digested sludge in accordance with DIN 38414-8. The inoculum was stored at 37C in such a way that the sludge reduced most of its own gas production and depleted the residual biodegradable organic material present in it.
The substrates were prepared for the batch test by shredding them into smaller sizes. This was done to provide large surface area for adsorbing the substrate that would result in increased microbial activity and hence increased gas production. The leaf was prepared by using the ratio of 30% midrib to 70% leaf blade, for the midrib it was shredded to a size of less than 5 mm but the leaf blade has already a high surface area due to its broad shape thus it was shredded to a size slightly larger than the midrib but enough to pass through the batch test bottle with ease. The stem and peduncle were shredded to particle sizes of less than 5 mm.
-
Substrates Characterization
The substrates were all in solid state and for the chemical composition, The Dry matter Total solids (TS) and organic dry matter content (oDM) was determined. The solids content of the samples was determined according to the European standards (EN 12879 and EN 12880). For determining the total solids (TS), samples with certain volume or weight were placed in ceramic vessels and dried in a drying oven at 105 ± 2°C for 24 hours until constant weight. After cooling in the desiccators, the samples were weighed for TS measurement. The samples then ignited at 550 °C for 2 hours in a furnace for Organic dry matter (oDM) determination. The oDM was determined by subtraction of the minerals content of the sludge sample (residual ash after ignition) from the total solids content. The formulas for calculation of Total solids and organic dry matter were as shown by equations 1 and 2.
TS (g/Kg) = {(Wd-Wv)/ (Ws-Wv)} x 1000 Equation 1 oDM (%) = {(Wd-Wi)/ (Wd-Wv)} x 100 Equation
2
Wv = weight of empty vessel.
Ws =Weight of vessel with sample.
Wd =Weight of vessel with dried sample. Wi =Weight of vessel with ignited sample.
-
Batch Test procedure
The fermentation test was conducted in accordance with (VDI-4630, 2006) and the apparatus were set up as shown in Figure 1. Batch fermentation were carried out in triple determinations, this also applied to the blank sample. The required amount of each substrate was weighed in triplicate and put into the fermentation vessel. Then water was measured and fed into the fermentation vessels, then followed by the seeding sludge. The blank was fed with equivalent amount of water instead of substrate. Before closing the fermentation vessels, the gas phase was flushed with nitrogen
for 10 seconds so as to remove residual oxygen from the gas phase. The vessels were then immediately sealed and put into the fermentation chamber, which was set up at a temperature of 37 C and this was maintained throughout the experiment, thus the fermentation batch test was carried out at mesophilic temperatures. The volume of the biogas produced was measured indirectly by a pressure measurement instrument. The test continued until the gas pressure changes reduced sufficiently to levels that showed there was very little or no further gas production. Thus, the fermentation batch test was on for 51 days. The recorded gas pressures after the 51 days were then collected and analyzed. After the batch test was complete, the pH of the fermentation residue was measured electrochemically using a pH meter. The COD of the residue (both Solid and liquid) was also determined. The gas produced was also subjected to gas chromatography to determine the composition of the gas.
Figure 1: Experimental apparatus in accordance with DIN EN ISO 11734 (Meier, Yuceer, Hinken, weichgrebe, & Rosenwinkel, 2009).
-
Kinetics study and Statistical Analysis
Kinetic modelling of the methane production helps to pre e r
Y Equation 1
Equation 2
Equation 3
Where,
Y(t) = cumulative methane production (mL/g oDM) Y= ultimate methane production potential (mL/g oDM) t= time in day
k = first-order model constant (1/day)
Rm = maximum methane production rate (mL/g oDM /day) e = 2.7183
= lag phase time (day)
The best fitting model will be used to predict the efficiency of an anaerobic digestion reactor and also analyze the mechanisms and metabolism involved in the Anaerobic digestion of banana wastes. Since each of the model has its strengths and weaknesses. To obtain the best fit model, the coefficient of determination (R2) and root mean square error (RMSE) were calculated for each model. R2 was calculated using Microsoft Excel 2019 for the First order kinetic model and statistical software, IBM SPSS Statistics 20 for Gompertz and Logistic Models. The root-mean-square error (RMSE) measures the differences between predicted by a model and the values observed it was calculated using Equation 6. The model with lower values of RMSE showed the best fit.
RMSE
Where
i is the model result
yi is the experimental result n is the data number
-
-
RESULTS AND DISCUSSIONS
dict th kinetic pa ameters therein predicting and
monitoring the performance of the anaerobic digestion reactor under different conditions. While designing full-scale systems, the results of kinetic modeling of methane production help to design and optimize the reactor parameters in comparison with the obtained experimental yields (Velusamy Mozhiarasi, 2020).
The kinetic models used in this study included Fist Order Kinetic Model (Equation 3), Modified Gompertz model (Equation 4) and Logistic Model (Equation 5). These models were used to fit the cumulative Methane data obtained from the Anaerobic batch tests. Microsoft Excel 2019 nonlinear regression was used to simulate the first-order kinetic model, and the kinetic parameters that is the first-order disintegration rate constant (k) and predicted methane yield (Y) were obtained by using the solver tool for Excel. The statistical software, IBM SPSS Statistics 20, was used for simulating and predicting the kinetic parameters (Rm maximum biogas production rate, lag phase time and Y predicted Methane yield) for modified Gompertz and logistic models. The equations for the Kinetic models used in the simulations are as shown in Equation 3, 4 and 5 respectively.
-
Total solids and organic dry matter
The values for total solids and organic dry matter calculated from Equation 1 and Equation 2 were as shown n Table 1.
Table 1: Total solids and Organic dry matter results and calculations
Substrate
Stem
Leaf
Peduncle
Wv (g)
42.5
48.6
41.5
Ws(g)
46.5
51.2
45.3
Wd(g)
42.7
49.2
41.8
TS (g)
0.2
0.6
0.3
TS (%)
5
23.08
7.89
TS (g/kg)
50
230.77
78.95
Wi(g)
42.51
48.66
41.54
oDM (%)
93.48
89.61
85.71
Where
Wv = weight of empty vessel.
Ws =Weight of vessel with sample.
Wd =Weight of vessel with dried sample.
Wi =Weight of vessel with ignited sample. TS= Total solids.
oDM = Organic dry matter.
The substrates (stem, leaf and peduncle) all had high organic dry matter. In the experiment setting the ratio of oDM substrate to oDM inoculum was less than 0.5 for all, thus there was minimal inhibition. The inoculums used had total solids of 56.42 g/kg and organic solids of 72.49%, thus its organic dry matter content was greater than 50% of the solid contents so the inoculum had sufficient microbial biomass for fermentation. The organic dry matter comprises the Biodegradable organic dry matter fraction and the Refractory organic dry matter. Knowledge of the Biodegradable organic dry matter fraction of the waste helps in estimation of the biodegradability of waste and estimation of biogas generation. Lignin is a complex organic material that is not easily degraded by anaerobic bacteria and constitutes the Refractory organic dry matter in organic wastes. Waste characterized by high oDM and low non-biodegradable matter is best suited to anaerobic digestion treatment. The composition of wastes affects the yield and biogas quality as well as the compost quality (Kumar, 2008).
-
Experimental Methane yield
Analysis of the biogas produced by gas enabled one to get the methane part of the gas. The methane quantity produced for the various substrates was as shown in Figure 2 and Table2. The gas quality was good as there was very little ammonia and hydrogen sulphide produced.
Table 2 : Methane yield from various components
Component
Methane Yield (m3CH4/kg oDM)
Stem
0.125
Peduncle
0.132
Leaf
0.032
Figure 1 : Methane yield for the substrates
The stem had a maximum methane yield of 124.45mLN/ g oDM. This can be compared to the results from (Khan, et al. 2009) which was found to be 0.256 m3CH4/kg oDM at a retention time of 35 days, thus the results obtained were half as much as those from (Khan, et al. 2009). One reason of the difference was probably, because the composition of the stem used by (Khan, et al. 2009) was both the leaf sheaths (pseudo
stem) and flower stalk (peduncle), Which in this case yields
256.78 mLN/ g oDM of Methane (124.45 mLN/ g oDM
+132.33 mLN/ g oDM) with a retention time of 35 days. The difference in methane production could be also attributed to the nature of pretreatment and storage of the substrate as was explained by (Kalia, Sonakya and Raizada 2000). Banana stem, due to its fibrous nature, is not very favourable for good methanogenesis. Various pre-treatment methods are required to breakdown the fibrous nature as was the case in (Kalia, Sonakya and Raizada 2000), the relatively higher yields could be due to the pre-treatment and temperature employed. The Peduncle produced 132.33 mLN/ g oDM of methane for the 51 days, fibrous residues when used the bacterial population needs time to adjust before being able to degrade the fiber component efficiently. There is no so much literature on the peduncle alone. Like in (Clarke, et al. 2008), the study used both waste green bananas and peduncle of which the methane yield was 0.398m3CH4/kg oDM.
Banana leaves from this study where 30% midrib and 70% leaf blade was used produced 62.34mLN/g oDM of methane. This is low, compared to the peduncle and the stem. (Mandal and Mandal 1997) had the value of biogas from banana leaves to be 0.0018m3 of biogas with a retention time of 90 days and from this study the biogas value was 445mL of biogas which is comparable results. The leaves are slowly degraded as even after the 51 days there was still some gas, though little being produced this was explained in (Reddy, et al. 2010), where by high concentration of hemicelluloses and lignin, inhibits the availability of cellulose for fermentation by the isolates. Hemicellulose and lignin are complex polymers and are not easily degraded by bacteria hence pretreatments of banana leaves are aimed at increasing the surface area of cellulose by removing lignin seal, solubilizing hemicellulose and disrupting crystallinity. Pretreated cellulosic biomass like water treated, alkali treated, acid treated and also dried banana leaves are used in fermentation for good results.
-
Kinetic Analysis and Model selection
The results of the kinetic modelling were represented in table 2 and figures 3,4 and 5. All the three models show a good fit with the experimental data but in order to get the most accurate model the values of R2 and RMSE were evaluated. The R2 was high for the Modified Gompertz Model and the RMSE was the lowest signifying that it is the most Robust model for maximum/ ultimate Methane Yield. This was also observed by (Zubayeda Zahana, 2017). The predicted methane yield difference from the experimental methane yield was also low with values below 10% which also showed that the modified Gompertz Model was most suitable for methane yield prediction.
The hydrolysis rate (k) from the first Kinetic order model was found to range between 0.09 to 0.21 per day, generally a higher value of k denotes faster degradation. For this case the hydrolysis was not so fast because of the lignin and hemicellulose components of the substrates, whereby these bonds have to be broken fast before degradation takes place hence the low values of k.
Table 3 : Estimated kinetic parameters for First Order Kinetic, Modified Gompertz and Logistic Models.
First Order Kinetics Model
Substrate
Hydrolysis rate constant (1/day)
R square
RMSE
Predicted methane yield (mLN/CH4/g oDM)
Measured methane yield (mLN/CH4/g oDM)
Difference in methane yield (%)
Stem
0.21
0.925
5.886
121.72
125
2.7
Leaf
0.091
0.969
2.427
55.29
62
12.1
Peduncle
0.10
0.94
7.511
129
132
2.33
Modified Gompertz Model
Substrate
Lag phase ()
(days)
Maximum Methane production rate (Rm) (mLN/CH4/g oDM)
R square
RMSE
Predicted methane yield (mLN/CH4/g oDM)
Measured methane yield (mLN/CH4/g oDM)
Difference in methane yield (%)
Stem
0.384
2.048
0.992
2.293
122.82
125
p>1.77 Leaf
4.392
23.322
0.974
2.382
57.00
62
8.77
Peduncle
0.496
12.314
0.994
2.340
121.349
132
8.78
Logistic Model
Substrate
Lag phase ()
(days)
Maximum Methane production rate (Rm) (ml CH4/g oDM)
R square
RMSE
Predicted methane yield (mLN CH4/g oDM)
Measured methane yield (mLN/CH4/g oDM)
Difference in methane yield (%)
Stem
0.496
22.739
0.987
2.938
122.486
125
2.05
Leaf
5.407
1.849
0.963
2.930
56.55
62
9.64
Peduncle
0.550
11.763
0.985
3.759
120.634
132
9.42
Figure 2: profile of measured and predicted methane Figure 3: profile of measured and predicted methane yield for stem yield for leaf
Figure 4: profile of measured and predicted methane yield for peduncle.
The maximum methane rate production (Rm) as shown by both Modified Gompertz and Logistic models, show that the substrates used were degradable. The lag phase () for the stem and the peduncle for both models was low as compared to the one of the leaves. According to (Sagor Kumar Pramanik, 2019) a high value could reduce the adaptation
ability of microorganisms to the reaction system and produce biogas within a longer timeframe
-
-
CONCLUSION
Based on the anaerobic batch tests performed in this study, based on VDI-4630 at mesophilic conditions of 37oC, conclusions can be drawn which include; the degradability of the substrates, the biogas yield and the methane yield. The rate of decomposition and hence the biogas production for the leaf was slow since it requires more than 51 days to fully degrade. The banana leaf has properties of a slowly degradable substrate due to the inhibition by the concentration of hemicelluloses and lignin in it, which inhibits the availability of cellulose for fermentation by the isolates. The methane production is also comparable to previous studies that include (Khan, et al. 2009) and (Mandal and Mandal 1997).
The banana waste apart from the leaf (due to inhibition), are suitable to be digested in one digester as none of the other wastes (stem and peduncle) exhibits inhibitory properties, when digested together, then the methane yield is economical for energy supply. The stem and peduncle due to their fibrous nature may require pre-treatment for optimal methane gas achievements.
Three kinetic models were used and the Modified Gompertz model was suitable as it had a high R2 values and A low RMSE values for all the substrates. thus, the Modified Gompertz model is best suited to predict the kinetics of the Anaerobic Digestion process and optimize the process parameters, thus improving the design and operations of the Anaerobic digestion process.
REFERENCES
-
A. Ware, N. P. (2017). Modeling methane production kinetics of complex poultry slaughterhouse wastes using sigmoidal growth functions. Renewable Energy, 50-59.
-
Andres Donoso-Bravo, J. M.-L. (2011, September 3). Model selection, identication and validation in anaerobic : A review. (P. Professor Eberhard Morgenroth, Ed.) Water Research, 45, 5347-5364.
-
Arzate, J. A. (2019). Modeling and simulation of biogas production based on anaerobic digestion of energy crops and manure. Berlin.
-
Borja Velázquez-MartÃ, O. W.-C.-R.-Q. (2018). Review of Mathematical Models for the Anaerobic Digestion Process. In Anaerobic Digestion. IntechOpen.
-
Chinenyenwa Nkeiruka Nweke, J. T. (2020). Kinetics of Anaerobic Digestion of Unripe Plantain Peels. Journal of Engineering Research and Reports, 7-17.
-
Clarke, W., Radnidge, P., Lai, T., Jensen, P., & Hardin, M. (2008). Digestion of waste bananas to generate energy in Australia. Waste Management 28 , 527533.
-
D. M. F. Lima, J. A.-M. (2016, December). Anaerobic modeling for improving synergy and robustness of a manure co-digestion process. Brazilian Journal of Chemical Engineering, 33(4), 871 – 883.
-
Elena Ficara, S. H. (2012). Anaerobic digestion models: A comparative study. 7th Vienna International Conference on Mathematical Modelling. Venna.
-
Fedailaine M, M. ,. ( 2015). Modeling of the anaerobic digestion of organic waste for biogas production. Procedia Computer Science 52 (pp. 730 737). ELSEVIER.
-
G.Lyberatos, I. (1999). Modelling of Anaerobic Digestion, A review
(Vol. 1). Patras, Greece: Global Nest, The International Journal.
-
Jing Yan Tock, C. L. (2010). Banana biomass as potential renewable energy resource: A Malaysian case study. Renewable and Sustainable Energy Reviews, vol. 14, issue 2, 798-805.
-
Kalia, V., Sonakya, V., & Raizada, N. (2000). Anaerobic digestion of banana stem waste. Bioresource Technology 73, 191 – 193.
-
Kumar, C. B. (2008). Dry continuous anaerobic digestion of municipal solid waste in thermophilic conditions. Thailand.
-
Mandal, T., & Mandal, N. K. (1997). Comparative study of biogas production from different waste materials. Energy Concerns Management .Volume 38 No 7, 679 -683.
-
Meier, S., Yuceer, S., Hinken, L., weichgrebe, D., & Rosenwinkel, K.-
H. (2009). Advanced interpretation of anaerobic batch tests conclusions for the practical operation. Internationale Wissenschaftstagung Biogas Science 2009 (pp. 335-344). Erding,Germany: Bayerische Landesanstalt für Landwirtschaft (LfL).
-
Nzila, C., Dewulf, J., Spanjers, H., Kiriamiti, H. and van Langenhove,
H. (2010). Biowaste energy potential in Kenya. Renewable Energy, 35(12):2698 2704.
-
Reddy, H. K., M., S., D., M. R., & Reddy, G. (2010). Coculture fermentation of banana agro-waste to ethanol by cellulolytic thermophilic Clostridium thermocellum CT2. African Journal of Biotechnology Vol. 9 (13), 1926-1934.
-
Sagor Kumar Pramanik, F. B. (2019). Performance and Kinetic Model of a Single Stage Anaerobic DigestionSystem operated at different successive operating stages for the treatment of food waste. Proccesses.
-
VDI-4630. (2006, April). Fermentation of organic materials, characterisation of the substrate, sampling, collection of material data, fermentation tests.
-
Velusamy Mozhiarasi, D. W. (2020, February 13). Enhancement of Methane Production from Vegetable, Fruit and Flower Market Wastes Using Extrusion as Pretreatment and Kinetic Modeling. Water Air Soil Pollut.
-
Zubayeda Zahana, M. O. (2017). Characterisation of Agro-industrial wastes and their anaerobic digestion/ co-digestion kinetic potential: A comparative batch study. Sixth International Symposium on Energy from Biomass and Wast, (pp. 14-17). Venice, Italy.