
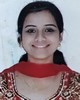
- Open Access
- Authors : Rakshitha. R, Dr. Usha Surendra
- Paper ID : IJERTV9IS050639
- Volume & Issue : Volume 09, Issue 05 (May 2020)
- Published (First Online): 30-05-2020
- ISSN (Online) : 2278-0181
- Publisher Name : IJERT
- License:
This work is licensed under a Creative Commons Attribution 4.0 International License
Integration of Coulomb Counting Method in Battery Management System for Electric Vehicle
Rakshitha. R
Phd Scholar, Christ University;
Assistant Professor RVITM
Dr. Usha Surendra
Professor EEE Department
Christ university
Abstract : The coulomb counting (CC) approach is widely
used in SOC estimation due to its simplicity and low
calculation cost. However, in practical applications, the lack
of error correction ability limits its accuracy due to the
measured noise in the practical occasion. To address the issue,
an improved CC (ICC) approach based on numerical
iteration is proposed in this paper. In the proposed approach,
a battery model based on a 2nd-order, RC circuit is first
formulated to determine the SOC-OCV curve, R-OCV curve,
and inner parameters. In the model, the slow dynamic and
fast dynamic voltages are described separately, and are
utilized for battery state assessment. Then, the SOC will be
estimated by the CC approach at the unsteady state but
through a numerical iteration approach at steady state.
Consequently, the accumulative SOC error from the CC
approach will be corrected when the numerical iteration
approach is applied.
INDEX TERMS: Improved coulomb counting (ICC), state of
charge (SOC), accumulative error correction, numerical
iteration, error accumulation rate.
I. INTRODUCTION:
To cut fossil energy consumption and mitigate the
greenhouse effect, electric vehicle (EV) has been widely
concerned. Battery system, as the energy provider in the
EV, requires a battery management system (BMS) to
ensure its safe operation [1]. Both over-charging and overdischarging
will reduce the lifespan of the battery system
or even lead to serious security accidents. Therefore,
monitoring the state of charge (SOC), which is defined as
the percentage of residual charge, has become one of the
key tasks of the BMS. However, due to the complex
electro-chemical behavior in a battery, estimating the exact
SOC for a battery is extremely difficult in practice. Hence,
the only available way is to perform an estimation of the
SOC based on the measurable external parameters of the
battery, such as current and voltage [2], [3]. The Coulomb
Counting (CC) approach and open-circuit voltage (OCV)
approach are the conventional methods to estimate SOC
[4]. Ideally, CC approach could obtain relatively accurate
SOC. However, in practice, there will be a large
accumulated error due to the unavoidable measured noise
and the lack of error correction ability. An enhanced CC
approach has been proposed to improve the SOC accuracy,
but the error is impossible to be eliminated fundamentally
[5]. As to the OCV method, the relationship curve between
SOC and battery OCV is usually applied for SOC
estimation. Nevertheless, the OCV can be unavailable
online due to the internal resistance and polarization
phenomena of the battery
Model-based SOC estimation algorithms are another hot
topic for SOC estimation [11]–[33]. In these algorithms,
OCV is calculated based on the battery model, and then the
SOC values obtained by OCV and CC are fused together
using a weight coefficient. Compared with CC, error
elimination ability is obtained. In general, model-based
algorithms, such as EKF, UKF and H infinite filters [11],
perform high accuracy and excellent stability if the battery
model is ideal. However, model error can hardly be
avoided in practice due to the complex chemical reaction in
the battery and the noise [3], [12], even through numerous
models have been proposed [13]–[24], such as the nth
Thevenin model [13], multi-time scale model [14]–[16]
and real-time updated model [17], [18]. To decrease the
influence caused by model error, many information
processing technologies are developed. The adaptive EKF
can update the process and measured noise covariance in
real-time [25]–[27]. The wavelet analysis technology has
been employed to preprocess the measured data, which
contain strong noise [28]. Algorithms using multiple filters
and information fusion technologies have also been
presented to improve the accuracy [29]–[33]. In these
algorithms, more factors such as state of health (SOH) and
models differences are considered. However, additional
technologies result in the increase of calculation cost and
implementation difficulty [9], [28]. In order to solve the
contradiction between SOC accuracy and calculation cost,
the ICC approach based on numerical iteration is proposed,
where the battery model based on a 2nd-order RC circuit is
built firstly with SOC-OCV curve, R-OCV curve and inner
parameters identified offline. Then, the SOC is estimated
by the CC approach in the unsteady state of the battery but
by a numerical iteration approach in the steady state.
Consequently, the accumulated SOC error from the CC
approach can be corrected through the numerical iteration
approach. Furthermore, a compensation coefficient is
employed into CC approach to reduce the error
accumulation rate. Hence, the proposed ICC approach
could make full use of the advantage of conventional CC in
the calculation rate and the numerical iteration approach in
error correction.
International Journal of Engineering Research & Technology (IJERT)
http://www.ijert.org ISSN: 2278-0181
IJERTV9IS050639
(This work is licensed under a Creative Commons Attribution 4.0 International License.)
Published by :
www.ijert.org
Vol. 9 Issue 05, May-2020
947
II. METHODOLOGY:
MODEL AND OFF-LINE PARAMETER
IDENTIFICATION OF LITHIUM-ION BATTERY A.
BATTERY MODEL
The nth-order Thevenin model is widely used for SOC
estimation because of its relatively high accuracy and
computation efficiency compared with other models [1].
Increasing the order of the Thevenin model will improve
the model accuracy further. However, higher order result in
additional calculation cost [17]. In the paper, a 2nd-order
RC model as shown in Fig. 1 is employed to estimate the
dynamic characteristics of the battery. The voltage source
UOCV represents the battery OCV. ro is the internal ohmic
resistance. The parallel RC network consists of rp1 and cp1
is aimed to model the fast dynamic voltage of the battery.
Similarly, the parallel RC network consists of rp2 and cp2
is aimed to model the slow dynamic performance. Up1 and
Up2 represent the polarization voltages across the two RC
networks, respectively. Uo and I are the terminal voltage
and load current, respectively. The electrical behavior of
the battery can be expressed as follows.
where, k denotes the present step, k-1 denotes the previous
step. T denotes the sampling period, QN denotes the
maximum available capacity of the battery, and τ1 and τ2
are the time constants of the RC networks. The time
constants denote the response rates of Up1 and Up2,
respectively. A higher constant means a longer time for the
polarization voltage to reach balance, for a given constant
load current
B. OFF-LINE PARAMETERS IDENTIFICATION To
verify the performance of the proposed algorithm, an
18650 Li-ion battery with the nominal capacity of 3Ah and
rated voltage of 3.6V was modeled. The parameter
identification tests were performed on a rek-8511
programmable electronic load, where the measuring
accuracy of voltage and current is 0.03%-0.05%. Then, the
data were processed in Matlab. Different from the
identification process in [2], the lumped resistance R,
which is the sum of ro, rp1 and rp2, was identified
independently. In practice, temperature and aging both
have effect on battery performance. Hence, the tests were
implemented under room temperature. Considering limited
charging process in the test, the influence of aging on the
battery performance is negligible.
1) IDENTIFICATION OF SOC-OCV CURVE:
The hybrid pulse power characterization (HPPC) test with
one hour interval and 5% SOC each time was carried out
[27]. Due to the employment of accuracy measuring
equipment, the SOC could be calculated accurately based
on measured data, and accuracy OCV could also be
accurately measured after a long rest of the battery. The
SOC-OCV relationship curve fitted by a 5-order
polynomial is shown in Fig. 2.
2) DENTIFICATION OF R-OCV CURVE In the paper,
the R-OCV curve will be utilized in the numerical iteration
approach, which will be discussed in Section III. Hence,
the identification accuracy of R is critical to identify the
OCV.
Generally, the lumped resistance R defined in (5) can be
calculated through the identification ofro,rp1 and rp2,
respectively. However, the curve fitting errors of ro, rp1
and rp2 will decrease the accuracy of R. Therefore, the
constant current discharg (CCD) test was carried out to
obtain better accuracy. During the CCD test, the battery
was discharged from 100% to 10% with the discharging
current of 0.8A. Consequently, the discharging process
would last more than three hours. As the Li-ion battery can
reach its steady state after only a few minutes [14]–[16],
the transient process into steady state can be neglected
compared with the whole discharging process. After the
dynamic voltage enters steady state, following equation
could be expressed as:
where, I(k) and Uo(k) are measured based on accurate
measuring instrument. Hence, SOC(k) could be obtained
through the CC approach. UOCV (k) could be calculated
using the SOC-OCV curve discussed in previous Part.
Then, R(k) could be solved through (6). The relationship
between OCV and R is shown in Fig.3. Obviously, the
OCV-R curve in Fig. 3 shows strong nonlinearity. Thus it
requires extremely high order polynomial to fit the curve.
To simplify, the OCV-R curve is decomposed into seven
linear segments according to the inflection points in the
OCV-R curve. The simplified curve is expressed as the
following equations:
3) IDENTIFICATION OF INNER PARAMETERS:
The other inner parameters are identified based on HPPC
test as well. Cooperating with the voltage response
expressions [2], [28], cp1, cp2ro,rp1 and rp2 can be
obtained through curve fitting method.
III. REAL-TIME STATE OF CHARGE
ESTIMATION USING THE IMPROVED COULOMB
COUNTING APPROACH A. STATE JUDGMENT
STRATEGY FOR THE BATTERY
The principle of conventional CC approach is shown in
(13). Due to the measured noise in I(k), an increasing
accumulative error will be introduced into the SOC.
Generally, if the battery model is precise, model-based
SOC estimation approaches, such as EKF and UKF,
perform well without accumulative error. However, the
accuracy of the model will be reduced at the unsteady state
of a battery and model-based SOC estimation approaches
have heavy calculation [16], [19], [34]. Hence, in the
proposed approach, CC approach is still employed at
unsteady state, and numerical iteration based on battery
model is proposed to correct the SOC value at steady state.
Before the proposed approach is implemented, whether the
battery has been steady should be assessed. As mentioned
previously, at the steady state of a battery, the current
flowing through the capacitors of the RC circuits in the
model are negligible. That is to say, it can be assumed that
the measured current I only goes through rp1 and rp2.
Therefore, following criterion could be formulated:
where, s1 and s2 are defined as the steady coefficients,
reflecting the divergence degree of the dynamic voltages
from steady state. In practice, the criterion can hardly be
achieved due to the complex operation condition of EVs.
To address the issue, a looser criterion is employed. This
criterion denotes that if Up1 and Up2 approximate to the
steady state in an adjacent time domain, their mean values
will also close to the steady state. Namely, the battery is in
quasi-steady state. The restrictions for the mean values are
aimed to eliminate the misjudgment caused by the
oscillation in I(k).
B. OCV ESTIMATION USING NUMERICAL
ITERATION A simple iteration algorithm is employed for
OCV estimation and then the SOC could be estimated
directly through SOCOCV curve. Corresponding principle
can be illustrated by (16)-(19).
The equation to be solved needs to be deformed into (16)
firstly, where 8(x) is named as iterative function. Further,
corresponding iteration structure can be discretized as (17),
where xl is the solution of the lth iteration and will be taken
as the input variable of the next iteration. If xl+1 satisfies
equation (18) after infinite iterations, it is treated as x ∗ ,
named the fixed point of 8(x), which is the approximate
solution of x. In practice, infinite iteration is unnecessary,
and xl satisfying equation is sufficient. ε is a pretty small
value, which is set according to the required accuracy.
Assuming that the battery is in steady state at k, following
iteration function can be obtained
International Journal of Engineering Research & Technology (IJERT)
http://www.ijert.org ISSN: 2278-0181
IJERTV9IS050639
(This work is licensed under a Creative Commons Attribution 4.0 International License.)
Published by :
www.ijert.org
Vol. 9 Issue 05, May-2020
948
Corresponding iteration structure is shown in (21). UOCV l
is the solution of the lth iteration, and UOCV l+1 is the
solution of the (l+1)th iteration. With iteration going on,
UOCV l+1 will converge to a certain value, which is the
solution of UOCV (k). It is notable that, the initial value of
UOCV 1 should be within the OCV range of the battery
C. COMPENSATION COEFICIENT TO PREVENT
ERROR ACCUMULATION IN CC APPROACH
Assuming that numeral iteration happens at ki . Then, the
accumulative SOC error Ei during each time interval can
be calculated by following equation
where, SOC(ki) is the precise SOC estimated by numerical
iteration. SOC(ki −1) is the last SOC value got by CC,
which contains the SOC error accumulated during [ki−1
+1, ki −1]. Therefore, the error accumulation rate α can be
estimated by following equation.
DESIGN OF ALGORITHMS USED:Algorithms used
for SOC and SOH Estimation are:
1. coulomb counting,OCV
2. kalman filter
3. Internal Resistance and Impedance Measurement
Method.
4. Neural Network
5. Support Vector Machine
6. Sliding Mode Observer
7. Fault Diagnostic Methods
8. fuzzy logic
9. incremental capacity analysis (ICA) method ,
10. Gaussian process regression method,
11. Bayesian network,
12. particle filter method,
13. Thevenin model.
State of charge (SOC) is a relative measure of the amount
of energy stored in a battery, defined as the ratio between
the amount of charge extractable from the cell at a specific
point in time and the total capacity. Accurate state-ofcharge
estimation is important because battery
management systems (BMSs) use the SOC estimate to
inform the user of the expected usage until the next
recharge, keep the battery within the safe operating
window, implement control strategies, and ultimately
improve battery life.
Traditional approaches to state-of-charge estimation, such
as open-circuit voltage (OCV) measurement and current
integration (coulomb counting), can be reasonably accurate
for cell chemistries with a significant OCV variation
throughout the SOC range, as long as the current
measurement is accurate. However, estimating the state of
charge for battery chemistries that exhibit a flat OCV-SOC
discharge signature, such as lithium iron phosphate (LFP),
is challenging. Kalman filtering is a promising alternative
approach that circumvents these challenges with a slightly
higher computational effort. Such observers typically
include a nonlinear battery model, which uses the current
and voltage measured from the cell as inputs, as well as a
recursive algorithm that calculates the internal states of the
system, including state of charge.
However, estimating the SOC for modern battery
chemistries that have flat OCVSOC discharge signatures
requires a different approach. Extended Kalman filtering
(EKF) is one such approach that has been shown to provide
accurate results for a reasonable computational effort.
1. Coulomb Counting Method.
The Coulomb counting method is associated with
monitoring the input and the output current continuously.
Since capacity is the integral of current with respect to
time, by measuring the input and the output current, change
in capacity or capacity degradation of a battery can be
measuredeasily.Inthismethod,SoHiscalculatedbydividingm
easured capacity (after discharging the battery to 0% SoC
International Journal of Engineering Research & Technology (IJERT)
http://www.ijert.org ISSN: 2278-0181
IJERTV9IS050639
(This work is licensed under a Creative Commons Attribution 4.0 International License.)
Published by :
www.ijert.org
Vol. 9 Issue 05, May-2020
949
value) to its
ratedcapacity.Itisanextensivelyusedmethodbyresearchersfor
itssimplicity.But,theaccuracyofthismethodisnotveryhigh.
Therefore, to improve its accuracy, for example, Ng et al.
proposed a smart coulomb counting method to estimate
both SoC and SoH accurately. Similarly, an adaptive
neurofuzzy inference system (ANFIS) was modeled in the
paper [51]. It considered the cell’s nonlinear characteristics
to get the relationship between SoC and open circuit
voltage (OCV) at different temperatures. During the
estimation of SoC, at some random OCV and temperature
,modelingofcellcharacteristicswasdonebyANFIS.Theassess
mentwas done on the cell level instead of the pack level for
better precision.
The coulomb counting method, also known as ampere hour
counting and current integration, is the most common
technique for calculating the SOC. This method employs
battery current readings mathematically integrated over the
usage period to calculate SOC values given by
where SOC(t0) is the initial SOC, Crated is the rated
capacity, Ib is the battery current, and Iloss is the current
consumed by the loss reactions. The coulomb counting
method then calculates the remaining capacity simply by
accumulating the charge transferred in or out of the battery.
The accuracy of this method resorts primarily to a precise
measurement of the battery current and accurate estimation
of the initial SOC. With a preknown capacity, which might
be memorized or initially estimated by the operating
conditions, the SOC of a battery can be calculated by
integrating the charging and discharging currents over the
operating periods. However, the releasable charge is
always less than the stored charge in the charging and
discharging cycle. In other words, there are losses during
charging and discharging. These losses, in addition with
the self discharging, cause accumulating errors. For more
precise SOC estimation, these factors should be taken into
account. In addition, the SOC should be recalibrated on a
regular basis and the declination of the releasable capacity
should be considered for more precise estimation.
Enhanced Coulomb Counting Algorithm:In order to
overcome the shortcomings of the coulomb counting
method and to improve its estimation accuracy, an
enhanced coulomb counting algorithm has been proposed
for estimating the SOC and SOH parameters of Li-ion
batteries. The initial SOC is obtained from the loaded
voltages (charging and discharging) or the open circuit
voltages. The losses are compensated by considering the
charging and discharging efficiencies. With dynamic
recalibration on the maximum releasable capacity of an
operating battery, the SOH of the battery is evaluated at the
same time. This in turn leads to a more precise SOC
estimation.
Technical Principle
The releasable capacity (Creleasable), of an operating
battery is the released capacity when it is completely
discharged. Accordingly, the SOC is defined as the
percentage of the releasable capacity relative to the battery
rated capacity (Crated), given by the manufacturer.
A fully charged battery has the maximal releasable capacity
(Cmax), which can be different from the rated capacity. In
general, Cmax is to some extent different from Crated for a
newly used battery and will decline with the used time. It
can be used for evaluating the SOH of a battery.
When a battery is discharging, the depth of discharge
(DOD) can be expressed as the percentage of the capacity
that has been discharged relative to Crated,
where Creleased is the capacity discharged by any amount
of current. With a measured charging and discharging
current (Ib), the difference of the DOD in an operating
period (Ʈ) can be calculated by
where Ib is positive for charging and negative for
discharging. As time elapsed, the DOD is accumulated.
DOD(t) = DOD(t0) + ΔDOD
To improve the accuracy of estimation, the operating
efficiency denoted as ŋ is considered and the DOD
expression becomes,
DOD(t) = DOD(t0) + ηΔDOD
with ŋ equal to ŋc during charging stage and equal to ŋd
during discharging stage. Without considering the
operating efficiency and the battery aging, the SOC can be
expressed as
SOC(t) = 100% – DOD(t)
International Journal of Engineering Research & Technology (IJERT)
http://www.ijert.org ISSN: 2278-0181
IJERTV9IS050639
(This work is licensed under a Creative Commons Attribution 4.0 International License.)
Published by :
www.ijert.org
Vol. 9 Issue 05, May-2020
950
Considering the SOH, the SOC is estimated as SOC(t) =
SOH(t) – DOD(t) .
Figure 1 shows the flowchart of the enhanced coulomb
counting algorithm. At the start, the historic data of the
used battery is retrieved from the associated memory.
Without any information for a newly used battery, the SOH
is assumed to be healthy and has a value of 100%, and the
SOC is initially estimated by testing either the open circuit
voltage, or the loaded voltage depending on the starting
conditions. The estimation process is based on monitoring
the battery voltage (Vb) and Ib. The battery operation
mode can be known from the amount and the direction of
the operating current. The DOD is adding up the drained
charge in the discharging mode and counting down with
the accumulated charge into the battery for the charging
mode. After a correction with the charging and discharging
efficiency, a more accurate estimation can be achieved. The
SOC can be then estimated by subtracting the DOD
quantity from the SOH one. When the battery is open
circuited with zero current, the SOC is directly obtained
from the relationship between the OCV and SOC. It is
noted that the SOH can be reevaluated when the battery is
either exhausted or fully charged, and the battery operating
current and voltage are specified by manufacturers. The
battery is exhausted when the loaded voltage (Vb) becomes
less than the lower limit (Vmin) during the discharging. In
this case, the battery can no longer be used and should be
recharged. At the same time, a recalibration to the SOH can
be made by reevaluating the SOH value by the
accumulative DOD at the exhausted state. On the other
hand, the used battery is fully charged if (Vb) reaches the
upper limit (Vmax) and (Ib) declines to the lower limit
(Imin) during charging. A new SOH is obtained by
accumulating the sum of the total charge put into the
battery and is then equal to SOC. In practice, the fully
charged and exhausted states occur occasionally. The
accuracy of the SOH evaluation can be improved when the
battery is frequently fully charged and discharged. finally
simple calculation and the uncomplicated hardware
requirements, the enhanced coulomb counting algorithm
can be easily implemented in all portable devices, as well
as electric vehicles. In addition, the estimation error can be
reduced to 1% at the operating cycle next to the
reevaluation of the SOH.
Figure: Flowchart of the enhanced coulomb counting
algorithm.
Charging and discharging methods of coulomb counting:
International Journal of Engineering Research & Technology (IJERT)
http://www.ijert.org ISSN: 2278-0181
IJERTV9IS050639
(This work is licensed under a Creative Commons Attribution 4.0 International License.)
Published by :
www.ijert.org
Vol. 9 Issue 05, May-2020
951
V. CONCLUSION
In the paper, an ICC approach with real-time error
correction ability is proposed. The SOC is estimated online
by the CC approach at unsteady state, leading a much
higher estimation rate. At steady state, numerical iteration
approach can accurately eliminate the accumulated SOC
error of CC approach, leading a much higher accuracy than
traditional CC approach. The numerical iteration approach
is based on a 2ndorder RC circuit model, where its
parameters were identified offline during HPPC and CCD
tests. Hence, the proposed approach could combine the
advantages of CC approach and model-based approach
together. Furthermore, a compensation coefficient α is
employed into the CC approach to reduce the error
accumulation rate. Experimental results suggest that the
SOC error of ICC is effectively limited within 1% and its
calculation cost is 94% lower than that of EKF. Therefore,
it provides beneficial guidance for the real-time SOC
estimation in EVs.
REFERENCES:
[1] Z. Li, J. Huang, B. Y. Liaw, and J. Zhang, ‘‘On state-of-charge
determination for lithium-ion batteries,’’ J. Power Sources, vol.
348, pp. 281–301, Apr. 2017.
[2] W.Yan,B.Zhang,G.Zhao,S.Tang,G.Niu,andX.Wang,‘‘Abatteryman
agementsystemwithalebesgue-samplingbasedextendedKalmanfilter,’’
IEEE Trans. Ind. Electron., vol. 66,
no. 4, pp. 3227–3236, Apr. 2019.
[3] C. Huang, Z. Wang, Z. Zhao, L. Wang, C. S. Lai, and D. Wang,
‘‘Robustness evaluation of extended and unscented Kalman filter
for battery state of charge estimation,’’ IEEE Access, vol. 6, pp.
27617–27628, 2018
[4] S. Piller, M. Perrin, and A. Jossen, ‘‘Methods for state-of-charge
determination and their applications,’’ J. Power Sources, vol. 96,
no. 1, pp. 113–120, Jun. 2001.
[5] K. S. Ng, C.-S. Moo, Y.-P. Chen, and Y.-C. Hsieh, ‘‘Enhanced
coulomb counting method for estimating state-of-charge and stateof-
health of lithium-ion batteries,’’ Appl. Energy, vol. 86, no. 9,
pp. 1506–1511, Sep. 2009.
[6] S.G.Li,S.M.Sharkh,F.C.Walsh,andC.N.Zhang,‘‘Energyandbattery
management of a plug-in series hybrid electric vehicle using fuzzy
logic,’’ IEEE Trans. Veh. Technol., vol. 60, no. 8, pp. 3571–3585,
Oct. 2011.
[7] J. Chen, O. Ouyang, C. Xu, and H. Su, ‘‘Neural network-based
state of charge observer design for lithium-ion batteries,’’ IEEE
Trans. Control Syst. Technol., vol. 26, no. 1, pp. 313–320, Jan.
2018.
[8] E. Chemali, P. J. Kollmeyer, M. Preindl, R. Ahmed, and A. Emadi,
‘‘Long short-term memory networks for accurate state-of-charge
estimation of Liionbatteries,’’
IEEETrans.Ind.Electron.,vol.65,no.8,pp.6730–6739,
Aug. 2018.
[9] G. O. Sahinoglu, M. Pajovic, Z. Sahinoglu, Y. Wang, P. V. Orlik,
and T. Wada, ‘‘Battery state-of-charge estimation based on
regular/recurrent Gaussian process regression,’’ IEEE Trans. Ind.
Electron., vol. 65, no. 5, pp. 4311–4321, May 2018.
[10] H. Chaoui and C. C. Ibe-Ekeocha, ‘‘State of charge and state of
health estimation for lithium batteries using recurrent neural
networks,’’ IEEE Trans. Veh. Technol., vol. 66, no. 10, pp. 8773–
8783, Oct. 2017.
International Journal of Engineering Research & Technology (IJERT)
http://www.ijert.org ISSN: 2278-0181
IJERTV9IS050639
(This work is licensed under a Creative Commons Attribution 4.0 International License.)
Published by :
www.ijert.org
Vol. 9 Issue 05, May-2020
951