
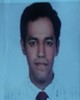
- Open Access
- Authors : Rachakonda Shri Rama Akshay , Rajesh Joseph Abraham
- Paper ID : IJERTV10IS080176
- Volume & Issue : Volume 10, Issue 08 (August 2021)
- Published (First Online): 30-08-2021
- ISSN (Online) : 2278-0181
- Publisher Name : IJERT
- License:
This work is licensed under a Creative Commons Attribution 4.0 International License
Improved Load Following in a Unilateral Market with STATCOM SMES
Rachakonda Shri Rama Akshay
Department of Avionics
Indian Institute of Space Science and Technology Thiruvananthapuram, India
Rajesh Joseph Abraham
Department of Avionics
Indian Institute of Space Science and Technology Thiruvananthapuram, India
Abstract This paper presents the advantages of integrating Static Synchronous Compensator (STATCOM) and Super Magnetic Energy Storage (SMES) for load following in a deregulated power system under a unilateral market. Load Following (LF) is an essential ancillary service that regulates the generation output power to meet the fluctuating load demand. In a deregulated power system, the network lines may not deliver the contracted power efficiently between Generation Companies (GENCOs) and the Distribution Companies (DISCOs) due to line congestion or short-term loss of line or generation leading to a spike in load demand. STATCOM SMES combination alleviate both the congestion and the short- term rise in load demand improving small signal stability and power transfer capability. A sample two area deregulated power system has been presented in the paper with linear first order model of STATCOM SMES for LF. The integral controller gains of both the areas have been derived to minimize quadratic performance index using Genetic Algorithm (GA). The simulation results obtained from MATLAB / SIMULINK show satisfactory improvement in the peak overshoots and settling times when compared to those without STATCOM SMES combination.
KeywordsUnilateral Market; Load Following; STATCOM; SMES.
-
INTRODUCTION
Lately, the electrical power system has been shifting their operation from a conventional regulated structure to a deregulated structure. The vertically integrated power system, where a single utility managed and operated generation, transmission and distribution has been unbundled. The private players have been encouraged to organize GENCOs, DISCOs, Transmission Companies (TRANSCOs) and Independent System Operators (ISOs) as separate entities [1]. The consumers have the option to choose economical services from multiple GENCOs, DISCOs and TRANSCOs. Thus, the private players have been forced to manage the entities economically and laydown tariff policies suitable for consumers. In conventional power system, the load demand in a control area is satisfied by the generating unit in the same control area. However, in a deregulated power system a DISCO can contract load demand from one or more GENCOs in same or different control areas. In a control area, a controller commands the GENCOs to commit to the generation based on the load demand contracts such as unilateral contract, bilateral contract or contract violation, between GENCOs and DISCOs [2]. The control command is derived by an error signal known as Area Control Error (ACE). Many heuristic algorithms have been implemented to
optimize the controller gains in the literature. A whale optimization based Proportional Integral (PI) controller has been presented in [3]. A cascaded Tilt Integral (TI) Tilt Integral Derivative (TID) controller to regulate frequency using Water Cycle Algorithm (WCA) has been implemented in [4]. A multi- area multi- source Automatic Generation Control (AGC) considering Modified Virus Colony Search (MVCS) has been presented in [5]. However, the control of a deregulated power system incorporating STATCOM SMES has not been attempted in the literature.
The key operational concern in the deregulated power system has been delivering the contracted load between GENCOs and DISCOs. The contracted power has to be within the transfer limit of the network transmission lines. The sudden load demand, line outages or short period generation loss causes the transmission line to operate beyond the transmission limit [6]. As the transmission line operate beyond the rated limits, the line losses increase and may lead to cascade line failure [7]. The ancillary services such as Load Following (to track sudden load demand), reactive power support (to enhance transmission line transfer limit) have been crucial to operate deregulated power system efficiently.
Maintaining frequency constant is important to interchange the power among the control areas as well as for constant speed applications. The load demand and power generation balance are maintained in the system to limit frequency within stable limit. The power generating units regulate the output power to meet fluctuating load demand through load following [8]. Traditionally, power plants with high ramp rate such as gas turbine power plants, fuel cell power plants, hydroelectric power plants, pressurized water reactor nuclear power plant etc. have been performing LF, as they can adjust the power output quickly [9- 12]. Recently, semi-conductor-based power converters, have been developed for custom energy storages such as SMES, Battery Energy Storage (BES), Plugged in Electrical Vehicles etc. for faster load levelling applications [13, 14].
The loading capacity of the transmission lines is constrained by thermal limits, dielectric limits and stability limits [15]. The stability limitations on the transmission lines have been overcome by FACTS devices and the conclusive limitation on the loading capacity are the thermal limit and dielectric limits. FACTS devices enable flexible control of power flow, thereby, increasing line loading capacity and minimize line congestion. In addition to the above advantages, STATCOM, has been reported to damp power oscillations and improve Bus voltage stability [16, 17].
Considering the advantages of the STATCOM SMES stated above, this paper has investigated the benefit of integrating STATCOM SMES in a sample two area deregulated system for LF in a unilateral contract scenario. An integral controller has been implemented for the controlling ACE. The gains of the integral controller have been searched to optimize quadratic performance index using Genetic Algorithm (GA). The linearized first order model of SMES for LF has been presented in section II. In section III, linearized STATCOM model has been derived to regulate tie line power deviations. The detailed two area deregulated power system, along with the unilateral contract description has been given in section IV. The optimization of quadratic performance index i.e., Integral Square Error (ISE) using GA has been presented in section V. Section VI, presents the simulation results using MATLAB / SIMULINK and the paper is concluded in section VII.
-
SMES MODELLING
SMES is an electromagnetic energy storage device. It has a loss less superconducting coil through which DC current flows. The energy is stored in the magnetic field created by the DC current [18]. The current through the coil does not vary instantaneously as the coil is inductive in nature [15]. An electronic converter controls the voltage across the coil such that power is absorbed from the grid or injected into the grid as shown in Fig. 1. The incremental current through the SMES coil is given as
(1)
where, is the incremental change in voltage across the coil which is given as
(2)
A negative feedback gain (KI) has been implemented in [19], such that the inductor current restores to the rated inductor current after a sudden load demand. with negative inductor feedback is given as
(3)
The SMES control block diagram is shown in Fig. 2.
-
STATCOM MDELLING
Before you begin to format your paper, first write and save STATCOM is a shunt connected Voltage Source Converter (VSC) based FACTS device that regulates the output capacitive or inductive current independent of the grid voltages [20]. With the frequency deviation as auxiliary control input, STATCOM has been used for power oscillation damping [21]. STATCOM acts a capacitor to reduce the congestion in the transmission line when load demand increases. However, when the load demand drops STATCOM
acts at the inductive limit to congest the active power flow.
The tie line power in steady state is given as
(4)
where V1, V2 are the bus voltages in the area 1 and area 2 respectively and 12 is the load angle between the two buses. x is the impedence of the transmission line connecting bus 1 and bus 2. Linearization of (4), around equilibrium point we get
(5)
Assuming the voltage deviations to be negligible, the STATCOM linearized model is shown in Fig. 3 and given as
(6)
-
TWO AREA MODEL WITH STATCOM SMES
The deregulated two area power system model analysed for LF is shown in Fig. 5. The control areas consist of two reheat generating units each. A Generation Rate Constraint (GRC) nonlinearity of 10 % per min [22] has been considered for each of the units. A STATCOM SMES combination has been implemented in area 1. The linearized state space equation for the deregulated system is given as
(7)
where A, B and are matrices of appropriate dimensions and X, U and P are as given below.
[X] = [f1, f2, Ptie12, PG1, PRH1, PG2, PRH2, PG3, PRH3, PG4, PRH4, PT1, PT2, PT3, PT4, PSMES, PSTATCOM] [U] = [u1, u2], [P] = [PD1, PD2]Each GENCO can participate in meeting the load demand from DISCO based on an agreed upon contract which can be visualized as DISCO Participation Matrix [23] as shown below.
where contract participation factor, cpfij is the fraction of the total load from jth DISCO to ith GENCO. The number of rows in DPM correspond to the number of GENCOs and the number of columns in DPM is equal to the number of DISCOs. Sum of the all the entries in a column in this matrix are unity. In our case since there are four GENCOs and DISCOs (m=4 and n=4), the DPM is a 4×4 matrix.
Fig. 1. SMES Schematic Diagram
Fig. 2. SMES Control Block Diagram
The power contracted by the ith GENCO with the DISCOs
is
It may be noted that, as there are no load contracts with DISCOs outside the same area, the remaining rows and columns of DPM are zero. The DISCOs in area 1 have load demand of PL1=PL2 = 0.1 p.u. MW. The parameters used in simulation are given in Table I.
Rated Area Capacities
PR1, PR2
1200 MW
Generator gain constants
KP1, KP2
120 (Hz/p.u.
MW)
Generator time constant
Tp1,Tp2
20 s
Turbine time constant
TT1,TT2,TT3
,TT4
0.3 s
Reheat time constant
TRH1,TRH2, TRH3,TRH4
10 s
Governor time constant
TG1,TG2,TG
3,TG4
0.08 s
Speed Regulation
R1, R2, R3, R4
2.4 (Hz/p.u. MW)
Frequency Bias Constant
B1,B2
0.4249
Synchronizing Coefficient
T12
0.0866
SMES Gain
Ksmes
0.03 p.u. MW
SMES time constant
Tsmes
0.1 sec
STATCOM Gain
Kstat
1
STATCOM time constant
Tstat
0.5 msec
Rated Area Capacities
PR1, PR2
1200 MW
Generator gain constants
KP1, KP2
120 (Hz/p.u.
MW)
Generator time constant
Tp1,Tp2
20 s
Turbine time constant
TT1,TT2,TT3
,TT4
0.3 s
Reheat time constant
TRH1,TRH2, TRH3,TRH4
10 s
Governor time constant
TG1,TG2,TG
3,TG4
0.08 s
Speed Regulation
R1, R2, R3, R4
2.4 (Hz/p.u. MW)
Frequency Bias Constant
B1,B2
0.4249
Synchronizing Coefficient
T12
0.0866
SMES Gain
Ksmes
0.03 p.u. MW
SMES time constant
Tsmes
0.1 sec
STATCOM Gain
Kstat
1
STATCOM time constant
Tstat
0.5 msec
TABLE I. THERMAL SYSTEM, STATCOM, SMES PARAMETERS [21] [28]
(8)
where PLj= total demand of jth DISCO and cpfij= contract participation factor. The scheduled power flow in the tie line at steady state is, Ptie12, scheduled = (Demand of DISCOs in area 2 from GENCOs in area 1)- (Demand of DISCOs in area 1 from GENCOs in area 2). Thus,
(9)
As in the traditional case, the respective Area Control Error (ACE) signal is generated using this tie line error signal as
(10)
(11)
In the unilateral contract case, the DISCOs demand power from the GENCOs in the same area and the following DPM is considered
Fig. 3. STATCOM Control Block Diagram
-
GENETIC ALGORITHM
The optimization algorithms search for the best solution in the search space and compare the fitness of each solution until a satisfactory solution is obtained [24]. Genetic algorithm (GA) [25] is an evolutionary algorithm which has been implemented for various applications [26].
The GA algorithm begins with initializing a random population. The population is encoded as chromosomes. The chromosomes are tested for the minimum value of the cost function based on which, the selection procedure takes place [27]. The selected chromosomes produce the next generation solutions by crossover and mutation and this process continues until the best values of Ki1 and Ki2 are achieved for which the cost function value lies between the confidence band before reaching the maximum number of iterations.
The cost function chosen for optimization is
(13)
It may be noted that w1, w2 and w3 are appropriate weights assigned to frequency deviations and tie line power deviations respectively. In this work equal weights have been assigned for f 1, f 2 and Ptie12 since these deviations should go to zero as quickly as possible and hence the normalized weight gains are unity each.
The genetic algorithm parameter values used are given in Table II. The optimized integral gains (Ki1, Ki2) without and with STATCOM-SMES combination in area 1 for different area participation factor combinations (apf) are presented in Table III.
It may be observed that the optimal gain settings with STATCOM-SMES are higher than those without STATCOM- SMES. The cost function values against the number of generations with and without STATCOM-SMES is shown i Fig. 4.
TABLE II. PARAMETERS FOR GENETIC ALGORITHM
Population
100
Number of Iterations
100
Crossover Rate
0.9
Elite Count
10
Initial Penalty
10
Penalty Factor
100
Mutation Rate
0.001
With STATCOM SMES
Without STATCOM SMES
apf1
apf2
Ki1
Ki2
Ki1
Ki2
0.9
0.1
0.77
0.39
0.6
0.4
0.75
0.25
0.51
0.35
0.47
0.17
0.5
0.5
0.08
0.06
0.07
0.05
0.25
0.75
0.06
0.05
0.07
0.07
0.1
0.9
0.1
0.08
0.02
0.06
With STATCOM SMES
Without STATCOM SMES
apf1
apf2
Ki1
Ki2
Ki1
Ki2
0.9
0.1
0.77
0.39
0.6
0.4
0.75
0.25
0.51
0.35
0.47
0.17
0.5
0.5
0.08
0.06
0.07
0.05
0.25
0.75
0.06
0.05
0.07
0.07
0.1
0.9
0.1
0.08
0.02
0.06
TABLE III. OPTIMIZED INTEGRAL GAIN CONSTANTS IN AREA 1
(a)
(b)
Fig. 4. Fitness Function value vs No. of Generations for (a) with STATCOM SMES (b) without STATCOM SMES
-
SIMULATION RESULTS
The two-area deregulated power system is simulated using MATLAB/SIMULINK with RK-4 method. Figs. 6 and 7 depict the deviations in area frequencies. It is evident that, with the STATCOM-SMES combination the frequency deviations are damped out faster and with reduced peak overshoots and undershoots. At steady state Ptie12, actual = Ptie12, schedule, since DISCOS in an area demand power from GENCOS in the same area only. Hence, from (9), tie line schedule power Ptie12, schedule = 0.
Fig. 8 shows the actual tie line power flow which clearly indicates an improvement in the response with STATCOM- SMES combination. Using (8), the deviation in power generation outputs is given as, PG1 = (0.5×0.1) +(0.5×0.1) =
0.1 p.u. MW. Similarly, PG2= 0.1 p.u. MW and PG3= PG4=
Fig. 5. Load Following Block Diagram of Two Area
0 p.u. MW. As depicted in Fig. 9,
PG1 and PG2 settles down to 0.1 p.u. MW each and PG3=PG4=0.
-
CONCLUSION
This paper has investigated the advantages of the linearized model of STATCOM SMES combination in a deregulated power system for unilateral contract. A STATCOM has been used to improve the line loadability of the tie line, while, an SMES compensates sudden load demands, thereby improving the LF. The simulation results show that, with STATCOM and SMES, the peak drop in frequency deviation has been minimized. Also, the tie line power oscillations damping has been improved. This paper has considered only unilateral contract transactions in a deregulated power system, while, contracts such as bilateral contract and contract violation needs to be investigated further.
Fig. 6. Frequency deviation (f1) in area 1
Fig. 7. Frequency deviation (f2) in area 2
Fig. 8. Actual Tie Line Power Deviation
(a)
(b)
(c)
(d)
Fig. 9. Deviations in Power Generated by (a) GENCO 1 (b) GENCO 2 (c) GENCO 3 (d) GENCO 4
REFERENCES
-
Pappachen, Abhijith, and A. Peer Fathima. "Critical research areas on load frequency control issues in a deregulated power system: A state- of-the-art-of-review." Renewable and Sustainable Energy Reviews, vol. 72, pp. 163-177, 2017
-
Parmar, KP Singh, S. Majhi, and D. P. Kothari. "LFC of an interconnected power system with multi-source power generation in deregulated power environment." International Journal of Electrical Power & Energy Systems, vol. 57, pp. 277-286, 2014.
-
Kumar, Rajiv, and V. K. Sharma. "Whale optimization controller for load frequency control of a two-area multi-source deregulated power system." International Journal of Fuzzy Systems, vol. 22, pp. 122-137, 2020.
-
Kumari, Sandhya, and Gauri Shankar. "Maiden application of cascade tiltintegralderivative controller in load frequency control of deregulated power system." International Transactions on Electrical Energy Systems, vol. 30, pp. e12257, 2020.
-
Ghasemi-Marzbali, Ali. "Multi-area multi-source automatic generation control in deregulated power system." Energy, vol. 201, pp. 117667, 2020.
-
Reddy, S. Surender. "Optimal placement of FACTS controllers for congestion management in the deregulated power system." International Journal of Electrical and Computer Engineering, vol. 8, pp. 1336, 2018.
-
Zarrabian, Sina, Rabie Belkacemi, and Adeniyi A. Babalola. "Reinforcement learning approach for congestion management and cascading failure prevention with experimental application." Electric Power Systems Research, vol. 141, pp. 179-190, 2016.
-
Locatelli, Giorgio, Sara Boarin, Andrea Fiordaliso, and Marco E. Ricotti. "Load following of Small Modular Reactors (SMR) by cogeneration of hydrogen: A techno-economic analysis." Energy, vol. 148, pp. 494-505, (2018).
-
Wang, Yifan, Debangsu Bhattacharyya, and Richard Turton. "Dynamic Modeling and Control of a Natural Gas Combined Cycle Power Plant for Load-following Operation." In Computer Aided Chemical Engineering, Elsevier, vol. 47, pp. 101-106, 2019.
-
Bizon, Nicu. "Real-time optimization strategies of Fuel Cell Hybrid Power Systems based on Load-following control: A new strategy, and a comparative study of topologies and fuel economy obtained." Applied Energy, vol. 241, pp. 444-460, 2019.
-
Killingtveit, Ã…nund. "Hydropower." In Managing Global Warming, Academic Press, pp. 265-315, 2019.
-
Hui, Jiuwu, and Jingqi Yuan. "Chattering-free higher order sliding mode controller with a high-gain observer for the load following of a pressurized water reactor." Energy, vol. 223, pp. 120066, 2021.
-
Olabi, A. G., C. Onumaegbu, Tabbi Wilberforce, Mohamad Ramadan, Mohammad Ali Abdelkareem, and Abdul Hai AlAlami. "Critical review of energy storage systems.", Energy, pp. 118987, 2020.
-
Jain, Prateek, and Trapti Jain. "Application of V2G and G2V coordination of aggregated electric vehicle resource in load levelling." International Journal of Emerging Electric Power Systems, vol. 19, 2018.
-
Narain G. Hingorani, and Laszlo Gyugyi. Understanding FACTS: concepts and technology of flexible AC transmission systems., Wiley- IEEE Press, 2000.
-
Ramirez, Juan M., and Jose L. Murillo-Perez. "Steady-state voltage stability with StatCom." IEEE Transactions on Power Systems, vol. 21, pp. 1453-1454, 2006.
-
Beza, Mebtu, nd Massimo Bongiorno. "An adaptive power oscillation damping controller by STATCOM with energy storage." IEEE Transactions on Power System, vol. 30, pp. 484-493, 2014.
-
Buckles, Warren, and William V. Hassenzahl. "Superconducting magnetic energy storage." IEEE Power Engineering Review 20, no. 5 (2000): 16-20.
-
Banerjee, S., J. K. Chatterjee, and S. C. Tripathy. "Application of magnetic energy storage unit as load-frequency stabilizer." IEEE Transactions on Energy Conversion, vol. 5, pp. 46-51, 1990.
-
Singh, B., R. Saha, Ambrish Chandra, and Kamal Al-Haddad. "Static synchronous compensators (STATCOM): a review." IET Power Electronics, vol. 2, pp. 297-324, 2009.
-
Padiyar, Krishna R. "FACTS controllers in power transmission and distribution.", New Age International, 2007.
-
K. Sudha and R. V. Santhi, Robust decentralized load frequency control of interconnected power system with generation rate constraint using type-2 fuzzy approach, International Journal of Electrical Power & Energy Systems, vol. 33, pp. 699707, 2011.
-
V.Donde, M. Pai, and I. A. Hiskens, Simulation and optimization in an agc system after deregulation, IEEE Transactions on Power Systems, vol. 16, pp. 481489, 2001.
-
S. Sivanandam and S. Deepa, Genetic algorithms, In Introduction to genetic algorithms, Springer, pp. 1537, 2008.
-
D. E. Goldberg, Genetic algorithms. Pearson Education India, 2006.
-
K.-F. Man, K.-S. Tang, and S. Kwong, Genetic algorithms: concepts and applications [in engineering design], IEEE Transactions on Industrial Electronics, vol. 43, pp. 519534, 1996.
-
Hassanat, Ahmad, et al. "Choosing mutation and crossover ratios for genetic algorithmsa review with a new dynamic approach." Information, vol. 10, pp. 390, 2019.
-
R. J. Abraham, D. Das, and A. Patra, Automatic generation control of an interconnected hydrothermal power system considering superconducting magnetic energy storage, International Journal of Electrical Power & Energy Systems, vol. 29, pp. 571579, 2007.