
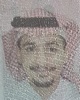
- Open Access
- Authors : Fisal Tobaigy , Mohammed Alamoudi , Omer Bafail
- Paper ID : IJERTV12IS030142
- Volume & Issue : Volume 12, Issue 03 (March 2023)
- Published (First Online): 29-03-2023
- ISSN (Online) : 2278-0181
- Publisher Name : IJERT
- License:
This work is licensed under a Creative Commons Attribution 4.0 International License
Human Development Index: Determining and Ranking the Significant Factors
Fisal Tobaigy, Mohammed Alamoudi , and Omer Bafail Department of Industrial Engineering, Faculty of Engineering, King Abdulaziz University, P.O. Box 80204, Jeddah 21589, Saudi Arabia.
AbstractThe Human Development Index (HDI) is a powerful tool that provides a comprehensive measure of a nation's overall well-being. This index was developed by the United Nations Development Programme (UNDP) in 1990 and has since become a widely used measure for assessing and comparing the standard of living of countries around the world. The HDI is composed of several key indicators that capture different aspects of human development, such as health, education, and income. Thus, a better understanding of the economic and social progress of countries can be seen through the HDI. In this study, several factors were investigated using multiple linear regression to test their relationships with the HDI. Four independent factors were significant among nine factors, as follows, skilled labor force, R&D expenditure, tourism and export & import. This study uses linear programming to figure out how much development a country needs in these areas to be number one on the HDI index, which in turn will improve the overall quality of life.
KeywordsHuman Development Index; united nations development; multiple linear regression; linear programming; sustainable development
-
INTRODUCTION
As a statistical instrument, the Human Development Index (HDI) evaluates how far forward a country is in political, educational, health care, and other critical areas of development [1][4]. Individuals' well-being, academic achievements, and standard of life significantly impact a nation's social and economic growth [5]. The HDI was founded by Mahbub ul Haq in 1990 and is currently used by the United Nations Development Programme (UNDP) to assess nations' degree of development [6]. The index is calculated using a four-factor methodology that considers a nation's health and wealth based on its life expectancy at birth, anticipated years of education, mean schooling years, and Gross National Income (GNI) per capita [7]. The HDI is used to rate nations in UNDP's yearly report. Since it incorporates all of the most critical social and economic variables liable for economic progress. It is considered one of the most outstanding instruments to monitor a country's level of development. The first Human Development Report was released in 1990, and it presented a fresh way of thinking about how to improve people's lives. The aim of human development, or the human development strategy, is to improve people's quality of life by focusing on increasing their access to opportunities and resources, rather than focusing solely on increasing the wealth of the economy in which people live. The UNDP is the principal organization for worldwide development, helping people in 170 nations and territories. The aim of human development strategy is to aid nations in achieving the sustainable development goals by strengthening policymaking, leadership, partnership, and institutional capacity, as well as
bolstering resilience. Human development results can vary significantly amongst nations with similar per capita incomes, and the HDI can be used to explain the discrepancies [8]. A significant disparity in HDI can exist across nations with comparable socioeconomic indicators, such as GDP per capita, if, for instance, one country has a substantially longer average life expectancy and more excellent literacy rates [9]. These differences prompt discussion of health and education policy in the public sphere as people strive to pin down why some nations seem able to accomplish goals that others cannot [10]. Differences across nations, provinces, sexes, ethnicities, and other socioeconomic groups are brought to light using the HDI.
The HDI was created to highlight the importance of people, or more specifically, their access to opportunities to live fulfilling lives. Besides traditional economic growth data like gross domestic product (GDP), assessing a country's capacity for resident development gives another criterion for judging that nation's degree of development. If two nations have almost similar GNI per capita, for instance, but create vastly different human development results, the HDI may be used to analyze the reasons behind this. Many people who support the HDI believe it may be used to kick off more fruitful discussions on public policy.
Human advancement is a notion of social welfare within the subject of global development centered on studying human situations through the lens of the capability framework. Human development is assessed using the HDI, which provides an alternate framework to the conventional emphasis on economic growth to wholly comprehend the concept of progress and its relation to equity issues [11]. The six cornerstones of human growth include sustainability, equality, empowerment, collaboration, productivity, and safety.
-
Equality entails fairness for every individual where both men and women have equal rights to health care and education.
-
Sustainability this pillar suggests that everyone should be able to make a livable wage and benefit from a more equitable allocation of resources.
-
Productivity provides for the full involvement of people in income generation processes. It also provides for the need for governments to create more effective and efficient programs for the people
-
Empowerment the opportunity to have a say in one's future and the choices that affect their well-being.
-
Cooperation entails collaboration and belongingness to groups and societies to enhance mutual enrichment and social meaning.
-
Security entails the free and safe provision of development opportunities with the assumption that they will not suddenly disappear in the future.
-
Dimensions of HDI
As a statistical tool and a single index that assesses a nations accomplishments and achievements, HDI aims to determine the three essential components of human advancement; education, health, and decorous living standard. The tool informs about the changes and improvements in human life through its significant social and economic indicators; thus, an appropriate tool to keep records of a nations economic progress.
-
Education
This dimension is measured in two levels; the average schooling years for a nations residents and the anticipated schooling years that children have at the mean age of beginning school. The United Nations indicates that the mean maximum schooling years is 18, and the average full years of schooling is 15.
-
Long and Healthy Life
This index illustrates the life years that a nations citizens will enjoy. This component is calculated as the average life expectancy at birth in every country, and it is normalized to zero when the life expectancy at birth is twenty years and one when it is eighty-five years.
-
Standard of Living
The UNDP uses the adjusted accurate per capita GDP indicator to assess the components of decent standards of living. This metric is typically normalized so that it equals 1 when the actual per capita GDP is $75,000 and zero when the indicator is $100, allowing for a straightforward comparison across countries with wildly different quality of living.
-
-
The Impetus for the Study
There are some sectors whose development will spur expansion in other related fields, as wellas in the country as a whole. However, the development of other sectors has a minimal effect on the growth of other sectors and the country as a whole. As a result, when it comes to identifying the areas that have the greatest impact on the country's development, it can be determined by the amount of development required to create a strategic plan, as well as the amount of progress the country will make in the coming years and its position among other nations. Therefore, it is crucial to determine which factors have the greatest impact on the development of countries; when these factors are improved through additional research, education, health, and standard of living will improve, resulting in a higher human development index and a high HDI rank. This study employs the multiple regression analysis tool to analyze the relationship between HDI as a dependent variable and several independent variables. Moreover, a correlation model will be presented to support and confirm the model Furthermore, a linear programming model will show how much development is needed for a country to be at the top of the HDI index list.
-
Structure of the Study
The rest of the paper is organized as follows: Section 2 represents recent studies in the literature that highlight important factors influencing HDI. Section 3 illustrates the step-by-step methodological use in this study. Section 4 presents the data used in this study as well as the application. Section 5 provides the discussion and findings of this study.
-
-
RELATIONSHIP ANALYSIS STUDIES FOR HDI IN THE
LITERATURE
The literature is heavy with previous studies that used relationship analysis to re-search factors affecting the human development index. First, while estimating HDI using health markers, Pelin & Tubas study [11] employed a tree-based regression model. After analyzing different tree-based regression models, the authors concluded that the gradient boosting technique provided the most accurate forecast of the impacts of the indicators of life on the HDI. From their research, the most critical contributors to HDI were the number of physicians and nurses and the current health spending per capita and midwives, with China, the Netherlands, Sweden, Turkey, Denmark, and Norway among the countries with the highest values.
Similarly, Asmita & Ruslans study [13] examined economic growth, poverty rates, public spending on education and healthcare, and income inequality to determine their effects on North Sumatra's human development index. The authors used a fixed effect model strategy, drawing information from 2009-2013's data culled from Central Statistics Indonesia and its Finance Ministry. They found that: the HDI was unaffected by income inequality and that economic progress had a favorable positive impact on HDI (as economic growth increases, HDI does as well); the percentage of poor residents had no impact on HDI; national expenditure on schooling did not affect HDI, and public expenditure on health care had a significant positive influence on HDI.
Imaningsih et al. in 2020 [14] analyzed variables impacting HDI in East Java. The research aimed to determine the effect of variables like the Gini and nonfood addiction expenditure ratio on life expectancy. The authors applied a multiple linear regression analysis about research ranking, using SPSS to examine the effect of the variables. They found that: The Gini ratio (showing the income distribution inequality) had no significant impact but exhibited a negative association with life expectancy (higher inequality in income distribution led to lower life expectancy in East Java); nonfood expenditure showed a positive association with life expectancy where high nonfood expenditure resulted in higher household income and percentage of food expenditures to all household expenses, and dependency ratio (indication burden residents bear at productive age) had no impact and negatively related to life expectancy (where higher dependency ratio resulted in lower life expectancy in the region).
Yanseni & Marhaenis paper [15] studied the impacts of investment, taxes, and balanced funds on HDI in the regencies. In Yanseni & Marhaenis paper, the study observation points were based on cross-section data from 21 regencies, one city, and 132 observation points. The data were collected within six years, and path analysis to predict the relationship between the variables. The authors found that: tax positively affected gross regional domestic product (GRDP), while investment and
balanced had no positive impact on GRDP; balanced funds, tax, and GRDP exhibited a favorable and positive effect on HDI, while acquisition did not affect HDI; and GRDP mediated the impact of taxation on HDI; however, it did not mediate the effect of balanced fund and investment on HDI. Similarly, a study by Lestari & Sanar [16] examined how various indicators impact HDI and established their relationship to provide a standard to enhance human resources quality. The authors used Pearson regression analysis to assess data extracted from the Central Bureau of Statistics in various publications. They established that life expectancy, income, and education indices positively affected human development. The correlation estimation indicated that each variable explained a variation of 14.788% of HDI.
Barus & Tarmizis paper [7] studied variables impacting the human development index in Binjai City with more emphasis on health, population, education, and income per capita. The authors conducted a causality analysis through multiple repression with data collected between 2005 and 2019. They concluded that the health shopping domain, education expenditures, population, and per capita income could impact Binjais local government. Using information from national data sources in the Yogyakarta region, Fadillah
& Setiartitis article [17] evaluated the determinants influencing HDI in the area. The authors analyzed the panel data technique and a fixed effect model. They found a positive and statistically significant relationship between the HDI, GDP per capita, and government spending on health. However, public investments in the education sector hurt HDI.
Yolandas research [18] looked into the effects of the Indonesian Bank (IB) rate, global bank rates, money supply, oil price, and gold price on inflation and HDI. She applied a multiple regression analysis to secondary data collected using a purposive sampling strategy. The author discovered that the national inflation rate is significantly influenced by the IB rate, global exchange rates, oil and gold prices, and money supply. Furthermore, inflation was found to have a favorable and beneficial impact on the HDI. Using a sample from different nations and a similar model, Yumashev et al. [19] assessed the influence of population energy utilization on the HDI. The study confirmed a correlation between energy use, HDI, and environmental effects (as measured by CO2 emissions). The authors determined that urbanization rate, GDP, GNI per capita, clean energy consumption proportion of total energy consumption by people and businesses, degree of socioeconomic development, and R&D expenditures predict the size and rating of the HDI. The findings demonstrate that a country's energy consumption determines its sustainable development level and human development index.
Humaira & Nugrahas paper [20] studied the HDI in west Kalimantan state using a regression model. In 2015, human development accomplishment was low at the national level and highly variable at the city level. Time series data from 2012 to 2015 was used for the regression analysis, where the authors established that life expectancy, per capita expenditure, schooling average, schooling expectation, and gross regional domestic product (GRDP) at constant prices affected the regions HDI. Using a similar regression method, Arisman [21] investigated the concept of HDI in ASEAN countries. The research aimed to determine the vaiables that impact ASEAN member states' HDI. Data analysis using a fixed effect model revealed that the HDI in ASEAN nations was affected by the
growth rate of population and per capita income but not by the variable inflation and unemployment rates. The study emphasized the vitality of government to control population expansion and foster economic expansion Research by Chen et al. [22] looked at the feasibility of mapping carbon per capita using HDI in Southwest China, and this was the first research to use decoupling analysis and index decomposition to examine the relationship between carbon emission per capita and HDI in Southwest China. The authors showed that the rise in carbon emissions per capita was promoted by economic production and suppressed by energy intensity. Looking at the province level, the authors found a correlation between their established emissions and historical highs in overall emissions. In their study, Arituan et al. [23] designed a study aimed at examining how municipal budgets in Indonesian provinces affected the proportion of residents who completed elementary school, the pro-portion who completed junior high school, the literacy rate of residents aged 15 to 55, the school participation rate of residents aged 7 to 12, the participation rate of residents aged 13 to 15, the proportion of residents who used family planning, the ratio of residents who were immunized, and the proportion of residents who assessed clean water and sanitation. Panel data regression was used to analyze Indonesian municipalities' trends between 2016 and 2019. They found that the number of persons in Indonesia who completed elementary school, the literacy rate for those aged 15 to 55, and the number of adolescents aged 13 to 15 who were enrolled in school were significantly affected by the amount of money the government spent on education. Users of family planning service in Indonesia's provinces, and municipalities were profoundly impacted by public spending on health. In contrast, the availability of safe water sanitation in Indonesian cities was affected by government expenditures on infrastructure.
Sudiarta & Utama [24] examined how cities in Bali's province fared financially and how it affected their economic growth and communal well-being. The research aimed to investigate the impact of municipal budgetary accomplishment on economic development and societys well-being in Bali Province between 2012 and 2017. The gathered information was subjected to a quantitative analysis utilizing the Structural Equation Model and Partial Least Squares. The authors found a positive relationship between financial accomplishment, economic advancement, and the well-being of the districts residents, with inclusive economic growth serving as a statistical mediator between the two. Finally, the impact of HDI factors on DIY economic growth was studied by Ari et al. [5]. The study aimed to examine how different aspects of the HDI have contributed to economic expansion in the Yogyakarta Special Region. Factors such as life expectancy, average schooling time, and GDP per capita were used to calculate the HDI. A quantitative study (common effect model) was utilized to determine the association. The authors observed that life expectancy did not affect economic growth; the average duration of education favorably predicted economic progress, while per capita expenditure negatively and favorably impacted economic development.
As seen throughout the research, HDI assesses every nations social and economic progress, focusing on various variables. The significant variables HDI centers on include; average schooling years, anticipated education years, life expectancy during birth, and GNI per capita. Literature consists of different researches that considered various fac-tors to study
their effects on HDI in multiple nations and cities. For instance, in their study, Tuba & Pelin [10], while analyzing the effect of education in HDI, used high school entrance examination, divorce rate, gross domestic product per city, rate of illiteracy, the population of higher education, internet access rates per household, book reading rate, and rate of a theatre child audience. The authors established that factors like divorce rate, GDP, internet access rate per household, book reading rate, and pro-portion of theatre child audience affected success rate and HDI.
Similarly, Runtunuwu [25] researched the impact of inflation and per capita income on HDI using multiple regression analysis. The author analyzed variables like per capi-ta income, inflation rate, and unemployment level as the independent factors and HDI as the dependent variable, where he found that all variables significantly affected HDI. Using the same model, research by Mutiha [26] analyzed how various funding sources, including sharing of tax revenue and general and special allocation, affected the HDI. Provincial governments' success in delivering public services was measured against the HDI. The research analyzed factors like tax revenue-sharing funds, general and special allocation funds, and regional-own source revenue.
On the other hand, Wahyuningrum1 & Soesilowati [27] employed ordinary least squares to investigate the factors influencing HDI in East Java Province. Factors analyzed included the rate of economic expansion, population, and the percentage of the unemployed actively seeking work. The authors found that the HDI is considerably affected by population size while only moderately affected by GDP growth and the open unemployment rate. Lastly, Purba [8] analyzed the HDI in the Highlands Region of North Sumatera Province, Indonesia, to investigate how monetary investments in health and education affected the HDI and the prevalence of poverty. Variables analyzed included the number of poor individuals, government, education, and health expenditures. He found that the number of poor residents and government and health expenditure favorably impacted HDI. The literature shows that various studies have used multiple factors to study a nations HDI. This study will select ten factors mentioned in the literature and determine how they affect HDI. Table 1 below summarizes the studies discussed above.
Table1. Recent studies on HDI in the literature
Article
Year
Prediction method used
Factors analyzed
Pelin & Tuba
2021
Tree-based regression model (gradient boosting technique
Asmita & Ruslan
2017
Fixed effect model strategy
Imaningsih, N., Priana, W., Sishadiyati, S., Asmara, K., & Wijaya,
R. S
2020
Multiple linear regression analysis
Yanseni & Marhaeni
2019
Multiple regression analysis
Lestari & Sanar
2018
Pearson regression analysis
Barus N. E., Tarmuzi HB., Rahmanta
2021
Causality analysis through multiple regression
Fadillah & Setiartiti
2021
Panel data technique and a fixed effect model
JERTV12IS030142
(
his work
www.ijert.org is licensed under a Creative Commons A
ttribution 4.0 International License.)
223344
-
Number of physicians
-
Number of nurses and midwives
-
Current health spending per capita
-
Income inequality
-
Economic growth
-
Percentage of poor residents
-
Government spending on education
-
Government spending on health
-
Gini ratio
-
Expenditure ratio of nonfood addiction
Dependency ratio
-
-
Life expectancy
-
Investment levels
-
Taxes
-
Balanced funds
-
Life expectancy
-
Income
-
Education indices
-
Health
-
Education expenditures
-
Population expenditures
-
Income per capita
-
GDP per capita
-
Government spending on health
-
Investment in education
-
Indonesian bank rate
-
Money supply
-
Foreign bank rates
-
Oil prices
-
Inflation
-
Gold prices
-
Population energy use
-
Environmental effect
-
Urbanization rate
-
GDP
-
GNI per capita
-
Degree of socioeconomic development
-
R&D expenditures
-
Clean energy consumption ratio of total energy consumption
-
Life expectancy per capita expenditure
-
Schooling average
-
schooling expectation
-
Gross regional domestic product
-
Carbon emission per capita
-
Economic production
-
Energy intensity
-
Population growth rate
-
Unemployment rate
-
Per capita income
-
Inflation rate
-
The proportion who completed elementary school
-
The proportion who completed junior school
-
Literacy rates of residents aged 15 to 55
-
The proportion of the population using family planning
-
The proportion of population immunized
-
The proportion who assessed clean water and sanitation
-
Financial performance
-
Inclusive economic growth
-
Life expectancy
-
Average schooling time
-
GDP per capita
-
High school entrance examination
-
Divorce rate
-
Gross domestic product per city
-
Rate of illiteracy
-
The population of higher education
-
Internet access rates per household
-
Book reading rate
-
Rate of a theatre child audience
-
Per capita income
-
Inflation rate
-
Unemployment level
-
Tax revenue-sharing funds
-
General and special allocation funds
-
Regional-own source revenue
-
Rate of economic expansion
-
Population rate
-
The percentage of the unemployed actively seeking work
-
Number of poor individuals
-
Government
-
Education
-
Health expenditures
-
Government expenditure on education
-
Total tax revenue
-
Unemployment
-
Foreign direct investment net inflows
-
Exports and imports
-
International inbound tourists
-
Hospital beds
-
Skilled labor force
-
Research and development expenditure
-
MATERIALS AND METHODS
The primary goal of this research is to predict the future HDI by finding the significant factors that contribute to increasing the HDI. Therefore, multiple linear regression will be utilized to find the association between the HDI as the dependent variable and various independent variables. Moreover, rank the relative importance of the significant factors that contribute to increased HDI.
-
Human Development Index Calculation
The HDI is consisting of three fundamental aspects of long- term development: (i) a long and healthy life, (ii) access to education, and (iii) a decent standard of living. The indicators for those dimensions are: life expectancy at birth, expected years of education, mean years of schooling and GNI per capita. They are calculated using mini-mum and maximum values in order to convert indicators expressed in different units into indices ranging from 0 to 1, as follows:
purchasing power parity (PPP) enables to highlight differences in purchasing power between countries by eliminating price level differences. The Income Index is logarithmically normalized in order to account for the diminishing contribution of higher incomes to human development [1]. considers two different information, related both to the average years of education and to the anticipated years of education. It is calculated as the arithmetic mean value of the related indexes, as follows:
= (5)
Where:
is the Average Years of Education index, defined as:
Dimension index =
(1)
= (6)
The three Dimension indices merged into an HDI by using
their geometric means. Therefore, the HDI is calculated as follows:
where MYS is the Average years of education, which is the average number of completed years of schooling, enrolled by
HDI = (2)
LEI is the normalized Life Expectancy Index
II is the normalized Income Index
is the normalized Education Index
the countrys population aged 25 years and older. is the Expected years of education Index, defined as:
= (7)
The LEI, II, and are defined as follows:
=
LEI
(3)
To determine the relationship between HDI and other important factors, multiple regression analysis wil be employed in this study. The regression analysis approach is
where LE is the life expectancy at birth [yr], and the
actual, max and min are the value of life expectancy at birth in the considered year, its maximum and minimum values adopted for the normalization by the United Nations, respectively. Moreover, the Gross National the normalized Income Index is calculated using equation
(4) as follow:
II = (4)
II analyzed using Gross National Income per capita (GNIpc) at purchasing power parities (PPP) [$pc PPP]. The GNIpc is defined as "the total of value added by all resident producers in the economy plus any product taxes (excluding subsidies) not included in the valuation of output plus net receipts of primary income (wages and rents) from outside, divided by midyear population. The value added is the industry's net output after totaling all outputs and deducting intermediate inputs. Considering
used to determine how a dependent variable may be predicted using an independent variable or variables, and it is defined as:
-
Multiple regression Calculation
(8)
Where:
Y is the dependent variable
are the independent variables on observation to i
are the regression coefficient
Error components that represent the deviations of the response from the true relation due to the effects of other factors on the response
The stander error for the estimate, defined as:
Where:
n is the number of observations
(9)
-
Correlation matrix Calculation
The Correlation among the independent variable to Answers: how strong is the linear relationship between the variables ,defined as:
K is the number of independent variables in the regression function
SSE is the residual sum of squares.
MSE is SSE/(n-k-1 ) the residual mean square.
(11)
Coefficient of determination R 2 ,defined as:
(10)
-
-
DATA COLLECTION AND APPLICATION
The data was collected from the United Nations Development Program Human Development Reports [12]. Statistical data from 96 countries were collected, including the 2019 Human Development Index (HDI) and other data obtained from the most recent year for which they are available for each country. The factors included in this study as well as the data for each included factor, are illustrated in Table 2 and Table 3, respectively.
Table 2. The independent variables employed in this study
Factors
Human Development Index (HDI): A composite index measuring average achievement in three basic dimensions of human developmenta long and healthy life, knowledge and a decent standard of living
government expenditure on education between 2013 – 2018 (% of GDP): Current, capital and transfer spending on education, expressed as a percentage of GDP.
Total tax revenue between 2014 – 2019 (% of GDP): Compulsory transfers to the central government for public purposes, expressed as a percentage of GDP.
The unemployment rate in 2019 (% of the labor force): Percentage of the labour force population ages 15 and older that is not in paid employment or self-employed but is available for work and has taken steps to seek paid employment or self-employment.
Foreign direct investment net inflows in 2019 (% of GDP): Sum of equity capital, reinvestment of earnings, other long-term capital and short-term capital, expressed as a percentage of GDP.
Exports and imports in 2019 (% of GDP): Sum of exports and imports of goods and services, expressed as a percentage of gross domestic product (GDP)
International inbound tourists in 2018 (in thousands) : Arrivals of nonresident visitors (overnight visitors, tourists, same-day visitors and excursionists) at national borders.
Hospital beds between 2010 2019 (per 10,000 people): Number of hospital beds available, expressed per 10,000 people.
Skilled labor force between 2010 – 2019 (% of the labor force) : Percentage of the labour force ages 15 and older with intermediate or advanced education, as classified by the International Standard Classification of Education.
Research and development expenditure between 2014 2019 (% of GDP): Current and capital expenditures (both public and private) on creative work undertaken systematically to increase knowledge, including knowledge of humanity, culture and society, and the use of knowledge for new applications. Research and development covers basic research, applied research and experimental development.
Table 3. The data for the HDI indexes and the independent variables employed in this study for 96 countries
Country
Y
Norway
0.957
7.9761
23.9
3.3
0.4
72.1
5,688
35.3
84.2811
2.06985
Ireland
0.955
3.71973
18.3
4.9
-20.4
239.2
10,926
29.7
85.047
1.14647
Switzerland
0.955
5.11332
10.1
4.6
-2.6
119.4
10,362
46.3
87.3141
3.37286
Iceland
0.949
7.45988
23.4
2.8
-1.8
85.9
2,344
28.3
76.1659
2.02994
Germany
0.947
4.80093
11.5
3.0
1.3
88.1
38,881
80
87.3212
3.09415
Sweden
0.945
7.66993
27.9
6.5
4.6
90.5
7,440
21.4
87.1154
3.33937
Australia
0.944
5.27163
23.0
5.3
2.8
45.7
9,246
38.4
78.94
1.87372
Netherlands
0.944
5.4796
23.0
3.2
3.1
154.3
18,780
31.7
78.5842
2.16374
Denmark
0.94
7.63485
32.4
4.9
-1.9
105.0
12,749
26
79.6615
3.06408
Finland
0.938
6.89735
20.8
6.6
3.1
79.5
3,224
36.1
90.5192
2.77381
Singapore
0.938
2.85435
13.1
4.1
28.3
319.1
14,673
24.9
84
1.94431
United Kingdom
0.932
5.48697
25.5
3.9
1.0
64.3
36,316
24.6
84.3688
1.72412
Belgium
0.931
6.54513
<>24.0 5.6
-5.4
163.3
9,119
55.8
85.6118
2.82119
New Zealand
0.931
6.44277
29.0
4.1
1.9
55.9
3,686
25.7
82.18
1.3657
Canada
0.929
5.262
12.9
5.6
2.6
65.0
21,134
25.2
91.9557
1.56625
United States
0.926
4.96174
9.6
3.7
1.5
26.4
79,746
28.7
96.4763
2.83766
Austria
0.922
5.5007
25.4
4.7
2.9
107.7
30,816
72.7
87.6045
3.17177
Israel
0.919
5.8493
23.1
3.9
4.6
58.5
4,121
29.8
90.3235
4.95278
Japan
0.919
3.18618
11.9
2.3
0.7
36.8
31,192
129.8
99.91
3.26451
Slovenia
0.917
4.80289
18.6
4.2
2.6
159.7
4,425
44.3
92.1087
1.94221
Korea
0.916
4.59437
15.6
4.1
0.6
76.7
15,347
124.3
85.9853
4.81009
Luxembourg
0.916
3.95519
26.5
5.4
-7.9
381.5
1,018
42.6
79.5526
1.23562
Spain
0.904
4.20911
14.2
14.0
1.3
66.9
82,773
29.7
67.6972
1.237
France
0.901
5.413
24.2
8.4
2.5
64.5
89,322
59.1
85.735
2.20002
Czechia
0.9
5.58738
14.9
1.9
3.8
144.9
10,611
66.2
95.3939
1.92829
Malta
0.895
5.20455
26.2
3.5
30.3
261.0
2,599
44.9
63.4538
0.57462
Estonia
0.892
5.17316
20.9
5.1
9.2
141.2
3,234
45.7
91.1513
1.42515
Italy
0.892
3.82926
24.3
9.9
1.5
60.1
61,567
31.4
69.9714
1.39909
UAE
0.89
3.103
0.1
2.3
2.5
160.9
21,286
13.8
52.4057
1.30316
Greece
0.888
3.667
26.2
17.2
2.2
74.4
30,123
42
81.3215
1.17732
Cyprus
0.887
6.29136
24.5
7.3
97.1
142.6
3,939
34
84.9979
0.55859
Lithuania
0.882
4.00847
16.9
6.4
2.5
150.6
2,825
64.3
96.4468
0.94496
Poland
0.88
4.63931
17.4
3.5
2.5
106.2
19,622
65.4
95.1002
1.21228
Latvia
0.866
4.71957
22.9
6.5
2.6
119.7
1,946
54.9
92.5467
0.63068
Portugal
0.864
4.88294
22.8
6.3
3.5
87.6
16,186
34.5
56.5709
1.36552
Slovakia
0.86
3.90267
18.7
5.6
2.2
185.2
2,256
57
95.6005
0.83252
Hungary
0.854
4.71078
23.0
3.4
3.8
163.0
17,552
70.1
88.824
1.55484
Saudi Arabia
0.854
5.138
8.9
5.9
0.6
62.2
15,334
22.4
58.66
0.81516
Country
Y
Chile
0.851
5.40274
18.2
7.1
2.0
56.8
5,723
20.6
71.2648
0.35518
Croatia
0.851
4.55695
22.0
6.9
1.9
102.8
16,645
55.4
91.7963
0.97489
Argentina
0.845
5.46223
10.1
9.8
1.4
32.4
6,942
49.9
66.9257
0.54152
Romania
0.828
2.97411
14.6
4.0
2.8
84.6
11,720
68.9
81.6709
0.5051
Kazakhstan
0.825
2.75095
11.7
4.6
2.0
64.4
8,789
60.6
80.8
0.12286
Russian
0.824
3.74303
11.4
4.6
1.9
49.1
24,551
71.2
96.0979
0.98988
Belarus
0.823
4.79499
14.7
4.6
2.0
133.3
11,502
108.3
98.63
0.60817
Uruguay
0.817
4.8403
20.1
8.7
1.3
41.0
3,469
24.3
26.0815
0.48393
Bulgaria
0.816
4.07685
20.2
4.3
2.2
123.7
9,273
74.5
87.894
0.76803
Georgia
0.812
3.84974
21.7
14.4
7.2
116.9
4,757
28.9
93.4484
0.30457
Costa Rica
0.81
7.02824
13.6
11.9
4.1
66.1
3,017
11
43.9963
0.42336
Malaysia
0.81
4.53477
12.0
3.3
2.4
123.1
25,832
18.8
66.8
1.43665
Serbia
0.806
3.71509
18.6
12.7
8.3
112.6
1,711
56.1
82.8695
0.92132
Mauritius
0.804
4.83459
19.1
6.7
2.6
92.8
1,399
34
61.6988
0.34597
Seychelles
0.796
4.41832
34.1
..
13.9
155.2
362
36
95.1513
0.22162
Albania
0.795
2.46866
18.6
12.3
7.9
76.7
5,340
28.9
79.5197
0.15412
Iran
0.783
3.95536
7.4
11.4
1.1
48.8
7,295
15.6
17.98
0.83027
Sri Lanka
0.782
2.11189
11.9
4.2
1.8
52.4
2,334
41.5
39.2365
0.10871
Mexico
0.779
4.90983
13.1
3.4
2.3
78.2
41,313
9.8
41.5812
0.31223
Ukraine
0.779
5.41226
20.1
8.9
2.0
90.2
14,104
74.6
79.9883
0.47134
Peru
0.777
3.71993
14.3
3.3
2.9
47.1
4,419
15.9
58.0614
0.12704
Armenia
0.776
2.70759
20.9
17.0
1.9
91.4
1,652
41.6
79.9449
0.18913
Colombia
0.767
4.50259
14.4
9.7
4.5
38.1
3,904
17.1
59.8693
0.23699
Brazil
0.765
6.24106
14.2
12.1
4.3
29.0
6,621
20.9
65.6896
1.26326
Azerbaijan
0.756
2.47382
13.0
5.5
3.1
86.0
2,633
48.2
93.3
0.18484
Moldova
0.75
5.51108
17.7
5.5
5.0
85.8
160
56.6
65.2196
0.25498
Tunisia
0.74
6.59921
21.1
16.0
2.5
110.5
8,299
21.8
55.7924
0.59997
Mongolia
0.737
4.08578
16.8
6.0
17.6
125.8
529
80
80.5507
0.10293
Paraguay
0.728
3.43834
10.0
4.8
1.0
69.2
1,181
8.3
43.6684
0.14883
Indonesia
0.718
3.5836
10.2
4.7
2.2
37.3
15,810
10.4
42.0142
0.22634
Philippines
0.718
3.234
14.0
2.2
2.0
68.6
7,168
9.9
29.87
0.16415
South Africa
0.709
6.15899
27.5
28.2
1.3
59.2
10,472
23
52.1689
0.83215
Egypt
0.707
3.931
12.5
10.8
3.0
48.3
11,196
14.3
57.196
0.72388
Kyrgyzstan
0.697
6.02619
18.0
6.3
1.7
103.1
423
44.1
92.68
0.10707
Morocco
0.686
5.261
21.9
9.0
1.3
87.5
12,289
10
18.68
0.71454
El Salvador
0.673
3.60075
18.1
4.1
2.7
77.2
1,677
12
41.0821
0.18115
Cabo Verde
0.665
5.17322
20.1
12.2
5.3
116.2
710
21
59.77
0.07264
Guatemala
0.663
2.92707
10.6
2.5
1.3
45.9
1,781
4.4
18.1558
0.028
Nicaragua
0.66
4.33953
15.7
6.8
2.7
95.1
1,256
9.3
30.5014
0.10742
Namibia
0.646
3.10315
29.3
20.3
-0.1
83.5
1,499
27
66.6696
0.33996
India
0.645
3.84467
11.2
5.4
1.8
40.0
17,423
5.3
21.2031
0.64998
Country
Y
Honduras
0.634
6.0812
17.3
5.4
3.8
96.6
851
6.4
28.1859
0.04
Ghana
0.611
3.98944
12.6
4.3
4.6
71.4
897
9
28.5341
0.37655
Nepal
0.602
5.16365
20.7
1.4
0.2
54.9
1,173
3
41.8908
0.3022
Kenya
0.601
5.32024
15.1
2.6
1.9
33.4
1,364
14
40.5334
0.78577
Cambodia
0.594
2.16286
17.1
0.7
13.7
123.6
6,201
9
14.3237
0.11823
Zambia
0.584
4.66738
16.2
11.4
2.1
70.0
1,072
20
39.1038
0.27819
Myanmar
0.583
1.9726
5.4
1.6
1.7
60.7
3,551
10.4
28.0812
0.03186
Uganda
0.544
2.52076
11.7
1.8
3.7
46.0
1,402
5
3.1526
0.17043
Tanzania
0.529
3.69644
11.5
2.0
1.8
32.2
1,378
7
4.9827
0.51456
Madagascar
0.528
3.23481
10.2
1.8
4.4
59.8
291
2
18.5092
0.01465
Togo
0.515
5.42885
16.9
2.0
-3.4
71.2
573
7
8.1846
0.26654
Senegal
0.512
4.65201
16.3
6.6
3.6
60.5
1,365
3
10.8479
0.5772
Gambia
0.496
2.43275
9.4
9.1
2.0
65.5
552
11
34.9911
0.0713
Ethiopia
0.485
4.73792
7.5
2.1
4.0
28.7
849
3.3
6.8709
0.27441
Mozambique
0.456
5.59847
21.6
3.2
18.2
112.2
2,743
7
7.0608
0.33751
Burkina Faso
0.452
6.03609
15.0
6.3
1.7
60.2
144
4
5.0302
0.70072
Mali
0.434
3.78684
11.6
7.2
2.7
57.2
14
1
5.7778
0.29175
-
Examining the Significant Factors on the HDI
Multiple linear regression analysis is one of the excellent methods for determining and assessing the association between two or more independent variables and one dependent variable [28]. Multiple regression can be defined as a regression where the independent variable predicts one dependent variable. Therefore, a multiple linear regression model is used to determine which factors affect the most on HDI. Statistical data from 96 different countries were included in the model. Table 4 shows the correlation matrix among the variables included in this study. Table 5 illustrates the outcome result of the regression analysis.
Table 4. Correlation matrix among the independent variables
Variable
Coefficient
SE
Coefficient
T-
Value
P-
Value
VIF
Constant
0.492700
0.032500
15.15
0.000
0.007030
0.007050
1.00
0.322
1.74
-0.000440
0.001590
-0.28
0.780
1.81
-0.001030
0.001800
-0.57
0.568
1.30
-0.000045
0.000658
-0.07
0.946
1.08
0.000293
0.000145
2.02
0.046
1.39
0.000001
0.000000
2.52
0.014
1.30
-0.000667
0.000406
-1.64
0.104
2.33
0.003542
0.000421
8.41
0.000
2.98
0.030000
0.009530
3.15
0.002
2.04
Table 5. Output results of multiple linear regression analysis
Y
0.296
0.331
0.474
-0.009
0.06
0.301
0.013
0.063
0.135
-0.004
0.348
-0.019
0.302
-0.102
0.182
0.393
0.019
-0.017
0.048
-0.093
-0.116
0.552
0.006
0.155
-0.047
-0.005
0.228
0.214
0.831
0.204
0.382
0.085
0.049
0.355
0.277
0.719
0.638
0.413
0.229
-0.162
-0.111
0.109
0.376
0.433
0.506
Model Summary
S
0.06887
73.91%
72.68%
71.28%
A Variance Inflation Factor (VIF) test was conducted to assess multicollinearity among the independent variables. A VIF near 1 suggests that multicollinearity is not a problem for that independent variable.. A stepwise procedure was also conducted to remove any insignificant variables from the model at alpha = 0.05. The final regression model is presented in Table 6. Moreover, Table 7, illustrates the Analysis of Variance (ANOVA) result of the regression model. Moreover, Residual plots for the regression mode are shown in Figure 2 and 3 .
Table 6. Final regression model
Variable
Coefficient
SE
Coefficient
T-Value
P-Value
VIF
Constant
0.517000
0.018100
28.60
0.000
0.000280
0.000134
2.080
0.040
1.21
0.000001
0.000000
2.460
0.016
1.24
0.003022
0.000305
9.910
0.000
1.58
0.034750
0.008040
4.320
0.000
1.46
Model Summary
S
0.0688018
77.47%
76.48%
75.06%
Source
DF
Adj SS
Adj MS
F-
Value
P-
Value
Regression
4
1.48148
0.370371
78.24
0.000
1
0.02057
0.020571
4.35
0.040
1
0.02855
0.028548
6.03
0.016
1
0.46494
0.464937
98.22
0.000
1
0.08848
0.088478
18.69
0.000
Error
91
0.43077
0.004734
Total
95
1.91225
TABLE 7. ANOVA OF THE REGRESSION MODEL
FIGURE 2. RESIDUAL PLOTS FOR THE REGRESSION MODEL
FIGURE 3. RESIDUAL PLOTS FOR THE REGRESSION MODEL
-
-
RESULTS AND DISCUSSION
From the result obtained. There is a strong correlation between the dependent variable Y (HDI) and the following independent variable of and . The or the coefficient of determination value is 0.7747. Therefore, 77.47% of the variation can be explained by the multiple regression model, and the identified equation can be used to make the prediction which may allow decision makers to create a strategic plan and predict the future HDI index and ranking countries. The regression model to predict HDI is as follows:
0.517+ 0.000280+ 0.000001+ (11)
-
The importance of the significant factors
Many previous studies focused on the relationship between human capital investment, production, and economic growth. This is evidenced by the long-term sustainable growth and development of countries, which is mostly driven by productivity growth [29]. According to the regression summarization of a previous study [30], the labor force acts as an external factor that relates its effect to economic growth with a strong R-value (97.5%) and an -value (95.1%) and this was confirmed in this study by the existence of an influencing relationship between the skilled labor force ( and the HDI.
Globally, Research & Development (R&D) spending has a large and favorable association with economic growth. The correlation between GDP growth and R&D spending across the long and short term, with the exception that the short-run coefficient appears to be negligible for emerging countries. Economies that invest more in R&D tend to expand at a faster rate [31]. Thus, it is not surprising to discover that the research and development expenditure () is a significant factor associated with HDI in this study.
In addition to societal and political relevance, modern tourism also brings substantial economic benefits. Tourism has grown to be one of the world's largest and fastest-growing sectors. Tourism expenditures have become a key source of economic activity, income, employment, and foreign money for many nations. Recognizing the increasing importance of tourism, governments, local authorities, and corporate sectors in numerous nations, regions, and communities have begun to invest in tourism development [32]. Developing tourism and transport are interconnected issues: tourists require safe, quick, and comfortable transport services that they pay for outside of their own budgets [33].
Exports and imports have a significant effect on the economic development of both developed and developing nations. Exports of goods and services are one of the most significant sources of foreign exchange profits, which help to alleviate the strain on the payments balance and create job opportunities, and strengthen local currencies [34]. Exports and imports both contribute to the influence of international trade on the economy. Exports are viewed as a contribution to national economy, but imports are viewed as an exit from the economy. A 1% increase in exports over the long run results
in a 0.38 % gain in the GDP, whereas a 1% growth in imports results in a 0.42 % increase in GDP. Exports have a greater long-term impact than the short-term impact [35].
-
Ranking the Relative Importance of the Significant Factors
Based on the findings of the study, four factors have proven to have a significant effects on increasing the HDI, namely: skilled labor force (), research and development expenditures (), and international inbound tourists (), and exports and imports (). The reason behind ranking the significant factors is to know on which elements the countries should focus the most, since these elements require a huge amount of resources. From the outcome result coming from regression model , has the highest T-value 9.910, followed by with 4.320, with 2.460, and with 2.080. Therefore, focusing on would contribute to increasing the HDI, among other significant factors. Moreover, was placed in the second position, followed by and .
Figure 4. Minitab Incremental impact of the significant factors
-
Linear programming to determine the amount of improvement required for the significant factors in a country to get to the First ranking on the HDI index
The objective of the model is to maximize a country's HDI index, indicated by the variable Y. To achieve the highest HDI index and the number one worldwide ranking. By increasing the significant factors by a specific amount determined by linear programming using the previous regression equation. constrained that every improvement at any factor cannot exceed not only the maximum amount of the highest country, but even 70% of the maximum amount of the highest country at each of the four significant factors, proving that a country can only improve this four factors or sectors by 70% in order to be at the top of the HDI index.
Model objective:
Maximize HDI(Y) = 0.517 + 0.000280 + 0.000001 +
0.003022+ 0.034750
O.B.; visualization, F.A., M.A., and O.B.; supervision, F.A.,
M.A., and O.B.; project administration, F.A., M.A., and O.B.; All authors have read and agreed to the published version of the manuscript.
Subject to:
<= 267.05, 70 % higher than other countries <= 62525.4, 70 % higher than other countries <= 69.937, 70 % higher than othercountries <= 3.466946, 70 % higher than other countries
Y <= 0.96, higher than the highest HDI index of 0.957
Result:
Y=0.96 = 267.05, = 36400.01, =69.937,
=3.466946
The skilled labor force should comprise 69.93% of the labor force, research and development expenditures should account for 3.46 % of GDP, exports and imports should account for 267% of GDP, and international inbound tourists should number 36,400 in thousands to be the first rank of the world.
-
-
CONCLUSIONS
I
Article |
Year |
Prediction method used |
Factors analyzed |
||
Yolanda |
2017 |
Multiple regression analysis |
|||
Yumashev, A., lusarczyk, B., Kondrashev, S., & Mikhaylov, A |
2020 |
Correlation analysis |
|||
Humaira & Nugraha |
2018 |
Linear regression analysis |
|||
Chen, L., Cai, W., & Ma, M |
2020 |
Decoupling analysis and index decomposition |
|||
Arisman |
2018 |
Fixed effect model |
|||
Arituan, B., Imansyah, M. H., & Sopiana, Y |
2021 |
Panel data regression analysis |
|||
Sudiarta & Utama |
2019 |
Structural Equation Model and Partial Least Squares |
|||
IJ |
ERTV12IS030142 (This work |
is license |
www.ijert.org d under a Creative Commons Attribution |
4.0 The welfare of district residents International License.) |
223355 |
Article |
Year |
Prediction method used |
Factors analyzed |
Ari, A. R., Wibowo, D., & Rahayu, K. D. |
2021 |
Quantitative analysis (common effect model) |
|
Tuba, K. O. C., & Pelin, A. K. I. N |
2022 |
Multiple regression analysis |
|
Runtunuwu, P. C. H |
2020 |
Multiple regression analysis |
|
Mutiha, A. H. |
2018 |
Multiple regression analysis |
|
Wahyuningrum¹, F., & Soesilowati, E. |
2021 |
Ordinary least squares |
|
Purba, B. |
2019 |
Regression analysis |
|
This study |
2022 |
Multiple Regression analysis |
The HDI is composed of three components: life expectancy, education, and standard of living. These components reflect the quality of life of individuals and the ability of countries to create a sustainable path towards development. The HDI has become a valuable tool for measuring and comparing the progress of countries. It is a comprehensive index that considers health, education, and income to better reflect a countrys overall well-being. Since its introduction in 1990, the HDI has undergone several revisions and remains the standard way of measuring human development.
In this study, nine factors were investigated to test their relationship with HDI. Using the multiple linear regression, four factors were significant out of nine factors. The relative importance of the four significant factors was ranked as follows, skilled labor force, R&D expenditure, tourism, and export & import moreover, a linear programming model showed that for a country to rank first on the HDI index, its four significant factors must be better compared to those of 70% of the world's other countries. This study gives a broader view to decision-makers about the most critical factors related to increasing the human development index, and in turns improve the quality of life.
Author Contributions: Conceptualization, F.A., M.A., and O.B.; methodology, F.A., M.A., and O.B.; software, F.A.,
M.A., and O.B.; validation, F.A., M.A., and O.B..; formal
analysis, F.A., M.A., and O.B.; investigation, F.A., M.A., and
O.B.; resources, F.A., M.A., and O.B.; data curation, F.A., M.A., and O.B.; writingoriginal draft preparation, F.A., M.A., and O.B.; writingreview and editing, F.A., M.A., and
Funding: This research received no external funding.
Informed Consent Statement: Not applicable.
Data Availability Statement: The data supporting the findings of this study are available within the article.
Conflicts of Interest: The authors declare no conflict of interest.
REFERENCES
[1] H. Omrani, A. Alizadeh, and M. Amini, A new approach based on BWM and MULTIMOORA methods for calculating semi-human development index: An application for provinces of Iran, Socioecon. Plann. Sci., vol. 70, p. 100689, 2020. [2] R. Prakash and P. Garg, Comparative assessment of HDI with Composite Development Index (CDI), Insights into Reg. Dev., vol. 1, no. 1, pp. 5876, 2019. [3] M. Amini, F. Zayeri, and M. Salehi, Trend analysis of cardiovascular disease mortality, incidence, and mortali-ty-to-incidence ratio: results from global burden of disease study 2017, BMC Public Health, vol. 21, no. 1, pp. 112, 2021. [4] P. Schröder, A. Lemille, and P. Desmond, Making the circular economy work for human development, Resour. Conserv. Recycl., vol. 156, p. 104686, 2020. [5] A. R. Ari, D. Wibowo, and K. D. Rahayu, The Influence of Human Development Index Components on Economic Growth in DIY, in The 3rd International Conference on Banking, Accounting, Management and Economics (ICOBAME 2020), 2021, pp. 213216. [6] N. Lind, A development of the human development index, Soc. Indic.Res., vol. 146, no. 3, pp. 409423, 2019.
[7] E. N. Barus and H. B. Tarmizi, Analysis of Factors Affecting Human Development Index in the City of Binjai, Int. J. Res. Rev., vol. 8, no. 4, pp. 161170, 2021, doi: https://doi.org/10.52403/ijrr.20210422. [8] B. Purba, Analysis of Human Development Index in the Highlands Region of North Sumatera Province Indonesia, in Multi-Disciplinary International Conference University of Asahan, 2019, no. 1. [9] S. Klasen, Human development indices and indicators: A critical evaluation, Hum. Dev. Rep. Off. Backgr. Pap., 2018. [10] K. O. C. Tuba and A. Pelin, Estimation of High School Entrance Examination Success Rates Using Machine Learning and Beta Regression Models, J. Intell. Syst. Theory Appl., vol. 5, no. 1, pp. 9 15, 2022. [11] A. Pelin and K. O. C. Tuba, Prediction of Human Development Index with Health Indicators Using Tree-Based Regression Models, Adyaman Univ. J. Sci., vol. 11, no. 2, pp. 410420, 2021. [12] United Nations Development Programme, Human Development Reports, United Nations Development Programme, 2022. [13] F. Asmita and D. Ruslan, Analysis of factors affecting the human development index in north sumatra province, IOSR J. Bus. Manag., vol. 19, no. 10, pp. 2736, 2017. [14] N. Imaningsih, W. Priana, S. Sishadiyati, K. Asmara, and R. S. Wijaya, Analysis of factors affecting human development index East Java, 2020. [15] Y. Yanseni and A. Marhaeni, The Analysis of Factors Affecting Human Development Index in The Regencies/City of East Nusa Tenggara Province, Indonesia, Int. J. Econ. Commer. Manag., vol. VII, no. 11, pp. 711722, 2019. [16] W. W. Lestari and V. E. Sanar, Analysis indicator of factors affecting human development index (Ipm), Geosfera Indones., vol. 2, no. 1, pp. 1118, 2018. [17] N. Fadillah and L. Setiartiti, Analysis of factors affecting human development index in special regional of Yogyakarta, J. Econ. Res. Soc. Sci., vol. 5, no. 1, pp. 88104, 2021. [18] Y. Yolanda, Analysis of factors affecting inflation and its impact on human development index and poverty in Indonesia, 2017. [19] A. Yumashev, B. lusarczyk, S. Kondrashev, and A. Mikhaylov, Global indicators of sustainable development: Evaluation of the influence of the human development index on consumption and quality of energy, Energies, vol. 13, no. 11, p. 2768, 2020. [20] U. H. Humaira and J. Nugraha, Analysis of factors affecting the human development Index in West Kalimantan Province using data panel data regression, EKSAKTA J. Sci. Data Anal., pp. 97105, 2018. [21] A. Arisman, Determinant of human development index in ASEAN countries, Signifikan, vol. 7, no. 1, pp. 113122, 2018. [22] L. Chen, W. Cai, and M. Ma, Decoupling or delusion? Mapping carbon emission per capita based on the human devel-opment index in Southwest China, Sci. Total Environ., vol. 741, p. 138722, 2020. [23] B. Arituan, M. H. Imansyah, and Y. Sopiana, The Effect of Education Expenditure, Health Expenditure, and Infrastructure Expenditure on Education Levels, Health Quality and Sanitation in Regencies/Cities in Indonesia, Budapest Int. Res. Critics Inst. Humanit. Soc. Sci., vol. 4, no. 4, pp. 1049110499, 2021. [24] D. M. Sudiarta and M. S. Utama, THE EFFECT OF REGIONAL FINANCIAL PERFORMANCE ON INCLUSIVE ECO-NOMIC GROWTH AND COMMUNITY WELFARE IN REGENCY/CITY OFBALI PROVINCE, South East Asia J. Contemp. Bus. Law, pp. 1560 2290, 2019.
[25] P. C. H. Runtunuwu, Analysis of Macroeconomic Indicators and Its Effect on Human Development Index (HDI), Society, vol. 8, no. 2, pp. 596610, 2020. [26] A. H. Mutiha, The Effect of Regional Own-source Revenue, Tax Revenue-sharing Fund, General Allocation Fund and Special AllocationFund to the Human Development Index (Based on the Study of Provincial Government in Indonesia), KnE Soc. Sci., pp. 609624, 2018.
[27] F. Wahyuningrum1 and E. Soesilowati, The Effect of Economic Growth, Population and Unemployment on HDI, 2021. [28] A. A. Muhartini, O. Sahroni, S. D. Rahmawati, T. Febrianti, and I. Mahuda, Analisis Peramalan Jumlah Penerimaan Mahasiswa Baru Dengan Menggunakan Metode Regresi Linear Sederhana, J. BayesianJ. Ilm. Stat. dan Ekon., vol. 1, no. 1, pp. 1723, 2021.
[29] H. Amir, M. Khan, and K. Bilal Impact of educated labor force on Economic growth of Pakistan: A human capital per-spective, Eur. Online J. Nat. Soc. Sci., vol. 4, no. 4, pp. 814831, 2015. [30] A. Wijaya, J. Kasuma, T. Tasene, and D. C. Darma, Labor force and economic growth based on demographic pressures, happiness, and human development, J. East. Eur. Cent. Asian Res., vol. 8, no. 1, pp. 4050, 2021. [31] A. Hafeez, K. B. S. Syed, and F. Qureshi, Exploring the Relationship between Government R & D Expenditures and Eco-nomic Growth in a Global Perspective: A PMG Estimation Approach, Int. Bus. Res., vol. 12, no. 4, pp. 163174, 2019. [32] L. Choong-Ki, T. Var, and T. W. Blaine, Determinants of inbound tourist expenditures, Ann. Tour. Res., vol. 23, no. 3, pp. 527542, 1996. [33] A.-M. Dinu, The importance of transportation to tourism development, Acad. J. Econ. Stud., vol. 4, no. 4, pp. 183187, 2018. [34] B. S. S. Hamdan, The effect of exports and imports on economic growth in the Arab countries: A panel data approach, J. Econ. Bibliogr., vol. 3, no. 1, pp. 100107, 2016. [35] A. Syzdykova et al., The Effect of export and imports on national income in Kazakhstan: Econometric analysis, Rev. Espac., vol. 40, no. 35,pp.114,2019.