
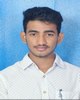
- Open Access
- Authors : Chava Girish Naidu , Tejasree Mankenapalli , Samudravijaya K, Yesu V V Nanda Kishore , Chebrolu Mohan Sai Teja, Suryakanth V G
- Paper ID : IJERTV12IS040149
- Volume & Issue : Volume 12, Issue 04 (April 2023)
- Published (First Online): 24-04-2023
- ISSN (Online) : 2278-0181
- Publisher Name : IJERT
- License:
This work is licensed under a Creative Commons Attribution 4.0 International License
Evaluation and Control of Speech Processing Quality in Indian Languages
Chava Girish Naidu
Department of Computer Science and Engineering, Koneru Lakshmaiah Education Foundation, Vaddeswaram,AP,India,
Yesu V V Nanda Kishore
Department of Computer Science and Engineering, Koneru Lakshmaiah Education Foundation, Vaddeswaram,AP,India,
Tejasree Mankenapalli
Department of Computer Science and Engineering, Koneru Lakshmaiah Education Foundation, Vaddeswaram,AP,India,
Chebrolu Mohan Sai Teja
Department of Computer Science and Engineering, Koneru Lakshmaiah Education Foundation, Vaddeswaram,AP,India,
Samudravijaya K
Department of Computer Science and Engineering, Koneru Lakshmaiah Education Foundation, Vaddeswaram,AP,India,
Suryakanth V G
Department of Computer Science and Engineering, Koneru Lakshmaiah Education Foundation, Vaddeswaram,AP,India,
Abstract:- Speech recognition is the process of paraphrasing of the spoken words into text. The process of recognizing speech requires recording and computing sound waves and converting them into words. The process will not be as easy as addressed. A lot more problems like noise data, fast speech, etc. will cause the model to loss its accuracy and quality in the assessment. So, we will use machine learning models like deep learning and deep neural networks, whose existence is much successful in recognizing and learning from the provided dataset. Not only recognizing, but there is also other thing that plays a key role in speech processing is the assessment of speech quality. So, there will be various methods through which the model will undergo to make a good quality speech recognition. In this research, we will be maximizing the accuracy and quality of the speech using some machine learning models.
-
INTRODUCTION:
From the origin, humans try to communicate with each other in a lot many ways like speech, text, hand gestures, etc. Among all of them communication through speech is thought to be the most significant act and also enhances the interaction. The speech will spread into air in the for, waves which are inorganic and without life. When two people communicate logically, both the sender and receiver have access to the same set of tools that enable them to understand each other's meanings. The researchers exploited this phenomena and expanded it into a crucial area of machine-human communication, where sound has aided in making the machine easier to use and the user-machine interaction more natural. The advancement in artificial intelligence, which aims to produce very flexible techniques in machine handling, has been considerably aided by speech recognition. This enables users to communicate and exchange data without the requirement for well-known input/output devices.
In this deep learning paradigm, Neural networks have considerably enhanced in speech recognition. Various approaches, including as convolutional neural networks (CNNs), recurrent neural networks (RNNs), and, most recently, Transformer networks, have been used.
-
RELATED WORK:
In [1] , for the research purpose, created a software for speech processing. The web application employs an authentic digital audio acquisition, modulation, and handling toolset and can visualize frequency waveforms, spectra, pitch, formants, and spectrograms. Every user can see the continuous pitch development of the waveform or for the selected frames or section of the audio by utilizing the "fundamental frequency" menu.
In [2], it states that they have utilized the feature extraction method. The suggested ZCPA model is made up of cochlear filter bank and a nonlinear layer at each bandpass filter's outcome. The non linear state includes of a zero- crossing vector, a peak detector and a comprehensive non linearity. Statistically, the dispersion of the level-crossing interval perturbation rises as the level value rises in the case of additive noise.
In [3], they have developed a special database, named multi-level speech database, which is quite useful for impulsive speech processing. They created the database to include text – based and audio versions ranging from descriptive to conversational speech. As they have mentioned, the speech outcomes came with a greater number of modification, repeats, and rests than the others. Despite the fact that several other criteria must be explored further, the features stated and used have reportedly helped in the speech processing.
I. Methodology
-
Gathering Dataset
the data set we utilize originates from a range of sources as shown in figure 1 and we believe that the best collection of data should be selected rather than the most comprehensive one after finishing the entire literature review we must now create a method to address every problem with the earlier strategies CNN, ASR and the techniques we used in this study both types of deep learning will result in accurate findings we used the speech recognition dataset available on Kaggle after receiving the results
-
Data Pre-processing
the superfluous data are deleted from the data collection process during this key stage providing the precise results we need to forecast successful outcomes categorization in our data gathering is still made easier due of this even though we do have the biggest files to get the biggest data pre-processing generally converts the data into a format that can be handled more quickly and efficiently in data mining machine learning and other data science operations one of the many anomalies that audio may have is noise which can be found with these scans audio filtering techniques can be used to eliminate these artefacts to lessen the noise a geometric mean filter is applied to the audio input
-
Understanding and Visualizing the data
after the work has been completed through data pre- processing the next task on the agenda is for the user to analyses the results of the data set we will show the data in a way that a person can only view it with their eyes because the large amount of data must be easily understood by everyone every everyone learns differently even though the brain processes 80 percent of information visually some people learn best by moving around while others learn best by listening however a sizable percentage of people more specifically 65 percent learn better through visual means quick understanding of the presented information is made possible by data visualization and online data visualization tools view figure 2 modern technology has transformed data into aesthetically beautiful easily understandable graphs since the development of spreadsheets
-
Classification
ASR involves text conversion from a specific aural utterance to train and evaluate an ASR system we need a text transcript of an audio speech since the acquired data already has this alignment by design no additional processing was needed to provide training or validation data for ASR customer care departments of global corporations are increasingly using ASR it is also used by various governmental bodies and other organizations simple ASR systems can understand single- word submissions like spoken numerals and yes-or-no inquiries an asr system might not always be able to correctly identify input from people who speak with a strong accent or dialect when someone blends terms from two different languages out of habit it has serious problems as well
E. Finding Total Parameters
the summary techniques results are displayed since each row represents a layer we can simply refer to these layers by their row names without creating any ambiguity in the figure below each layer that was added to the model in the previous code sample can be seen weights that have been trained serve as the parameters frequently they are the weight matrices that are changed as part of the back-propagation process to improve the predictive power of the model they alter their values depending on the training technique you use particularly the optimization approach
II. ACTED EMOTIONAL DYNAMIC DATABASE A database of emotional speech recordings known as the Acted Emotional Speech Dynamic Database (AESDD) was developed especially for research. The database contains recordings of performers speaking while expressing various emotions, including joy, sadness, rage, and terror. The actors' vocal and facial expressions were intended to be captured in the recordings. The AESDD was established to promote study in a number of areas, such as human-computer interaction, emotion recognition, and speech recognition. The database can be used by researchers to investigate the ways in which emotions are expressed in speech and to create algorithms for identifying emotional states in speech signals.
Recordings in several languages, including English, Spanish, Chinese, and Portuguese, can be found in the AESDD. Actors of all sexes, ages, and races provided the recordings for collection. The repository
-
ARABIC SPEECH CORPUS
The Arabic Speech Corpus is a collection of recordings in the spoken Arabic language that has been assembled and edited for research. Recordings of Arabic speech from a range of sources, including news broadcasts, radio shows, and conversational speech, can be found in the corpus.The corpus contains recordings of Arabic being spoken in a variety of regional and Modern Standard Arabic (MSA) dialects. The recordings, which span a variety of themes and genres, were gathered from speakers of various ages, genders, and backgrounds.For academics researching speech recognition, natural language processing, and related topics, the Arabic Speech Corpus is an invaluable tool. It can be used to design and test voice recognition software, research the grammatical and acoustic characteristics of spoken Arabic.
-
VISUALIZATION OF SPEECH DATA.
In the field of speech processing, visualisation of speech data is a crucial tool for practitioners and academics. Techniques for speech data visualisation are used to show and examine vast volumes of linguistic and acoustic data present in voice signals.The use of spectrograms, which show the acoustic energy of speech with time and frequency, is a typical method for visualising speech data. A spectrogram is a three- dimensional diagram that illustrates how a signal's frequency content varies over time. The intensity of the colour or shading represents the amplitude or energy of the signal at each point in time and frequency, while the x-axis and y-axis stand for time and frequency, respectively.Another interesting visualisation tool for speech data is the waveform display, which illustrates the variations in air pressure over time that are created by speech. Waveform displays make it possible to see the fundamental frequency of speech as well as other features of pitch and timing.Researchers and practitioners may also utilise scatter plots, heat maps, and network graphs in addition to spectrograms and waveform displays to visualise speech data. These visualisations can assist in highlighting correlations and patterns in speech data that may be challenging to identify using other techniques.
-
WAVEFORM
A waveform is the representation of a voice signal as a graph of air pressure changes over time when it comes to speech processing. Analog signals can be caught and stored as a result of the pressure waves that are produced as sound moves through the atmosphere. The amplitude of the signal at each instant in time can then be represented numerically as a waveform, which can be used to digitally transform and store the analogue signal.A graph with time on the x-axis and amplitude on the y-axis can represent a waveform. The pattern of air pressure variations over time that make up the voice signal are represented by the waveform's shape. The waveform can reveal details about the speech's fundamental frequency, or pitch, as well as other acoustic characteristics like timing and intensity.In speech processing research, waveform analysis is a key tool that is utilised in a number of applications, such as speech recognition, speaker identification, and emotion recognition. Researchers can create algorithms to automatically extract elements from voice signals that are important for various applications by examining the waveform of the signals.Moreover, waveform visualisation can be applied to activities like forensic speech analysis and voice quality evaluation. Overall, the waveform serves as a crucial illustration of speech signals and offers insightful data for speech processing and analysis.
-
WAVE TRANSFORM,
A mathematical method called the wavelet transform is used in signal processing, especially voice processing, to examine and represent signals at various scales. It is a potent time- frequency analysis method that can assist in illuminating the underlying structure of complicated signals like speech.A signal is divided into a number of wavelets, which are mathematical functions that are localised in both time and frequency, in a wavelet transform. The signal is then examined using these wavelets at various scales or resolutionshigh resolution for high-frequency components and low resolution for low-frequency components. This enables the examination of the signal's small features as well as its overall structure.Wavelet transforms can be applied to speech processing in a variety of ways, including feature extraction,
compression, and denoising. While denoising, high-frequency components that are irrelevant to the signal are removed using the wavelet transform to separate the signal from the noise. Wavelet transform can be used in compression to shrink the size of the signal while keeping the most crucial components. The wavelet transform can be used to extract important information from the signal, such as pitch and formants.Wavelet transforms can take many different forms, such as continuous wavelet transform (CWT) and discrete wavelet transform (DWT). The wavelet transform comes in two different iterations: CWT, which is continuous, and DWT, which is discrete and uses a limited number of wavelets. Due to its computational effectiveness and capacity to handle signals in the time-frequency domain, DWT is increasingly frequently employed in practise.Wavelet transform, in general, is a strong and adaptable method for evaluating speech signals and has a variety of uses in speech processing.
-
SPEED ENHANCEMENT
When speech signals are damaged by noise or other factors, speech enhancement is a technique used to restore the quality and understandability of the signals. In order to minimise or remove undesirable noise while maintaining the voice content, the speech signal must be processed.For speech augmentation, a variety of techniques are used, including time-domain and frequency-domain approaches. Frequency-domain approaches entail converting the speech signal into the frequency domain for processing, whereas time-domain techniques directly manipulate the speech signal's waveform.Spectral subtraction is a popular time-domain technique for improving speech quality. It entails calculating the noise power spectrum and subtracting it from the noisy speech spectrum to produce an improved speech spectrum. Another time-domain method is Wiener filtering, which estimates the speech signal and filters out noise using a statistical model of the speech and noise signals.Filter-bank techniques, which separate the signal into sub-bands and apply filtering to each sub-band, and spectral enhancement techniques, which alter the speech signal's
spectral envelop to improve the content of the speech, are examples of frequency-domain approaches for speech enhancement.Speech enhancement is a crucial method for enhancing the effectiveness of speech processing systems in noisy settings, including speaker identification and speech recognition. It is also employed in different applications such as hearing aids, telecommunication systems, and audio restoration.It is difficult to precisely estimate the noise characteristics and separate it from the voice signal without introducing distortion or artefacts, making speech enhancement a difficult undertaking. In order to attain higher performance and dependability, researchers are always creating new and improved approaches for voice augmentation.
-
CONCLUSIONS
-
We have experimented with deep learning in this. Speech processing is the study of voice signals and signal processing methods. supervised and unsupervised procedures are the two main categories. ASR is used to translate a specific auditory speech into text. Simple ASR systems can understand single- word submissions like spoken numerals and yes-or-no inquiries. Convolutional Neural Network (CNN) technique uses a variety of emotion detection modules and classifiers to differentiate between emotions based on spoken emotion identification.
We draw this conclusion from the trials conducted thus far since training and testing errors decline as the number of training models rises..
REFERENCES
[1] P. Lakkhanawannakun, Speech Recognition using Deep Learning, June2019 [2] Raghib, E.Sharma, T.Ahmad, F.Alam, Emotion Analysis and Speech Signal Processing, June 2018 [3] A.H. Poorjam, Quality Control in Remote Speech Data Collection, Jan 18, 2019 [4] Philipos C. Loizou Speech Quality Assessment, Vol 346 [5] S. Benkerzaz, Y. Elmir, A. Dennai, A Study on Automatic Speech Recognition, Aug2019. [6] Y. Hu, Evaluation of Objective Quality Measures for Speech Enhancement, Feb 2008. [7] N. Dimmita P. Siddaiah, Speech Recognition Using Convolutional Neural Network, Sep 2019 [8] https://www.kaggle.com/datasets/uwrfkaggler/ravdess- emotional-speech-audio [9] M.S. Hossain and G. Muhammad, Emotion recognition using deep learning approach from audiovisual emotional big data, Inf. Fusion, vol. 49, pp. 6978, Sep. 2019. [10] M. Chen, P. Zhou, and G. Fortino, Emotion communication system, IEEE Access, vol. 5, pp. 326337, 2016. [11] S. Lalitha, A. Madhavan, B. Bhushan, and S. Saketh, Speech emotion recognition, in Proc. Int. Conf. Adv. Electron. Comput. Commun. (ICAECC), Oct. 2014, pp. 14. [12] K. R. Scherer, What are emotions? And how can they be measured? Social Sci. Inf., vol. 44, no. 4, pp. 695729, 2005. [13] S. G. Koolagudi and K. S. Rao, Emotion recognition from speech: A review, Int. J. speech Technol., vol. 15, no. 2, pp. 99117, 2012. [14] J. Schmidhuber, Deep learning in neural networks: An overview, Neural Netw., vol. 61, pp. 85117, Jan. 2015. [15] S. Demircan and H. Kahramanl, Feature extraction from speech data for emotion recognition, J. Adv. Comput. Netw., vol. 2, no. 1, pp. 2830, 2014.