
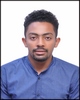
- Open Access
- Authors : Sofonyase Bekele , Brook Abate
- Paper ID : IJERTV9IS120252
- Volume & Issue : Volume 09, Issue 12 (December 2020)
- Published (First Online): 30-01-2021
- ISSN (Online) : 2278-0181
- Publisher Name : IJERT
- License:
This work is licensed under a Creative Commons Attribution 4.0 International License
Estimation of Sediment Yield using Swat Model: A Case of Soke River Watershed, Ethiopia
Sofonyase Bekele1,a*
1Masters Student
at Department of Civil Engineering, College of Architecture and Civil Engineering,
Addis Ababa Science and Technology University, Addis Ababa, Ethiopia
Brook Abate2,b
2Associate Professor
at College of Architecture and Civil Engineering, Addis Ababa Science and Technology University, Addis Ababa, Ethiopia
Abstract:- Soil erosion is a process of detachment/dispatch/ of soil particles due to raindrop and runoff. This results in the deposition of sediment causing reduced flood-carrying capacity affects water qualify instability of structure and natural streambeds and banks. This study is about the estimation of sediment yield and reduction of sediment yield with appropriate mitigation measures. The input data for the model were obtained from different organizations; After the input data are organized and assembled it was used for model simulation. Later simulation result was calibrated and validated using Calibration Uncertainty Procedure (SWAT- CUP). The efficiency evaluation criterias Coefficient of determination (R2), and the Nash-Sutcliffe simulation efficiency (ENS) were used for performance evaluation of the model. The result from the calibration and validation of both flow and sediment were greater than 0.7; this indicated that there was very good agreement between observed and simulated outputs. The model prediction result shown that the annual sediment yield produced from the watershed to be 8.80 tons/ha/year. Also, the most sediment yielding and erosion- prone subbasins were identified as well as ranked from first to fifth as subbasin 6, 9, 8, 5, and 3 respectively. Finally, for proper management of the watershed seven scenarios were developed and the best management practice identified was scenario three (30% of agricultural land changed to forest mixed land) with 26.13% sediment reduction. Moreover, applying filter strips and terracing in low slope areas reduces 24.28% and 18.83% respectively could be implemented as an operational management strategy.
Key Words: SWAT model, Stream Flow, Sediment Yield, Soke river, Calibration, Validation, SWAT-CUP
-
INTRODUCTION
Erosion is the geological process in which earthen materials are worn away and transported by natural forces such as wind or water. It causes soil loss and generating sediment yield from catchment areas even in the absence of human alterations of land cover. Soil erosion by water occurs in a two-phase process, consisting of the detachment of individual particles from the soil mass and their transport by erosive agents, and when sufficient energy no longer available to transport deposition occurs [1]. Soil erosion not
only the deposition of sediment transported by river from upstream to downstream but also sediment deposition on riverbeds and banks causes widening of the flood plain during floods. Sediment can consist of rocks and minerals, as well as the remains of plants and animals. It can be as small as a grain of sand or as large as a boulder. It is still one of the problems for the river ecosystem around the world. A study was done on the world's 145 major rivers with consistently long-term sediment records and the results show that about 50% of the rivers have statistically a significantly downward flow trend due to sedimentation [2]. Land degradation due to soil erosion is a major problem facing Ethiopia today. In most of the catchments, soil erosion is caused by intense rainfall, steep topography, construction, and poor vegetation cover coupled with the cultivation of steep lands, and inadequate conservation practices. Hence, the more pressure exerted on land the more it leads the soil to lose its structure and cohesion, which means it can be eroded more easily.
Soke River is located in the southern part of Ethiopia in the rural catchment with agricultural activities and estimation of sediment yield and implementation of appropriate reduction measure for sediment yield under different scenarios in the catchment is significant to minimize the extent of soil erosion. Therefore, the Soil and Water Assessment Tool (SWAT) model integrated with GIS techniques was used to simulate the sediment yield of the study area.
-
MATERIALS AND METHODS
-
Description of the Study Area
Soke River is located in the Southern part of Ethiopia in Kembata Tembaro Zone, Hadero Tunito Woreda. It is bordered on the south by the Wolayita Zone, on the west by Tembaro, on the north by the Hadiya Zone, and on the east by Kacha Bira. It is geographically located at Latitude between 70100 – 70200 and Longitude between 370350
– 370500. It starts from Mugunja Kebele flowing across Ajora falling water to Omo-Gibe River. It is one of the tributaries of the Omo-Gibe River Basin.
-
Description of the SWAT model
Figure 2.1 Map of the study area
of information about the watershed. In the SWAT model, the
Soil and Water Assessment Tool (SWAT) is a physically- based continuous-event hydrologic model developed to predict the impact of land management practices on water, sediment, and agricultural chemical yields in large complex watersheds with varying soils, land use, and management conditions over long periods of time [3] & [4]. The SWAT (Soil and Water Assessment Tool) [4] was developed by the
U.S. Department of Agriculture, Agricultural Research Service (USDA-ARS) to overcome all limitations of the previous model. The Arc SWAT extension of Arc GIS is a graphical user interface for the SWAT model [3]. To create a SWAT dataset, the interface will need to access Arc GIS compatible raster (GRIDs) and vector datasets (shapefiles or feature classes), and database files that provide certain types
water-balance is the backbone of the hydrologic simulation in a watershed; and the hydrology of a watershed can be separated into two major divisions, land phase and routing phase [4].
-
SWAT Model Input and Data Collection
The data for this study mainly relay on secondary data, which will be obtained from a different organization. The materials used for the model input were the digital elevation model (DEM), land use/ land cover map, and meteorological data. The organizations were Ministry of Water, Irrigation, and Energy (MoWIE) for hydrological data, Food and Agriculture Organization (FAO) for Soil map, National Meteorological Agency (NMA) for weather data, United States Geological Survey (USGS) for land use/cover map, and Alaska satellite facility for DEM.
Table 2.1 List of material and data collection
Data
Data Type
Organization
Resolution
DEM
Spatial
ALASKA
12.5m
Land use/Landcover
Spatial
USGS
30m
Soil
Spatial
FAO
30m
Hydrological
Time series
MoWIE
11 year
Meteorological
Time series
NMA
30 year
Table 2.2 Land use and land cover of the study area
No
Land use and Landcover
Area in ha
Coverage (%)
1
Agriculture
10893.76
58.37
2
<>Forest 5427.57
29.08
3
Pasture
2178.62
11.67
4
Settlement
164.02
0.88
Total
18664
100
Tab
le 2.3 Soil distribution of t
he study area
No
Soil Type
Hydrologic Soil Group
Area in ha
Coverage (%)
1
Chromic Luvisols
B
7154
38.33
2
Eutric Vertisol
D
701
3.76
3
Humic Nitisoils
B
10891
57.91
Total
18664
100
Table 2.4 List of selected meteorological gaging stations for this study
No.
Station Name
Latitude
Longitude
Elevation
1
Wolaita
6.81
37.73
1854
2
Hossana
7.5673
37.85383
2307
3
Boditischool
7.310583
38.09392
1772
4
Angacha
7.3405
37.8572
2317
-
Model setup
-
Watershed Delineation
The first step in creating Arc SWAT model input is the delineation of the watershed from a DEM. Inputs entered into the Arc SWAT model were organized to have spatial characteristics. Before going in hand with spatial input data
i.e. the soil map, Land use/land cover map, and the DEM
were projected into the same projection as UTM Zone 37N, which is a projection parameter for Ethiopia. A watershed was delineated into several sub-basins, for modeling purposes. The watershed delineation process includes five major steps, DEM setup, stream definition, outlet and inlet definition, watershed outlets selection and definition, and calculation of sub-basin parameters.
Figure 2.2 Delineated Watershed of the study area
-
Hydrological Response Units (HRU)
Hydrologic response units (HRUs) are defined as those lumped land areas within the sub-basin that are made of unique land cover, soil, and management combinations. HRUs enables the model to reflect differences in evapotranspiration and other hydrologic conditions for different land covers and soils. The land area in the sub- basin was divided into HRUs. The HRU analysis tool in Arc SWAT loads of land use, soil layers, and slope delineation
to the project. The delineated watershed by Arc SWAT and the prepared land use /land cover, the soil was overlaid. HRU analysis in Arc SWAT includes divisions of HRUs by slope classes in addition to land use and soils. The multiple slope option (an option that considers different slope classes for HRU definition) was selected. The land use/land cover, soil, and slope map were reclassified to correspond with the parameters in the Arc SWAT database. After reclassifying the land use, soil, and slope in the Arc SWAT database, all
these physical properties were overlaid. Another step in HRU analysis is HRU definition. The HRU distribution in
this study was determined by assigning multiple HRU to each sub-watershed.
Figure 2.3 Soil map of the study area Figure 2.4 Land use land cover map of Soke Watershed
-
Weather Data Definition and Weather Generator After HRUs are defined, the following step is importing the climate data. This is computed in the Write input table tab in SWAT next to the HRU analysis tab. In this section, the weather data definition in SWAT contains tabs for precipitation, air temperature, solar radiation, wind speed, and relative humidity. These data were fed correspondingly. On top of these data statistical analysis of monthly daily average, standard deviations, and the probability of wet and dry days, skewness coefficients and dew temperature were determined by FORTRAN program known as pcpSTAT.exe and program dew02.exe for ease of computation currently there exists a SWAT weather database program for calculating all statistical weather parameters used by the WGEN and it also has options for converting SWAT weather inputs into text format.
-
-
Simulation
After all the input data are gathered and organized as per the model input, simulation takes place. Simulation of the model
-
Scenario Management
Scenarios are a reasonable and often simplified depiction of possible future event through a coherent and internally
was done after delineation of the watershed, HRU definition, and Weather data inputs. To run simulation first period of the simulation was defined (starting and ending date), setting warm-up period and printout setting (daily, monthly, or yearly); then setup SWAT run and run SWAT. For this specific study, the starting and ending period was from 1989 to 2018 with a warm-up period of three years.
The outcome from the model prediction must not be adopted for other analysis. Rather, the capability of the model in efficiently predicting the streamflow and sediment yield was assessed with sensitivity analysis, model calibration, and model validation. Daily streamflow values and sediment concentration for Soke River were obtained from the Hydrology Department of the MoWIE, Ethiopia. These daily streamflow and sediment concentrations at Soke River were used for model calibration and validation.
consistent assumption about key driving forces and relationships.
Table 2.5 List of developed scenarios
Scenarios
Description
Conditions
Scenario 0
Baseline
Existing
Scenario 1
Agricultural land to forest mixed land
10%
Scenario 2
Agricultural land to forest mixed land
20%
Scenario 3
Agricultural land to forest mixed land
30%
Scenario 4
Agricultural land to forest mixed land
40%
Scenario 5
Agricultural land to forest mixed land
50%
Scenario 6
Filter strip
<25%
Scenario 7
Terrace
<25%
-
Sensitivity Analysis, Calibration, and Validation
-
Sensitivity Analysis
Sensitivity analysis was conducted to minimize the influence of over-parameterization. For this study parameters sensitive to flow and sediment were analyzed before calibration.
-
Model Calibration and Validation
For this specific study, the model calibration and validation process for both flow and sediment was conducted by using the SUFI-2 (Sequential Uncertainty Fitting Version 2 program) in SWAT_CUP since it's a widely used calibration tool and [5] found that SUFI-2 needed the smallest number
of model runs to achieve a similarly good calibration and
( )2
= 1 =0 . (2.2)
prediction uncertainty results in comparison with the four
other techniques.
=0
( )2
Calibration and validation were carried out for both streamflow and sediment modeling on daily basis. A total of 11 years (1992-2002) of historical data was used; out of which, six years 1992-1998) was for calibration and the remaining five years (1998-2002) for validation.
-
-
Model Efficiency Evaluation
The efficiency of SWAT is tested through statistical tools for determination of quality and dependability of simulations for comparing the simulated value to observed values. The performance of the model in simulating streamflow and sediment yield were evaluated using SWAT-CUP. The goodness of fit measures used to evaluate model prediction during calibration and validation period were Coefficient of determination (R2) and Nash-Sutcliffe simulation efficiency (ENS).
The Coefficient of determination (R2): – is an indicator of the strength of the relationship between the observed and simulated values. It is computed by the equation:
[(, ) (, )]2Where, is the observed (measured) streamflow on day i (m3/s), and is the simulated streamflow on day i (m3/s).
-
-
RESULT AND DISCUSSION
3.1 Sensitivity Analysis
Sensitivity analysis for both flow and sediment was carried out prior to calibration of the model to save time during calibration. Distinguishing sensitive parameters let us to aim only on those parameters which affect most the model output during calibration since the SWAT model has a number of parameters to deal with. The model was calibrated and validated on a daily basis to check its applicability in modeling streamflow and sediment yield from the Soke river watershed. A time-series dataset of 1990 to 2002 was used. The beginning three years of the simulation period was used for model warm-up. Data from the period 1992 to 1997 were used for calibration and the rest for validation of the model.
Table 3.1 Duration used in the warm-up, calibration, and
2
2
2 =
(, )
(, )
2 . (2.1)
validation
Time Frame |
No of year |
||
Description |
From |
To |
|
Model Warm-up |
1/1/1989 |
1/31/1991 |
3 |
Calibration |
1/1/1992 |
1/31/1997 |
6 |
Validation |
1/1/1998 |
1/31/2002 |
5 |
SWAT simulation |
1/1/1990 |
12/31/2018 |
30 |
Time Frame |
No of year |
||
Description |
From |
To |
|
Model Warm-up |
1/1/1989 |
1/31/1991 |
3 |
Calibration |
1/1/1992 |
1/31/1997 |
6 |
Validation |
1/1/1998 |
1/31/2002 |
5 |
SWAT simulation |
1/1/1990 |
12/31/2018 |
30 |
Where, , is the observed (measured) stream flow on day
,
,
i (m3/s), is the simulated streamflow on day i (m3/s),
is the mean observed (measured) stream flow (m3/s), and
is the mean simulated stream flow (m3/s).
The Nash-Sutcliffe simulation efficiency (ENS): – indicates how well the plot of observed versus simulated value fits the 1:1 line. The value of ENS ranges between negative infinity and 1 (best) therefore, (-, 1]. If the
measured value is the same as all predictions, ENS is 1. If the ENS is between 0 and 1, it indicates deviations between measured and predicted values. If ENS is negative, predictions are very poor, and the average value of output is a better estimate than the model prediction [6]. This coefficient is calculated by equation
-
Parameters sensitive to flow
The list of parameters adopted for the sensitivity analysis are stated in table 3.2. Daily flow data was used from 1992 to 1997 for calibration of the model.
Table 3.2 List of parameters used for sensitivity analysis
Parameter
Range Value
t-value
p-value
Rank
ALPHA_BF
0
1
4.1425
0.0002
1
SOL_K
0
92
-3.8953
0.0005
2
CN
-0.2
0.2
-2.62
0.014
3
HRU_SLP
0
1
-2.2960
0.0293
4
OV_N
10
23.4
2.2297
0.0339
5
EPCO
0
1
-1.6471
0.1107
6
BIO MIX
0
1
-1.4764
0.1509
7
CH_K2
-0.01
500
-1.4403
0.1608
8
SOL_AWC
-0.25
0.25
1.3936
0.1744
9
GW_DELAY
30
450
-1.3158
0.1988
10
SLSUBBSN
10
150
1.2026
0.2391
11
RCHRG_DP
0
1
0.909
0.3706
12
GWQMN
0
2
-0.7987
0.4311
13
CH_N2
-0.01
0.3
0.6922
0.4944
14
SOL_ALB
-0.25
0.25
0.5781
0.5677
15
ALPHA_BNK
0
1
-0.4882
0.6291
16
CANMX
0
100
0.3859
0.7024
17
ESCO
0
1
0.3680
0.7156
18
SURLAG
0.05
24
-0.3216
0.7501
19
REVAPMN
0
500
0.16
0.8739
20
TLAPS
-10
10
-0.0737
0.9417
21
The result from the global sensitivity analysis indicated, Base-flow alpha-factor (ALPHA_BF), Saturated hydraulic conductivity (SOL_K), and the curve number (CN2) to be the most sensitive parameter followed by the rest as presented in table 3.2.
-
Parameters sensitive to sediment
Sensitivity analysis was done for sediment and the list of parameters adopted are described in table 3.3
Table 3.3 List of parameters used for sensitivity analysis
Parameter |
Range Value |
t-value |
p-value |
Rank |
||
OV_N |
10 |
23.4 |
17.37 |
0.0 |
1 |
|
LAT_SED |
0 |
5000 |
17.20 |
0.0 |
2 |
|
SLSUBBSN |
10 |
150 |
8.01 |
0.0 |
3 |
|
SPEXP |
1 |
1.5 |
-2.90 |
0.017 |
4 |
|
CH_BED_D50 |
1 |
10000 |
-1.885 |
0.092 |
5 |
|
USLE_P |
0 |
1 |
1.56 |
0.151 |
6 |
|
CH_CHOV2 |
-0.001 |
1 |
-1.162 |
0.274 |
7 |
|
SPCON |
0.0001 |
0.01 |
1.037 |
0.326 |
8 |
|
CH_COV1 |
-0.05 |
0.6 |
-0.978 |
0.353 |
9 |
|
LAT_TIME |
0 |
180 |
-0.36 |
0.72 |
10 |
The parameters listed above were selected for the sensitivity analysis of sediment calibration. Daily sediment data set similar to flow sensitivity analysis was used for identifying sensitive parameters. The result from the global sensitivity analysis indicated that Manning n value for overland flow (OV_N), Sediment concentration in lateral flow and groundwater flow (LAT_SED), and the Average slope length (SLSUBBSN) were the keen parameter as ranked in table 3.3. These were determined through t-stat and p-value as described in the table.
3.2 Model calibration and validation 3.2.1Model calibration and validation for flow
3.2.1.1 Model calibration
The overall performance of the model for calibration has been evaluated with the Coefficient of determination (R2) and the Nash Sutcliff Efficiency (NS); It resulted in 0.82 and
0.80 values respectively. Figure 3.1 below shows the result of measured and simulated values of SWAT_CUP calibration.
observed simulated
35
Flow(m3/s)
Flow(m3/s)
30
25
20
15
10
5
01-01-1992
01-07-1992
01-01-1993
01-07-1993
01-01-1994
01-07-1994
01-01-1995
01-07-1995
01-01-1996
01-07-1996
01-01-1997
01-07-1997
01-01-1992
01-07-1992
01-01-1993
01-07-1993
01-01-1994
01-07-1994
01-01-1995
01-07-1995
01-01-1996
01-07-1996
01-01-1997
01-07-1997
0
Date
Figure 3.1 Calibration of observed and simulated flow hydrograph
The Graph above in figure 3.1 is the plot of the measured and predicted flow overlay of the daily time step. The graph indicates a slight overestimation with a low flow period in the year 1997; similarly, it also shows some under- prediction in the peak flow period of the year 1997. In general, the daily observed and simulated calibration indicates a very good agreement between the measure and simulated flow.
Figure 3.2 Plot of 1:1 slope of observed and simulated flow calibration through a web-based hydrograph analysis tool
3.2.1.2 Model validation
Model validation is mandatory to increase the level of confidence of the user through the confirmation of the best- fitted calibration parameters. It was conducted for the year (1998-2002). This was computed through the same parameters which were used and adjusted during the calibration process. The parameters are used without further adjustment. As shown in the model's performance statistics (Figure 3.4); R2, and NSE were 0.75, and 0.74 respectively.
observed simulated
30
Flow(m3/S)
Flow(m3/S)
25
20
15
10
5
01-01-1998
01-07-1998
01-01-1999
01-07-1999
01-01-2000
01-07-2000
01-01-2001
01-07-2001
01-01-2002
01-07-2002
01-01-1998
01-07-1998
01-01-1999
01-07-1999
01-01-2000
01-07-2000
01-01-2001
01-07-2001
01-01-2002
01-07-2002
0
Date
Figure 3.3 Validation of observed and simulated daily flow hydrograph
The observed and simulated flow in the daily time step of the validation period indicates that the model slightly overestimated at low flow time periods, and underestimated at peak flow time period; slight overestimation in the years 1999, and 2001 as well as slight underestimation in the years 2000, and 2001. This is due to the missing data from weather data which were estimated and filled through different missing data filling mechanisms. Other reasons are mistakes made during the record of weather and flow data resulted in some variation between observed and predicted flows of low and peak periods.
Figure 3.4 Plot of 1:1 slope of observed and simulated flow validation through a web-based hydrograph analysis tool
-
Model calibration and validation for sediment
-
Model calibration
SWAT model was calibrated for daily sediment yield after calibration and validation of streamflow at the outlet of Soke river watershed. The measured sediment data was generated from the prepared sediment rating curve. The measured and simulated sediment were compared for the calibration period (1992-1997) in SWAT_CUP. The SWAT model
performance was evaluated through Coefficient of determination (R2) and Nash Sutcliff Efficiency (NSE) with a result of R2=0.79, and NS=0.78.
observed simulated
96
96
35000
Sediment load(ton/day)
Sediment load(ton/day)
30000
25000
20000
15000
10000
5000
01-01-1992
01-09-1992
01-05-1993
01-01-1994
01-09-1994
01-05-1995
01-01-19
01-09-1996
01-05-1997
01-01-1992
01-09-1992
01-05-1993
01-01-1994
01-09-1994
01-05-1995
01-01-19
01-09-1996
01-05-1997
0
-5000
Date
Figure 3.5 Calibration of observed and simulated daily sediment hydrograph
Figure 3.6 Plot of 1:1 slope of observed and simulated sediment calibration through a web-based hydrograph analysis tool
-
Model validation
-
Sediment validation of the SWAT model was computed after sediment calibration without further adjustment fitted calibration parameters. The model validation was done outside the calibration period (1998-2002). Its performance was evaluated with the coefficient of determination (R2) and Nash-Sutcliff Efficiency (NS) with values of R2 =0.74, and NS=0.74.
observed simulated
30000
Sediment load(ton/day)
Sediment load(ton/day)
25000
20000
15000
10000
5000
01-01-1998
01-07-1998
01-01-1999
01-07-1999
01-01-2000
01-07-2000
01-01-2001
01-07-2001
01-01-2002
01-07-2002
01-01-1998
01-07-1998
01-01-1999
01-07-1999
01-01-2000
01-07-2000
01-01-2001
01-07-2001
01-01-2002
01-07-2002
0
-5000
Date
Figure 3.7 Validation of observed and simulated daily sediment hydrograph
The above graph indicates that the model slightly overestimated at low time periods, and underestimated at
peak time period; slight overestimation in the years 1999, and 2001 as well as slight underestimation in the years 2000, and 2001. This is due to the missing data from weather data which were estimated and filled through different missing data filling mechanisms.
Figure 3.8 Plot of 1:1 slope of observed and simulated sediment validation through a web-based hydrograph analysis tool
The total annual sediment yield estimated by the SWAT model from the river at the outlet during the calibration and validation period was 164,243.2 tons/year. Hence, 164,243.2 tons/year of sediment is generated by the river. The total area of the watershed is 186.64 km2 and the specific sediment yield from the watershed is 8.80 ton/ha/year.
3.4 Spatial distribution of sediment yield in soke watershed
Identification of hotspot sub-basins that are prone to erosion in the catchment allows the watershed management practice as functional to the properly-identified sub-basins to lower the sediment yield of the area. Spatial visualization is one of the abilities of SWAT. This allows to visualize which areas produce high or low sediment. After calibration and validation, the model was simulated for a period of 30 years (1989-2018). According to the result from the model; hotspot areas have been identified in the watershed. The corresponding spatial visualization of sub-basin wide sediment yield in tons/ha is shown in figure 3.9 below. The SWAT model simulated annual average sediment yield was
8.80 t/ha/year.
Table 3.4 Subbasin areas and sediment yield [7]
Class |
Sediment yield (t/ha/year) |
Category of soil erosion region |
1 |
0.001-7 |
Low |
2 |
7-18 |
Moderate |
3 |
18-46.86 |
Sever |
Figure 3.9 Spatial visualization of sediment output from SWAT
As represented on the above map subbasins 3, 5, 6, 8, and 9 were identified as the highest sediment yield producing and more erosion-prone subbasins. These subbasins are categorized under agricultural land with the soil property of Chromic Luvisol.
-
Scenario management for Mitigation Measure
Based on the output generated from the model among the land use/cover of the area agricultural land covers the major portion of the watershed and it is the main source of sediment of an area, and forest mixed, pasture, and urban covers a small area and contribute little sediment to Soke Watershed. The advantage of the SWAT model is its ability to build different scenarios.
From the model output HRU report, 58.37 % of the Soke watershed is covered with agricultural land, which makes it to be susceptibly impacted by soil erosion and the source of sedimentation. Therefore, to minimize soil erosion and sediment deposition in the river watershed; scenario development is crucial for future prediction and plan. The solutions provided were changing agricultural land use to forest mixed land, and the two other operational best Management Practices (OBMP): applying filter strip and terracing were also adopted. These scenarios were developed to assess the change in sediment yield from the watershed. Applying filter strips and terracing (stone bunds) in low slope areas of the catchment could give potential effect of BMPs [8].
Table 3.5 Summary of scenario development result
Scenario
Annual Total Sediment (ton)
Change in Sediment (ton)
Change in percentage
S0
164243.2
0
0
S1
142779.6
21463.6
-13.07%
S2
137219.84
27023.36
-16.45%
S3
121316
42927.2
-26.13%
S4
113169.08
51074.12
-31.09%
S5
99852.4
64390.8
-39.2%
S6
124355.48
39887.72
-24.28%
S7
133304.6
30938.6
-18.83%
0
-0.05
Scenario Development
S0 S1 S2 S3 S4 S5 S6 S7
-0.1
-0.15
-0.2
-0.25
-0.3
-0.35
-0.4
-0.45
Figure 3.10 Comparison of the sediment load change
The result of the SWAT model estimation for the Soke river watershed was 164243.2 tons/year. Developing watershed management scenarios provide better results in terms of applying the best management practice for the watershed. The chosen critical subbasin level showed spatial variability on sediment reduction from baseline scenarios as indicated previously in table 3.5 The favored critical subbasin resulted in a sediment reduction ranging from 13.07% to 39.2% in general; Out of which agricultural land changed to forest mixed land indicated a sediment yield reduction of 13.07% to 39.2%, construction of filter strip with 24.28%, and construction of terrace with 18.83%. Thus, from the prepared scenarios as described above the corresponding results could be ranked as agricultural land to forest mixed land, construction of filter strip, and construction of terrace respectively. In spite of the fact that 50% of agricultural land to forest mixed land resulted in a 39.2% reduction to sediment, it is difficult due to the socio-economic activity of the area. Therefore, implementing Scenario five as the best management strategy is not acceptable because it highly reduces the functional agricultural land. To reduce sediment yield and balance the functionality of agricultural land, scenario three (30% of agricultural land changed to forest mixed land) is preferable best management practice with 26.13% sediment reduction. Moreover, the construction of filter strips and terracing in low-slope areas could be implemented as an operational management strategy.
5.1 CONCLUSION
The hydrology modeling software; Soil and Water Assessment Tool (SWAT) was used to estimate the sediment yield of the Soke river watershed; It successfully accomplished both the modeling of streamflow and sediment. Automatic calibration and validation of the SWAT model were done using SWAT_CUP with SUFI_2 algorithm. This helps to minimize model uncertainty and increase the level of user confidence. This was done after
identifying important parameters through sensitivity analysis of both streamflow and sediment. Thereafter the model was calibrated for seven years (1992-1997) and validated for five years (1998-2002) for both streamflow and sediment respectively at the outlet of Soke river. The model performance evaluation from daily streamflow calibration and validation period at the outlet indicated that R2 =0.82, NS=0.80 and R2 =0.75, NS=0.74 respectively. Similarly, the model performance evaluation from daily sediment calibration and validation period indicated that R2 =0.79, NS=0.78, and R2 =0.74, NS=0.74 respectively. This shows that SWAT has the ability to simulate both runoff and sediment for the Soke river watershed. The total annual sediment yield of Soke river estimated using the SWAT model during calibration and validation is 164,243.2 ton/year. Hotspot areas have been identified for proper application of management practice on the identified critical subbasins. Those were subbasins 3, 5, 6, 8, and 9 producing the highest amount of sediment in the watershed. After the erosion-prone subbasins were identified, different management scenarios have been developed for sediment reduction measures. The best management practice provided for sediment reduction is 30% of agricultural land changed to forest mixed land which is 26.13% reducing sediment yield.
ACKNOWLEDGMENT
Above all, I would like to thank the almighty God for his graciousness and unlimited kindness in all endeavors that made the work successful. And I would like to acknowledge Ethiopian Road Authority for the scholarship opportunity.
REFERENCE
-
Morgan, R. (2005). Soil Erosion and Conservation, Blackwell Publishing, Oxford.
-
Walling, D. and Fang, D. (2003): Recent trends in the suspended sediment loads of the worlds rivers, Global Planet. Change, 39, 111126.
-
Arnold, J.G., R. Srinivasan, R.S. Muttiah, and J.R. Williams. (1998). Large area hydrologic modeling and assessment part I: model development. J. American Water Resources Association, 34(1),73-89.
-
Neitsch, S. L., Arnold, J. G., Kiniry, J. R., and Williams, J. R. (2011). Soil and water assessment tool theoretical documentation version 2009. Texas Water Resources Institute.
-
Yang J., Peter R., Abbaspour K.C, Junxia & Hang Y. (2008). Comparing uncertainty analysis techniques for swat application to the chaohe basin in china. Institute of Geographical Sciences and natural resources research, bejing china.
-
Nash, I. and Sutcliffe, J. (1970). River flow forecasting through conceptual models part1 a discussion of principles Journal of Hydrology, 10(3), 282-290.
-
Hurni H. (1983). Soil Formation Rates in Ethiopia Highlands Reclamation Study.
-
Betrie, G. D., Mohamed, Y. A., Griensven, A. V., & Srinivasan, R. (2011). Sediment management modeling in the Blue Nile Basin using SWAT model. Hydrology and Earth System Sciences, 15(3), 807-818.
-