
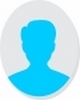
- Open Access
- Authors : Hiwot Hailu Tehsome , Agizew Nigussie Engida , Geremew Sahilu Gebrie
- Paper ID : IJERTV10IS110174
- Volume & Issue : Volume 10, Issue 11 (November 2021)
- Published (First Online): 13-12-2021
- ISSN (Online) : 2278-0181
- Publisher Name : IJERT
- License:
This work is licensed under a Creative Commons Attribution 4.0 International License
Effect of Watershed Delineation on SWAT Model Performance for Daily Streamflow Simulation, in Blue Nile, East Africa
Hiwot Hailu Tehsome1
1A PhD candidate at the School of Civil and Environmental Engineering, Addis Ababa Institute of Technology, Addis Ababa, Ethiopia
Agizew Nigussie Engida2 , Geremew Sahilu Gebrie3 2,3Associate Professor at the School of Civil and Environmental Engineering, Addis Ababa Institute of Technology, Addis Ababa, Ethiopia
Abstract:-This study was conducted in the Blue Nile Basin that has a catchment area of 307,189 Km2. The major objective of the study was to investigate effect of watershed delineation on SWAT performance for daily streamflow simulation. Two model setups were developed, which are called scenario1 and scenario2, with 25 and 145 numbers of subbasins, respectively. The sequential uncertainty fitting (SUFI-2) algorithm in the SWAT calibration and uncertainty programs (SWAT-CUP) was used for sensitivity analysis, calibration and validation processes. The sensitivity rank and type of SWAT flow parameters were different for the two scenarios. Calibration and validation were done at multi- gauge stations (at Kessie, El-Diem and Khartoum). Values of the Nash Sutcliffe Efficiency (NSE) and Coefficient of determination (R2) were found to be in between 0.5 and 0.9 after calibration and validation of each scenario. For scenario2, the performance of SWAT in terms of NSE improved by 1% at Kessie, 12% at El- Diem and 7% at Khartoum station relative to scenario1. For the given HRU thresholds (10-20-10 for land use-soil type-slope), the major land uses distribution deviated from the original (i.e., 0% threshold) distribution by some percent. This was due to the regroup of minor land uses into the major land uses. For scenario2, croplands increased by 2.5-4% for catchment areas draining into Kessie, El-Diem and Khartoum gauge station. The increment of the percentage of croplands increased the Curve Number (CN), which is the main parameter that increases the surface runoff. Overall, it is concluded that increasing the number of subbasins has effect on streamflow simulation using SWAT in the Blue Nile Basin. Therefore, for a better SWAT result it is necessary to delineate a basin optimally.
Keyword:–Streamflow simulation, SWAT model, SUFI-2 algorithm, Sensitivity Analysis, Calibration, Validation
-
INTRODUCTION
Streamflow modeling is essential in water resources management, for example, for dam design, hydropower management, flood forecasting, assessment of climate and land use change impacts, and water quality assessment [1]. Watershed models simulate the hydrology in two phases: land phase and in-stream phase. Land phase simulation controls loadings of water and parameters into the main stream channel of each subbasin and in-stream phase simulation routes the hydrological variables into a watershed outlet [2]. Water balance is the main driving force for all processes that found in watershed modeling.
Watershed models such as Hydrological Simulation Program-FORTRAN (HSPF), Annualized Agricultural Non- point Source Pollution (AnnAGNPS), soil and water
assessment tool (SWAT), and Source are used for simulating hydrologic processes and water quality parameters (pollutant loadings) in a continuous time step. The models can also be used for assessing the interaction between land use and total suspended solids and/or nutrients in large watershed systems. The AnnAGNPS and HSPF models require intensive input datasets and may not be suitable for data scarce large transboundary river basins [3]. Of the above models, SWAT is the most widely used model for different scale watersheds to assess the impact of land use/cover change and management on the quantity and quality of water.
Watershed delineation is a primary process for streamflow simulation using SWAT model. Watershed delineation defines the extent of landscape areas that contribute surface runoff to a particular river outlet [4] based on a digital elevation model (DEM) [5]. Some researchers have examined the effect of watershed delineation on streamflow simulation using SWAT [6][12]. They used different watershed delineation techniques: minimum drainage area (MDA), stream order (from 2nd to 5th order), 8-digit and 12-digit hydrologic unit code. Minimum drainage area (MDA) method of delineation is found in SWAT model. Review of studies conducted on large scale river basins are presented below.
Ref. [10] investigated the impact of watershed delineation on streamflow prediction in four different size watersheds located inside Iowa. One of the watersheds has a larger size (Area = 17,941km2). Different subwatersheds generated using different minimum threshold drainage areas and 0% HRU thresholds set for land use and soil type. They compared SWAT simulated streamflow for the coarsest and finest subwatersheds and it fluctuated by 4 percent on average. They concluded that SWATs streamflow is insensitive to changes in the number of subwatersheds. This is because the surface runoff has a direct relation with the Curve Number (CN), which is not affected by the size of subwatersheds.
Ref. [11] delineated the Kaskaskia River watershed (Area
= 14,152 km2) into five levels of subwatersheds using 2nd to 5th stream orders and MDA of 3000ha. The number of subwatersheds for the SWAT delineation was 304 and for the 5th stream order delineation was 19. For the other stream orders the number of subwatersheds were in between 19 and 304.Three levels of HRU thresholds ranging from 5% to 15% were used for land use and soil type. They used parameter values calibrated previously for a monthly streamflow at four subwatershed outlets. There was a very little difference in SWAT model performance for subwatersheds delineated by the 2nd and 3rd stream orders and MDA method.
Ref. [12] delineated the Upper Mississippi River Basin (UMRB) into subbasins using 8-digit and 12-digit Hydrologic Unit Codes (HUCs) in Hydrologic and Water Quality System (HAWQS) platform. The 8-digit and 12-digit HUC are equivalent to coarser and finer numbers of subbasins. Next, the subbasins are apportioned into HRUs using two HRU thresholds (70%/70% and 1km2/1km2) for land use and soil type. The 1km2 and 70% HRU threshold are equivalent to multiple HRUs and dominant HRUs. In total five delineation scenarios were developed from the combination of HUC, HRU thresholds and climate data densities. The total area of 8- digit subbasins and 12-digit subbasins are not equal. This difference in total drainage area was caused by the input hydrology data that obtained from the source. SWAT was simulated at multi-gauge sites using previously calibrated set of parameter values and monthly streamflow for all scenarios. The values of NSE and R2 are better, for both HUC8 and HUC12 scenarios, at the St. Paul station than the remaining stations. From the study one can understand that the need for calibrating for each watershed delineation to get better SWAT model performance.
The effect of watershed delineation on SWAT model performance has not been well addressed in previous studies. This may be due to the dependency of watershed delineation effects on the characteristics of a watershed, sensitivity analysis and calibration of parameters for individual delineation levels. None of the reviewed studies performed sensitivity analysis and calibration of SWAT setups for different delineations. As far as we know, the effect of watershed delineation on streamflow in Blue Nile basin and sensitivity of SWAT flow parameters to watershed delineations are not studied. Large river basins are heterogeneous because of the differences intopography, climatic variables, soil, geology, and land use/cover. Effects of scaling are related to heterogeneity [13].
The major objective of this study was to investigate the effect of watershed delineation on SWAT model performance for daily streamflow simulation. Identification of the sensitivity of SWAT flow parameters for the number of subbasins and calibration and validation of the model at multi- gaging stations have also been addressed.
-
MATERIALS AND METHODS
-
Description of the study area
Blue Nile River Basin covers an area of 307,189 Km2 (Fig. 1). The river starts from Lake Tana, in Ethiopia. It joins the White Nile River at Khartoum after traveling a 1,769Km long distance. The geographic extent of the basin is at longitude 32o3705E to 39o3705E and latitude 7o2100N to 16o1200N. The terrain elevation varies between 350m and 4,265m above sea level. The mean annual rainfall is between 1200 and 1800 mm/year for basin areas upstream of Ethiopia- Sudan border [14] and <800 mm/year for the areas downstream of the border. Some of the major tributaries that flowing into Blue Nile River are Beshilo, Giamma, Guder, Birr, Diddessa, Dabus, and Beles. Blue Nile River is the major tributary of the Nile River which contributes over 85% of the Nile flows. The basin has potentials for ecotourism, livestock production, large-scale irrigation, and hydroelectric power generation. Since 2012, the Ethiopian government has been
constructing a huge dam for hydro-electric power production. When the dam becomes operational, it boosts the Ethiopian economy and benefits downstream and neighboring countries indirectly by attenuating extreme floods and reducing sediment and pollutant loads.
-
Input data types
-
Digital elevation model (DEM)
12.5m resolution digital elevation model (DEM) was downloaded from (https://vertex.daac.asf.alalska.edu). For this study area, the size of the DEM was more than 4.0GB, which is not allowed to use for terrain processing in Geographic Information System software (QGIS and ArcMap). Hence, the 12.5m DEM resampled into a 60m pixel size using the bilinear resampling technique in the ArcMap 10.2 and used for river network and catchment delineation purposes.
-
Land use/cover and soil type data
Land use/cover raster data (20m) was downloaded from (https://datacatalog.worldbank.org/dataset/cci-land-cover-s2- prototype-land-cover-20m-map-africa-2016) and it was then resampled into a 60m pixel using a bilinear resampling technique (Fig. 2B). The land use naming is different from SWAT naming system therefore; each land use class was matched with SWAT code as shown in Table 1. The land use/cover classes were linked with the SWAT land use classes through a lookup table.
Additionally, Harmonized World Soil Data (HWSD), 500m resolution, was downloaded from (http://www.fao.org/soils-portal/soil-survey/soil-maps-and- databases/harmonized-world-soil-database-v12/en/). The Harmonized World Soil Database (HWSD_version1.2.1) lacks information for some soil parameters such as albedo, soil erodibility and saturated hydraulic conductivity. Therefore, the missing soil parameters were computed from a hydrological point of view for topsoil and subsoil layers by adopting equations from [15] (Table 2) and taking the required input data from the HWSD. Then a user soil database was prepared for SWAT use. Fig. 2C shows soil type map of the study area.
-
Hydro-meteorological datasets and data gaps filling
Daily meteorological (rainfall, minimum and maximum air temperature) and streamflow data were collected from Ethiopia (National Meteorological Agency and Basin Development Authority) and Sudan (Meteorology Agency and Ministry of Irrigation and Water Resources). The datasets were collected for the period 2002-2014. Hydro- meteorological data gaps (< 20% per a station) were filled using HYFO the R-package [16] and linear regression method. The filled daily rainfall and temperature data and their geographic coordinates were prepared in a text file format before imported into the model setup. The collected streamflow data has missing records at Kessie and El-Diem stations particularly for the last three months of 2014. These data gaps were filled with a regression method.
-
-
SWAT model
Soil and Water Assessment Tool (SWAT) is a semi- distributed, time continuous, and process based model. It was developed by the United States Department of Agriculture (USDA) for predicting the impact of land use change and managements on water quantity and non-point source pollution in watersheds and large river basins [2], [13].
Fig. 1. Location of the study area
The SWAT model was developed, through a long period, by including the fundamental components that contributed from the USDA Agricultural Research Service (ARS) field scale models, Groundwater Loading Effects of Agricultural Management Systems (GLEAMS) model, Chemicals, Runoff and Erosion from Agricultural Management Systems (CREAMS) model, Environmental Policy Integrated Climate (EPIC) model, QUAL2E model, CFARM model, Green-Ampt infiltration method and other routines [2]. SWAT is a process based and computationally efficient. The major SWAT model components are the following hydrology, weather, crop growth, sediment, nutrients, pesticides, and agricultural management. Description for some of the components as given in [13] is as follows:
Value
CCI Land uses
SWAT code
1
trees cover areas
FRST
2
shrubs cover areas
RNGB
3
grassland
PAST
4
cropland
AGRR
5
vegetation aquatic or regularly flooded
WETN
6
lichen mosses/sparse vegetation
RNGE
7
bare areas
SWRN
8
built-up areas
URMD
10
open water
WATR
Value
CCI Land uses
SWAT code
1
trees cover areas
FRST
2
shrubs cover areas
RNGB
3
grassland
PAST
4
cropland
AGRR
5
vegetation aquatic or regularly flooded
WETN
6
lichen mosses/sparse vegetation
RNGE
7
bare areas
SWRN
8
built-up areas
URMD
10
open water
WATR
Table 1.Swat code assigned for CCI land use classes
Hydrology model depends on the water balance equation (1), which is the key force behind a watershed hydrology process. The total runoff leaving the basins is obtained by routing the amount of runoff that predicted for each subbasin.
(1)
Where, SWt and SW0 are the final and initial soil water content on day i, t is the time (day), and R, Q, ET, P, and QR are the daily precipitation, surface runoff, Evapotranspiration, percolation and return flow in millimeter (mm).
Table 2.SWAT Equations adopted for determining soil parameters [15]
No.
Soil parameter
Equation
Author
1
Albedo
0.1807+0.1019*exp (-3.53*33)
[17] 2
Hydraulic conductivity (Ks)
6.96-10^(-0.884+0153*S)
[18] 3
Soil erodibility (KUSLE)
Es*EC-T*EOC*EHS
[19] Where, S = percentage of sand, T = percentage of silt,
OC = percentage of organic carbon, C = percentage of clay, and 33= soil water content at field capacity.
-
(B)
Fig. 2. Spatial input data type (A) digital elevation model, (B) land use/cover and (C) soil type
Surface Runoff volume is predicted by the Soil Conservation Service (SCS) Curve Number (CN) method (2) using rainfall.
, R > 0.2s (2)
, R < 0.2s
Where Qsurf is the daily surface runoff (mm), R is the daily rainfall (mm), and s is the retention parameter which is given by (3), and CN is the curve number. The retention parameter varies with the type of soil, land use and management, slope and soil water content in watersheds.
(3)
The peak runoff rate is used for estimating sediment loss and calculated by a modified rational method. In order to allow a realistic peak runoff simulation, a stochastic element is included in the rational method.
Evapotranspiration (ET) – is based on the availability of weather variables, potential ET can be estimated using the Hargreaves, Priestley-Taylor, or Penman-Monteith method. More details on the SWAT can be found in the theoretical documentation (http://swatmodel.tamu.edu) and [13].
-
-
Model setup and scenarios
The SWAT model was setup following three sequential steps: watershed delineation, HRU definition and writing input tables for weather variables. The weather variables such as daily precipitation, minimum and maximum temperature were
defined for 36 meteorological stations that are found inside and near the study area. The surface runoff and potential evapotranspiration were computed by the SCS-CN and Hargreaves methods, and the Muskingum method was used for routing flows into subbasins outlets. HRUs were defined by setting HRU thresholds (10-20-10 percent) for land use, soil type and slope. The land use, soil type, and slope that cover more than the specified percent, in each subbasin, were considered in the simulation. HRUs are the subdivision of subbasins and have homogeneous land use, management, and soil characteristics.
In general, two SWAT setups were created. Both setups had the same input data, hydrological processes computation methods, and HRU thresholds except the number of subbasins. The study basin was delineated using a minimum threshold drainage area, which is required for defining the origin of a river. The minimum drainage areas that were used for the SWAT model setup1 (hereinafter called scenario1) and setup2 (hereinafter called scenario2) were 720,095ha and 120,095ha, respectively. Scenario1 and Scenario2 had 25 and 145 number of subbasins, respectively (Fig. 3) with corresponding 125 and 962 HRUs. The first three years (2002-2004) meteorological data was used for warming up the model. The measured streamflow data were equally divided for SWAT model calibration (2005-2009) and validation (2010-2014).
-
-
RESULTS AND DISCUSSION
-
Sensitivity Analysis
The purpose of sensitivity analysis is to decrease the number of parameters in the calibration process by removing the less sensitive parameters [20]. Sensitivity analysis was done using the more reliable all-at-a time (global) method that uses a multiple regression approach for quantifying sensitivity. The minimum number of runs required to perform sensitivity analysis is 500. But based on the number of parameters more number of runs are allowed [20].
Sensitive flow parameters of the SWAT model (Table 3) were collected from previous journals [9], [21], [22].The sensitivity of 22 parameters was tested in the two delineation scenarios using 800 simulation runs.
As shown in the Fig. 4, the rank and kind of sensitive parameters were different in the two scenarios for the same number of simulations (800) and length of historical data. The rank of sensitive parameters may be influenced by the number of iterations and length of flow and rainfall data [22]. It is a must to determine the most sensitive flow parameters for a given watershed [22]. Because, the type of input data observations are different in different regions and also the changes in model input parameters affect modeling results. Additionally, it is helpful to define the influential hydrological processes [2]. For scenario1 the first four sensitive flow parameters were CN2, CH_N2, CANMX and CH_K2, respectively (Fig. 4B). But for scenario2 CH_N2, ALPHA_BF, GWQMN and CH_K2 were found to be the most sensitive parameters (Fig. 4A).
-
Calibration
Calibration minimizes errors between observations and model simulations by optimizing an objective function. In this study automatic calibration was done at multi-gauge stations (Kessie, El-Diem, and Khartoum), for the two delineation scenarios, for the period 2005-2009. For the calibration 13 sensitive parameters obtained from a combination of the first ten sensitive parameters of each scenario were used.
The values of R2 and NSE resulted in, respectively, 0.65 and 0.54 at Kessie, 0.78 and 0.76 at El-Diem, and 0.80 and
0.71 at Khartoum for scenario1; and 0.68 and 0.63 at Kessie,
145 subbasins
25 subbasins
145 subbasins
25 subbasins
0.80 and 0.77 at El-Diem, and 0.84 and 0.84 at Khartoum for scenario2 after calibration (Table 5).
Fig. 3. A schematic representation of the study area in SWAT model with subbasins
Fig. 4. Values for t-stat and p-value for the first ten sensitive parameters (A) delineation scenario2 (B) delineation scenario1
Table 4 shows fitted values for the calibrated SWAT flow parameters for delineation scenario 1 and 2. The fitted values were different for both scenarios. The graphical performance of the model after calibration is shown in Fig. 5.
Ref. [12] Simulated SWAT model for finer (8-digit) and coarser (12-digit) watershed delineations, to evaluate the effect of delineations on the runoff predictions, using monthly streamflow data. The values of R2 and NSE declined for the 12-digit delineation scheme since the model preliminary was calibrated for the 8-digit delineation. This indicates the need for calibrating the SWAT model, for a better runoff prediction capability, when the numbers of subbasins/HRUs are changed.
-
Validation
Validation was performed for the delineation scenarios using a daily streamflow data recorded from 2010 to 2014 at multi-gauge stations. The same number of simulations was used as in the last calibration iteration. Fig. 6 shows graphical plots of the observed and simulated flows after the validation step. The performance of SWAT was evaluated using NSE and R2 for the two scenarios as shown in Table 5.
The SWAT model underestimated the peak flows, particularly at Kessie station, which may be due to the quality of either the spatial or climate input data. However, the model well captured the low flows, rising and recession limbs at Kessie and Khartoum stations in scenario2. But there is a big discrepancy between the simulated and measured flows for the recession limbs at El-Diem station. As shown in Fig. 6, the recession limb is not well captured starting from 2012 which may be due to upstream developments that were not adequately represented in the model.
Table 3.Description and values range for flow parameters that selected for sensitivity analysis. The method r multiplies the existing value by (1+ the given value), and v replaces existing value by the given value. Units given by (-) denote no unit
Type of change_ parameter
Description (units)
Min. value
Max. value
r_CN2
Initial SCS runoff curve number for moisture condition-II (-).
-0.3
0.3
v_ALPHA_BF
Bae flow alpha factor (1/days).
0
1
v_GW_DELAY
Groundwater delay time (days).
0
500
v_GWQMN
Threshold depth of water in the shallow aquifer required for return flow to occur (mm H2O).
0
5000
r_SOL_K
Saturated hydraulic conductivity (mm/hr)
-0.5
-0.35
r_SOL_BD
Moist bulk density (Mg/m3 or g/cm3 )
-0.65
-0.54
r_SOL_AWC
Available water capacity of the soil layer (mm H2O/ mm soil)
-0.1
0.1
v_ESCO
Soil evaporation compensation factor (-).
0
1
v_EPCO
Plant uptake compensation factor (-).
0
1
v_GW_REVAP
Groundwater revap coefficient (-)
0.02
0.2
v_CH_N2
Mannings n value for the main channel (-).
0.01
0.3
v_CH_K2
Effective hydraulic conductivity in main channel alluvium (mm/hr).
0.01
500
v_SLSOIL
Slope length for lateral subsurface flow (m).
0
150
v_SLSUBBSN
Average slope length (m).
10
150
v_SURLAG
Surface runoff lag coefficient (-).
0.05
24
v_REVAPMN
Threshold depth of water in the shallow aquifer for revap or percolation to the deep aquifer to occur (mm H2O)
0
500
v_RCHRG_DP
Deep aquifer percolation fraction (-).
0
1
v_CANMX
Maximum canopy storage (mm H2O).
0
100
r_SOL_Z
Depth from soil surface to bottom of layer (mm)
-0.3
0.3
v_TLAPS
Temperature lapse rate (oC/Km).
-10
10
v_OV_N
Mannings n value for overland flow (-).
0.01
1
v_ALPHA_BNK
Baseflow alpha factor for bank storage (days).
0
1
r_SOL_ALB
Moist soil albedo (-)
-0.3
0.3
-
Effect of watershed delineation on streamflow simulation
Table 5 shows values for the most widely used statistical indices, which were calculated to investigate the effect of watershed delineation on SWAT simulation accuracy. The performance of SWAT increased for the simulated flow for scenario2 (finer delineation). After validation of scenario2, the value of NSE was increased by 1%, 12% and 7% at Kessie, El-Diem and Khartoum station, respectively related to scenario1. This variation might have occurred due to regroup of minor land uses into major land use as the number of subbasins increased (Table 6).
Parameters
Min. value
Max. value
Fitted values
Scenario1
Scenario2
r CN2.mgt
-0.2
0.2
0.013
0.020
v CH_N2.rte
-0.01
0.3
0.140
0.277
v CANMX.hru
0
100
62.617
21.803
v CH_K2.rte
-0.01
500
266.402
176.755
v RCHRG_DP.gw
0
1
0.212
0.333
v HRU_SLP.hru
0
1
0.492
0.290
v ALPHA_BF.gw
0
1
0.298
0.534
v GW_REVAP.gw
0.02
0.2
0.144
0.102
r SOL_AWC.sol
-0.2
0.2
0.133
-0.121
v OV_N.hru
0.01
1
0.227
0.266
v_GWQMN.gw
0
5000
1716.162
4113.273
v_ESCO.hru
0
1
0.744
0.724
v_SLSOIL.hru
0
150
37.389
103.590
Parameters
Min. value
Max. value
Fitted values
Scenario1
Scenario2
r CN2.mgt
-0.2
0.2
0.013
0.020
v CH_N2.rte
-0.01
0.3
0.140
0.277
v CANMX.hru
0
100
62.617
21.803
v CH_K2.rte
-0.01
500
266.402
176.755
v RCHRG_DP.gw
0
1
0.212
0.333
v HRU_SLP.hru
0
1
0.492
0.290
v ALPHA_BF.gw
0
1
0.298
0.534
v GW_REVAP.gw
0.02
0.2
0.144
0.102
r SOL_AWC.sol
-0.2
0.2
0.133
-0.121
v OV_N.hru
0.01
1
0.227
0.266
v_GWQMN.gw
0
5000
1716.162
4113.273
v_ESCO.hru
0
1
0.744
0.724
v_SLSOIL.hru
0
150
37.389
103.590
Table 4.Calibrated parameters and their fitted values for sceanrio1and Scenario2
These results are similar to the findings of [6], [7], [9] that computed model accuracy using the measured and uncalibrated simulated streamflow. Ref. [6] found 12% streamflow increment between the coarsest and finest watershed delineations. Ref. [9] found less than 10 percent increment as the number of subwatersheds increased by 26 percent. Ref. [7] obtained improved SWAT flow prediction accuracy due to the increase in the number of subwatershed and/or HRUs.
In this study area the major land uses are croplands (53.24%), forest (23.53%) and pasture (17.38%). As shown in Table 6, for scenario1, land uses distribution deviated by < 0.78% from the original (0% threshold) distribution except for forest and croplands at El-Diem and water at Kessie. At El- Diem, forest increased by 5.44% but croplands decreased by 6.47%. At Kessie, water areas decreased by 1.33%. However, for scenario2 the discrepancy of land use distribution compared to the original data is noticeable at the three stations. Croplands increase from 53.24% to 56.43% at Khartoum, from 53.37% to 56.53% at El-Diem, and from 78.03% to 80.54% at Kessie.
Table 5.Values of statistical indices for model calibration and validation
Scenario 1
Scenario 2
Gauge stations
Calibration
validation
calibration
validation
R2
NSE
R2
NSE
R2
NSE
R2
NSE
Kessie
0.65
0.54
0.76
0.74
0.68
0.63
0.79
0.75
El-Diem
0.78
0.76
0.67
0.47
0.8
0.77
0.66
0.59
Khartoum
0.8
0.71
0.81
0.78
0.84
0.84
0.86
0.85
Land uses distribution (%)
FRST
RNGB
PAST
AGRR
WETN
RNGE
SWRN
URMD
WATR
A) For areas upstream of Khartoum station (drainage area = 307,189 Km2)
Original
23.53
3.57
17.38
53.24
0.13
0.17
0.43
0.25
1.33
Scenario 1
23.29
3.17
17.1
53.46
0.2
0.41
0.62
0.66
1.19
Scenario 2
20.37
3.35
16.83
56.43
0.18
0.62
0.6
0.72
2.07
B) For areas upstream of El-Diem station (drainage area = 174,947 km2)
Original
27.76
3.03
13.56
53.37
0.13
0.00
0.04
0.135
1.97
Scenario 1
33.2
3.8
14.1
46.90
0.1
0.00
0.0
0.1
1.7
Scenario 2
25.81
2.61
12.49
56.53
0.15
0.00
0.04
0.14
2.27
C) For areas upstream of Kessie station (drainage area = 64,737 Km2 )
Original
2.06
2.98
11.62
78.03
0.24
0.00
0.09
0.19
4.79
Scenario 1
2.69
2.71
11.95
78.68
0.22
0.00
0.08
0.21
3.46
Scenario 2
2.05
2.21
9.94
80.54
0.27
0.00
0.08
0.2
4.76
Land uses distribution (%)
FRST
RNGB
PAST
AGRR
WETN
RNGE
SWRN
URMD
WATR
A) For areas upstream of Khartoum station (drainage area = 307,189 Km2)
Original
23.53
3.57
17.38
53.24
0.13
0.17
0.43
0.25
1.33
Scenario 1
23.29
3.17
17.1
53.46
0.2
0.41
0.62
0.66
1.19
Scenario 2
20.37
3.35
16.83
56.43
0.18
0.62
0.6
0.72
2.07
B) For areas upstream of El-Diem station (drainage area = 174,947 km2)
Original
27.76
3.03
13.56
53.37
0.13
0.00
0.04
0.135
1.97
Scenario 1
33.2
3.8
14.1
46.90
0.1
0.00
0.0
0.1
1.7
Scenario 2
25.81
2.61
12.49
56.53
0.15
0.00
0.04
0.14
2.27
C) For areas upstream of Kessie station (drainage area = 64,737 Km2 )
Original
2.06
2.98
11.62
78.03
0.24
0.00
0.09
0.19
4.79
Scenario 1
2.69
2.71
11.95
78.68
0.22
0.00
0.08
0.21
3.46
Scenario 2
2.05
2.21
9.94
80.54
0.27
0.00
0.08
0.2
4.76
Fig. 5. Simulated versus observed streamflow for scenario1 (A-C) and scenario2 (D-F) after calibration Table 6.Land use distribution (%) for areas contributing flows to gauging stations
Water increased by less than 0.75% at Khartoum and El-Diem. But forest and pasture decreased by 3.16% and 0.55% at Khartoum, by 1.45% and 1.05% at El-Diem and by 0.01% and 1.68% at Kessie (Table 6). In particular, land use/cover changes have a major effect on
surface runoff and groundwater flow [23]. The effect of land use change on the rate and volume of runoff is considerable [24]. The runoff rates and volumes are excessive in a watershed with much row cropping, grazing and urbanization.
Fig. 6. Simulated versus measured flow after validation for Scenario1 (A-C) and scenario2 (E-G)
The physical watershed characteristics such as climate,
slope, watershed shape and area, rainfall, soil type and land use/cover have effect on hydrological processes. In particular, land use/cover changes have major effects on surface runoff and groundwater flow [23]. The effect of land use change on the rate and volume of runoff is considerable [24]. The runoff rates and volumes are excessive in a watershed with much row cropping, grazing and urbanization.
Curve Number method depends on the Curve Number coefficient to estimate the direct runoff from rainfall. The surface runoff over the study area was estimated by the SCS- CN method (2) using SWAT. The method defines CN by integrating soil type, watershed condition, land use and cover density. All land uses have different CN values. The CN of row crops and pasture land use are larger than the CN of forest land use [25]. Increasing the CN increases the peak flood magnitude [26].
Larger number of subbasins had negligible effect on SWAT model performance for streamflow simulation in the upper subbasin outlet (Kessie station). For a watershed area (below 64,737 km2 in this study) a default MDA of SWAT or higher numbers might be used for subbasin delineation. However, a smaller MDA should be used for a larger basin areas (>174,947 km2 in this study). Ref. [11] indicated that as the area of a watershed should be considered when delineating a watershed for streamflow simulation.
-
-
CONCLUSION
The effect of watershed delineation on daily streamflow simulation using SWAT was investigated in the Blue Nile Basin. The basin was delineated with 25 and 145 subbasins for scenario1 and scenario2, respectively. Sensitivity analysis, calibration and validation were done for each scenario using Sequential Uncertainty Fitting Algorithm (SUFI-2), same input datasets, HRU thresholds (10-20-10 for land use-soil type-slope), flow parameters, and equal number of simulation runs. The study revealed the following key points mentioned below:
-
The sensitivity rank and fitted values (after calibration) of selected SWAT flow parameters were different for the scenario1 and scenario2.
-
There was a good agreement between the measured and simulated daily flows with the values of R2 and NSE found between 0.46 and 0.87 after calibration and validation for both scenarios at multi-gauge stations.
-
The performance of SWAT improved by less than 12%, in terms of NSE, during validation of scenario2 relative to scenario1. This result was in line with the findings of [6], [7], [9], who evaluated SWAT model performance using the measured and uncalibrated simulated flows.
Overall, it can be concluded that the number of subbasins has effect on streamflow simulation using SWAT in the Blue Nile Basin. We recommended SWAT users to use optimal number of subbasins instead of the default MDA for watershed delineation. The optimal number of subbasins can be found by evaluating the measured and uncalibrated simulated streamflow for different MDAs.
4. ACKNOWLEDGMENT
We are grateful to the different Ethiopian institutions that provided us with relevant hydro-meteorological and spatial datasets. Our appreciation also goes to the Sudanese Meteorology Agency and Ministry of Irrigation and Water Resources for giving us observed hydro-meteorological datasets free of charge. We are also thankful to the Ethiopian Water Resources Institute for the financial and administrative supports.
REFERENCE
-
D. R. Bourdin, S. W. Fleming, and R. B. Stull, Streamflow modelling: A primer on applications, approaches and challenges, Atmos. – Ocean, vol. 50, no. 4, pp. 507536, 2012, doi: 10.1080/07055900.2012.734276.
-
J. G. Arnold et al., SWAT: Model use, calibration, and validation, Trans. ASABE, vol. 55, no. 4, pp. 14911508, 2012.
-
K. Ly, G. Metternicht, and L. Marshall, Transboundary river catchment areas of developing countries: Potential and limitations of watershed models for the simulation of sediment and nutrient loads. A review, J. Hydrol. Reg. Stud., vol. 24, no. September 2018, p. 100605, 2019, doi: 10.1016/j.ejrh.2019.100605.
-
W. D. Troolin and K. Clancy, Comparison of Three Delineation Methods Using the Curve Number Method to Model Runoff, J. Water Resour. Prot., vol. 08, no. 11, pp. 945964, 2016, doi: 10.4236/jwarp.2016.811077.
-
Y. Luo, B. Su, J. Yuan, H. Li, and Q. Zhang, GIS techniques for watershed delineation of SWAT model in plain polders, Procedia Environ. Sci., vol. 10, no. PART C, pp. 20502057, 2011, doi: 10.1016/j.proenv.2011.09.321.
-
T. W. FitzHugh and D. S. Mackay, Impacts of input parameter spatial aggregation on an agricultural nonpoint source pollution model, J. Hydrol., vol. 236, no. 12, pp. 3553, 2000, doi: 10.1016/S0022- 1694(00)00276-6.
-
S. Mamillapalli, R. Srinivasan, J. G. Arnold, and B. a Engel, Effect of Spatial Variability on Basin Scale Modeling, Third Int. Conf. Integr. GIS Environ. Model., no. 1994, pp. 111, 1996.
-
H. Rouhani, P. Willems, and J. Feyen, Effect of watershed delineation and areal rainfall distribution on runoff prediction using the SWAT model, Hydrol. Res., vol. 40, no. 6, pp. 505519, 2009, doi: 10.2166/nh.2009.042.
-
S. G. Setegn, R. Srinivasan, and B. Dargahi, Hydrological Modelling in the Lake Tana Basin, Ethiopia Using SWAT Model, Open Hydrol. J., vol. 2, no. 1, pp. 4962, 2008, doi: 10.2174/1874378100802010049.
-
M. Jha, P. W. Gassman, S. Secchi, R. Gu, and J. Arnold, Effect of watershed subdivision on swat flow, sediment, and nutrient predictions,
J. Am. Water Resour. Assoc., vol. 40, no. 3, pp. 811825, 2004, doi: 10.1111/j.1752-1688.2004.tb04460.x.
-
L. Chiang and Y. Yuan, The NHDPlus dataset , watershed subdivision and SWAT model performance The NHDPlus dataset , watershed subdivision and SWAT model performance, vol. 6667, 2015, doi: 10.1080/02626667.2014.916408.
-
M. Chen, Y. Cui, P. W. Gassman, and R. Srinivasan, Effect of watershed delineation and climate datasets density on runoff predictions for the upper mississippi river basin using SWAT within HAWQS, Water (Switzerland), vol. 13, no. 4, 2021, doi: 10.3390/w13040422.
-
J. G. Arnold, R. Srinivasan, R. S. Muttiah, and J. R. Williams, Large area hydrologic modeling and assessment part I: Model development,
Journal of the American Water Resources Association, vol. 34, no. 1. pp. 7389, 1998, doi: 10.1111/j.1752-1688.1998.tb05961.x.
-
Z. K. Tesemma, Y. A. Mohamed, and T. S. Steenhuis, Trends in rainfall and runoff in the Blue Nile Basin: 1964-2003, Hydrol. Process., vol. 24, no. 25, pp. 37473758, 2010, doi: 10.1002/hyp.7893.
-
K. C. Abbaspour, S. A. Vaghe, H. Yang, and R. Srinivasan, Global soil
, landuse , evapotranspiration , historical and future weather databases for SWAT Applications, pp. 111, 2019, doi: 10.1038/s41597-019- 0282-4.
-
Y. Xu, Package hyfo , 2020.
-
K. Wang, P. Wang, J. Liu, M. Sparrow, S. Haginoya, and X. Zhou, Variation of surface albedo and soil thermal parameters with soil moisture content at a semi-desert site on the western Tibetan Plateau, Boundary-Layer Meteorol., vol. 116, no. 1, pp. 117129, 2005, doi: 10.1007/s10546-004-7403-z.
-
B. J. Cosby, G. M. Hornberger, R. B. Clapp, and T. R. Ginn, A Statistical Exploration of the Relationships of Soil Moisture Characteristics to the Physical Properties of Soils, Water Resour. Res., vol. 20, no. 6, pp. 682690, 1984, doi: 10.1029/WR020i006p00682.
-
J. R. Williams, The EPIC Model in Computer Models of Watershed Hydrology Chapter 25 (Water Resources Publications, Highlands Ranch, CO., 1995.
-
K. C. Abbaspour, S. A. Vaghefi, and R. Srinivasan, A Guideline for Successful Calibration and Uncertainty Analysis for Soil and Water Assessment: A Review of Papers from the 2016 International SWAT Conference, no. ii, 2018, doi: 10.3390/w10010006.
-
S. Rebecca, S. Dhungana, and N. Bhatta, Application of SWAT Model for Estimating Runoff in Upper Nile River Basin, Eur. J. Eng. Sci. Technol., 2019, doi: 10.33422/ejest.2019.09.35.
-
L. L. Moreira, D. Schwamback, and D. Rigo, Sensitivity analysis of the Soil and Water Assessment Tools (SWAT) model in streamflow modeling in a rural river basin, Ambient. Ãgua – An Interdiscip. J. Appl. Sci., vol. 13, p. 1, 2018, doi: 10.4136/ambi-agua.2221 Received:
-
S. B. Aynalem and M. G. Liben, Evaluating the Effects of Watershed Characteristics on River Flow for the Case of Fetam River , Ethiopia, vol. 8, no. 6, pp. 139149, 2020, doi: 10.11648/j.ajce.20200806.12.
-
S. Satheeshkumar, S. . Venkateswaran, and R. Kannan, Rainfall runoff estimation using SCS CN and GIS approach in the Pappiredipatti watershed of the Vaniyar sub basin , South India, Model. Earth Syst. Environ., vol. 3, no. 1, pp. 18, 2017, doi: 10.1007/s40808- 017-0301-4.
-
D. ietz and R. Hawkins, Effects of Land Use on Runoff Curve Number, vol. 40499, no. November, 2001, doi: 10.1061/40499(2000)110.
-
J. Swingly, F. Sumarauw, and K. Ohgushi, Analysis on Curve Number
, Land Use and Land Cover Changes and the Impact to the Peak Flow in the Jobaru River Basin , Japan, no. April, 2012.