
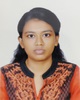
- Open Access
- Authors : Adlin Chericha D J , Sakthivel R , Linda Theres B
- Paper ID : IJERTV11IS040233
- Volume & Issue : Volume 11, Issue 04 (April 2022)
- Published (First Online): 05-05-2022
- ISSN (Online) : 2278-0181
- Publisher Name : IJERT
- License:
This work is licensed under a Creative Commons Attribution 4.0 International License
Deciding Flood Premium Rates based on Flood Risk Zones using Remote Sensing and GIS Techniques: A Case Study of Cuddalore District, Tamil Nadu, India
1Adlin Chericha D J 1Department of Geography, University of Madras Chennai, India
2*Sakthivel R
*Corresponding author Department of Geography,
University of Madras, Chennai, India
3Linda Theres B
3School of Civil Engineering SASTRA Deemed to be University Thanjavur, India
Abstract Flood hazards have increased in frequency due to climate changes. A flood can lead to catastrophic consequences. Flood insurance helps us to cope with the damages of a flood. The risk of flood varies for each property due to heterogeneity in the earths topography. The insurance policyholders are currently paying a uniform premium for flood insurance unaware of the risk associated with their property. GIS can be used to add the risk factor to the premium. The flood insurance premium can be discounted for properties in low-risk zones. The insureds in the high-risk zone have higher chances of claiming flood insurance, hence can be assigned a higher premium rate. GIS when integrated with various layers can offer significant benefits for strategic planning, marketing and sales, and claims handling. GIS techniques can be used for visualization as well as analysis of the risk. A flood insurance model has been generated to calculate the discounted premium for flood insurance based on the risk.
KeywordsGIS; flood insurance, flood risk zonation, GIS- based flood insurance discounted premium
-
INTRODUCTION
Floods are one of the most recurring and devastating natural hazards, impacting human lives and causing severe economic damage throughout the world. With the onset of climate change, flood intensity and frequency threaten many regions of the world. The economic development has paved way for a potential increase in flood casualties and damages in many regions. Flood hazard is expected to increase in frequency and severity through the impacts of global change on climate, severe weather in the form of heavy rains, and river discharge conditions. The urban development in the flood sinks is hazardous making it prone to floods. The flood frequency and intensity will not subside shortly. The current trend and future scenarios of flood risks, therefore, demand accurate spatial and temporal information on the potential hazards and risks of floods. In past years several researchers efficiently produced a flood hazard map using remote sensing and GIS techniques [1, 2, 3, 4, 5, 6, 7]
Insurance is a plan in which a large number of people associate with them and share the risks associated with an individual. The insurance guards the people against the unexpected manmade/natural losses in a region. Insurance provides protection, certainty, and distribution of risk. The damage caused by water that is in contact with the ground outside like rivers, creeks, and flash flooding is protected by a flood insurance policy. The flood risk is based on the precise location of the property. The flood insurance premium can be discounted/increased within the maximum and minimum premium rates based on the location of the property. Geographic Information System (GIS) is useful in delineating the Flood Risks Zone (FRZ) and for the determination of the location of the property. This present study attempted to synthesize a spatial framework for flood insurance. Without knowledge of the real geographic exposure patterns, insurers face the risk of concentrating a large portfolio in high flood- prone areas and can lose their profit opportunities or it can even lead to bankruptcy. So, an insurer can avoid bankruptcy by being aware of the risk he is going to indulge in and generating an insurance policy based on that. Re-insurance can be opted for by the insurance companies to diversify their risk. Re-insurers have increasingly adapted to the utility of GIS technology. GIS-aided risk concentration analysis is already a standard feature. When disaster strikes, claim managers will use GIS technologies in their work. Theyll be able to generate detailed maps showing the location of their affected risks, enabling rapid response to assist their insureds. The Thematic layers for the study area were determined based on the work by [8, 9, 10, 6]. The weights and ranks were assigned based on the hierarchy of contribution of the various layers in the above studies. The weightage and ranks have been modified based on the study area characteristics. The GIS model discounted flood insurance premium rates was inspired by the work of
reference [11].
-
STUDY AREA
The Cuddalore district is located in the eastern coastal region in the state of Tamil Nadu. Cuddalore district lies within the geographic coordinates of latitude: 150 5 /110 11 and 120 35 N, longitude: 780 38 to 800 00. The district is bordered by the Bay of Bengal on the eastern side. It is bordered by the districts of Viluppuram, Nagapattinam, and Perambalur. The district headquarters in Cuddalore town is located at the estuary of river Gedilam and Pennaiyar with the Bay of Bengal. The district has a coastline of 57.5kms. The total area of the Cuddalore district is 3678 sq. km. It has an average altitude of 4.6 MSL. The Study area map is shown in Fig 1.
The district has a hot tropical climate. Cuddalore district is influenced by the Southwest and North-East Monsoons. Cuddalore district normally receives an annual rainfall of 1086.4 mm. The normal annual rainfall over the district varies from about 1050 mm to about 1400 mm. Cuddalore district comprises 10 Administrative Taluks, 683 village panchayats, 14 Panchayat Unions, 5 Municipalities and 16 Town Panchayats, and One Township. It is divided into 896 revenue villages which in turn form 3 Revenue divisions, viz Cuddalore, Chidhambaram, and Virdhachalam.
Fig 1. Location map of the study area Cuddalore District, Tamil Nadu, India
-
MATERIALS AND METHODS
The Present study uses elevation, slope, flow accumulation, soil, geomorphology, and drainage density to delineate the flood-prone regions in the study area. A prescriptive model was framed to calculate the flood insurance premium rate. The Data for the study was collected from the following sources Table I.
TABLE I. DATA SOURCES
The Software used for the study is ArcGIS 10.5. Microsoft Excel was used for calculation purposes. GIS and criteria evaluation was employed to access the risk in the villages. The criteria have been evaluated in GIS to suggest flood risk zones based on the physical characteristics of the study area and the workflow of methodology for the study is shown in Figure 2.
The Thematic layers were prepared from remote sensing data using geotechnical methods. The weighted sum analysis was carried out for the thematic layers by assigning the weights based on their flooding tendency. The generated flood risk zonation map classified the study area into five classes (i.e.) very high, high, moderate, low, and very low- risk zones. The revenue village map was overlaid and the average risk for the village was extracted. The extracted flood risk value was substituted in the developed model and the flood insurance premium rates were calculated.
Fig 2. Methodology
-
Weighted Sum Analysis
The weighted sum tool is used to solve multi-criteria problems such as site selection and suitability models. Unlike the weighted overlay, it doesnt calibrate to a scale again. The weighted overlay was carried out for the various layers with the above (Table II) mentioned weights and ranks. The weighted sum layer was classifid into five categories: – very high, high, moderate, low, and very low prone to flood. The discounted premium rates were calculated for each polygon. The classification was done in the Natural breaks (Jenks) method.
Layer
Weightage
Categories
Rank
ELEVATION
28
Very high
5
High
4
Moderate
3
Low
2
Very low
1
SLOPE
24
0 – 0.9331
5
0.9332 – 1.6
4
1.601 – 2.266
3
2.267 – 3.199
2
>3.2
1
SOIL
10
Clay
5
Clay loam
4
Loamy sand
2
Sandy loam
1
Miscellaneous
3
TABLE II. WEIGHTAGE AND RANKS ASSIGNED TO EACH LAYER
Elevation
SRTM DEM, USGS Earth Explorer
Slope
SRTM DEM, USGS Earth Explorer
Flow accumulation
SRTM DEM, USGS Earth Explorer
Soil
National Bureau of soil survey and land use planning
Geomorphology
Bhuvan
Drainage density
SRTM DEM, USGS Earth Explorer
Cuddalore district revenue village boundary
nirt.in; State ground and surface water resources data centre, Taramani, Chennai.
DRAINAGE DENSITY
18
Very high
5
High
4
Moderate
3
Low
2
Very low
1
FLOW ACCUMULAT ION
14
4400000 –
7700000
5
2500000-
4400000
4
1300000-
2400000
3
340000-
1200000
2
0-330000
1
GEOMORPHO LOGY
6
Floodplain
5
Coastal
4
Pediplain
3
Alluvial plain
2
Upland
1
The GIS-based premium rates were determined using the above formula (equation 1) for each revenue village.
-
-
RESULTS AND DISCUSSIONS
Insurance coverage was the same for different land suitability, but the premiums were different. GIS can integrate all the necessary data to facilitate rating decisions and is indeed valuable to insurers. GIS technology can be used as an analytic tool to assist in developing predictive loss models. GIS facilitates sales planning and monitoring by analyzing concentrations of risks in conjunction with other available geographic data such as demographic and historical loss data. When integrating the buyer characteristics with rating and hazard information, key areas of opportunity can be uncovered. GIS offers significant benefits for strategic planning, marketing and sales, and claims handling.
-
Risk Assessment for revenue villages
The centroid of the revenue village polygons was found. The point layer was overlaid over the risk layer and the risk associated with each point was interpolated and found. The risk value for each polygon was rationalized with the highest value and multiplied by the difference between the highest and lowest premium rates and added to the minimum premium rate to maintain the premium rates above the minimum premium rate. The discounted rates for the premium were estimated using the model developed below in Fig 3.
-
Thematic Layers
The present study uses the thematic layers elevation, slope, flow accumulation, soil, geomorphology, and drainage density to delineate the flood-prone regions in the study area.
-
Elevation
The elevation is a factor that plays an important role in flooding. The elevation of the study area was determined from the SRTM DEM data. The higher the elevation lowers the chances of flooding. Hence higher elevation was given lower ranks. The uplands were found in the northwestern part of the district. The elevation increased from the east to the west. The coastal region was the low-lying region.
C. Flood insurance prescriptive model
A model was generated for flood insurance based on
-
Slope
-
The Slope influences the direction and amount of
the risk. The unique risk probability ratios were generated for each revenue village by dividing the difference between the highest risk value and each villages risk value with the highest risk value. This ratio was then multiplied by the difference between the regulated maximum and minimum premiums rates to get the GIS discountable premium rate. The GIS discounted premium rates are added to the minimum premium rate. Gmax is only used for the calculation of the discounted premium. The reason for using upper limits is to ensure maximizing the risk load and minimizing the risk discount. Rmax is 10% while Rmin is 0.3%. The Gmax and Gmin are calculated for each revenue village in the city.
GIS Highest risk territory upper limit=Imax Maximum regulated rate=Rmax
Minimum regulated rate=Rmin
GIS risk territory upper limit=Gmax GIS risk territory lower limit=Gmin
The constraints or conditions under which the model operates
optimally are GIS Discounted Rate (GR) cannot be more than IRA Maximum Regulated Rate (Rmax) and cannot be less than IRA Minimum Regulated Rate (Rmin).
surface runoff or subsurface drainage reaching a site. The
Slope has a dominant effect on the contribution of rainfall to streamflow. It controls the duration of overland flow, infiltration, and subsurface flow. The slope indicates the geomorphological properties of the soil and refers to the topography of the area. The thickness of the soil layer decreases with the increase of the slope. The Slope was calculated from the maximum rate of change between each cell and its neighbors. Every cell in the output raster had a slope value. A lower slope value indicates a flatter terrain that has high chances of flooding and a higher slope value indicates a steeper terrain that has high runoff. Hence the low slope areas were given higher ranks and high slope areas were given lower ranks. The slope map was generated using SRTM DEM data.
Fig 3. GRi Formulae
(1)
Fig 3. Elevation and Slope Map of Cuddalore District
-
Drainage density
Drainage density indicates the closeness of spacing of streams and is a key detrimental factor for water to travel from source to sink. Drainage density is an inverse function of infiltration, lesser the infiltration of rainfall, it conversely tends to be concentrated in surface run-off. Drainage density is classified into 5 categories, high drainage density values are favorable for runoff and hence indicate low flood chance. Higher ranks have been assigned to poor drainage density areas and lower ranks have been assigned to areas with adequate drainage.>
The coastal plains have poor drainage and hence have been assigned the rank of 4. The alluvial plain has a good drainage system and is utilized for agriculture.
-
Soil
The Soil types in an area are important as they
control the amount of water that can infiltrate into the soil, and hence the amount of water that becomes to flow. The chance of flood hazards increases with a decrease in soil infiltration capacity, which causes an increase in surface runoff. When water is supplied at a rate that exceeds the soils infiltration capacity, it moves down the slope as runoff on sloping land and can lead to flooding. Ranks are assigned for soil categories based on infiltration capacity. The clay soils are less porous and hold water longer than sandy soils. This implies that areas characterized by clay soils are more affected by flooding and are likely to become waterlogged in the rainy season. Clay loam contains more clay hence it tends to get heavy. It has poor drainage as it gets wet, it swells to retain it. Loamy sand contains all the three size particles. Hence it has good drainage. Sandy loam is made up of varying amounts of sand, silt, and clay allowing it to have good drainage.
Fig 4. Drainage Density and Soil Map of Cuddalore District
-
Flow accumulation
The Flow accumulation calculates accumulated flow as the accumulated weight of all cells flowing into each downslope cell in the output raster. The flow accumulation pixels were classified into five classes: very high accumulation, high accumulation, moderate accumulation, low accumulation, and very low accumulation.
-
Geomorphology
The Study Area has the geomorphological features: upland, alluvial plain, Pediplain, coastal plain, and flood plain. Pediplain covers nearly 48% of the study area while floodplain is 7%, alluvial plain 24.5%, coastal plain 8.5%, and upland 11.5%. The flood plains are low-lying areas beside the waterbodies hence they are highly flood-prone.
Fig 5. Flow Accumulation and Geomorphology Map of Cuddalore District
-
Risk segmentation
The flood risk segmentation (Fig 7 & 8) was done for the Cuddalore district and the study area was classified into very high, high, moderate, low, and very low flood- prone. 11.98% of the study area falls under the very low-risk zone while 22.92% under the low-risk zone, 24.27% under the moderate risk zone, 25.08% under the high-risk zone, and 15.75% under the very high-risk zone.
Fig 6. Flood Risk Zones of Cuddalore District
Fig 7. Flood Risk Zones in Villages
-
GIS Discounted premium rates
The highest normalized risk value for the villages was found to be 410. The difference between the maximum and minimum rate is 9.7%. GIS discounted rate is the premium rate for flood insurance (Fig 9). The GIS discounted rate ranges from 3.61% to 10% in the study area.
Fig 8. GIS Discounted Premium Rates in Villages
Table 3 shows the calculation carried out below for a few sample points.
TABLE III. GIS DISCOUNTED PREMIUM RATES
-
-
CONCLUSIONS
Flood insurance helps the insured cope after a disaster without seeking help from the government or any third parties. The conventional methods drawback is that the insured or insurer may benefit from it. The insured and insurer will both enjoy benefits optimally when Flood Insurance premium rates are calculated based on risk. A large part of the Coastal Zone is low-lying with a gentle slope, resulting in large inundation, and therefore increased vulnerability to flooding. Hence most of the regions with high premium rates of flood insurance were found in the coastal region. The flood insurance will empower the coastal residents as they are often prone to floods.
The appropriate flood risk for each village has been demarcated based on multiple criteria using the weighted sum analysis method and the calculated flood insurance premium rates. The current study utilized and proved that remote sensing data and GIS data is an efficient tools for decision- making in real-time scenarios.
REFERENCES
[1] Adam Luke, Brett F. Sanders, Kristen A. Goodrich, David L. Feldman, Danielle Boudreau, Ana Eguiarte, Kimberly Serrano, Abigail Reyes, Jochen E. Schubert, Amir AghaKouchak, Victoria Basolo, and Richard-
Matthew, Going beyond the flood insurance rate map: insights from flood hazard map co-production., Nat. Hazards Earth Syst. Sci., 18, 2018, pp: 10971120.