
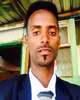
- Open Access
- Authors : Nesru Temam
- Paper ID : IJERTV9IS080270
- Volume & Issue : Volume 09, Issue 08 (August 2020)
- Published (First Online): 04-09-2020
- ISSN (Online) : 2278-0181
- Publisher Name : IJERT
- License:
This work is licensed under a Creative Commons Attribution 4.0 International License
Correlation and Path Coefficent Analysis of Pod Yield and Related Traits of Okra [Abelmoschus Esculentus (L.) Moench] Genotypes Evaluated at Melkassa, Centeral Ethiopia
Nesru Temam (MSc.)
Crop and Horticultural Biodiversity Directorate
Ethiopian Biodiversity Institute P.O. Box: 30726 Addis Ababa, Ethiopia
Abstract-Okra [Abelmoschus esculentus (L). Moench] is one of indigenous and endemic genetic resource of Ethiopia as well as Benishangul Gumuz. However, only few studies were carried out to assess its diversity and performance throughout the country and specifically no research conducted to assess diversity of okra within regional state. Therefore, this study was conducted with the objective to determine the association of traits and the direct and indirect effects of traits on fruit yield. A total of 36 genotypes of which 33 okra genotypes were collected from different areas of Benishangul Gumuz Regional State, 3(three) checks, of 2 introduced and 1(one) released were evaluated for 22 quantitative traits at MARC in 2018/19 using simple lattice design (6 x 6). The results from analysis revealed that number of primary branches (rg=0.8), fruit diameter (rg=0.329), number of tender fruit per plant (rg= 0.61), seed yield (rg=0.82), and frequency of harvests (rg=0.646) are correlated to fruit yield at genotypic and phenotypic levels, whereas average fruit weight was correlated at phenotypic level. Traits like number of primary branches, seed yield and average fruit weight had positive and significantly high direct effect on fruit yield. Therefore, they should be considered during selection for fruit yield improvement.
Keywords: Genotypic-correlation, Direct effect, Indirect effect, Path analysis, Phenotypic analysis
-
INTRODUCTION
Okra [Abelmoschus esculentus (L.) Moench] belongs to family Malvaceae and it is very important vegetable crop grown in tropical and sub-tropical parts of the world [1]. It is originated and native to North Eastern Africa in the area of Ethiopia and Sudan from where it extensively spread to Asia, America, Southern Europe and other countries [2, 3]. It is self-pollinated, mainly propagated by seeds with a duration of 3 to 4 months [4, 5].
It has a considerable area under cultivation in Africa and Asia in particular because of its contribution to the human diet by supplying fats, proteins, carbohydrates, minerals, and vitamins. Okra typically differs from most other common vegetables in having high mucilage content [6]. Its mucilage is suitable for medicinal and various industrial applications [7, 8 & 9]. The unripe green finger-like pod are processed and consumed as stews and salads, soups, sliced, boiled and fried vegetables [10, 11]. The seed is used as a coffee additive or substitute [12].
There is no complete data on production area and productivity of okra in Ethiopia, although it is a traditional crop in southwestern, western and northwestern Ethiopia [13].The crop is cultivated from landraces over the years in the country [14]. Recently in 2016, the first improved variety (Bamia Humera) has been recommended for cultivation [15]. Ethiopia is not benefiting from okra either for food and nutrition security as well as foreign currency income generation as an export commodity. However, the few studies conducted indicated the country is rich for the diversity of the crop [13, 16, 17, 18, 14 & 19] which is a good opportunity to improve the crop through characterization and evaluation the available germplasm in the country.
The collection of desirable plant germplasm relies on the proven accession features and genetic divergence, which are essential in genetic resources utilization through improvements [20, 21].
Improvement in plant breeding scheme leans on high genetic differences in the population and the magnitude of inheritance of favorable attributes [22]. The progress and gain from the selection in any breeding program depend upon the magnitude of useful variability present in the population and the degree to which the desired traits are heritable. Yield is a complex quantitative traits and controlled by several genes that interact with the environment. It is the product of various factors known as yield components. Moreover, the efficiency of selection in any breeding program mainly depends upon the knowledge of the association of the characters. The knowledge of associations between yield and its related traits could appreciably enhance the efficiency of the crop improvement through the utilization of the appropriate selection indices [23, 24]. Therefore, its imperative study the variability and association of traits among genotypes collected from regional state. Thence, the objective of this study was to determine the association of quantitative traits okra genotypes for yield and yield components that helps us to generated information for okra improvements.
-
MATERIALS AND METHODS
-
Description of Study Area
The study was conducted at Melkassa Agriculture Research center (MARC), Ethiopia in 2018/19 main cropping season. Malkasa is located 8024N latitude and 39021E by having a distance of around 112 K.M from Addis Abeba on the Eastern direction at an altitude of 1550 m.a.s.l. The area is characterized by low and erratic rainfall with a mean annual rainfall of 763 mm with peaks in July and August. The dominant soil type of the center is andosol of volcanic origin with pH that ranges from 7 to 8.2. The mean annual temperature is 21.20C with a minimum of 140C and a maximum of 28.40C [25].
-
Experimental Materials and Design
A total of 36 genotypes were evaluated of which 33 okra genotypes were collected from different areas of Benishangul Gumuz Regional State of Ethiopian by Ethiopian Biodiversity Institute and 2 (two) of the varieties were introduced from India and now registered as commercial variety in Ethiopia by one company and 1 (one) variety is released from Humera research center. The okra genotypes were collected at different altitudes ranging from 661 to 1518 m.a.s.l. The three registered varieties will be used as the standard checks. Genotypes was evaluated on the field in 6 x 6 simple lattice designs. Each plot had 0.8 m x 5.4 m (4.32 m2) consisting of one row and a total of 12 plants per row or per plot. The spacing between plant, plots and adjacent replications were 0.45, 0.8 and 2m, respectively. Three seeds were sown and thinned to one plant per hill when plants reached 4-5 leaves stage.
-
Data Collections
International Plant Genetic Resources Institute [26] descriptor list for okra species were used to record data on quantitative and qualitative traits. Quantitative traits were recorded from 10 plants per row leaving the two plants grown at both ends of the row as border plants and the two border plants were used for mature pod and seed traits measurement. Five randomly selected tender fruits from each harvest in each plot were used to record tender fruit characters.
-
Crop Phenology and Growth Traits
Days to emergence (50%), days to first flowering, days to 50% flowering, days to 90% maturity, number (frequency) of harvest, plant height (cm), stem diameter (cm), number of primary branches, number of internode, internodes length (cm), and peduncle length (cm) was measured properly.
-
Pod Yield and Yield Component
Fruit length (cm), fruit diameter (mm), average fruit weight (g), number of tender fruit per plant, fruit yield per hectare (t/ha-1), number of seed per fruit, hundred seed weight (g), and seed yield per hectare (kg) was taken accordingly.
-
Tender Frit Quality Related Traits
Dry matter content of tender fruit (%) and estimation of mucilage content of fruit (%) were also employed at laboratory.
-
-
Data Analysis
-
The Phenotypic and Genotypic Correlation Coefficient
Phenotypic (rp) and genotypic (rg) correlations between two traits were estimated using the formula suggested by [27, 28].
(1)
Where, = phenotypic correlation coefficient between character x and y
= phenotypic covariance between character x and y
= phenotypic variance for character x
= phenotypic variance for character y
(2)
Where: = genotypic correlation coefficient between character x and y
= genotypic covariance between character x and y
= genotypic variance for character x
= genotypic variance for character y
The coefficient of correlation at the phenotypic level was tested for significance by comparing the values of correlation coefficient with tabulated r-value at g-2 degree of freedom, where g is a number of genotypes. However, the coefficient of correlations at the genotypic level was tested for the significance using the formula described by Robertson (1959).
(3)
The calculatedt value was compared with the tabulatedt value at g-2 degree of freedom at 5% level of significance. Where, g= number of =genotypic correlation coefficient and, = standard error of genotypic correlation coefficient between character x and y which was calculated as:
(4)
Where: =standard error of genotypic correlation coefficient between character x and y, =Heritability value of character x and
= heritability value of character y.
-
Path Coefficient Analysis
-
Based on genotypic and phenotypic correlations, path coefficient analysis which refers to the estimation of direct and indirect effects of the fruit yield attributing characters (independent character) on fruit yield (dependent character) was calculated based on the method used [29] as follows:
(5)
Where, = mutual association between the independent character (i) and dependent character (j) as measured by the genotypic and phenotypic correlation coefficients. = direct effects of the independent character (i) on the dependent variable
(j) as measured by the genotypic path coefficients, and = Summation of components of indirect effects of a given independent character (i) on a given dependent character (j) via all other independent characters (k).
The residual effect, which determines how best the causal factors account for the variability of the dependent factor yield, was computed using the formula;
(6)
Where, is the residual effect and is the product of direct effect of any variable and its correlation coefficient with yield.
-
-
RESULT AND DISCUSSION
-
Association of Traits
-
Genotypic and Phenotypic Correlation of Fruit Yield with Other Traits
The results of genotypic and phenotypic correlation coefficients is presented in (Table 1). The results showed that number of primary branches, fruit diameter, average fruit weight, number of tender fruit per plant, seed yield and frequency of harvest had positive and significant genotypic correlation with fruit yield per hectare at (P<0.01). Whereas, fruit diameter and weight of hundred seed had significant correlation at (P<0.05). However, all those traits had positive and significant phenotypic correlation with fruit yield per hectare at (P<0.01) level of significance. The presence of significant and positive correlation of number of primary branches, number of tender fruit per plant, seed yield, frequency of harvests, hundred seed weight and fruit diameter with fruit yield per hectare indicated that the importance of these traits in a selection program to identify the genotypes with high fruit yield. The signficant and positive correlation coefficients among the quantitative traits of okra indicate that the selection of one of genotypes for the correlated traits may increase the value of the other traits while; the negative correlations between traits showed in selection of genotypes for one trait may result the selection of genotypes for the lower mean value of the other negatively correlated traits.
The genotypic correlation coefficents were higher in magnitude than the corresponding phenotypic correlations coefficient in growth traits and yield components that had positive and significant genotypic correlation with fruit yield. This is in agreement with other authors reports that estimates of genotypic correlation coefficients were in most cases higher than their corresponding phenotypic correlation coefficients among agronomic traits of okra genotypes [30, 31, 32, 33 & 18]. [18] reported that fruit yield ha-1 had positive and highly significant (P<0.01) genotypic correlation with days to pod formation, number of branches per plant and number of tender fruit per plant in 25 okra genotypes evaluated at Werrer. [16] also reported positive and significant genotypic corrleations of fruit yield with average fruit weight, fruit diameter, seed yields, hundred seed weight and a number of tender fruit per plant. [34] also reported that genotypic correlation between fruit yield and seed yield was positive and highly significant (P<0.01). Positive and significant correlation of fruit yield with hundred seed weight and number of total fruits per plant was reported by [35]. [32] reported positive and significant correlation of fruit yield per plant with the number of branches per plant, number of fruits per plant, and fruit length.
Correlated characters are of prime importance for breeders because of genetic causes of correlations through pleiotropic action, linkage or developmental interactions of genes and changes brought about by a natural or artificial selection [36, 37]. The presence of strong correlation of traits enables to perform indirect selection for a quantitative trait, usually hard to be selected by another directly correlated trait of higher genetic gain or easy visual selection. Besides, it is also able to access how a trait can interfere on another.
The genotypic and phenotypic correlation coefficient between different trait pairs being similar in sign and nature indicates the corresponding of heritable factors to phenotypic expression [30, 31, & 32]. Phenotypic correlation measures the extent to which the two observed characters are linearly related while genotypic correlation measures the extent to which degree the same genes or closely linked genes cause co-variation or simultaneous variations in two different characters [28, 36, 37 & 31]. The genotypic correlation between two traits determines the genetic breeding as it involves an association of heritable nature whereas, the phenotypic value is lessened by the significant interaction of environment [38]. They also suggested that considerable number of growth traits and yield components had positive and significant genotypic and phenotypic correlations
with tender fruit yield indicating the correspondence of genetic factors and phenotypic expressions and the strong association between those characters genetically.
-
Genotypic and Phenotypic Correlations among other Characters
-
It was observed positive and significant genotypic and phenotypic associations among phenology (days to 50% emergence, days to first flower, days to 50% flowering, days to 90% maturity and number of harvest) of okra genotypes except days to 50% emergence and number of harvest had positive nonsignificant genotypic and phenotypic correlation. Days to first flower and days to 50% flowering with number of primary branches and peduncle length showed positive and significant genotypic and phenotypic correlations, and days to first flowering and days to 50% flowering with number of primary branches showed positive and significant correlation at genotypic and phenotypic levels.
In agreement with 39], that phenological traits (days to emergence, days to first flowering, days to50% flowering and days to maturity) showed significant correlation among them both at genotypic and phenotypic levels except the genotypic correlation between days to emergence and days to maturity was positive but non-significant. She also reported the genotypic correlation of phenological traits with growth traits of okra was non-significant except the genotypic correlation of days to emergence with number of internodes per plant and days to maturity with stem diameter showed negative and positive significant genotypic association, respectively.
Crop phenological traits (days to 50% emergence, days to first flowering, days to 50% flowering and maturity) had nonsignificant correlation with growth traits (plant height, stem diameter, number of inter node and inter node length) at genotypic level. However, primary branches had positive and significant correlation with first flowering, days to 50% flowering and maturity both at genotypic and phenotypic level. Days to first flowering with number of tender fruits per plant and seed yield per hectare had positive significant correlation at both genotypic and phenotypic level. Positive and significant genotypic and phenotypic correlation were observed between days to 50% flowering and number of tender fruits per plant. Days to 50% flowering with seed yield per hectare and days to 90% maturity with number of tender fruits per plant showed positive and significant correlation at phenotypic level.
Positive and significant association among phenological traits of okra was reported by [16]. They also stated that days to 50% flowering and days to maturity with average fruit weight, fruit diameter and seed per pod had negative correlations at genotypic and phenotypic levels. [17] also observed positive and significant correlations of days to pod maturity with days to 50% flowering and number of branches. [40] also reported that days to first flowering with days to 50% emergence and number of branches had positive and signficant correlation in okra genotypes.
All phenology parameters of studied okra genotypes had negative and significant genotypic and phenotypic correlation coefficients with fruit length (Table 1). The negative correlation of these phenological traits with fruit length suggested that selection for earliness has negative effect on fruit length. Negative and significant correlations of traits with one another both at phenotypic and genotypic levels will make difficult the simultaneous selection and improvement of traits [41]. [17] observed negative and significant correlation between days to pod maturity and fruit length.
The presence of strong genotypic and phenotypic correlation among phenology parameters of okra genotypes suggested that the selection of genotypes based on one phenology traits resulted in the selection of the genotypes for the other four traits. The positive and significant correlations between most of phenology parameters with growth traits indicated that as the crop had delayed flowering and maturity, did the higher mean values of genotypes for growth traits. However, only one or two phenology parameters showed positive and significant correlations with only two yield components (number of tender fruits per plant and seed yield per hectare) suggested that the late or early flowering and maturity had negligible effects on yield components. Besides fruit length with all the phenology parameters had negative and significant correlation coefficients suggested simultaneous selection of genotypes for phenology parameters and fruit length was hardly possible since selection for one vary the other trait in opposite direction.
Stem diameter had positive and significant correlation with pant height and number of internode both at genotypic and phenotypic level. Plant height also had positive and significant correlation with number of internode and internod length both at genotypic and phenotypic level. Number of primary branches had positive and significant correlation with number of internode both at genotypic and phenotypic level. However, number of primary branch had positive and nonsignificant correlation with stem diameter and plant height both at genotypic and phenotypic level.
Similar result was reported by [39] Plant height with stem diameter showed positive and significant association at phenotypic level. Number of branches and number of internodes per plant had positive and significant correlations with plant height; stem diameter at genotypic and phenotypic levels. Number of branches with number of internodes per plant at both levels had positive and significant correlation coefficient. [38] reported the presence of positive and significant phenotypic correlation among plant height, number of branches per plant and internodes length.
Stem diameter and plant height with fruit diameter, number of primary branches and number of internode with number of tender fruit per plant and seed yield per hectare, and internode length with average fruit weight and number of fruit ridges showed positive and significant genotypic and phenotypic correlations. Fruit length and diameter with average fruit weight, number of fruit ridges and number of seeds per pod, and average fruit weight with number of fruit ridges and number of seeds per pod showed positive and significant correlation at genotypic and phenotypic levels. Number of tender fruits per plant with seed yield per hectare and pod mucilage content while number of fruit ridges and number of seeds per pod had strong genotypic and phenotypic correlation. The genotypic correlation between hundred seed weight and seed yield per hectare was positive and significant.
The genotypic and phenotypic correlations within and between growth traits and yield components suggested that selection of genotypes for robust plant growth had a positive effect to increase the mean performance of genotypes for yield components. Increasing internode number might increase the number of tender fruit per pod this is because a pod of okra is usually borne in the leaf axil at nodes of the stem [24]. Increase in primary branches results in increases of the number of tender fruits per plant which is principal component of fruit yield. [1] reported that selection of genotype with more primary branches leads to correlated selection for more number of fruits per plant. [16, 14] reported fruit length was positive and highly correlated to a number of seed per pod.
[41, 33 & 17], reported that plant height had positive and significant phenotypic and genotypic correlation with stem diameter, number of branches per plant, number of internodes per plant, fruit diameter, number of fruit per plant and number of seeds per pod. [38] reported growth traits had positive and significant phenotypic correlation with number of fruits per plant, fruit length and fruit weight.The present study showed that negative and significant correlations were observed between number of primary branches with fruit length, number of inter node with fruit length and fruit ridge, internode length with fruit mucilage content, peduncle length with number of tender fruit per plant, fruit yield per hectare, seed yield per hectare, number of harvest and mucilage content at phenotypic level. Negative and significant correlations of traits with one another make difficult the simultaneous selection and improve traits [41].
Average fruit weight with hundred seed weight, and hundred seed weight with seed yield per hectare and pod mucilage content had positive and significant phenotypic correlations. Fruit mucilage content showed negative and significant genotypic and phenotypic correlations with fruit diameter, average fruit weight and number of fruit ridge, and it had also negative and significant phenotypic correlations with fruit length and fruit dry matter content. The genotypic and phenotypic correlation between number of tender fruits per plant and number of seed per pod was negative and significant and the genotypic correlation between number of tender fruit per plant and number of fruit ridges was negative and significant. The simultaneous selection of genotypes for those growth traits and yield components showed either positive phenotypic correlations alone or negative significant correlation at phenotypic and/or genotypic level may be hardly possible since traits had not strong genetically correlations. Seed yield is correlated to number tender fruit per plant, fruit length, number of seed per pod, fruit yield and hundred seed weight [33, 24, 1 & 17].
It was noted that traits that exhibited significant genotypic correlation coefficients showed also significant phenotypic correlation. Moreover, the higher significant correlation magnitude were observed for genotypic level than the cross ponding phenotypic correlation level which indicated traits were genetically controlled over environments. Therefore, traits that are positive and significantly correlated to each other at the genotypic level had to be directly advanced to selection progress whereas those shows low correlation at phenotypic level and none correlated traits suggested independence association that would possible to select independently in question for diverse directions. This is in agreement with other authors reports that estimates of genotypic correlation coefficients were in most cases higher than their corresponding phenotypic correlation coefficients among agronomic traits of okra genotypes [42, 33]. [41] reported that negative and significant correlations both at phenotypic and genotypic correlation with one another will be difficult to select for characterization of desirable traits to those with the negative association. However, nonsignificant correlation will be disregarded in selection for crop improvement
Table 1.
Genotypic
(above dia
gonal) and
phenotypic
(below di
agonal) cor
relation c
oefficients
among 22
quantitativ
e traits
Variable
Emer
Dflo
Flo
Mat
SD
PH
PB
NI
IL
PL
FL
Emer
0.555**
0.543**
0.458**
-0.007ns
0.176ns
0.226ns
0.192ns
-0.083ns
0.103ns
-0.3378*
Dflo
0.489**
0.994**
0.890**
0.211ns
0.179ns
0.431**
0.261ns
-0.080ns
0.333*
-0.454**
Flo
0.474**
0.989**
0.894**
0.224ns
0.199ns
0.403*
0.268ns
-0.073ns
0.337*
-0.444**
Mat
0.392**
0.852**
0.852**
0.294ns
0.219ns
0.381*
0.261ns
-0.063ns
0.317ns
-0.430**
SD
-0.012ns
0.190ns
0.199ns
0.281ns
0.447**
0.136ns
0.503**
-0.025ns
-0.205ns
0.067ns
PH
0.156ns
0.184ns
0.204ns
0.210ns
0.428**
0.163ns
0.484**
0.578**
-0.022ns
0.079ns
PB
0.199ns
0.402**
0.369**
0.367**
0.131ns
0.160ns
0.395**
-0.082ns
-0.196ns
-0.337**
NI
0.201ns
0.249*
0.255*
0.251*
0.469**
0.466**
0.372**
-0.220ns
-0.242ns
-0.307ns
IL
-0.076ns
-0.057ns
-0.054ns
-0.052ns
-0.020ns
0.561**
-0.083ns
-0.221ns
0.255ns
0.292ns
PL
0.064ns
0.307**
0.315**
0.312**
-0.166ns
-0.012ns
-0.191ns
-0.220ns
0.231ns
-0.016ns
FL
-0.298*
-0.412**
-0.405**
-0.424**
0.066ns
0.085
-0.322**
-0.303**
0.277*
-0.014ns
FD
0.183ns
0.156ns
0.178ns
0.214ns
0.452**
0.431**
0.035ns
0.199ns
0.275*
0.037ns
0.200ns
AFW
-0.112ns
-0.218ns
-0.212ns
-0.172ns
0.285*
0.274*
-0.161ns
-0.119ns
0.347**
0.089ns
0.828ns
NTFPP
0.195ns
0.367**
0.331**
0.285*
0.007ns
0.005ns
0.837**
0.397**
-0.241*
-0.317**
-0.489**
NR
-0.215ns
-0.253*
-0.247*
-0.270*
0.101ns
0.034ns
-0.152ns
-0.358*
0.241*
0.029ns
0.515**
NSPP
-0.134ns
-0.165ns
-0.176ns
-0.204ns
0.086ns
-0.004ns
-0.107ns
-0.099ns
0.102ns
-0.004ns
0.446**
SDW
0.134ns
-0.111ns
-0.131ns
-0.095ns
0.040ns
0.047ns
0.159ns
-0.008ns
0.147ns
0.015ns
0.116ns
Syph
0.204ns
0.299*
0.260*
0.216ns
0.095ns
0.016ns
0.832**
0.372**
-0.183ns
-0.314**
-0.264*
Fha
0.220ns
0.453**
0.417**
0.369**
0.169ns
0.152ns
0.757**
0.503**
-0.135ns
-0.246*
-0.453**
DMC
-0.128ns
0.179ns
0.191ns
0.247*
0.137ns
0.045ns
-0.064ns
0.089ns
0.006ns
0.500**
-0.063ns
PMC
0.175ns
0.085ns
0.072ns
0.035ns
-0.017ns
-0.048ns
0.224ns
0.229ns
-0.252*
-0.240*
-0.299*
Yhaton
0.123ns
0.189ns
0.151ns
0.113ns
0.207ns
0.147ns
0.797**
0.208ns
0.012ns
-0.232
0.108ns
ns, *and **, nonsignificant, significant at P<0.05 and P<0.01, respectively. Eme= Days to 50% emergence, Dflo= Days to first flower, Flo= Days to 50% flowering, Mat= Days to 90% maturity, SD=Stem diameter (mm), PH= Plant height (cm), PB= Number of primary branch, NI= Number of internode, IL= Internode length (cm), PL= Peduncle length (cm), FL= Fruit length (cm), FD= Fruit diameter (mm), AFW= Average fruit weight (g), NTFPP= Number of tender fruit per plant, NR= Number of fruit ridge, NSPP= Number of seed per pod, SDW= Hundred seed weight, Syph= Seed yield per hectare (Kg), Fha= frequency of harvest, DMC= Dry matter content (%), PMC= Mucilage content (%)Yhaton= Pod yield per hectare (tons).
Table 1. Continued
Variable
FD
AFW
NTFPP
NR
NSPP
SDW
Syph
Fha
DMC
PMC
Yhaton
Emer
0.209ns
-0.131ns
0.226ns
-0.257ns
-0.173ns
0.185ns
0.228ns
0.292ns
-0.121ns
0.182ns
0.149ns
Dflo
0.167ns
-0.238ns
0.405*
-0.300ns
-0.218ns
-0.121ns
0.333*
0.501**
0.218ns
0.085ns
0.201ns
Flo
0.184ns
-0.229ns
0.377*
-0.296ns
-0.219ns
-0.124ns
0.305ns
0.470**
0.242ns
0.073ns
0.172ns
Mat
0.217ns
-0.170ns
0.324ns
-0.309ns
-0.244ns
-0.124ns
0.241ns
0.420*
0.262ns
0.033ns
0.128ns
SD
0.473**
0.302ns
0.017ns
0.128ns
0.116ns
0.020ns
0.115ns
0.214ns
0.144ns
-0.021ns
0.228ns
PH
0.441**
0.280ns
-0.004ns
0.044ns
-0.002ns
0.069ns
0.015ns
0.174ns
0.058ns
-0.049ns
0.156ns
PB
0.037ns
-0.171ns
0.891**
-0.257ns
-0.124ns
0.168ns
0.877**
0.797**
-0.064ns
0.225ns
0.800**
NI
0.202ns
-0.123ns
0.442**
-0.436**
-0.110ns
0.036ns
0.432**
0.586**
0.111ns
0.238ns
0.243ns
IL
0.290ns
0.364*
-0.280ns
0.338*
0.118ns
0.152ns
-0.223ns
-0.179ns
0.007ns
-0.256ns
0.014ns
PL
0.033ns
0.093ns
-0.312ns
-0.009ns
-0.010ns
0.039ns
-0.310ns
-0.242ns
0.552**
-0.244ns
-0.242ns
FL
0.205ns
0.831**
-0.543**
0.637**
0.490**
0.146ns
-0.291ns
-0.507**
-0.073ns
-0.308ns
0.104ns
FD
0.601**
-0.179ns
0.469**
0.340**
0.254ns
0.081ns
0.130ns
0.044ns
-0.354**
0.329*
AFW
0.599**
-0.473**
0.676**
0.600**
0.287ns
-0.109ns
-0.325ns
0.086ns
-0.353*
0.322ns
NTFPP
-0.177ns
-0.433**
-0.513**
-0.360*
0.029ns
0.845**
0.837**
-0.107ns
0.364*
0.610**
NR
0.363**
0.554**
-0.391**
0.646**
0.152ns
-0.201ns
-0.443**
-0.001ns
-0.480**
0.119ns
NSPP
0.310**
0.549**
-0.334**
0.500**
0.264ns
0.130ns
-0.221ns
0.153ns
-0.156ns
0.212ns
SDW
0.201ns
0.240*
-0.014ns
0.128ns
0.271*
0.339*
0.165ns
0.180ns
0.031ns
0.373*
Syph
0.062ns
-0.101ns
0.834**
-0.123ns
0.153ns
0.328**
0.820**
0.024ns
0.307ns
0.820**
Fha
0.110ns
-0.291*
0.791**
-0.309**
-0.207ns
0.182ns
0.782**
-0.002ns
0.335ns
0.646**
DMC
0.037ns
0.083ns
-0.085ns
0.006ns
0.145ns
0.125ns
0.025ns
-0.011ns
-0.254ns
-0.010ns
PMC
-0.339**
-0.347**
0.348**
-0.367**
-0.125ns
0.028ns
0.300*
0.302**
-0.251*
0.131ns
Yhaton
0.302**
0.311**
0.599**
0.175ns
0.186ns
0.311**
0.789**
0.636**
-0.004ns
0.131ns
ns, *and **, nonsignificant, significant at P<0.05 and P<0.01, respectively. Eme= Days to 50% emergence, Dflo= Days to first flower, Flo= Days to 50% flowering, Mat= Days to 90% maturity, SD=Stem diameter (mm), PH= Plant height (cm), PB= Number of primary branch, NI= Number of internode, IL= Internode length (cm), PL= Peduncle length (cm), FL= Fruit length (cm), FD= Fruit diameter (mm), AFW= Average fruit weight (g), NTFPP= Number of tender fruit per plant, NR= Number of
fruit ridge, NSPP= Number of seed per pod, SDW= Hundred seed weight, Syph= Seed yield per hectare (Kg), Fha= frequency of harvest, DMC= Dry matter content (%), PMC= Mucilage content (%), Yhaton= Pod yield per hectare (tons).
-
Path Coefficient Analysis
-
Genotypic Path Analysis
In this study, th genotypic and phenotypic path analyses conducted that pod yield as dependent variable and the other traits as independent variables. The results of genotypic path analysis area presented in (Table 2). This study result revealed that seed yield per plant/or ha-1, number of primary branches, number (frequency) of harvest, number of tender fruit per plant had a positive and highly significant genotypic correlation with fruit yield of okra plant. Weight of hundred seed and fruit diameter had a positive and significant genotypic correlation with okra pod yield and had also had a direct effect on pod yield of okra plant.
The mere estimation of correlation coefficients does not give an idea about the real contribution of an independent character to the yield. Path coefficient is a tool, which provides an effective measure of the direct and indirect cause of association and depicts the importance of each factor involved in contributing towards yield [43, 34]. The correlation coefficients were classified into indirect and direct effects utilizing the path coefficient analysis as suggested by [29]. In order to obtain such developmental relations, the cause and effect relationship between pod yield and yield components were studied in okra through path coefficient analysis, the results of which are discussed below.
According to the rate set by [44], the direct and indirect effects into negligible (0.00-0.09), low (0.10-0.19), moderate (0.20- 0.29), high (0.30-1.00) and very high (>1.00). Based on these rates the genotypic path analysis resulted that number of primary branches (0.706) and seed yield per plant (0.621) had a positive high direct effect on okra fruit yield. But a number of tender fruit per plant (-0.445) had a negative high direct effect on okra fruit yield and the rest traits, fruit diameter (0.177), hundred seed weight (0.025), and number of harvests (-0.08) shows positive low and negligible and negative negligible direct effect on okra fruit yield, respectively.
Number of a primary branch and seed yield per plant or/ha-1 had revealed a highly significant correlation with fruit yield per hectare and they had also high positive direct effect on fruit yield but the number of primary branches had negative high indirect effect on fruit yield via a number of tender fruit per plant. Therefore, it is important to consider this trait directly while we are selecting for improvement programs.
Number of tender fruit per plant shows positive and highly significant genotypic correlation with pod yield as well as a negative and high direct effect on pod yield of okra. Even though it had a negative direct effect on pod yield; it had a high positive indirect effect on pod yield via a number of primary branches per plant and seed yield per hectare. Selecting this character were possible indirectly via a number of branch and seed yield per hectare. Frequency of harvest had a positive and highly significant genotypic correlation with pod yield and negative and negligible direct effect on pod yield at genotypic level but this trait had a positive and high indirect effect on pod yield via number primary of branches and seed yield per hectare.
Almost similar results reported by [45] number of plant branch, number of tender fruit per plant, seed yield was a high positive direct effect on pod yield and even other with low and negligible direct effect had moderate and positive high indirect effects. He also summarizes that traits with a positive direct effect on fruit yield per plant favors yield improvement through selection and indirect selection based on plant height will be effective in yield improvement. [46] reported that number of tender fruit per plant, number of the fruit harvest, hundred seed weight and seed per pod had a direct positive effect on pod yield. Number of pods per plant had the greatest direct influence on pod yield, followed by fresh weight per pod, which had a positive genotypic association with pod yield. Since it had positive and high indirect effect on pod yield and high association at genotypic level; considering this trait indirectly during selection will be better. But direct selection of this trait might be not effective [24].
Table 2. Genotypic path analysis of 36 okra genotypes of six quantitative data those had significant correlation with pod yield.
Traits
PB
FD
NTFPP
SDW
Syph
Fha
rg
PB
0.706
0.006
-0.397
0.004
0.544
-0.064
0.800**
FD
0.026
0.177
0.080
0.006
0.050
-0.010
0.329*
NTFPP
0.629
-0.032
-0.445
0.001
0.525
-0.067
0.610**
SDW
0.119
0.045
-0.013
0.025
0.210
-0.013
0.373*
Syph
0.619
0.014
-0.376
0.009
0.621
-0.066
0.820**
Fha
0.563
0.023
-0.373
0.004
0.509
-0.080
0.646**
Residual
0.182
PB= Nunmer of primary branches, FD= fruit diameter, NTFPP= Number of tender fruit per pod, SDW= hundred seed weight, Syph= Seed yield per hectare, Fha= Frequency of harvest and rg= Genotypic correlation .
-
Phenotypic Path Analysis
The current study result showed a positive and highly significant correlation of all traits at phenotypic correlation levels as shown in (Table 3). Eventhough, it shows positive and highly significant phenotypic correlation with fruit yield, it is mandatory to understand their direct and indirect effect as well as their implications on fruit yield. According to partitioning range the
phenotypic path coefficient analysis resulted for average fruit weight (0.526) and number of primary branches (0.498) had high and positive direct effect on okra fruit yield. Whereas, seed yield per plant/or hectare (0.241) and number (frequency) of harvest (0.195) had positive moderate direct effect on fruit yield. Eventhough, all these traits show highly significant phenotypic correlation with fruit yield; fruit diameter (-0.059) negative, a number of tender fruit per plant (0.044) and hundred seed weight (0.004) had a positive negligible direct effect on fruit yield. Therefore, looking at the indirect effect of those traits could be effective. Among traits number of tender fruit per plant had a positive and high indirect effect on fruit yield via a number of primary branches, moderate indirect effect via seed yield per hectare and frequency of harvest.
Similar result had been reported by [33] fruit weight and total number of fruits per plant had a direct strong influence on fruit yield per plant and are the main determiners of fruit yield per plant. [47] reported that seed yield per plant and number of primary branches per plant had a maximum direct effect on fruit yield per plant. Average fruit weight had positive and high direct effects on fruit yield, indicating their importance as reliable selection criteria for the improvement of yield in okra [48, 49].
Table 3. Phenotypic path analysis result of seven quantitative traits and had significant correlation with pod yield 36 okra genotypes
Traits
PB
FD
AFW
NTFPP
SDW
syph
Fha
rp
PB
0.498
-0.002
-0.085
0.037
0.001
0.200
0.147
0.797**
FD
0.017
-0.059
0.315
-0.008
0.001
0.015
0.021
0.302**
AFW
-0.080
-0.035
0.526
-0.019
0.001
-0.024
-0.057
0.311**
NTFPP
0.417
0.010
-0.228
0.044
0.000
0.201
0.154
0.599**
SDW
0.079
-0.012
0.126
-0.001
0.004
0.079
0.035
0.311**
syph
0.414
-0.004
-0.053
0.037
0.001
0.241
0.152
0.789**
Fha
0.377
-0.007
-0.153
0.035
0.001
0.188
0.195
0.636**
Residual
0.116
PB= Nunmer of primary branches, FD= fruit diameter, AFW= average fruit weight, NTFPP= Number of tender fruit per pod, SDW= hundred seed weight, Syph= Seed yield per hectare, and Fha= Frequency of harvest, rp= Phenotypic correlation.
-
-
-
SUMMARY AND CONCLUSION
The study result confirmed that association of quantitative traits at genotypic and phenotypic correlation coefficient between fruit yield and related traits were positive and significantly correlated with number of primary branches, number of tender fruit per plant, seed yield, and frequency of harvests both at genotypic and phenotypic correlations whereas average fruit weight was only correlated at phenotypic levels. Hundred seed weight and fruit diameter are positive and significantly correlated to fruit yield at (P<0.01) phenotypic correlation and significantly correlated at (P<0.05) at genotypic correlations. All traits with positive and significant genotypic correlation levels were higher in magnitude than the corresponding phenotypic correlations coefficient.
Number of primary branches had positive and highly direct effect on fruit yield at both genotypic and phenotypic path whereas, seed yield per plant/or ha-1 and average fruit weight had positive and significantly high direct effect at genotypic and phenotypic level, respectively. Number of tender fruit per plant and frequency of harvest had high negative and negative negligible direct effect genotypically in respective sequence. However, they had positive and high indirect effects via number of branches and seed yield per hectare. Consequently, this traits should be considered indirectly during selection besides frequency of harvest and fruit diameter had negative and negligible direct effect on pod yield genotypically and phenotypically, respectively. Even though they are negligeble they had contributed for yield via other traits. Therefore, all those traits listed above had significant importance during selection for pod yield improvements.
ACKNOWLEDGEMENTS
First, I would like to thank the almighty God for giving me the strength and substance to complete this work; as well as for giving me opportunity to express my heartfelt gratitude to people whose moral support and kind cooperation encouraged me during the study like my brother Adem Temam for direct encouragements and financial support and many thanks to Dajane Dida and Ayelech Woldatsadik staff member of biodiversity for laboratory work mainly mucilage extraction. My appreciation goes to Melkasa agricultural research center for their provision of land as well as other necessary input and materials during field work, Ethiopian Biodiversity Institute for access to experimental material and financial support.
-
REFERENCE
-
Kisher D S, Arya K, Yogeesh K J, Vinod K Y and Hee-Jong K (2016) Genotypic variation among okra (Abelmoschus esculentus (L.) Moench) germplasms in South Indian Plant Breeding and Biotechnology, 4(2): 234241.
-
Oyelade, O.J., Ade-Omowaye, B.I.O. and Adeomi, V.F. 2003. Influence of variety on protein, fat contents and some physical characteristics of okra seeds. Journal of Food Engineering, 57: 111-114.
-
Santos B M, Dittmar P J, Olson S M, Webb S E, Zhang S (2012) Okra production in Florida. University of Florida IFAS Extension. ; 163-171.
-
Muhammad R S, Muhammad A, Khurram Z, Muhammad M J, Saeed A, Qumer I and Aamir N (2013) Growth, yield and seed production of okra as influenced by different growth regulators. Pakistan Journal of Agricultural Science, 50(3): 387392.
-
Osawaru M E, Ogwu M C, and Omologbe J (2014) Characterization of three Okra [Ablemoschus esculentus (L.)] accessions using morphology and SDS-PAGE for the basis of conservation. Egyptian Academic Journal of Biological Sciences H. Botany, 5(1): 55 65.
-
Jideani V A, and Bello B M (2009) Functional properties of okra protein products containing different levels of mucilage. Journal of Food, Agriculture & Environment, 7(2): 252255.
-
Lamont W J (1999) Okra-A versatile vegetable crop. Horticultural Technology, 9: 179184.
-
Saifullah M and Rabbani M G (2009) Evaluation and characterization of Okra (Abelmoschus esculentus L. Moench.) cultivars. SAARC Journal of Agriculture, 7(1): 9299.
-
Haruna S, Aliyu B S, and Bala A (2016) Plant gum exudates (Karau) and mucilages, their biological sources, properties, uses and potential applications: A review. Bayero Journal of Pure and Applied Sciences, 9(2): 159165.
-
Akanbi W B, Togun A O, Adediran J A, and Ilupeju E A O (2010) Growth, dry matter and fruit yield components of okra under organic and inorganic sources of nutrients. American-Eurasian Journal of Sustainable Agriculture, 4(1): 113.
-
Daniela F O, Alicia M, Alicia R C, Rodolfo H and Sonia Z V (2012) Quality attributes of okra (Abelmoschus esculentus (L.) Moench) pods as affected by cultivar and fruit size. Journal of Food Research, 1(4), 224235.
-
Moekchantuk T and Kumar P (2004) Export okra production in Thailand. Inter-country programme for vegetable IPM in South and SE Asia phase II Food and Agriculture Organization of the United Nations, Bangkok, Thailand, 56.
-
Mihretu Yonas, Weyessa Garedew and Adugna Debela (2014a) Multivariate Analysis among okra [Abelmoschus esculentus (L.)Moench] collection in South Western Ethiopia. Journal of Biological science, 9(2): 43-50.
-
Tesfa Binalfew and Yosef Alemu (2016) Characterization of Okra (Abelmoschus esculentus (L.) Moench) Germplasms Collected from Western Ethiopia. International Journal of Research in Agriculture and Forestry, 3(2):11-17.
-
MoANR (Ministry of Agriculture and Natural Resources) (2016) Crop variety registers Issue No. 19, p.211. Addis Abeba, Ethiopia.
-
Mihretu Yonas, Weyessa Garedew and Adugna Debela (2014b) Variability and association of quantitative characters among okra [Abelmoschus esculentus (L.)Moench] collection in South Western Ethiopia. Journal of Biological science, 14: 336-342.
-
Muluken Demelie, Wassu Mohammed, and Endale Gebre (2015) Genetic Diversity of Ethiopian Okra Collections through Multivariate Analysis at Werer, Rift Valley of Ethiopia:,The International Journal Of Science and Technolodge, 3(8): 186193.
-
Muluken Demelie, Wassu Mohammed and Endale Gebre (2016) Variability, Heritability and Genetic Advance in Ethiopian Okra [Abelmoschus esculentus (L.) Monech] Collections for Tender Fruit Yeld and Other Agro-morphological Traits, Journal of Applied Life Sciences International, 4(1): 112.
-
Wassu Mohammed, Anteneh Bekele, and Kumar V (2017) Characterization and Evaluation of Okra [Abelmoschus esculentus (L.) Moench] Collections in Eastern Ethiopia. Edited and Compiled by, p.211- 238. Proceedings of 34th Annual review workshop, 6-8 April 2017. Haramaya University, Ethiopia.
-
Olaoye G, Bello O B, Olayiwola L S and Abubakar A Y (2009) Analyses of moisture deficit grain yield loss in drought tolerant maize (Zea mays L.) germplasm accessions and its relationship with field performance. African Journal of Biotechnology, 8(14): 32293238.
-
AdeOluwa O O. and Kehinde O B (2011) Genetic variability studies in West African Okra (Abelmoschus caillei). Agriculture and Biological Journal of North America, 2(10): 13261335.
-
Olawuyi O J, Bello O B, Ntube C V, and Akanmu A O (2015) Progress from selection of some maize cultivars response to drought in the derived savanna of Nigeria. Agrivita, 37(1): 817.
-
Adekoya M A, Ariyo O J, Kehinde O B, Adegbite A E (2014) Correlation and path analyses of seed yield in okra (Abelmoschus esculentus (L.) Moench) grown under different cropping seasons. Pertanika Journal of Tropical Agricultural and Science, 37(1): 3949.
-
Aminu D, Bello O B, Gambo B A, Azeez A H, Agbolade O J, Iliyasu A and Abdulhamid U A, (2016) Varietal performance and correlation of okra pod yield and yield components. Acta Universitatis Sapientiae, Agriculture, and Environment, 8(1), pp.112-125.
-
Melkassa Agricultural Research Center (2019) Home page datas. http://www.eiar.gov.et/marc
-
IPGRI (1991) Okra Descriptor list. International Crop Network Series 5. International Board for Plant Genetic Resources (IBPGR), Rome, Italy.
-
Robinson H F (1966) Quantitative genetics in relation to breeding on the centennial of Mendelism. Indian Journal of Genetics., 26: 171-187.
-
Singh R K and Chaudhary (1977) Biometrical methods in quantitative genetic analysis. Kalyani publishers, New Delhi-Ludhiana, India.
-
Dewey D R and Lu K H (1959) A correlation and path coefficient analysis of components of crested wheatgrass seed production. Agronomy Journal of Horticulture, 57(4): 342346.
-
Bello D, Sajo A, Chubado D and Jellason J J (2006) Variability and correlation studies in okra (Abelmoschus esculentus (L.) Moench). Journal of Sustainable Development in Agriculture and Environment, 2(1): 120-126.
-
Mehta D R, Dhaduk L K and Patel K D (2006) Genetic variability, correlation and path analysis studies in okra (Abelmoschus esculentus (L.) Moench). Agricultural Science Digest, 26(1): 15-18.
-
Rashwan A M A (2011) Study of genotypic and phenotypic correlation for some agro-economic traits in okra (Abelmoschus esculentus (L.) Moench). Asian Journal of Crop Science, 3(2): 85-91.
-
Reddy M T, Babu K H, Ganesh M, Begum H, Reddy R S K and Babu J D (2013) Exploitation of hybrid vigor for yield and its components in okra [Abelmoschus esculentus (L.) Moench]. American Journal of Agricultural Science and Technology, 1: 117. [49] Adeniji O T, Kehinde O B, Ajala M O, Adebisi M A (2007) Genetic studies on seed yield of West African okra (Abelmoschus caillei) (A chev.) Stevels). Journal of Tropical Agriculture, 45(12): 3641.
-
Simon S Y, Gashua I B, and Musa I (2013) Genetic variability and Trait Correlation Studies in Okra (Abelmoschus esculentus (L.) Moench). Agriculture and Biology Journal of North America, 4(5): 532-538.
-
Oppong-Sekyere D, Akromah,R, Nyamah E Y, Brenya E and Yeboah S (2012) Evaluation of some okra (Abelmoschus spp L.) germplasm in Ghana. African Journal of Plant Science, 6(5), pp.166-178.
-
Falconer D S, and Mackay T F C (1996) An Introduction to quantitative genetics. Ed, 4.Printice Hall London. 1996; 464.
-
Sharma J R (1998) Statistical and Biometrical Techniques in Plant Breeding. New Age International (P) Limited Publishers, New Delhi. Pp 432.
-
Kumar S and Reddy M T (2016) Correlation and path coefficient analysis for yield and its components in okra (Abelmoschus esculentus (L.) Moench). Advances in Agricultural Science, 4(1), pp.72-83. [41] Akinyele B O, Oseikita O S (2006) Correlation and path coefficient analyses of seed yield attribute in okra (Abelmoschus esculentus (L.) Moench). African Journal of Biotechnology, 5: 1330-1336.
-
Fozia Yimam (2018) Genetic diversity and association of seed yield and related traits of Okra [Abelmoschus esculentus (L.) Moench] in Ethiopia. MSc thesis, Haramaya University, Haramaya, Ethiopia.
-
Samim S., Sonia S., Akhilesh S., Anuradha V. and Amandeep K. 2018. Morphological Characterization of Okra [Abelmoschus esculentus (L.) Moench]. International Journal of Current Microbiology and Applied Sciences ISSN: 2319-7706 Volume 7(10). Department of Vegetable Science and Floriculture CSK Himachal Pradesh Krishi Vishvavidyalaya, Palampur (HP)-176 062, India.
-
Akinyele B O, Oseikita O S (2006) Correlation and path coefficient analyses of seed yield attribute in okra (Abelmoschus esculentus (L.) Moench). African Journal of Biotechnology, 5: 1330-1336.
-
Somashekhar G, Mohankumar H D and Salimath P M (2011) Genetic analysis of association studies in segregating population of okra. Karnataka Journal of Agricultural Science. 24(4), 432-435.
-
Patel M, Ganga M, Jawaharlal M and Jeyakumar P (2017) Assessment of Qualitative, Quantitative and Visual Flower Quality Parameters of Certain Commercial Jasmine Varieties during Peak Flowering Season. Int. J. Curr. Microbiol. App. Sci, 6(11), pp.3246-3251.
-
Lenka D and Mishra B (1973) Path coefficient analysis of yield in rice varieties. Indian Journal of Agricultural Sciences, 43: 376-379. [47] Kaul T G and Peter K V (1978) Correlation and path coefficient analysis of components of earliness, pod yield and seed yield in okra. Indian Journal of Agricultural Science, 48: 459-463.
-
Rambabu B, Waskar D P and Khandare V S (2019) Correlation and Path Coefficient Analysis of Fruits Yield and Yield Attributes in Okra [Abelmoschus esculentus (L.) Moench]. International Journal of Current Microbiology and Applied Science. 8(04): 764-774.
-
Prasath G, Reddy K R, and Pidigam Saidaiah (2017) Correlation and Path Coefficient Analysis of Fruits Yield and Yield Attributes in Okra (Abelmoschus esculentus (L.) Moench). International Journal of Current Microbiology and Applied Sciences ISSN: 2319-7706 Volume 6 (3): 463- 472
-
Kaul T G and Peter K V (1978) Correlation and path coefficient analysis of components of earliness, pod yield and seed yield in okra. Indian Journal of Agricultural Science, 48: 459-463.
-
Subrata S, Hazra P and Chattopadhyay A (2004) Genetic variability, correlation and path analysis in okra (Abelmoschus esculentus L. Moench). Horticulture Journal, 17(1): 59-66.
-
Adeniji O T, Kehinde O B, Ajala M O, Adebisi M A (2007) Genetic studies on seed yield of West African okra (Abelmoschus caillei) (A chev.) Stevels). Journal of Tropical Agriculture, 45(12): 3641.