
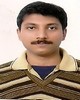
- Open Access
- Authors : Prashant Nigam , Dr. Nidhi Singh , Lillie Dewan
- Paper ID : IJERTV11IS110113
- Volume & Issue : Volume 11, Issue 11 (November 2022)
- Published (First Online): 25-11-2022
- ISSN (Online) : 2278-0181
- Publisher Name : IJERT
- License:
This work is licensed under a Creative Commons Attribution 4.0 International License
Controller Design Techniques for Nonlinear Systems- A Review
Prashant Nigam1, Nidhi Singp,Lillie Dewan3
*1 Research Scholar, Electrical Engineering Department, Gautam Buddha University, Greater Noida, U.P. India.
*2Assistant Professor, Electrical Engineering Department, Gautam Buddha University, Greater Noida, U.P. India.
*3Professor, Electrical Engineering Department, National Institute of Technology, Kurukshetra, Haryana, India.
Abstract:- Nonlinear systems being a vital part of real-world applications have earned measureless attention these days. Control of these systems is a grave concern owing to insufficient and proper control schemes. This paper sheds some light on control schemes and elucidates their significance in controlling nonlinear systems, and describes some general nonlinear control approaches employed in the literature. It reviews the different controllers such as robust controllers, intelligent, adaptive controllers, and hybrid controllers designed for handling diverse classes of nonlinear systems. It reveals the chief technical hurdles involved with the control of nonlinear systems for promoting extensive and more profound research to develop better controllers for managing future nonlinear systems.
Keywords:-Nonlinear systems, Adaptive controllers, Controller designs, Intelligent control, Robust Control
-
INTRODUCTION
Real-world or practical applications are instinctively nonlinear when considered over a broad operating range. Nonlinear differential equations govern nonlinear models representing various physical phenomena. Examples include electrostatic and gravitational attraction, drag on a moving vehicle, current-voltage characteristics of several electronic systems, to name a few. Nonlinear systems use linear control theory for meeting the diverse design demands in several nonlinear systems. Recently, several researchers from different realms like biomedical engineering, process control, spacecraft control, aircraft control, and robotics have shown a vast attraction towards the analysis and development of nonlinear control techniques [1-3]. Some characteristics of nonlinear dynamic systems include:
-
These systems do not obey the superposition principle (homogeneity and linearity).
-
Nonlinear systems exhibit properties like a limit cycle, chaos, and bifurcation.
-
They may possess multiple separated equilibrium points.
-
Solutions may not be present at all times.
The fundamental reasons behind rising attention towards nonlinear control include analysis of strong nonlinearities, improvement of control systems, design simplicity, and necessity to handle several model uncertainties. Coulomb friction, hysteresis, saturation, backslash, and dead zone are the hard nonlinearities which do not allow linear estimation of practical- world systems. Nonlinear approaches appropriately compensate for these by predicting the nonlinearities for achieving unmatched performance [4]. Practical systems typically exhibit ambiguities in the model parameters predominantly owing to slow or sudden variations in the parameter values. Existing control strategies are not adequate and robust enough for dealing with such nonlinearities in real-world mechanisms. Therefore, it is essential to devise controllers for handling complex ambiguities in real-world nonlinear systems. The utilization of adaptive, intelligent, and robust nonlinear controllers can aid in handling the consequences owing to model ambiguities [5].
Moreover, these controllers can support systems which are not linearly observable or controllable but are observable/controllable in a nonlinear way. This paper intends to explore such controllers for achieving effective control of practical nonlinear systems. The notable contributions of this review include:
-
To study standard nonlinear control methods.
-
To investigate different controllers employed in nonlinear systems for different applications.
-
To explore the significance of adaptive control in nonlinear systems.
-
To compile the various controller techniques applied on the specific applications.
-
The rest of the paper is organized as follows: Following a short introduction in Section I, popularly employed nonlinear control approaches are mentioned in section II. Section III reviews the combination of controllers popularly known as hybrid controllers, followed by a conclusionin Section IV and references.
-
-
COMMON NONLINEAR CONTROL APPROACHES
The nonlinear system is complex and difficult to manage; it becomes unstable due to the nonlinear system's unique characteristics, such as limit cycle, bifurcation, jump resonance etc. Therefore, special control techniques have to be applied to get the desired response as the techniques applied on the linear system fail when applied directly to nonlinear systems. The standard nonlinear control approaches include Feedback Linearization Control (FLC), Adaptive Controllers (AC), Robust Controllers (RC), Sliding Mode Control (SMC),Back-stepping Control (BSC), Intelligent controller (IC).
-
Feedback Linearization Control
Feedback Linearization Control (FLC) is a well-known method for stabilizing nonlinear systems. By modifying variables and applying the proper control input, nonlinear systems may be transformed into linear systems. FLC attempts to eliminate the nonlinear characteristics of a nonlinear system by using linear control methods [6-7]. The primary drawback of FLC is that it removes critical nonlinear features during the design phase of the control. Though it does not ensure a robust performance against uncertainties, it can alleviate the consequences of uncertainties to a considerable level. FLCs have a variety of applications, including converters [8], microgrids [9], and maglev trains [10].
Despite the remarkable advancements in control systems, the application of PID (Proportional Integrative Derivative) controllers has gained immense significance in industrial applications because of its simplicity and efficacy in controlling the behavior of linear systems [11]. PID controllers also have advantages such as cost-effectiveness, ease of installation, and simple operation. Despite the advantages, certain limitations restrict the application of PID controllers in real-time control of nonlinear systems. Linearity is the major limitation and due to which the performance of a PID controller in nonlinear systems is variable. To overcome these problems in the PID controller, fractional order PID(FOPID) controller has been introduced. FOPID outperforms the conventional PID controller as it gives better stability to a nonlinear system and improved disturbance rejection[12]. It provides robustness to the variation in the gain of the plant and high-frequency noise and eliminates steady- state error [13].
-
Adaptive Controllers
Adaptive control is a feedback control system capable of adjusting its characteristics in a changing environment to satisfy some specified criteria.The fundamental idea of Adaptive controllers is estimating uncertain parameters and onlinetuning of controllers for adapting dynamic conditions wherein variations in environment and system parameters are significant. Application of Adaptive controllers include robotics [14], small-scale autonomous vehicles [15], magnetic levitation [16], hypersonic vehicles [17], power systems [18] etc. Model-Reference Adaptive Control (MRAC) and Self-Tuning Control (STC) are the two primary kinds of adaptive control methods. MRAC, adjusts controllr parameters directly, whereas, in STC, they are adjusted indirectly through parameter estimation and design. The Adaptive controllers may utilize dynamic model-based or model-free controllers for estimating uncertain parameters. Tuning dynamic model-based Adaptive controllers is cumbersome, leading to communication and computation issues, thus making controllers implementation challenging. Hence, dynamic model-free Adaptive controllers are the right choice for handling nonlinear systems.
In [14], a piecewise invariant adaptive approach was used to resolve nonlinear ambiguities that may be either unmatchable or matchable. The proposed controller has been capable of dealing with distinct uncertainties and showed good control performance. [19] discusses an intelligent adaptive controller for ambiguous nonlinear systems. The controller's hidden node variables were modified through an extreme learning machine (ELM) technique. In this adaptive controller, the output weight has been updated using norm penalty for ensuring entire system stability. The proposed adaptive controller displayed fine tracking performance, control performance, robustness and anti-interference potential for nonlinear structures.In [20], a decentralized adaptive controller has been described for widespread nonlinear structures with unknown interconnections and unfamiliar nonaffine subsystems. It guaranteed the closed loop system stability using Lyapunov theory of stability analysis. Convergence of tracing error to origin, robustness against disturbances and closed loop system stability were the chief merits of this controller. A direct adaptive controller has been presented in [21] for handling nonlinear systems. No previous knowledge of the control direction or the system's nonlinearities were needed for the adaptive controller design to be effective. To account for unpredictability in control directions, a Nussbaum-type function was used, and the system's stability was determined using the Lyapunov method. When coupled with a closed-loop system, the suggested adaptive controllermaintained stability while showing practical and effective performance. The proposed adaptive controller guaranteed closed loop system stability and displayed feasible and effective performance. In [22], an adaptive controller based on output-feedback tracking scheme has been proposed for ambiguous nonlinear mechanisms whose input has been quantized using a freshly-proposed quantizer. The proposed adaptive controller guaranteed global boundedness of signals and allowed tracking error to converge towards an adjustable compact set.
-
Robust Controllers
Robust control (RC) is an approach for controller design that explicitly deals with uncertainty. It seeks to bound the uncertainty rather than express it in the form of distribution. The goal of robust design is to retain the assurance of system performance despite model inaccuracies and changes.RCs are designed to handle significant ambiguities bounded in size through either some pre-defined time and state functions or some constant,controlling bounded uncertainties by assuming known bounding functions. Application of robust technique is important to build dependable embedded systems. It allows exploration of the design space for alternatives that are insensitive to changes in the system and able to maintain their stability and performance. RCs have attracted considerable attention owing to their robustness and are vastly used in various applications [23-25], e.g., industrial robotics,aerospace,process industry,automotive control, etc.Sliding mode control is a relatively recent development in the field of robust control, which will be explored in more depth in the next section.
-
Sliding Mode Control
Sliding mode control (SMC) is another nonlinear control method that is becoming more prominent. By providing a discontinuous control signal, SMCs alter the dynamics of a nonlinear system, forcing the system to slide over a cross-section of
the systems normal behaviour [26]. The design of an SMC controller is split into two phases: the first phase involves creating a sliding surface that satisfies the design requirements, and the second phase involves selecting a control law that makes the switching surface appealing to the system state [27]. The first step focuses on developing a sliding surface that adheres to the design requirements. It has robust performance against external disturbances and uncertainties [28]. In SMC, the use of discontinuous signals causes chattering, resulting in decreased system performance, higher energy consumption, actuator degradation owing to increased mechanical wear, and system instability. On the other hand, this approach has the primary benefit of changing the dynamic system's behaviour by selecting an appropriate switching function that makes the closed-loop response insensitive to the system's matched uncertainty [29-30]. [31] summarizes extensive experimental findings on applications of DC motor control using second-order sliding modes. Apart from that, SMC has been used in a variety of fields, including industrial process control (IPC), motion control (MC), robotics (RC), power electronics (PE), and aerospace applications [32-34].
-
Back-stepping Control
Another standard control method utilized particularly in controlling high order systems is Back-stepping Control (BSC). This scheme provides a recursive technique for stabilizing the system origin in strict-feedback form. The design procedure could be started at the familiar-stable system, and new controllers which stabilize every outer subsystem could be backed out. The process is terminated on reaching the ultimate external control. BSC ensures improvement of transient and tracking performance and global stability. The prime limitations of classical BSC include steady-state error, the requirement of a larger extent of the control mechanism, and knowledge of all system states. Apart from constraints, BSC offers better uncertainty handling capacity, asymptotic convergence, and guaranteed stability at each design step [35]. BSC has been employed for various practical systems ac motors [36], missiles [37], energy sectors [38], drug injection [39], and drones [40].
-
Intelligent controller
Intelligent controllersare most capable of dealing with nonlinear systems even in the presence of disturbances. The term "intelligent controller" refers to a set of control methods that make use of a range of artificial intelligence computer methodologies, including fuzzy logic, neural networks, machine learning, reinforcement learning, evolutionary computation and consequently named after them.
Fuzzy logic controllers (FLC) are logic controllers that are based on fuzzy sets which are classes of objects having a smooth transition from membership to non-membership. Fuzzy logic is utilized to regulate the plant's operation, as a consequence of its design including a very flexible set of IF-THEN rules. This method is extensively used in a wide variety of nonlinear applications, such asmachines,air conditioners, heating systems, traffic control, medical [41]and many more.
A neural network (NN) is a kind of machine learning model in computer science that is intended to mimic the organization of the human brain. NNs are built using a network of mathematical equations as their building component. In each subsequent step, networks of neurons (NNs) are utilized to predict the system output based on the system's previous output and input data. A considerable amount of training time is required for NN controllers. However, it is capable of rationally and efficiently dealing with uncertainties. NN controllers are utilized in a variety of applications, including flight vehicles [3], robotics [42], industrial machines [42], and motor drives [43].
Evolutionary algorithms are optimization methods that get their inspiration from biological processes and are named after that like elephant hazard approach, grey olf approach, jelly fish, ant colony, honey bee approach etc. Higher-level procedures or heuristics are used to find or choose the most suitable heuristic (partial search algorithm) for solving an optimization issue. They provide a variety of benefits, including methods for directing search operations ranging from local to sophisticated learning processes. Because it is not confined to a particular problem, the number of possible applications is almost limitless [44].
Machine learning (ML) is a subset of artificial intelligence that makes use of statistical techniques to enable computers to learn and improve over time. In the case of machines, the experience of a system, represented as data, may be utilized to learn, make choices, and make predictions. Machine learning allows a computer to operate and make data-driven choices without being expressly taught to do so. Machine learning applications include, but are not limited to, nonlinear magnetic bearing systems [45], voice recognition, predictive analysis, data extraction, medical diagnosis, to name a few.
Reinforcement learning is a subfield of machine learning that focuses on training models to make segmented judgements in response to input data. Through the activity, an agent interacts with its environment and therefore learns about mistakes and rewards [46]. Reinforcement learning is a branch of machine learning that encompasses supervised and unsupervised learning, among other things. However, models are taught fundamentally differently using reinforcement learning than they are using other machine learning techniques. The primary benefit is that it can learn highly complicated behaviour without requiring labelled training data and can make immediate choices while optimizing for a long-term objective.
Because PID controllers are intended to govern linear systems, the majority of their techniques are based on an empirically proven linear process model for the nominal operating state. As a result, the stability of a typical PID controller is significantly reduced, and it becomes unstable when employed with a nonlinear application running under a wide variety of operating circumstances. The most commonly used nonlinear system control techniques are the FLC, SMC,Adaptive, Robust, and Intelligent nonlinear system control approach. Numerous optimization techniques have also been employed to enhance the performance of nonlinear systems, like particle swarm optimization (PSO), genetic algorithms (GA), and ant colony optimization (ACO) to name a few. These techniques have been utilized to optimize controller settings to enhance nonlinear
system performance [47-50]. In practice, however, many Controllers fall short of expectations when the right performance varies significantly and utilization increases, thus leading to trade-offs and form hybrid controllers.
-
-
HYBRID CONTROLLERS FOR NONLINEAR SYSTEMS
To increase the efficiency of nonlinear controllers and to achieve optimum performance in a variety of applications, hybrid controllers are modeled from the standard approaches described in the previous section.
-
Hybrid Robust Controllers
This section discusses the use of robust controllers in combination with other nonlinear system controllers.
-
SMC based RC
In [25] a finite-time limited RC design based on SMC for a broad class of nonlinear conic-kind systems had been discussed. Within the time constraints imposed on this assignment, the state of a particular sliding region, as well as its dynamic trajectories, were generated using a scalar criterion-based SMC law. It has been feasible to build a delay-based condition that guarantees the boundedness of SMC within the permitted finite-time period using the slack matrix technique. The proposed RC addressed the conic type's time delays and ambiguity, among other problems.In [51], the artificial nonlinear robust controller design is proposed to design a high-performance nonlinear controller and focus on eliminating limitations associated with the sliding mode controller and applied to the robot manipulator. System stability has been achieved using the proposed robust controller.A novel method for regulating shape memory alloy (SMA) actuators using neural networks and a sliding-based robust controller, is described in [52]. Two controllers are implemented for this: one to minimize or eliminate hysteresis, and another to adjust for uncertainties and maintain system stability.
-
Fuzzy based RC
According to [53], a RC was developed utilizing Takagi-Sugeno (T-S) fuzzification and other methods for a kind of uncertain nonlinear time-delay system. Fuzzy rules were used to decompose a large nonlinear system into a set of smaller time-delay systems with unknown nonlinear functions. According to the control matrix utilized to produce the input signals, the system has been split into two subsystems. Through the employment of a virtual control method, it was able to establish exponential stability in the first subsystem. It has proven feasible to build a memoryless state feedback controller by using the virtual control policy. In laboratory testing, the suggested RC demonstrated exponential stability and enhanced control performance. [24] proposes that nonlinear suspension systems be managed using an RC design. T-S fuzzy logic was used to produce the output for the controller. The system's stability was accomplished via use of a predictive control method. The suggested RC demonstrated a significant increase in closed-loop (CL) stability. [54] explored the application of an advanced hybrid control method to a pneumatically powered microrobot, resulting in findings demonstrating excellent trajectory tracking capabilities even in the face of external disturbances. [55] describes a novel controller for regulating the speed of a brushless DC motor. The suggested controller is proven to be successful in reducing torque ripple and producing a zero-overshoot response in the motor's speed response.
-
RL based RC
A reinforcement learning (RL)-based RC architecture was presented and shown to be successful for a class of perpetual-time input-constrained ambiguous nonlinear systems in [23]. The use of reinforcement learning technology resulted in the attainment of optimum control. The suggested RC ensured the ambiguous nonlinear system's stability in terms of uniform boundedness, which was a critical concern. The use of event trigger methods to solve resilient constrained control issues for nonlinear systems has been discussed in [56]. Stability has been achieved via the use of strong nonlinear system controllers. [57] proposes a zero- shot transfer method that may be used in many driving situations, including lane-keeping and lane shifting, as well as obstacle avoidance. It is suggested that the technique be utilized to ensure temporal and frequency domain stability and responsiveness, which will then be evaluated. [58] proposes a model-free solution to the robust stabilization issue. By solving the ARE (Algebraic Riccati Equation) and robustly stabilizing the uncertain system, it has been found that an optimum controller may be constructed.
-
-
Hybrid Adaptive Controllers
An Adaptive controller is united with several different techniques to model hybrid controllers for nonlinear systems in order to get the desired output.
3.2.1Adaptive-NN
In [2], a decentralized adaptive controller architecture for frequently used ambiguous nonlinear systems is proposed. It employed NN technology to approximate unknown functions, including the conditions of nonlinear interconnections and dynamic uncertainty in every subsystem. The proposed Adaptive Controller design comprise of a feedforward with feedback control. The measurable and known types of disturbances were responded rapidly using feedforward control while deviations from expected system behavior were handled using feedback control. In [59], an Adaptive Controller has been designed for nonlinear multiple-input-multiple output (MIMO) systems using neural networks. To improve the transient behaviour of unknown parameters, it was chosen to add filter-dependent modification in their update laws. The actuation faults, external disturbances and modeling uncertainties were combinedly accommodated with the proposed Adaptive control laws. This adaptive controller ensured robustness and stability for nonlinear mechanisms. An improved intelligent Adaptive Controller has been proposed for handling nonlinear systems in [60] to compensate for the system's nonlinearity, which were overlooked by the conventional adaptive controller. A decentralized Adaptive Controller design was proposed in [61]. An affine subsystem
adaptive controller was developed based on Lyapunov theory, and the closed-loop system's stability was ensured by incorporating a correctly directed adaptation rule in the closed-loop system's design. Additionally, a neuro-dependent structure for nonaffine subsystems was suggested, and system's stability analysis was done using Lyapunov theory. The unknown interactions were modeled using nonlinear functions. The proposed decentralized adaptive controller structure exhibited better control performance. A simplified adaptive controller has been constructed in [62] for controlling nonlinear switched systems. The Adaptive controller has been designed through combining the structural quality of NNs and the standard Lyapunov function method. The proposed hybrid controller shows better tracking control within finite-duration under random switching.
-
Adaptive-Fuzzy Controller
In [63] an adaptive fuzzy controller has been proposed for dealing with nonlinear systems. Rather than utilizing an unknown function, it approximated the unknown control signal using fuzzy logic. The consistent adaptive fuzzy control has been achieved using small-gain method and backstepping scheme. The proposed adaptive controller comprised just three parameters that were updated online and guaranteed a uniformly bounded closed loop system. [64] describes an observer-based adaptive fuzzy controller. No exact information regarding nonlinear system's ambiguities or differentiation of output has been required in controller design.Design of control term and inherent update law relied on proper filtering of tracking error thereby eliminating the need of filtering observation error.The proposed adaptive controller showed better nonlinearity control performance.[65] describes the construction of a fuzzy indirect adaptive controller.This article discusses a system whose parameters were repeatedly found using the least-squares approach and using theT-S fuzzy method. The parameters were used in combination with particle swarm optimization to estimate the goal function and enhance predictive control. The proposed adaptive controller design maintained a good consistency during disturbances and has been capable of handling extremely nonlinear systems.The authors of [66] suggested a fuzzy indirect adaptive controller for estimating unknown smooth nonlinear functions by using an observer-based tracking error method for identifying unavailable states. The suggested adaptive controller requires no previous knowledge of the system's nonlinearities or state estimates. This controller guaranteed closed-loop system stability. In [67] the unfamiliar nonlinearities and system uncertainties were estimated using the fuzzy logic notion. Online tuning of fuzzy logic parameters has achieved the system's real-time control. The error boundness and system consistency were proved through Lyapunov stability criterion.In [68], an observer-based fuzzy tracking method was proposed for ambiguous rigid-feedback nonlinear networks with uncertain gain control functions. The proposed controller was developed by combining backstepping and finite control design methods with Lyapunov logarithm functions and other mathematical methodologies. This controller guaranteed that the parameters of the closed-loop system were restricted and that tracking and observer errors converged to zero. [69] discusses the fuzzy adaptive controller for ambiguous multivariable systems. Two fuzzy adaptive controllers have been suggested, with and without time delays. The intrinsic control systems were determined to be stable, and the related tracking errors were found to be convergent to their source. In [70], a decentralized fuzzy adaptive control was suggested for handling widespread nonlinear systems wherein system interactions and functions were unknown.Lyapunov theory demonstrated that the resulting adaptation rules ensured both closed-loop system stability and tracking error convergence to the point of origin. Besides, this controller displayed robustness against approximation errors and external perturbations.[71] describes a new adaptive fuzzy dynamic surface control method which when applied to closed-loop unknown nonlinear output- delay systems, ensures that all signals are uniformly bounded.
-
Adaptive Backstepping approach
In [20] an adaptive backstepping approach is discussed. It adopted an integral barrier lyapunov functions for realizing direct constraints on state values. The proposed adaptive controller illustrated that all signals were bounded and desired control objectives were satisfied.Another intelligent adaptive control design has been proposed for nonlinear stochastic switched systems in [21]. In the controller design procedure, not just the backstepping scheme and global intelligent approximation technique were applied successfully to the intelligent control of nonlinear systems but even the design issues resulting from non-lower triangular and sheer-feedback structures were resolved using scaling methods. The proposed adaptive control method ensured that all closed-loop system signals were bounded. [22] presents a new adaptive control technique for a kind of sheer- feedback nonlinear system. Through utilizing the backstepping method, adaptive law and adaptive controller were designed.According to the Lyapunov analysis, no outputs were violated and all closed-loop signals were bounded.
-
Adaptive-Dynamic Surface Control method
An adaptive controlhas been proposed in [59] for resolving the output tracking issue of nonlinear systems. Both input saturation and asymmetric output bounds were considered. A high-gain observer has been making the best use for relaxing the demand of Lipschitz continuity regarding nonlinear dynamics. The dynamic surface control (DSC) method has been employed to filter every subsystem's control signal. The theoretical investigation confirmed that the tracking error and parameter estimation were uniformly bounded. Intelligent adaptive control for ambiguous nonlinear systems has been described in [60]. The DSC has been employed for strictfeedback while SMC has been used for controlling the canonical system. For swift convergence, a finite-time design has been introduced. The closed-loop system stability has been realized using the Lyapunov technique. The developed controller guaranteed the boundedness of closed-loop signals. Moreover, it showed superior tracking control with stronger robustness and greater precision in case of flight dynamics and standard nonlinear systems. [61] discusses the challenge of developing robust adaptive control for nonlinear systems with unknown time-varying characteristics and disturbances. The
suggested control method ensures the system's overall stability and allows the tracking error to converge to a specified minimal residual value in an acceptable amount of time.
-
Adaptive- Backstepping with NN's
The authors in [62], proposed an intelligent adaptive control for a general category of ambiguous higher-order nonlinear structures with fully unknown nonlinearities. The employed control algorithm combined the backstepping technique with NN's approximation ability. The tracking error between the output and time-dependent target signal has been minimized through tuning the proposed adaptive controller design parameters. The adaptive controller provided effective tracking control performance in higher-order nonlinear structures. A class of nonlinear networks with unobservable states and unmodeled dynamics is addressed in [63], which offers an adaptive controller design for these networks. System states were estimated using an observer.An adaptive controller by combining the backstepping method with neural networks capacity was designed to approximate global functions. Lyapunov's theory has been used for analyzing the closed loop system stability. The proposed adaptive controller ensured rapid convergence and boundedness of signals.
-
Adaptive- SMC
In [64], the pitch angle of a wind turbine is regulated using an intelligent adaptive controller based on SMC. SMC coefficients for pitch angle control were computed using a support vector machine (SVM)-PSO technique. The proposed adaptive controller reacted to disturbances more gently and steadily than the traditional adaptive controller. [65] proposed an adaptive global SMC technique to enhance the transient and steady-state performance of ambiguous nonlinear systems. A tuning strategy has been created to eliminate the repercussions of redundant disturbances and ensure the states convergence to the switching region. This controller not just ensured robustness against disturbances and nonlinearities but also prevented the chattering problem.[66] suggested an intelligent adaptive controller based on SMC and fuzzy logic for controlling nonlinear systems. Additionally, the system has been stabilized using Lyapunov-derived generic stability criteria. The proposed adaptive controller is capable of effectively and rapidly controlling nonlinear multivariable networks. [67] discusses an optimal hybrid adaptive robust sliding mode control framework for the stabilization of a ball and wheel system. The controller was created by combining a feedback linearization with a PD controller, and the resulting model is referred to collectively as the ARSMC-PD controller. The PD controller has been added to overcome the ineffectiveness of the adaptive SMC and feedback li nearization in
reducing the tracking error. In [68], an Htracking-dependent adaptive fuzzy SMC has been designed for handling nonlinear
mechanism involving external disturbances and system uncertainties. The proposed adaptive controller inhibited the chattering
issues and ensured Htracking performance for attenuating the lumped ambiguities produced by external perturbations,
approximation error and unmodeled dynamics.
-
Adaptive-STR with SVM
In [69] describes an adaptive control system based on a Self-Tuning Regulator (STR). It provides enhanced transient response, no output oscillations, with minimum settling time[88]. It is simple to implement and efficient in terms of computing. The suggested method is used in a nonlinear system of the third order. [70] described the generalized self-tuning regulator for nonlinear systems based on online support vector regression. The proposed scheme results show that the generalized self-tuning regulator and online support vector regression attain good control and modeling performances.In [71], using real-time RLS algorithm as a parameter estimator, a pure hardware implementation of self-tuning regulators has been presented. The design has been verified with real time experiments. The proposed scheme shows stability and achieves higher performance[89].
-
Adaptive-Fuzzy and Backstepping approach
In [72] an adaptive control scheme using a fuzzy and backstepping method has been discussed. The tracking error has been reduced by using adaptive control and fuzzy logic to learn the unknown system dynamics. External perturbations and approximation mistakes were effectively compensated by the use of strong compensators. It was shown that tracking error was reduced by appropriately choosing controller parameters and that all variables in the closed-loop system were evenly confined. In [73], an intelligent adaptive controller for higher-level nonlinear systems with significant uncertainties, output constraint, and input saturation was proposed. A control method for ensuring the boundedness of all states in a closed-loop system has been developed using a power integrator, fuzzy control, and backstepping technique. [15] proposes an intelligent adaptive controller for monitoring the course of a small-scale autonomous helicopter using the concept of backstepping and fuzzy logic for controllerdesign. The weigh updation rules and adaptive laws were established for ensuring the closed-loop system's boundedness using Lyapunov theory-based stability analysis. [74] discusses a fuzzy control-based adaptive control scheme for no-triangular stochastic nonlinear switching systems. The Lyapunov function and backstepping technique were used to construct the controller which ensured the closed-loop system's stability and signal boundedness.
3.3Hybrid Intelligent Controllers
The neural network is combined with several methods such as SMC, PID, RBF, and FUZZY in this research to create a hybrid controller for nonlinear systems.
[3] presents a non-backstepping NN-based controller for supersonic flight dynamics. The lumped ambiguous nonlinearity was approximated using NN technology, and the tracing control was performed using a minimal-parameter-learning technique. The suggested neural network controller reduced the computational load associated with parameter updating and demonstrated acceptable control performance. In [75], an NN-based controller for full-state restricted nonlinear systems was proposed. The effect of full-state constraints has been addressed by introducing the barrier Lyapunov function in a backstepping process. It employed minimal learning variables in the backstepping design and ensured that all signals in the closed loop system wereuniformly bounded. [76] describes a controller structure based on neural networks. The control law and updated law of NN controller has been derived using Lyapunov theorem. Proposed NN controller has guaranteed the bounded of closed loop system and stability. In [77], ANN controllers were used for controlling nonlinear tank heater system. The ANN parameters were updated at each sampling time for providing the adaptability. The proposed ANN controller offered sufficient damping to subsystem interactions and eliminated step disturbances. The authors proposed a NN-based controller structure for nonlinear sheer-feedback switched systems in [78]. In the NN controller design course, the unfamiliar time-delay terms were compensated using desired stabilizing functions, Lyapunov-Krasovskii functions and tangible control input has been approximated using RBFNNs. The suggested NN controller guaranteed that all signals in the closed-loop system were evenly bounded on a semi- global scale. [79] proposed an NN-based control technique for analyzing and stabilizing nonlinear processes that integrated T-S
fuzzy and Hcontrol. Modeling error issues associated with nonlinear systems were addressed using the NN method. The
proposed NN-based controller ensured better system stability.
3.3.1NN-based RBF
[80] proposes an NN-based controller for a popular class of ambiguous nonlinear systems. The unexpected nonlinearities were estimated using radial basis function (RBF) neural networks. A supervisory control has been designed to attenuate the consequences of NN approximation errors and external perturbations for handling the approximation errors. The suggested neural network-based controller guaranteed a desired closed loop performance. [81] proposes a controller based on RBF neural networks to dynamically adjust the necessary parameters. The proposed NN controller performed effectively even when functional changes and system mode swings occurred. Compared to multiple and single fuzzy reference Adaptive controllers, the proposed NN controller provided superior tracking with minimized tracking eror in case of functional variations. Additionally, it offered swift and unpredictable system control. [82] proposes an NN-based controller for ambiguous nonlinear systems. The saturation nonlinearity effect has been compensated using NNs approximation. The proposed NN controller offered better stability and provided a clear bound on tracking error performance with regard to design parameters. In [83], the authors used RBFNNs to approximate ambiguous functions relating to system dynamics. The backstepping method was used to improve the overall precision of the control process. The suggested NN controller outperformed conventional controllers without NNs in terms of resilience and control performance. [43] discusses a neural network-based controller for a broad class of nonlinear systems with limited disturbances and unknown parameters. RBFNN was used in conjunction with an adaptive control method to solve problems presented by external disturbances and unknown functions. By selecting suitable settings, the suggested NN-based controller demonstrated acceptable performance on intended signal tracking and verified the closed-loop system's stability. It ensured the closed-loop system's uniform boundedness.3.3.2ANN-PID
In [42], a self-tuned proportional-integral-derivative (PID) controller based on an artificial neural network (ANN) was suggested for regulating the position of a synchronous motor. For the purpose of upgrading controller gains, an online training method based on iterative least-squares was used. The proposed ANN controller showed greater robustness against external disturbances and process parameter changes. Additionally, it had online learning capacity and demonstrated increased accuracy in the presence of uncertainty and disruption. [84] presents a hybrid method for overcoming the difficulties inherent in discrete nonlinear systems. The research used a Levenberg-Marquardt (LM) method in conjunction with a PID neural network equipped with relay feedback (LM-PIDNN-RF). While the relay feedback initializes the PIDNN by assigning initial weights, the LM is used to properly assign the input and output parameters. The PD controller's output is sent to the PIDNN, which changes the PIDNN's weights after each iteration. The suggested hybrid method is responsible for correctly updating the NN controller's settings in the presence of random disturbances. The Lyapunov stability theory was used to ensure the control system's stability. The findings indicate that the suggested hybrid method is more successful in improving the system's tracking capability than PIDNN RF-PID approaches.
3.3.3NN-based SMC
In [85] describes the use of a neural network-based controller for ambiguous nonlinear strict-feedback systems. SMC law has been designed for tracking the referenced output using backstepping method. The chattering produced from SMC has been reduced using RBFNNs. The proposed NN controller showed better tracking control and lowered the chattering efficiently. In [86], control of ambiguous nonlinear system has been accomplished using NN-based SMC. The switching and corresponding control terms of traditional SMC have been matched using a particular design ofadaptive NN with tangent activation procedures. The stability has been achieved using Lyapunov's theory of stability. The developed controller guaranteed uniform boundedness of closed loop signals and powerful robustness against nonlinearities and unknown dynamics. In [87], a NN-
dependent H SMC approach has been proposed for ambiguous nonlinear structures with unmatched disturbances and actuator
faults. The actuator fault effects were reduced through a discontinuous SMC and unmatched disturbances were attenuated using
Hnonlinear control. The Lyapunov method was used to achieve stability. The proposed control method ensures the closed-
loop system's stability and signal boundedness.
-
-
CONCLUSION
This paper thoroughly discussed the various controllers employed for dealing with diverse nonlinear systems. The general control techniques of nonlinear systems that are utilized majorly in existing studies like SMC, FLC, and BSC were described along with Robust controller, Adaptive controller and Intelligent controllers. The distinct controllers, such as robust controllers, adaptive controllers, and NN-based controllers, were comprehensively reviewed to control nonlinear systems varieties. The
reviewed controllers were compared based on control techniques utilized and applications. Comparison of control techniques reported that NNs were utilized in most of the explored works, indicating their superior ability to control nonlinear systems than other control schemes. The important research barriers involved in nonlinear system control were revealed. This extensive review identified the applications of diverse controller designs for dealing with different categories of nonlinearities. The survey elaborated the control behavior of existing controllers and their robustness to ambiguities while handling different nonlinear control mechanisms. This paper summarized that though sliding mode control (SMC) is robust against external disturbances and uncertainties but suffered from chattering problems causing deterioration in system performance, back-stepping control ensures global stability. However, it also suffered from limitations that include steady state error, robust and adaptive controllers are easily tunable, experienced communication and computation issues, and NN-based controllers displayed better performances. However, they suffered from high training time problems, indicating the desire for better and more intelligent controller designs by combining the advantageous attributes of robust, adaptive and NN-based controllers to satisfy the control demands of modern and future nonlinear systems.
REFERENCES
[1] Iqbal, J., Ullah, M., Khan, S. G., Khelifa, B., &ukovi, S. (2017). Nonlinear control systems-A brief overview of historical and recent advances.Nonlinear Engineering, 6(4), 301-312.
[2] Tan, K. K., Huang, S., & Lee, T. H. (2009). Decentralized adaptive controller design of large-scale uncertain robotic systems. Automatica, 45(1), 161-166. [3] Xu, B., Fan, Y., & Zhang, S. (2015). Minimal-learning-parameter technique based adaptive neural control of hypersonic flight dynamics without back-stepping. Neurocomputing, 164, 201-209. [4] Tee, K. P., Ren, B., & Ge, S. S. (2011). Control of nonlinear systems with time-varying output constraints. Automatica, 47(11), 2511-2516. [5] Bechlioulis, C. P., & Rovithakis, G. A. (2008). Robust adaptive control of feedback linearizable MIMO nonlinear systems with prescribed performance. IEEE Transactions on Automatic Control, 53(9), 2090-2099. [6] Yeildirek, A., & Lewis, F. L. (1995). Feedback linearization using neural networks. Automatica, 31(11), 16591664. [7] Kokotovi, P. V., Kanellakopoulos, I., & Morse, A. S. (n.d.),1995. Adaptive feedback linearization of nonlinear systems. Lecture Notes in Control and Information Sciences, 309346. [8] Callegaro, L., Ciobotaru, M., Pagano, D. J., & Fletcher, J. E. (2018). Feedback linearization control in photovoltaic module integrated converters.IEEE Transactions on Power Electronics, 34(7), 6876-6889.
[9] Zolfaghari, M., Abedi, M., &Gharehpetian, G. B. (2019). Robust nonlinear state feedback control of bidirectional interlink power converters in grid- connected hybrid microgrids. IEEE Systems Journal, 14(1), 1117-1124. [10] Leng, P., Li, Y., Zhou, D., Li, J., & Zhou, S. (2019). Decoupling control of maglev train based on feedback linearization. IEEE Access, 7, 130352- 130362. [11] Chen, J., & Huang, T. C. (2004). Applying neural networks to online updated PID controllers for nonlinear process control. Journal of process control, 14(2), 211-230. [12] Swati Sondhi, Yogesh V Hote(2012). Fractional Order Controller and its Applicatins: A Review. Proceedings of the IASTED Asian Conference on Modelling, Identification and Control, April 2-4, 2012, Phuket, Thailand. [13] VineetShekher, Dhiraj Kumar and Neel Kamal Saini (2011). Comprehensive Study of Fractional Controller. VSRD International Journal of Electrical, Electronics & Communication Engineering, 1(1), 30-35. [14] Zhang, A., Ma, S., Li, B., Wang, M., Guo, X., & Wang, Y. (2016). Adaptive controller design for underwater snake robot with unmatched uncertainties. Science China Information Sciences, 59(5), 1-15. [15] Tsai, C. C., Lee, C. T., & Hwang, K. S. (2011, October). Intelligent adaptive trajectory tracking control using fuzzy basis function networks for an autonomous small-scale helicopter. In 2011 IEEE International Conference on Systems, Man, and Cybernetics (pp. 2255-2260), 9-12 Oct. 2011, Anchorage, AK, USA, IEEE. [16] Lin, C. M., & Le, T. L. (2017). WCMAC-based control system design for nonlinear systems using PSO. Journal of Intelligent & Fuzzy Systems, 33(2), 807-81. [17] Li, H., Sun, Z., Min, H., & Deng, J. (2011). Fuzzy dynamic characteristic modeling and adaptive control of nonlinear systems and its application to hypersonic vehicles. Science China Information Sciences, 54(3), 460-468. [18] Jiang, N., Chen, X., Liu, T., Liu, B., & Jing, Y. (2011). Nonlinear steam valve adaptive controller design for the power systems. Intelligent Control and Automation, 2(1), 31-37. [19] Chen, L., Sun, J., & Xu, C. (2019). Regularized extreme learning machinebased intelligent adaptive control for uncertain nonlinear systems in networked control systems. Personal and Ubiquitous Computing, 23(3), 617-625. [20] Ghasemi, R., Abdi, B., &Mirtalaei, S. M. M. (2013). Advanced Intelligent Controller Design for Canonical Nonlinear Systems. International Journal of Computer and Electrical Engineering, 5(1), 22. [21] Boulkroune, A. (2016). A fuzzy adaptive control approach for nonlinear systems with unknown control gain sign. Neurocomputing, 179, 318-325. [22] Xing, L., Wen, C., Zhu, Y., Su, H., & Liu, Z. (2016). Output feedback control for uncertain nonlinear systems with input quantization. Automatica, 65, 191-202. [23] Liu, D., Yang, X., Wang, D., & Wei, Q. (2015). Reinforcement-learning-based robust controller design for continuous-time uncertain nonlinear systems subject to input constraints. IEEE transactions on cybernetics, 45(7), 1372-1385. [24] Bououden, S., Chadli, M., & Karimi, H. R. (2016). A robust predictive control design for nonlinear active suspension systems. Asian Journal of Control, 18(1), 122-132. [25] He, S., Song, J., & Liu, F. (2017). Robust finite-time bounded controller design of time-delay conic nonlinear systems using sliding mode control strategy. IEEE Transactions on Systems, Man, and Cybernetics: Systems, 48(11), 1863-1873. [26] Spurgeon, S. (2014). Sliding mode control: a tutorial. 2014 European Control Conference (ECC), June 24-27, Strasbourg, France,2272-2277. [27] V.I. Utkin, Variable Structure systems with Sliding Modes. IEEE Transaction on Automatic Control, 22, 2, 212-222, 1977. [28] Gambhire, S. J., Kishore, D. R., Londhe, P. S., & Pawar, S. N. (2021). Review of sliding mode-based control techniques for control system applications. International Journal of Dynamics and Control, 9, 363-378. [29] Elmali, H., &Olgac, N. (1992). Robust output tracking control of nonlinear MIMO systems via sliding mode technique. Automatica, 28(1), 145151. [30] Feng, Y., Han, F., & Yu, X. (2014). Chattering free full-order sliding-mode control. Automatica, 50(4), 13101314. [31] Damiano A., Gatto G.L., Marongiu I., PISANO A." Second-order sliding-mode control of DC drives"IEEE Trans. on Industrial Electronics, vol. 51, n. 2, pp. 364-373, 2004. [32] Islam, S., & Liu, X. P. (2010). Robust sliding mode control for robot manipulators. IEEE Transactions on Industrial Electronics, 58(6), 2444-2453. [33] Alsmadi, Y. M., Utkin, V., Haj-ahmed, M. A., & Xu, L. (2018). Sliding mode control of power converters: DC/DC converters. International Journal of Control, 91(11), 2472-2493. [34] Velasco, J., Calvo, I., Barambones, O., Venegas, P., &Napole, C. (2020). Experimental Validation of a Sliding Mode Control for a Stewart Platform Used in Aerospace Inspection Applications. Mathematics, 8(11), 2051. [35] Kim, W., Kang, C. M., Son, Y. S., & Chung, C. C. (2019). Nonlinear Backstepping Control Design for Coupled Nonlinear Systems under External Disturbances. Complexity, 2019, 1-13. [36] Lin, F. J., Chen, S. G., & Hsu, C. W. (2018). Intelligent backstepping control using recurrent feature selection fuzzy neural network for synchronous reluctance motor position servo drive system. IEEE Transactions on Fuzzy Systems, 27(3), 413-427. [37] Ye, Q., Liu, C., & Sun, J. (2018). A backstepping-based guidance law for an exoatmospheric missile with impact angle constraint. IEEE Transactions on Aerospace and Electronic Systems, 55(2), 547-561. [38] Yin, W., Wu, X., & Rui, X. (2018). Adaptive robust backstepping control of the speed regulating differential mechanism for wind turbines. IEEE Transactions on Sustainable Energy, 10(3), 1311-1318. [39] Butt, R. S., Ahmad, I., Iftikhar, R., & Arsalan, M. (2019). Integral backstepping and synergetic control for tracking of infected cells during early antiretroviral therapy. IEEE Access, 7, 69447-69455. [40] Ali, R., Peng, Y., Iqbal, M. T., Amin, R. U., Zahid, O., & Khan, O. I. (2019). Adaptive backstepping sliding mode control of coaxial octorotor unmanned aerial vehicle. IEEE Access, 7, 27526-27534. [41] Phuong, N. H., &Kreinovich, V. (2001). Fuzzy logic and its applications in medicine. International Journal of Medical Informatics, 62(2-3), 165 173. [42] Kumar, V., Gaur, P., & Mittal, A. P. (2014). ANN based self-tuned PID like adaptive controller design for high performance PMSM position control. Expert Systems with Applications, 41(17), 7995-8002. [43] Yang, H., & Liu, J. (2018). An adaptive RBF neural network control method for a class of nonlinear systems. IEEE/CAA Journal of AutomaticaSinica, 5(2), 457-462. [44] Mehmet Konar, Aytekin Bagis, Performance Comparison of Particle Swarm Optimization, Differential Evolution and Artificial Bee Colony Algorithms for Fuzzy Modelling of Nonlinear Systems, ELEKTRONIKA IR ELEKTROTECHNIKA, ISSN 1392-1215, 2016. [45] Hai-Liang Zhao, & Ming Qing. (n.d.). Method to obtain effective rule base for unknown nonlinear systems. Proceedings of the 2003 International Conference on Machine Learning and Cybernetics (IEEE Cat. No.03EX693), 5-5 Nov, Xi'an, China, pp-519-524. [46] Yang, X., Liu, D., Wang, D., & Wei, Q. (2014). Discrete-time online learning control for a class of unknown nonaffine nonlinear systems using reinforcement learning. Neural Networks, 55, 3041. [47] Gharghory, S. M., & Kamal, H. A. (2012). Optimal tuning of PID controller using adaptive hybrid particle swarm optimization algorithm.International Journal of Computers Communications & Control, 7(1), 101-114.
[48] Mohideen, K. A., Saravanakumar, G., Valarmathi, K., Devaraj, D., & Radhakrishnan, T. K. (2013). Real-coded Genetic Algorithm for system identification and tuning of a modified Model Reference Adaptive Controller for a hybrid tank system. Applied Mathematical Modelling, 37(6), 3829-3847. [49] Mohamed, B., Kara, K., Oussama, A., & Hadjili, L. (2019, June). Adaptive neural network PID controller. In 2019 IEEE International Conference on Environment and Electrical Engineering and 2019 IEEE Industrial and Commercial Power Systems Europe (EEEIC/I&CPS Europe), 11-14 June, Genova, Italy, (pp. 1-6). IEEE.
[50] Carlucho, I., De Paula, M., & Acosta, G. G. (2020). An adaptive deep reinforcement learning approach for MIMO PID control of mobile robots. ISA transactions, 102,280-294. [51] Farzin Piltan, N. Sulaiman, Z.Tajpaykar, P.Ferdosali & M.Rashid(2011), Design Artificial Nonlinear Robust Controller Based on CTLC and FSMC With Tunable Gain, International Journal of Robotics and Automation (IJRA), Volume (2) : Issue (3). pp. 195-210. [52] Song, G., Chaudhry, V., & Batur, C. (2003). Precision tracking control of shape memory alloy actuators using neural networks and a sliding-mode based robust controller. Smart Materials and Structures, 12(2), 223231. [53] Hua, C., Wang, Q. G., & Guan, X. (2008). Robust adaptive controller design for nonlinear time-delay systems via TS fuzzy approach. IEEE Transactions on Fuzzy Systems, 17(4), 901-910. [54] Y Sabzehmeidani, M. Mailah, M. Hussein, E.Gatavi, M.Z Md Zain (2010). A hybrid fuzzy-based robust controller for pneumatically actuated micro robot. International Review on Modeling and Simulations, Vol 3, N.6, pp. 1308-1316. [55] M. Zolfagharia, S. A. Tahe (2015), Fuzzy Approximation Model-based Robust Controller Design for Speed Control of BLDC Motor, IJE TRANSACTIONS C: Aspects Vol. 28, No. 3, (March 2015) 426-432. [56] Yang, D., Li, T., Zhang, H., & Xie, X. Event-trigger-based Robust Control for Nonlinear Constrained-input Systems Using Reinforcement Learning Method. Neurocomputing. © 2019 Elsevier, pp.159-170. [57] Xu, Z., Tang, C., & Tomizuka, M. (2018). Zero-shot Deep Reinforcement Learning Driving Policy Transfer for Autonomous Vehicles based on Robust Control. 2018 21st International Conference on Intelligent Transportation Systems (ITSC), 7 Dec 2018, Maui, HI, USA. [58] Yang, Y., Guo, Z., Xiong, H., Ding, D.-W., Yin, Y., & Wunsch, D. C. (2019). Data-Driven Robust Control of Discrete-Time Uncertain Linear Systems via Off-Policy Reinforcement Learning. IEEE Transactions on Neural Networks and Learning Systems, 113. [59] Gao, H., Song, Y., & Wen, C. (2016). Backstepping design of adaptive neural fault-tolerant control for MIMO nonlinear systems. IEEE transactions on neural networks and learning systems, 28(11), 2605-2613. [60] Prakash, R., & Anita, R. (2011). Design of Model Reference Adaptive Intelligent Controller using Neural Network for Nonlinear Systems.International Review of Automatic Control, 4(2), 153-161.
[61] Karimi, B., Menhaj, M. B., Karimi-Ghartemani, M., &Saboori, I. (2009). Decentralized adaptive control of large-scale affine and nonaffine nonlinear systems. IEEE Transactions on Instrumentation and Measurement, 58(8), 2459-2467. [62] Wang, F., Zhang, X., Chen, B., Lin, C., Li, X., & Zhang, J. (2017). Adaptive finite-time tracking control of switched nonlinear systems. Information Sciences, 421, 126-135. [63] Liu, Z., Wang, F., Zhang, Y., Chen, X., & Chen, C. P. (2013). Adaptive fuzzy output-feedback controller design for nonlinear systems via backstepping and small-gain approach. IEEE transactions on cybernetics, 44(10), 1714-1725. [64] Boulkroune, A., Tadjine, M., MSaad, M., &Farza, M. (2008). How to design a fuzzy adaptive controller based on observers for uncertain affine nonlinear systems. Fuzzy sets and systems, 159(8), 926-948. [65] Bououden, S., Chadli, M., Allouani, F., &Filali, S. (2013). A new approach for fuzzy predictive adaptive controller design using particle swarm optimization algorithm. International Journal of Innovative Computing, Information and Control, 9(9), 3741-3758. [66] Boulkroune, A., Bounar, N., &Farza, M. (2014). Indirect adaptive fuzzy control scheme based on observer for nonlinear systems: A novel SPR-filter approach. Neurocomputing, 135, 378-387. [67] Das, T., & Kar, I. N. (2006). Design and implementation of an adaptive fuzzy logic-based controller for wheeled mobile robots. IEEE Transactions on Control Systems Technology, 14(3), 501-510. [68] Tong, S., Min, X., & Li, Y. (2020). Observer-based adaptive fuzzy tracking control for strict-feedback nonlinear systems with unknown control gain functions. IEEE Transactions on Cybernetics, 50(9), 3903-3913. [69] Boulkroune, A., MSaad, M., & Chekireb, H. (2010). Design of a fuzzy adaptive controller for MIMO nonlinear time-delay systems with unknown actuator nonlinearities and unknown control direction. Information sciences, 180(24), 5041-5059. [70] Ghasemi, R., Menhaj, M. B., & Afshar, A. (2009). A new decentralized fuzzy model reference adaptive controller for a class of large-scale nonaffine nonlinear systems. European Journal of Control, 15(5), 534-544. [71] Zahra Rahmani,BarmakBaigzadehnoeb and BehroozRezaie (2020). Tracking control of a class of nonlinear systems with output delay based on adaptive fuzzy dynamic surface control. International Journal of Systems Science, pp- 1280-1306. [72] Hou, Z. G., Zou, A. M., Cheng, L., & Tan, M. (2009). Adaptive control of an electrically driven nonholonomic mobile robot via backstepping and fuzzy approach. IEEE Transactions on Control Systems Technology, 17(4), 803-815. [73] Sun, W., Su, S. F., Liu, Z. G., & Sun, Z. Y. (2019). Adaptive intelligent control for input and output constrained high-order uncertain nonlinear systems. IEEE Transactions on Systems, Man, and Cybernetics: Systems, 1-10. [74] Sun, K., Mou, S., Qiu, J., Wang, T., & Gao, H. (2018). Adaptive fuzzy control for nontriangular structural stochastic switched nonlinear systems with full state constraints. IEEE Transactions on Fuzzy Systems, 27(8), 1587-1601. [75] Liu, Y. J., Li, J., Tong, S., & Chen, C. P. (2016). Neural network control-based adaptive learning design for nonlinear systems with full-state constraints. IEEE transactions on neural networks and learning systems, 27(7), 1562-1571. [76] Zhao, X., Su, Q., Chen, S., & Tan, Y. (2021). Neural network adaptive control of nonlinear systems preceded by hysteresis. Journal of Intelligent Material Systems and Structures, 32(1), 104-112. [77] Ang, G. J. N., Huang, L., & Lim, C. M. (2018, November). Artificial Neural Network-Based Controllers for A Continuous Stirred Tank Heater Process. In 2018 15th International Conference on Control, Automation, Robotics and Vision (ICARCV) (pp. 1414-1419), 18-21 Nov. 2018,Singapore, IEEE. [78] Niu, B., Li, H., Qin, T., & Karimi, H. R. (2017). Adaptive NN dynamic surface controller design for nonlinear pure-feedback switched systems with time-delays and quantized input. IEEE Transactions on Systems, Man, and Cybernetics: Systems, 48(10), 1676-1688. [79] Chen, C. W. (2011). Stability analysis and robustness design of nonlinear systems: an NN-based approach. Applied Soft Computing, 11(2), 2735- 2742. [80] Yuan, R., Tan, X., Fan, G., & Yi, J. (2014). Robust adaptive neural network control for a class of uncertain nonlinear systems with actuator amplitude and rate saturations. Neurocomputing, 125, 72-80. [81] Kamalasadan, S., &Ghandakly, A. A. (2007). A neural network parallel adaptive controller for dynamic system control. IEEE Transactions on Instrumentation and Measurement, 56(5), 1786-1796. [82] Zhou, J., Er, M. J., & Zhou, Y. (2006, December). Adaptive neural network control of uncertain nonlinear systems in the presence of input saturation. In 2006 9th International Conference on Control, Automation, Robotics and Vision (pp. 1-5), 5-8 Dec, Singapore IEEE. [83] Tavoosi, J., & Mohammadi, F. (2019, October). Design a new intelligent control for a class of nonlinear systems. In 2019 6th International Conference on Control, Instrumentation and Automation (ICCIA) (pp. 1-5), 30-31 Oct, Sanandaj, Iran IEEE. [84] Hao, J., Zhang, G., Liu, W., Zheng, Y., & Ren, L. (2020). Data-Driven Tracking Control Based on LM and PID Neural Network with Relay Feedback for Discrete Nonlinear Systems. IEEE Transactions on Industrial Electronics, V(68), 11587 – 11597. [85] Yueneng, Y. A. N. G., & Ye, Y. A. N. (2018). Backstepping sliding mode control for uncertain strict-feedback nonlinear systems using neural- network-based adaptivegain scheduling. Journal of Systems Engineering and Electronics, 29(3), 580-586. [86] Debbache, G., & Golea, N. (2012). Neural network based adaptive sliding mode control of uncertain nonlinear systems. Journal of systems Engineering and Electronics, 23(1), 119-128. [87] Qu, Q., Zhang, H., Yu, R., & Liu, Y. (2018). Neural network-based H sliding mode control for nonlinear systems with actuator faults and unmatched disturbances.Neurocomputing, 275, 2009-2018. [88] Udai Singh and Nidhi Singh Pal, Roll Angle Control of an Aircraft using adaptive controllers, IEEE International Conference on Automation, Computational and Technology Management (ICACTM-2019), Amity University, London, 24-26 April,2019. [89] Udai Singh and Nidhi Singh Pal, Antenna Azimuth Position Control using Model Reference Adaptive Controller and Self Tuning Controller, ELSEVIER International Conference on Advances in Electronics, Electrical and Computational Intelligence (ICAEEC-2019), Indian Institute of Information Technology (IIIT) Allahabad, Prayagraj, India, 31st May-1st June, 2019.