
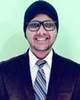
- Open Access
- Authors : Obaid Ahmad , Dr. Ranjana Vyas
- Paper ID : IJERTV10IS030311
- Volume & Issue : Volume 10, Issue 03 (March 2021)
- Published (First Online): 07-04-2021
- ISSN (Online) : 2278-0181
- Publisher Name : IJERT
- License:
This work is licensed under a Creative Commons Attribution 4.0 International License
Brief Check on the Feasibility of Prescriptive Analytics as A Subset of Supply Chain Management
Dr. Ranjana Vyas Under the guidance of DAAD Fellow (Germany)
Assistant Professor (Information Technology) Coordinator-New Gen IEDC, IIIT Allahabad,
Indian Institute of Information Technology, Allahabad (An Institute of national importance enacted by Parliament of India)
Obaid Ahmad
Author
Department of Management Studies
Indian Institute of Information Technology, Allahabad (An Institute of national importance enacted by Parliament of India)
-
Abstract:- This paper aims to dig into the most nascent field in the analytics phenomenon namely prescriptive analytics. As more and more businesses adopt a data-driven approach while decision making using descriptive or prescriptive analytics. Prescriptive analytics is one more step ahead of them by prescribing the course of action to take in order to tackle any business- related problem like procurement inventory, profit maximization, increasing operational efficiency and effectiveness, resource allocation and optimization. Analytics comes into play because things are getting complicated with every nick of a second passing by and we need a more and more sophisticated and data-driven approach because the supply chain isnt linear anymore it is becoming more of a network or wave with many entities joining in like more manufacturers, vendors and wholesalers. As a consequence of which we need to re-calibrate our decision-making processes. By means of this paper, we can have a further look at the role analytics play to tackle various business problems relating to the supply chain and try to find an optimum solution using analytics.
keywords – Prescriptive analytics, echelon inventory, data, risk reduction, educated guess
-
INTRODUCTION
At the beginning of the 20th century, the first revolutionary change in the field of the supply chain was seen when fort motors integrated the entire supply chain from mining iron ore to delivering finished cars in the span of mere 81 hours. At that time it was named as the most efficient supply chain. But there was a slight wrinkle in this process that the car should be of only black colour and T model only in order to fulfil the 81-hour promise. After the Second World War, the Japanese automobile giant Toyota came up with a supply chain model having multiple vendors with different expertise and skillset which successfully met consumers diversified demands. Toyota model filled the gap of inflexibility as the Ford model was highly rigid and absolutely inflexible. The latest revolution was an Information Technology-driven approach towards revolutionizing the supply chain was made by Dell computers. Earlier Dell used to take information from the customers and forward it to its various vendors to provide customized products to its customers. Dell leveraged the power of information technology for better customer satisfaction. Things took a turn when Dell realized that customer needs are not much different in fact most of the general customers have the same requirements. From 2006 onwards Dell changed its supply chain model and its products are also available in retail stores while also keeping its old customization option. Lead time also affected Dells decision as earlier for the sake of uniqueness of their product customers was ready to wait for 15-20 days but when they are not having unique requirements why they should wait. It can be clearly observed from Dells case that information technology plays an important role in knowing what customer wants and subsequently making supply chain alterations and that is why supply chain analytics is important.
This paper primarily focuses on achieving total supply chain profitability between and among different supply chain stages like plant location, inventory management, manufacturing, selling, storing, disposing of etc. using Information Technology-driven scientific as well as a logical approach towards supply chain decision making. This can be made possible by improving supply chain visibility, managing volatility and reducing fluctuation in cost as customer requirements are getting diversified day by day. It has become necessary for the businesses to include more designs more variations of products while also keeping a check on the quantities they should keep in order to maintain a good service level and minimize the risk of over or under inventory. It is also important to keep the lead time short and costs minimum to increases customer satisfaction and retention to a great extent. And in order to do so various kinds of the trade-off between efficiency and responsiveness are to be made for better serving.
-
DRIVERS OF SUPPLY CHAIN
-
FACILITIES
-
An important driver of the supply chain where one should have to be very careful while formulating a strategy regarding facilities like the role of the facility whether it is a manufacturing facility, warehouse, distributor or retailer, its sizes and location also plays an important part in deciding a successful supply chain. If the unit
cost of the product is very high we go for a centralized facility and vice versa. If the organizations want to achieve high economies of scale they go for bigger sizes of their facilities.
-
-
INVENTORY
-
Inventory is very crucial for any supply chain. It is available to every player in the supply chain like a wholesaler, retailer etc. here the question arises what is the optimum level of inventory one should keep in order to avoid stock-outs or surplus because keeping an inventory invites two types of variable cost ordering cost and carrying cost, so that makes inventory a liability when not managed efficiently.
-
-
TRANSPORTATION
-
Movement from one point of the supply chain to another incurs transportation cost. Economies of scale are maintained when goods move from manufacturer to wholesaler in big lots and then it is bifurcated into smaller lots and sent to different retailers by losing economies of scale with it but transportation incurs anyway.
-
-
PRICING
-
Supply chain management ensures a proper balance between demand and demand. There are various areas where demand fluctuates drastically which affect supply. By creating a differential pricing mechanism to some extent we can maintain the balance between demand and supply.
-
-
-
-
BACKGROUND
Data analytics contains a large chunk of tools and techniques to analyze the available knowledge. Theyre loosely classified as descriptive, diagnostic, prognostic and prescriptive analytics (Hardoon, D., Shmueli, G., 2013).. Theres a continous trade-off between the insights generated and recommended solution (Barga,R.,Fontama,V.,Tok,W.H,2014) . Hence, it's important to utilize analytics to rework information into valuable knowledge (insights) therefore on act upon them effectively and to satisfy their objectives (Kaisler, S.H., Espinosa, J.A. et al, 2014). Prescriptive analytics helps to bridge this gap and is taken into account because it is the next frontier in the business analytics (Evans, J.R, lindner, C.H., 2012). Prescriptive analytics is blend of descriptive and predictive analytics. Prescriptive analytics aims to determine the most effective and optimum solution or outcome among varied decisions, given the far-famed parameters. It provides organizations with adaptive, agile and flexible sequences of operational actions. It answers queries like What Why when and extremely importantly how ought o be done (Delen, D., Demirkan, H., 2013). However, within the preview of Big-data, analytics engine should be dynamic enough to produce the most effective doable cause-effect relationship for the choice manufacturers within the enterprise.
lately, the overwhelming majority of business analytics efforts square measure spent on descriptive analytics and prognostic analytics with typical methodology together with data processing, machine learning, Artificial Intelligence and simulation (den Hertog & Postek, 2016; Habeeb et al., 2018; Larose & Larose, 2015). It helps organizations adapt to the reasons of the events that occured within the past and perceive relationships among completely different styles of knowledge (Soltanpoor & Sellis, 2016). However, equally to different analysis works (Krumeich et al., 2016; iknys & Pedersen, 2016), we have a tendency to take into account it as a part of descriptive analytics. the explanation for this is often to confirm consistency among the 3 stages of analytics so all answers the queries What? and Why?.. Recently, however, prescriptive analytics has been more and more gathering analysis interest (Larson & Chang, 2016). it's been thought-about because the next step towards increasing knowledge analytics maturity and resulting in optimized call making, prior to time, for overall business throughput improvement (den Hertog & Postek, 2016; Gartner, 2017).
CHALLENGES OF SUPPLY CHAIN
-
Lack of integration among various activities like planning and execution creates a difference which hampers organizational objective in the long run.
-
Absence of real-time data visibility with no common view across all businesses and channels refers to the amount of information collected by different members of the supply chain individually creates an atmosphere of uncertainty.
-
Unaccounted reviews of buffer stock level, causing frequent stock out results in a decrease in customer satisfaction or excess inventory will pull down the profitability
-
As customer demands are getting more diversified flexibility is required with respect to both varieties as well as quantity to increase customer satisfaction.
-
Price volatility and difficulty de-risking. Minimizing risk proved to be a great difficulty on the road to achieve efficiency and effectiveness. Globalization, exchange rate issues, competition are posing regular challenges to the supply chain.
-
Misbalanced manufacturing line and irregular batch sizes. Creating asset underutilization. The improper tradeoff between asset utilization and flexibility results in underperformance, on the one hand, we have reduced costs on the other hand we have better customer satisfaction. A quantifiable middle-ground solution is necessary in order to have a win-win scenario.
-
-
HOW CAN ANALYTICS HELP
Analytics has always been a boon for businesses with the advent of enterprise resource planning (ERP) since the 90s and more recently with the buzz of big data have brought up analytics to a whole new level. it is valuable to those areas where data and information are recorded to discover and communicate quantifiable pattern in the raw data using statistics, computer
programming, analysis tools and mathematics of operation research to gain insights and evaluate. Supply chain analytics rely on the data collected on the different stages of the supply chain via various sources like POS, RFID and GPS or unstructured sources like camera and surveillance footage, social media, blogs and forum discussions.
Analytics uses the descriptive, predictive and prescriptive model to derive meaningful insights from the data simultaneously highlighting the plan of action to cater to business decision making using extensive big data computation and optimization techniques.
Supply chain analytics is different from supply chain management as it focuses on a data-driven scientific and logical approach and Information Technology to make a decision relating to various supply chain operations like the flow of materials, money and information. Also helps in maintaining the balance between supply and demand and increase profitability of the organization by contributing directly towards the bottom lining of sourcing, logistical, carrying and disposal costs.
However, this paper focuses on the feasibility of prescriptive analytics which is a whole new phenomenon as it helps in finding the solution to the problem. In the supply chain, prescriptive analytics unearths countermeasures based on descriptive and predictive analytics models and optimization techniques.
DATA TYPE
SIZE
SPEED
DIVERSIFICATION
SALES
Price, quantity, items, time of day, date
From monthly & weekly to daily
Direct sales, distributor sales, internet sales, competitor sales
CUSTOMER
Item browsed and bought, frequency, dollar value
From click-through card usage
Shopper identification, emotion detection, like, tweets and product reviews
RAW MATERIAL
Perpetual colour etc.
inventory
by
style,
From monthly updates to hourly updates
Warehouse, internet store, vendor inventories, outlet
LOCATION/TIME
Sensor data to detect location, better inventory control
Frequent updates within the store and in transit
Not only where, but what is close, who moved it, path, future path, mobile device evidence
(Source: Waller and Fawcett, 2013a, Journal of Business Logistics)
-
METHODOLOGY
-
Forecasting is the basis of all strategic, operational and tactical decision making. It helps in anticipating upcoming scenarios and it is been used extensively in all business activities like production, marketing, finance and human resource as all of these works together as a whole in a coordinated manner. The forecast is not exact values because there is always a factor of uncertainty involved but projections with a possibility of error with the help of sophisticated models we try to reduce this error as much as possible.
From a forecasting point of view, we generally divide the data into two components systematic(S) and random(R). Out of the two, we can only determine systematic component(S) using tools and techniques while random component (R) cannot be determined and attributed to forecasting error. So to make a better forecast we need to minimize systematic component(S) as much as possible.
Actual demand (A) = Systematic(S) + Random(R)
Exponential smoothening is recommended for forecasting when data contains level (), trend () and seasonality () components to smoothen the fluctuations in data. The most common trend and seasonality models are additive trends and ratio seasonality. So here is the forecast model accustomed to additive trend and ratio seasonality
t = ( ) + (1- ) (
+Tt-1)
Ft, 1: Desired forecast of the period in the current cycle
t : updated base value of the period in the current cycle
1
: updated trend value of the period in the current cycle
t = ( t – 1 ) + (1-) Tt-1 t
I () (1-) I
It-L+1 : seasonal index of the period in the previous cycle
t =
t-L
Dt : current demand
Where,
Ft,1 = ( t + )( It-L+1)
St-1 : base value of the previous period in the current cycle
Tt-1 : trend value of the previous period in the current cycle
It: seasonal index of the previous cycle
-
The Echelon inventory works on the concept of inventory unification earlier inventores are treated as a separate entity by their location like manufacturer, wholesaler or retailer while the echelon concept unifies this as a whole meaning thereby amount of inventory at all the locations of our supply chain is the total inventory.
To better understand this concept of inventory management here is an illustration having two installations 1 & 2 their quantity demanded are q1 and q2.
Inventory cost(C)
=
order
cost
(k) + holding
cost
(h)
C2 = dk2/q2 + q2p/2
Where,
d = required inventory
k2 = ordering cost at
installation
2
When differentiated and equated to zero it gives
Q2 = 22/2 (the amount of economic order quantity at installation 2) By putting this value in above equation we get
C2 min = 222
Similarly,
C1 = dk1/nq2 + (n-1) q2p/2 (q1 = nq2)
When differentiated with respect to n n = 1/2 2/1 where n I
For simultaneous echelon model
Inventory cost(C) = order cost (k) + holding cost (e) where
C = C1+C2
C = dk2/q2 + dk1/nq2 + q2p/2 + (n-1) q2p/2 C = d/q2(k2+k1/n)+q2/2(ne1+e2)
q2 = 2(2 + 1/)/1 + 2
Cmin = 2(2 + 1/(1 + 2))
When differentiated with respect to n
n = 1/2 2/1 where n I
e1 = p e2 = p-p
by applying this echelon model of the simultaneous inventory we can reduce the cost to some extent.
-
Decision making regarding the location of the facility requires utmost priority because this requires a lot of capital and resources and many factors are taken into consideration while deciding the optimum location of setting up the facility. By using analytical tools and sophisticated methods we can find an ideal location. One of the methods is the gravity method which uses coordinates, annual tonnage to find an optimum solution.
x,y : warehouse coordinates
dn= ( )^2 + ( )^2
xn,yn : coordinates of delivery location n
/
x = =1
dn : distance to delivery location n
Fn : yearly load(tonnes) to delivery
=1
/
location n
y=
y=
=1
=1
/
/
Along with it minimizing the cost MIN
-
-
RESULT
The above methods and techniques of prescriptive analytics have given more logical, attainable and efficient results when applied. Although a bit of uncertainty will always be there as all the parameters are not quantifiable or can be taken into account but affects the decision-making process to a great extent. By applying analytics the extent of risk associated can be reduced a great extent by better forecasting we can anticipate fluctuation in demand and act accordingly, better inventory management we can reduce the frequency of stockouts and excess inventory which decreases profitability when become deadstock, and optimized location decision will lead to an effective resource and capital allocation and its efficient utilization with minimum possible wastage.
Furthermore, analytics can give you an educated guess but along with it having experience can increase the chances of better decision making.
-
CONCLUSION
In developing countries like India due to many constraints supply chain analytics isnt being used extensively in organizations. It is being used but not to the extent to which it should have been used. First of all the top management needs to divert their focus from obsolete techniques which businesses are using for a long time and create an analytics culture in an organization. An awareness campaign should be run in the organization to familiarize all the personnel about analytics using simple and easy to understand models. The organization must rely on the figures and insights gained from using various analytical models.
Organizations must realize that analytics isnt any magic or mantra through which we can see into the future but it is an educated guess backed by science and logic which can give a justifiable figure within the quantifiable environment to work upon.
-
REFERENCES
-
Barga, R., Fontama, V., Tok, W.H.: Predictive Analytics with Microsoft Azure Machine Learning: Build and Deploy Actionable Solutions in Minutes.
Apress (2014). ISBN-13 (pbk): 978-1-4842-1201-1 and ISBN-13 (electronic): 978-1-4842-1200-4
-
Kaisler, S.H., Espinosa, J.A., Armour, F., Money, W.H.: Advanced analytics-issues and challenges in a global environment. In: 2014 47th Hawaii International Conference on System Sciences (HICSS), pp. 729738. IEEE (2014)
-
Evans, J.R., Lindner, C.H.: Business analytics: the next frontier for decision sciences. Decis.Line 43(2), 46 (2012)
-
Delen, D., Demirkan, H.: Data, information and analytics as services. Decisi. Support Syst.55(1), 359363 (2013)
-
Den Hertog, D., & Postek, K. (2016). Bridging the gap between predictive and prescriptive analytics-new optimization methodology needed available
at:. Netherlands: Technical report, Tilburg University. http://www.optimization-online.org/DB_HTML/2016/ 12/5779.html.
-
Soltanpoor, R., & Sellis, T. (2016). Prescriptive analytics for big data. In M. A. Cheema, W. Zhang, & L. Chang (Vol. Eds.), 27th Australasian database conference: ADC 2016. Databases theory and applications, LNCS: Vol. 9877, (pp. 245325). Sydney, NSW: Springer International Publishing.
-
Krumeich, J., Werth, D., & Loos, P. (2016). Prescriptive control of business processes. Business & Information Systems Engineering, 58(4), 261 280.
-
Gartner (2017). Planning guide for data and analytics. Last Accessed: 03 April 2018 https://www.gartner.com/binaries/content/assets/events/keywords/catalyst/catus8/2017_ planning_guide_for_data_analytics.pdf.
-
Larson, D., & Chang, V. (2016). A review and future direction of agile, business intelligence, analytics and data science. International Journal of Information Management, 36(5), 700710.