
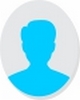
- Open Access
- Authors : Pranav Addepalli , Sundaram Thirukkurungudi
- Paper ID : IJERTV10IS010070
- Volume & Issue : Volume 10, Issue 01 (January 2021)
- Published (First Online): 18-01-2021
- ISSN (Online) : 2278-0181
- Publisher Name : IJERT
- License:
This work is licensed under a Creative Commons Attribution 4.0 International License
An Investigation of in Fill Process Variables to Reduce Warpage in Fused Filament Fabrication
Pranav Addepalli Academy of Science Academies of Loudoun Leesburg, United States
Sundaram Thirukkurungudi Academy of Science Academies of Loudoun Leesburg, United States
AbstractCurrently, three-dimensional (3D) printing is being widely used for rapid pro-totyping and Just in Time manufacturing. Many commercial 3D printers use fused filament fabrication (FFF), a printing technique where a solid thermo-plastic filament is melted and extruded onto sequential two-dimensional layers to produce a 3D object. In FFF printing, thermal stresses between layers due to inhomogeneous thermal conduction during cycles of heating and cooling create distortions, known as warpage. Process variables, especially infill density and infill pattern, cause thermal properties to become anisotropic because of thermal conduction through plastic and natural convection in air gaps. In order to identify the effect of these process variables on thermal warpage, Polylactic Acid (PLA) discs with varying infill densities and patterns were printed and the spatiotemporal temperature distribution was modeled. Trajectory similarity analysis using the Dynamic Time Warping algorithm as well as a temperature gradient analysis with statistical tests were conducted. Based on the analysis of the data, this study recommends that 30% infill density and trihexagonal infill pattern be used in FFF printing with PLA to mitigate the warpage.
KeywordsWarpage; fused filament fabrication; infill; temperature distribution; thermal stress; dynamic time warping
-
INTRODUCTION
-
3D Printing Techniques and Filaments
3D printing, a form of additive manufacturing that uses a digital design to create structures, is being widely used in many major industries for rapid prototyping and Just in Time manufacturing due to its efficiency in transferring digital design of complex structures into an end product. Some of the common industries which use the techniques of 3D printing are construction, medicine, electronics, automotive, robotics, aerospace, and defense industries. It is also becoming an attractive research field for chemical, material, and biomedical 10 projects due to its ease of operation, low cost, and high manufacturing speed [1]. For example, Bhattacharjee, Urrios, Kang, & Folch were able to fabricate micro fluidic systems with 3D printing due to the assembly-free, automated fabrication as well as the increasing resolution and throughput offered by 3D printing [2]. Raney & Lewis studied the ability of 3D printing to design and rapidly fabricate complex mesoscale architectures without the need for lithographic masks or expensive tooling [3]. In addition, Trachtenberg et al. systematically evaluated novel biomaterials for 3D printing as a technique for fabricating tissue engineered scaffolds, finding that it is possible to print high viscosity biomaterial solutions with control over pore size and fiber diameter [4]. Peele, Walling, Zhao, & Shepherd implemented 3D printing to design
antagonistic systems of artificial muscle via soft actuators, finding that the full range of motion is achieved with a period of less than only 70 ms [5]. Furthermore, Goole & Amighi extensively studied 3D printing in pharmaceutics, concluding that 3D printing of customized dosage forms will revolutionize drug delivery [6]. Park, Gao, Jang, & Cho specifically researched the use of 3D printed structures for delivery of cells and biomolecules for tissue repair and regeneration, finding that 3D printing enables the precise placement of multiple biological components with design freedom for specific spatial geometric arrangements [7].
In 3D printing, parts are manufactured by fusing layers. Computer software is used in 3D printing to slice a 3D digital model vertically along the Z-axis and create a set of commands for the printer to create each layer. Different techniques are used to convert a digital design into a solid part, most notably stereolithography (SLA), selective laser sintering (SLS), and fused filament fabrication (FFF) [8]. FFF is more common due to its simpler technology and low cost.
SLA printing implements photopolymerization, a process in which ultraviolet light links chains of molecules to create polymers. The polymers formed fuse to create the solid body of an object. SLS printing is a relatively new technology that uses a laser to sinter a powdered material and create a solid structure. SLS printing methods are used in direct metal laser sintering (DMSL) to melt and fuse powdered metal together with a laser. DMSL, SLS, and SLA, although having substantial capability, are expensive and are primarily only used in advanced industrial applications.
FFF printing, however, is commonly employed in commercial 3D printers. In FFF printing, a solid thermoplastic filament is melted and then extruded onto a bed to create a two- dimensional layer. The extruded semi-liquid polymer solidifies almost immediately after leaving the extruder. Layers are printed on top of previous layers repeatedly to create a three- dimensional solid object from the digital design. In FFF printing, one of the most important qualities of the filament for it to be extruded through the nozzle is the ability to melt or soften at high temperatures, which can cause some difficulties for freeform features [9]. Dizon, Espera, Chen, & Advincula explain that the unique quality of FFF printing to melt and cool thermoplastics layer by layer, along with its high speeds and low costs, has resulted in FFF becoming the commonly used technique for 3D printing [8].
Polylactic acid (PLA) is the most widely used commercial thermoplastic filament for FFF printing because it is derived from renewable lactic acid, which is biodegradable and bioactive [10]. PLAs melting temperature, around 180°C to
220°C, is lower than those of other filament materials such as acrylonitrile butadiene styrene (ABS), which is around 190°C to 270°C, and polyethylene terephthalate (PET), which is around 260°C to 290°C. Lower melting temperatures and better print quality make PLA a preferable material for FFF printing.
-
Warpage in FFF
FFF printing involves processing filament through thermal cycles which can cause distortions, known as warpage, in the objects. Since FFF printing is done layer by layer, the 3D printing process is not continuous but rather a step by step deposition along the Z-axis [11]. Since each layers specific component distribution is programmed and deposited on assigned locations in a controlled manner, the physical properties of the printed materials will be anisotropic. Importantly, there are many interfaces between the layers [1]. During the printing process, after a layer of plastic is deposited, the cooling of the layer causes the plastic to contract and create stress along the objects lateral surfaces. Increased rate of cooling increases the stress. This stress has the greatest effect at corners of objects since they are structurally anisotropic. This stress causes the corners to be pulled both upwards and inwards. Any detachment of the object from the printer bed can compromises printing of successive layers. The repeated heating and cooling cycles during the printing process of each successive layer leads to cascading of this problem, resulting in inconsistent print qualities and varying levels of warpage. Larger variation in temperature per cycle caused by larger time lag between layers exacerbates this problem in wider prints. After analyzing the warpage problem, Armillotta, Bellotti, and Cavalaro suggested that in addition to the thermal cycles, warpage occurs because of the extension of thermal stresses to multiple layers due to heat conduction from the previous layer [12].
-
Process Variables in FFF
Most modern 3D printers do not print a fully dense structure to reduce time and cost for each print; instead, objects are made with different internal structures [13]. The internal structures of printed objects are modified using different process variables. Important process variables in 3D printing are infill density and infill pattern.
Infill Density. Infill density is the percentage of the object that is plastic. A 0% infill indicates that the object is completely hollow, and 100% infill indicates the object is fully plastic. Common infill densities in FFF printing are typically below 40%.
Infill Pattern. The infill pattern is the specific geometric pattern that constitutes the majority of the internal structure. Common infill patterns include grid/linear, triangular, honeycomb, and trihexagonal. Varying the infill density and the infill pattern has multiple advantages. For example, prints with higher densities tend to have higher tensile strength and prints with more complex infill patterns tend to have higher compressive strength [14].
-
Heat Transfer in FFF
Within a printed object, conduction and convection allow heat to be transferred throughout the material. However, conduction in plastics takes priority over both conduction and convection in enclosed spaces because of greater transfer of heat by conduction in plastic. Research in other fields
corroborates this, as Kim and Viskanta show that increased wall heat conduction reduces the average temperature difference in a cavity, stabilizes the heat flow, and, most importantly, reduces the rate of heat transfer by natural convection [15]. The most significant impact of their research in this context is that they find that conduction is the superior heat transfer process in an enclosure such as the internal structure of a 3D printed object.
Generalizing their results supports the idea that plastic has a much greater impact on heat transfer and overall temperature distribution of a 3D printed object than air. Han supports this in his study on the thermal conductivity of PLA objects and found that the thermal conductivity depends nearly linearly on the volume infill percentage [13]. He noted that the only major discrepancy between the results, although being minimal, could be due to the natural convection caused by air in enclosed spaces.
-
Current Research
Studies have been done on the heat transfer and thermal conductivity of porous structures for various materials, but none have studied extensively plastic, PLA, or the effect of a specific internal pattern on the heat transfer. For example, Deng et al. investigated the effect of 3D printed hollow structures in sand mold manufacturing and found that hollow structures could be used as heat insulators due to the increased number of air cavities and less solid material [16]. Their research shows how increased porosity in sand molds leads to a decrease in thermal conductivity.
Although there is published research on the thermal conductivity and heat transfer properties of porous media of different materials, there is limited research on the heat transfer of porous plastic materials. Zhuang et al. were able to create objects with anisotropic heat distributions through 3D printing [1]. Additionally, efforts have been made to 3D print heat exchangers; for example, Haertel and Nellis used various designs through density-based topology optimization to create a fully developed 3D printed heat exchanger [17]. They found that more thin walls and higher unit cell heights within the heat exchanger increased thermal conductivity.
Current research in 3D printing does not focus on the thermodynamics and heat transfer properties of the objects. Rather, they mainly focus on application of 3D printing to various industries. Zhang, Wang, Yu, and Deng numerically analyzed the influence of 3D printing conditions on heat transfer [18]. Their research offers an extremely specific mathematical model during and after printing; however, the conditions set by the researchers are impractical for use of the model in any other situation. They assumed the objects printed were pore-free, essentially a 100% infill, which is impossible to recreate because of the slight errors in the extrusion of plastic from a 3D printer. They also neglected heat radiation within the object, polymer crystallization and energy balance, and thermal expansion.
Fig. 1. Top and side view of template disc CAD model.
-
-
MATERIALS AND METHODS
-
Disc Design and Preparation
A template disc was designed using CAD software, specifically, Fusion360, with a diameter of 8 inches and a height of 0.5 inches. Construction circles were created on the template disc with smaller diameters, incrementing by 1 inch, to create circles with diameters 7, 6, 5, 4, 3, 2, and 1 inches. Random positions on these construction circles were chosen to embed thermistors, and rectangular channels were designed so the thermistors and their wiring could be embedded. The channels connected each of the randomly chosen positions to a single channel along the diameter, which led to open ends on both sides of the print. These channels began at a height of 0.15 inches and ended at a height of 0.35 inches so that the entire model was composed of 0.15 inches of regular infill, 0.2 inches of channels and infill, and another 0.15 inches of regular infill from the bottom of the model to the top. Figure 1 shows multiple views of the model.
The model was sliced multiple times using Ultimaker Cura to transform it to G-code for the printer. All print settings were kept as the default values as shown in Table 1, except for infill density and infill pattern. A gCreate gMax 1.5 XT+ printer with heated bed addition and PLA filament from Hatchbox was used for all prints.
TABLE I. IMPORTANT PRINTER AND SLICER SETTINGS
Setting
Value
Nozzle Temperature
200°C
Bed Temperature
85°C
Layer Height
0.2 mm
Flow
102%
Print Speed
80 mm/sec
Wall Thickness
0.04 m
A post-processing script to pause after the channels had been printed (at height of 8.89 millimeters) was added in Ultimaker Cura. G-code was produced for 6 combinations of process variables as shown in Table 2 and saved to an SD card before being printed.
TABLE II. PROCESS VARIABLE VALUES
Disc
Infill Pattern
Infill Density
1
Lines
10%
2
Lines
20%
3
Lines
30%
4
Trihexagonal
10%
5
Trihexagonal
20%
6
Trihexagonal
30%
Fig. 2. A single Arduino voltage divider circuit for temperature measurement using thermistors. Voltage for the thermistor is measured using pin A0.
Lines and Trihexagonal patterns as well as 10%, 20%, and 30% densities were chosen because of their common prevalence in commercial 3D printing.
-
Temperature Measurement System
Eight voltage divider circuits were designed and built on a breadboard using an Arduino Mega, 10kOhm resistors, wiring, and 20kOhm NTC thermistors from Vishay/BC Components, model number NTCLG100E2203JB. Figure 2 shows the basic wiring for the voltage divider circuit used. Voltage at each thermistor was measured from each Arduino pin./p>
The voltage was converted into temperature values using the Steinhart-Hart Equation (Equation 1), where T is the thermistor temperature in Celsius; TA, TB, and TC represent the thermistor alpha, beta, and gamma coefficients respectively; R0 represents the fixed resistance in the circuit, and V represents the analog thermistor reading.
(1)
Fig. 3. Embedding thermistors into a disc.
Thermistor coefficients were determined using a datasheet provided by the manufacturer. An Arduino sketch was created to read the voltages, calculate temperature in Celsius, and output temperature values to a serial port for every second. puTTY was used to read the values in the serial port and log them to a text file.
-
Temperature Data Collection
During the printing process of each disc, 8 thermistors were embedded into the positions previously determined and wired through the channels to the outside of the disc. As shown in Figure 3, four thermistors were placed in one side and wired to the main channel and to an opening on the right, and another four were placed on the other side and wired to the main channel and to an opening on the left. The wires from the openings were connected to the voltage divider breadboard circuit.
After embedding thermistors, puTTY was used to log the Arduino session output into a text file. The print was resumed such that PLA would cover the channels and print the rest of the disc model as shown in Figure 4. This process of printing and embedding thermistors was repeated for all of the combinations in Table 2. The positions of the thermistors within the disc can be modeled in the Cartesian coordinate plane. Table 3 shows the coordinate locations of each thermistor, where (0, 0) is the center of the disc, and Figure 5 shows a map of the locations.
Fig. 4. Disc with thermistors fully embedded.
Fig. 5. Locations of all thermistors in the Cartesian coordinate system.
TABLE III. THERMISTOR LOCATIONS IN THE CARTESIAN SYSTEM
Thermistor
X
(mm)
Y
(mm)
1
70.0
-70.0
2
55.0
56.0
3
32.4
-41.8
4
24.0
15.0
5
0.0
-12.0
6
-22.5
33
7
-45
48
8
-72.5
56
-
-
ANALYSIS
Due to the sheer size of the raw data, consisting of over 400 thousand individual points, the data is not displayed here. However, the raw data is available upon request. The following models and methods were used to analyze the data in Python 3.7: the temperature-weighted mean model, isotropic distance analysis, and the dynamic time warping algorithm. The analysis was predicated upon the identification of the extent of the isotropy of the thermal distributions. Isotropic thermal distributions are defined as those that are uniform in all directions. Since anisotropic thermal properties cause warpage, it was assumed that discs with the most isotropic temperature distributions should theoretically have the least warpage.
-
The Temperature-Weighted Mean Model
Since the temperature, T, is a scalar function of position and time, the temperature distribution of the disc can be modeled by the function T(x,y,t) where x and y are distances in millimeters from the center along two perpendicular 220 axes on the horizontal plane and t is the time in seconds. However, T is known for only 8 discrete values of (x,y) pairs at any time t the positions of each thermistor at any time t. Taking the temperature-weighted mean of the (x,y) pairs for every time t for a single disc yields a distribution of temperature centers that can be described by the function Tc(t) that returns a coordinate point.
(2)
The distribution of temperature centers for each disc shows the movement of the position of the center of temperature during the printing process, allowing for a better understanding of the thermal processes in each disc. This model was used to apply the isotropic distance analysis method and the dynamic time warping algorithm.
-
Isotropic Distance Analysis
Comparing the isotropy of each temperature distribution required a distance analysis method to be created in order to show a rough measure of the isotropy. Temperature data from an initial reference steady-state, specifically the first 20 seconds, was passed into Tc(t) in order to find a temperature center that represented the thermal equilibrium for each disc.
For every time t for the specific disc, the Euclidean distance from the temperature center at t, Tc(t), to the thermal equilibrium point was calculated. Because the thickness of the disc is significantly smaller than the distances that the temperature distribution was measured along, the model of the disc was assumed to be two-dimensional. Theoretically, smaller mean distance values indicate more isotropic distributions as their equilibrium points are closer to the center of temperature over time. The distance distributions for each disc were compared to each other using multiple Students t- tests with independent samples. The results of these tests are shown in Table 4 in the Results section.
-
Dynamic Time Warping
The Dynamic Time Warping (DTW) algorithm is a technique used in time series analysis to measure the similarity between two temporal sequences that vary in length. The DTW algorithm finds the optimal alignment between two time series if one time series is warped non-linearly by stretching it or shrinking it along its time axis [19]. The warp path distance, or similarity score, is the difference between the two time series and is calculated by finding the sum of the linear distances between pairs of corresponding points on each sequence. Thus, two identical time series would have a similarity score of zero.
The DTW algorithm is commonly used in speech recognition to determine if two speech waveforms represent the same spoken phrase. It is also commonly used in data mining to determine the similarity between two time series [19]. In this study, heat flow trajectories in the form of time series can be generated based on the distribution of temperature centers for each disc. Each temperature centers position in the Cartesian system was used to generate a temporal sequence that represented the movement of the center of temperature during the printing process. The DTW algorithm was applied to compare the heat flow trajectories. The results are shown in Table 5 in the Results section.
-
-
RESULTS
TABLE IV. RESULTS OF THE T-TESTS
Sample 1
Sample 2
p
T
10% Lines
10% Trihexagonal
0
48.293
20% Lines
20% Trihexagonal
0
127.985
30% Lines
30% Trihexagonal
2.137 x 10252
34.499
10% Lines
20% Lines
0
– 140.179
20% Lines
30% Lines
0
120.111
10% Trihexagonal
20% Trihexagonal
0
-53.354
20% Trihexagonal
30% Trihexagonal
1.692 x 107
5.233
TABLE V. RESULTS OF THE DTW ALGORITHM
Sample 1
Sample 2
Similarity Score
10% Lines
10% Trihexagonal
13654.523
20% Lines
20% Trihexagonal
12627.314
30% Lines
30% Trihexagonal
9859.541
10% Lines
20% Lines
16607.523
20% Lines
30% Lines
12024.950
10% Trihexagonal
20% Trihexagonal
6309.220
20% Trihexagonal
30% Trihexagonal
3997.648
Table 4 shows the results of the statistical tests used to compare the Euclidean distances from the isotropic distance analysis for each distribution. The two-tailed p-value and the T-value are also shown for each test. p-values below the significance level of 0.025 indicate that the mean distances of each distribution are statistically significantly different, and the sign of the T-value provides information as to which of the distributions mean distance is greater. Positive T-values indicate that the mean distance of the first sample is greater than that of the second sample, and negative T-values indicate that the mean distance of the first sample is smaller than that of the second sample. In rows one through three, the mean distances of the infill pattern distributions are compared while keeping the infill density constant to study the effect of the infill pattern. In rows five through eight, the mean distances of the infill density distributions are compared while keeping the infill pattern constant to study the effect of the infill density.
Table 5 shows the results of the DTW algorithm. The similarity score is shown for each test. Because the similarity score is found by calculating the sum of the distances between each warped series, smaller scores represent more similar sequences. The results in Table 5 are arranged similarly to Table 4. Rows one through three compare the heat flow trajectories of the infill pattern distributions while keeping the infill density constant to study the effect of the infill pattern. In rows five through eight, the heat flow trajectories of the infill density distributions are compared while keeping the infill pattern constant to study the effect of the infill density.
-
DISCUSSION
Based on the hypothesis in the Analysis section, there is a negative correlation between the isotropy of the temperature distribution and warpage. In addition, based on the assumptions of the isotropic distance analysis method, discs with a smaller mean distance have more isotropic temperature distributions. Therefore, for each row, the sign of the T-value will specify which disc has the least warpage. As all p-values in Table 4 are below the significance level, the mean distances of each sample distribution for each test are all statistically significantly different.
In Table 4, rows one, two, and three compare the lines and trihexagonal infill patterns for the 10%, 20%, and 30% infill densities, respectively. The positive T-value in all three rows indicates that for 10%, 20%, and 30% infill densities, discs with a trihexagonal infill pattern had smaller mean distances than those with the lines infill pattern. Therefore, the trihexagonal infill pattern causes the disc to become more isotropic in its temperature distribution and is thus better at reducing warpage than the lines infill pattern.
Row five compares the 10% and 20% infill densities for the lines infill pattern. The negative T-value indicates that discs with the lines infill pattern and 10% infill density had smaller distances than those with 20% infill density. Row six compares the 20% and 30% infill densities for the lines infill pattern. The positive T-value indicates that discs with the lines infill pattern and 30% infill density had smaller distances than those with 20% infill density. Similarly, row seven compares the 10% and 20% infill densities for the trihexagonal infill pattern. The negative T-value indicates that discs with the trihexagonal infill pattern and 10% infill density had smaller distances than those with 20% infill density. Row eight compares the 20% and 30% infill densities for the trihexagonal infill pattern. The positive T-value indicates that discs with the lines infill pattern and 30% infill density had smaller distances than those with 20% infill density.
Therefore, for both infill patterns, 20% infill density causes the most anisotropy in the temperature distribution and is thus the worst density for reducing warpage of the infill densities tested. Previous research supports that in most mechanical applications, 30% infill density should be used rather than 10% infill density due to the significant increase in both tensile and compression strength. However, the strength benefits of 30% infill density may be outweighed by the lower production costs of 10% infill density. The choice between 10% and 30% infill density is therefore dependent upon the specific application and requirements of the part; however, this study recommends 30% infill density as it provides greater mechanical and thermal benefits.
The results of Table 5 show important trends that can influence decisions on the infill process variables. Rows one, two, and three show the results of the DTW algorithm on the heat flow trajectories of the discs with lines and trihexagonal infill patterns for the 10%, 20%, and 30% infill densities. Interestingly, with increase in the infill density, there is a decrease in the similarity score for the infill patterns. This means that the heat flow trajectories for the lines and trihexagonal infill patterns are becoming more similar as the infill density is increased. Thus, the effect of choosing lines or trihexagonal infill is marginalized at 30% infill density but is
enhanced at 10% infill density. Therefore, the choice of a specific infill pattern becomes less influential at higher infill densities.
Rows five and seven show the results of the DTW algorithm on the heat flow trajectories of the discs with 10% and 20% infill densities with the lines infill pattern and the trihexagonal infill pattern. Rows six and eight show these results for the discs with 20% and 30% infill densities with the lines infill pattern and the trihexagonal infill pattern. For both patterns, the similarity score for the comparison between 10% and 20% infill density is larger than the similarity score for the comparison between the 20% and 30% infill density. This indicates that for both patterns, the heat flow trajectories for discs with the 20% and 30% infill densities are more similar than those with the 10% and 20% infill densities. Thus, at high infill densities, an additional increase results in a smaller effect than an additional increase at a low infill density. Therefore, the effect of increasing infill density is reduced for every additional increase. In addition, the similarity scores in rows seven and eight are substantially lower than the similarity scores in rows five and six, meaning that despite the difference in infill density, the discs with trihexagonal infill pattern were more similar than the discs with lines infill pattern. Therefore, the effect of increasing infill density on the heat flow trajectory is smaller with the trihexagonal infill pattern than with the lines infill pattern.
Based on the results of this study, the infill process variables, infill pattern and infill density, seem to have a substantial effect on the isotropy of the temperature distributions and consequently on part warpage. Studying more variables, such as wall thickness and layer height, can widen the range of parameters used in this analysis. More process variables and measurements for each increment of these variables enables the problem of warpage to be treated as a convex optimization problem. Such a mathematical model based on the temperature distributions will provide ideal values for the process variables for different geometrics. Future work may also include atempts to identify the role of natural convection on warpage, where the fluid motion of the air inside the infill transfers heat throughout the internal structure. As natural convection is a process that is difficult to quantify and study, this could be accomplished with thermal analysis and computational fluid dynamics simulation.
-
CONCLUSIONS
With growing applications for fused filament fabrication in industry, the thermal properties of manufactured parts become critical in understanding the widespread problem of warpage due to part size. This study identifies that infill pattern and infill density are major factors on the isotropy of temperature distributions as well as the pattern of heat flow trajectories. Since increase in isotropy of temperature distribution reduces warpage, the results demonstrated that the choice of the trihexagonal infill pattern over the lines infill pattern reduces warpage significantly. In addition, 30% infill density is recommended in order to reduce warpage and promote part strength, but 10% infill density is still a viable option if cost is an important factor. The results also support that with increase in infill density, the effect of the choice of infill pattern on warpage reduces significantly, and that increasing infill density
becomes less influential on warpage with successive increases. Finally, the results show that the effect of increasing infill density on the temperature distribution is smaller with the trihexagonal infill pattern than with the lines infill pattern. It will be beneficial to incorporate convex optimization into future analyses involving a greater variety of process variables. Additional work is required to understand the specific impact of convection within enclosed spaces on warpage. However, this study establishes the role of infill process variables in reducing warpage in fused filament fabrication.
ACKNOWLEDGMENT
We would like to thank the Loudoun Academy of Science for providing the facilities and equipment used in this study. This work was supported by the Academies of Loudoun.
No potential competing interest was reported by the authors.
REFERENCES
-
Y. Zhuang, W. Song, G. Ning, X. Sun, Z. Sun, G. Xu, B. Zhang, Y. Chen, S. Tao, 3d-printing of materials with anisotropic heat distribution using conductive polylactic acid composites, Materials & Design 126 (2017) 135 140. doi:10.1016/j.matdes.2017.04.047.
-
N. Bhattacharjee, A. Urrios, S. Kang, A. Folch, The upcoming 3D- printing revolution in microfluidics, Lab Chip 16 (10) (2016) 1720 1742. doi:10. 1039/C6LC00163G.
-
J. R. Raney, J. A. Lewis, Printing mesoscale architectures, MRS Bulletin 40 (11) (2015) 943-950. doi:10.1557/mrs.2015.235.
-
J. E. Trachtenberg, J. K. Placone, B. T. Smith, C. M. Piard, M. Santoro,
-
W. Scott, J. P. Fisher, A. G. Mikos, Extrusion-Based 3D Printing of Poly(propylene fumarate) in a Full-Factorial Design, ACS Biomaterials Science & Engineering 2 (10) (2016) 17711780. doi:10.1021/ acsbiomaterials.6b00026.
-
-
B. N. Peele, T. J. Wallin, H. Zhao, R. F. Shepherd, 3D printing antagonistic systems of artificial muscle using projection stereolithography, Bioinspiration & Biomimetics 10 (5) (2015) 055003. doi:10.1088/1748-3190/10/ 5/055003.
-
J. Goole, K. Amighi, 3D printing in pharmaceutics: A new tool for designing customized drug delivery systems, International Journal of Pharmaceutics 499 (1) (2016) 376394.
doi:10.1016/j.ijpharm.2015.12.071.
-
J. Y. Park, G. Gao, J. Jang, D.-W. Cho, 3D printed structures for delivery of biomolecules and cells: tissue repair and regeneration, J. Mater. Chem. B 4 (47) (2016) 75217539. doi:10.1039/C6TB01662F.
-
J. R. Dizon, A. H. Espera, Q. Chen, R. C. Advincula, Mechanical characterization of 3d-printed polymers, Additive Manufacturing 20 (2018) 4467. doi:10.1016/j.addma.2017.12.002.
-
R. D. Farahani, K. Chizari, D. Therriault, Three-dimensional printing of freeform helical microstructures: a review, Nanoscale 6 (18) (2014) 10470 10485. doi:10.1039/C4NR02041C.
-
W. Liu, J. Zhou, Y. Ma, J. Wang, J. Xu, Fabrication of PLA filaments and its printable performance, IOP Conference Series: Materials Science and Engineering 275 (2017) 012033. doi:10.1088/1757- 899x/275/1/012033.
-
An, Byeong Wan and Kim, Kukjoo and Lee, Heejoo and Kim, So-Yun and Shim, Yulhui and Lee, Dae-Young and Song, Jun Yeob and Park, Jang-Ung, High-Resolution Printing of 3D Structures Using an Electrohydrodynamic Inkjet with Multiple Functional Inks, Advanced Materials 27 (29) (2015) 43224328. doi:10.1002/adma.201502092.
-
A. Armillotta, M. Bellotti, M. Cavallaro, Warpage of FDM parts: Experimental tests and analytic model, Robotics and Computer- Integrated Manufacturing 50 (2018) 140152.
doi:10.1016/j.rcim.2017.09.007.
-
Y. Han, Influence of 3D printing infill patterns on the effective thermal properties, Journal of Purdue Undergraduate Research 6 (1) (2016) 89. doi:10.5703/1288284316207.
-
L. Baich, G. Manogharan, H. Marie, Study of infill print design on production cost-time of 3D printed ABS parts, International Journal of Rapid Manufacturing 5 (2015) 308. doi:10.1504/ijrapidm.2015.074809.
-
D. M. Kim, R. Viskanta, Heat transfer by conduction, natural convection and radiation across a rectangular cellular structure, International Journal of Heat and Fluid Flow 5 (4) (1984) 205213. doi:10.1016/0142- 727x(84)90053-5.
-
C. Deng, J. Kang, H. Shangguan, Y. Hu, T. Huang, Z. Liu, Effects of hollow structures in sand mold manufactured using 3D printing technology, Journal of Materials Processing Technology 255 (2018) 516523. doi:10.1016/j.jmatprotec.2017.12.031.
-
J. Haertel, G. Nellis, A fully developed flow thermofluid model for topology optimization of 3D-printed air-cooled heat exchangers, Applied Thermal Engineering 119 (2017) 1024.
doi:10.1016/j.applthermaleng.2017. 03.030.
-
J. Zhang, X. Z. Wang, W. W. Yu, Y. H. Deng, Numerical investigation of the influence of process conditions on the temperature variation in fused deposition modeling, Materials & Design 130 (2017) 5968. doi:10.1016/j.matdes.2017.05.040.
-
S. Salvador, P. Chan, Toward accurate dynamic time warping in linear time and space, Intelligent Data Analysis 11 (5) (2007) 561580. doi:10.3233/ida-2007-11508.