
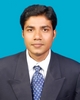
- Open Access
- Authors : S. Suresh , R. Karishma , Ch. Raju , N. Ravi Kiran , G. S. S. R. Chaitanya
- Paper ID : IJERTV9IS050734
- Volume & Issue : Volume 09, Issue 05 (May 2020)
- Published (First Online): 30-05-2020
- ISSN (Online) : 2278-0181
- Publisher Name : IJERT
- License:
This work is licensed under a Creative Commons Attribution 4.0 International License
An Empirical Analysis of Leaky Integrate and Fire Neuron Model
S Suresh
Department of ECE Raghu Institute of Technology
Visakhapatnam India
R Karishma
Department of ECE Raghu Institute of Technology
Visakhapatnam India
Ch Raju
Department of ECE Raghu Institute of Technology
Visakhapatnam India
G S S R Chaitanya
Department of ECE Raghu Institute of Technology
Visakhapatnam India
N Ravi kiran
Department of ECE Raghu Institute of Technology
Visakhapatnam India
Abstract: To evaluate the behaviour of neural networks, there are availability of the many bio-neuron model this leaky integrate and fire neuron paradigm features a vital role to play in assessing what the system a nervosum does how it interface and why it operates especially the inbuilt model may be a simple and effective devour neuron paradigm that's prevalently used and analyzed for the function of sub threshold and possible threshold of spike generation .when the dielectric potential penetrates some threshold voltage an nerve impulse is acquired but transitions actually correlated with the membranes voltage The article deals with the available calculation technique to elucidate the integrated neuron model while MATLAB simulation works.
Keywords: Neuron membrane, action potential, spike conduction, ionic current, kinetics
I.INTRODUCTION
The neuronal connectivity mechanism represents a critical step in the effective modeling of the human brain's high cognitive activity. A neuron gives details to other neurons through electrical pulses, known as action potential or spike[1,2].At synapse, a fluidic region between two adjacent neurons, neuron spic transforms to neuron spike. A pre-synaptic neuron electrical pulse is translated into a synapse chemical signal and then transferred to electrical signals on post-synaptic neurons [1,2].Dendrite receives sensory information and sends information to the cell process, which sends information to axon. The signal transmission is accomplished with the aid of a group of chemicals called neurotransmitters through a chemical synapse. When a threshold reaches the potential of soma, a spike is formed at axon hillock pre synaptic neuron. A typical Spike is an electrical pulse of maximum amplitude from about 100mV and a length of about 2-4ms[3].The resultant
spike moves through Ranvier's nodes on the axon of a pre- synaptic neuron and enters a pre-synaptic axonal termination. Na+ and K+ voltage-gated channels at Ranvier nodes will help restore growing node's spike. From node to node, a spike jumps. This spread of a spike through Ranvier's nodes is known as the saltatory lead. There is also a minimum time period, defined as total refractory duration (Tr)[4], of two consecutive spikes. Conduction of electrical impulses (action potential) along the axon is essential for advice transfer between neurons. Neuron structure is shown in below figure 1.
Fig 1: Neuron structure
-
INTEGRATE AND FIRE NEURON MODEL:
The integrated neuron paradigm is that the largest sort of implementations, and therefore the simplest paradigm to be used for neural network study and demeanour. Hill performed a more extensive analysis of integrate-and-fire neurons suggested by Lapicque in 1907 with injected current. The key insight into the neuronal activity of this method is that the period of time distinction of the relatively slow
integration of the sub-threshold and therefore the extremely fast spike generation, rather than being simple, this method was very useful though quick voltage changes are especially conventional during spike generation. The schematic model for a neuron's firing process describes the input only firing when the quantity reaches the edge.[5] ,as shown in below figure 2.
Fig.2. RC circuit model of the nerve cell membrane used in the LIF model.
-
Leaky integrate-fire neuron model:
Perhaps one of the most basic spiking neuron models is a Leaky Integrate-And-Fire (LIF) neuron, but still common because of its speed and ease for analysis and simulation. A neuron is modelled as a ' Leaky Integrator ' input I(t) .
In this instance, the input driven current can be divided into two components i.e., Resistance current and capacitance current as shown in eq.1 given below
() = + (1)
The given first component is the resistance current which passes through the linear resistor R. It can be calculated according to Ohms law as given eq.2
the membranes potential u(t) is reset to a specified threshold
(spiking level), and therefore the leaky integration process defined by Equation begins again with the initial value .For the steadiness of the LIF model to introduce only a touch complexity, you ought to add a totally refractory time abs ref just after u(t) reaches uth. During total refractory time u(t) might be pulled back to and the leaky phase of integration is started again after the spike is delayed by abs- ref The neuron is leaky because its cumulative inputs to the membrane potential degradation have a typical time constant. If this depletion in membrane potential is disregard over time, the model may be a great part. If there's a (fixed) level, the membrane capability hits a performance increase the incorporation and fire mechanism.
-
Refractory Period:
A refractory phase may be a duration when an organ or cell is unable to duplicate a particular act or (more accurately) the quantity of your time it takes for an excitable membranes to be ready for a second stimulation once the cell returns after excitement The biological time is described in two ways:(1) absolutely the biological time (2) The relative biological time.
the entire refractory duration is that the period during which a second nerve impulse cant be triggered, no matter the intensity of a stimulus. The relative refracting duration is that the period immediately after which a second potential for intervention is restricted, but not unlikely.
-
-
MAT LAB SIMULATION:
This segment explains how we implement our leaky MATLAB fire and integration simulation and which parameters we are using.
3.1 Building the Simulation:
The multiplex biological coordination is already one
=
(2)
single neuron. Yet neurons are, in theory, electrically
The second component charges the capacitor C. From the
activated cells, composed of a soma, a dendrite tree and an
definition of the capacitance C =
(Q is the charge and
axon. To construct a neural network, dendrites and axons
connect (by synapses). Therefore, for occurrence, to make a
is the voltage membrane), we find capacitive current as given below in eq.3
=
model, we will select geometry and certain rules that produce a neuronal relation law.
-
RESULTS:
= =
(3)
We clarify our leads to this division. We represents, and
The total input current is given by
() = +
(4)
describe the effects of this model, that our simulations have an entire biologic neuron interface. A leaky combine fire and
I(t) is multiplied by R and consider
the time constant
=
neuron model analysis.
Simulation 1: Subthreshold regmie / free solution = 0
of the leaky integrator. So the standard form is given by
eq.5
= ( ) + () (5)
Exponential decayto resting potential of the membrane. The growing the time constant, the further the probability of the membrane falls against the resting value for the membrane potential.
Where, u is the membrane potential
is the membrane time constant of the neuron
The eq.4 represents the linear differential equation of a leaky
integrator or RC circuit where resistance R and capacitance C are arranged in parallel. As per the neuroscientist the eq.4 is called the equation of a passive membrane.
This equation illustrates a basic resistance capacitor (RC) circuit, where the resistance is induced by the leakage term and I(t) integrating the condenser like the resistor The spiking incidents aren't directly created by the LIF model. instead of ,
Fig. 1a.Depolarization of the membrane. (flagS =1)
EFig.1b. Hyperpolarization of the membrane (flags=1)
Simulation 2: Subthreshold regime (pulse input)
Fig. 2. The product of a sequence of input pulses is a linear summation of the membrane reaction to each pulse. The membrane potential persists at a value below the potential level. There are no poles. (flags=1)
Simulation 3: Spiking neuron
Fig.3When the membrane potential exceeds its threshold value, an action potential is produced. The membrane potentiality is restored to the original voltage after the neuron has exploded. (flags=3)
Simulation 4: Spiking neuron with a step input
A step-input stimulus results in a normal fire of the neuron. The absolute refractory period is set at zero, while the refractory period in Figure 5b is set at zero is = 5. (flagS = 3).
Fig. 4a. Time evolution of the membrane potential and the external current input stimulus for an absolute refractory time other than zero. = 5 ig. 5a. Time evolution of the membrane potential and the reference signal for the external current. The total refractory time shall be at zero
Fig.4b. Time evolution of the membrane potential and the external current input stimulus for a non zero absolute refractory period. = 5 Simulation 5: A ramp input stimulus produces potentials for action with a rising firing rate as the input intensity increases (figure 6 flagS = 5)
-
CONCLUSION:
-
The merge and fire neuron model has proven useful to answer many of the questions regarding the knowledge processing of
neurons and neuronal networks. We should infer that we've transferred a spectrum of synaptic currents to the circuit. For instant, but that the spikes are sustained in size. Thus the actions and sophisticated properties of this neuronal model are combined and fired within the leaky neuron.
Fig.5a. Firing rate of neuron increases as strength of the input stimulus increases. The absolute refractory period is set to zero. = 0
td/> | |||||||||
Fig. 5b. Firing rate of neuron increases as strength of the input stimulus increases. A non zero absolute refractory period reduces the firing rate.
= 5
REFERENCES
-
Kandel ER, Schwartz JH, Jessell TM, Siegelbaum SA, Hudspeth AJ.Principles of Neural Science. New York, NY: McGraw-Hill; 2013.
-
Purves D, Augustine GJ, Fitzpatrick D, et al. Neuroscience. Sunderland, MA: Sinauer Associates, Inc; 2012.
-
Neishabouri A, Faisal AA. Axonal noise as a source of synaptic variability. PLoS Comput Biol. 2014;10(5):e1003615.
-
Dayan P, Abbott LF. Theoretical Neuroscience. Cambridge, MA: MIT Press; 2001.
-
Brillinger DR, Segundo JP (1979) Empirical examination of the threshold model of neuron firing Biol Cybern 35:213 220.