
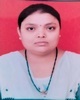
- Open Access
- Authors : Soni R Ragho , Rohit V Aute , Akash Ashok More , Rushikesh Prakashrao Mukdam, Rohit Rajendra Suryavanshi, Krushna Ramdas Tekale
- Paper ID : IJERTV12IS040013
- Volume & Issue : Volume 12, Issue 03 (March 2023)
- Published (First Online): 10-04-2023
- ISSN (Online) : 2278-0181
- Publisher Name : IJERT
- License:
This work is licensed under a Creative Commons Attribution 4.0 International License
Agromarketing & Crop Recommender System
1Soni R, Ragho 2Rohit Vijay Aute 3Akash Ashok More
4Rushikesh Prakashrao Mukdam5Rohit Rajendra Suryavanshi 6Krushna Ramdas Tekale
1,2,3,4,5,6 Guide, Student, Vidya Prasarini Sabhas College of Engineering and Technology, Lonavala, Savitribai Phule Pune University, Pune, Maharashtra, India
Abstract Agriculture and its allied sectors are certainly the biggest companies of livelihoods in rural India. agriculture is a main contributor to the Indian financial system. the common problem existing a few of the Indian farmers are they do not pick the proper crop based on their soil requirements. Precision agriculture is a current farming technique that makes use of studies information of soil traits, soil kinds, crop yield records series and suggests the farmers the proper crop based totally on their soil. This reduces the wrong desire on a crop and increases the productivity. And 2nd one is marketplace cost of crop. situation is worst sufficient which ends up in the suicide of farmers. farmers are nonetheless in down role to get benefits of theirpaintings. they're nonetheless no longer having any idea about theway to sale? the way to get right fee of their produce? they do not know the way to channelize their produce? and in the event that they face any losses then how to conquer from this? they do not know the state-of-the-art technology. so far, the betterment of farmers and ultimately for the agricultural development there's a want to know the significance of right marketing of agriculture produce and how the better advertising and marketing affects the farmers livelihood. it's far concluded that proposed set of rules has an average accuracy of 85on the given dataset. The executedaccuracy is extra in evaluation to existing paintings.
Keywords. Precision Agriculture, yield prediction, gadget getting to know.
-
INTRODUCTION
Agriculture has an in-depth history in India. recently, India is ranked 2nd in the farm output international [14]. Agriculture is the fundamental source of food supply of all of the countries of the arena whether underdeveloped, growing or maybe evolved. the world population is estimated to be approximately
9.7 billion with the aid of 2025. This delivered with unpredictable climate situations makes it tough to make certain food sustainability. fortunately, there may be a solution for this trouble as for many others. A farmer's choice about which crop to grow is typically clouded via his intuition and other inappropriate factors like making instant profits, lack of understanding approximately market demand, overestimating a soil's ability to assist a specific crop, and so on. a very misguided choice on the part of the farmer should region a enormous stress on his circle of relatives's economic circumstance. possibly this could be one of the many motives contributing to the limitless suicide instances of farmers that we listen from media on a day-by-day basis. We propose a device, an intelligent device that would bear in mind environmental parameters temperature, rainfall, geographical vicinity in terms of nation and soil traits soil kind and nutrients awareness earlier than recommending the maximum suitable crop to the user.
additionally, India is a worldwide agricultural powerhouse. Agricultural marketing in India still is still in a very horrific form in rural area. there is also the corruption that is growing now a day. Farmers didn't get proper advertising facilities so; they should depend on neighborhood buyers and middlemen for the disposal in their farm produce that's sold at very low priceby promoting agricultural marketing, we are able to offer many opportunities to farmers. The facilities that can be provide E-farming is one of the approaches in an effort to assist the farmers to perform the agro- advertising. affords privilege for each farmers and customers to shop for and promote the desired farm products without the involvement of a intermediary at its proper worthwhile fee. To beautify the percentage of farmers within the ultimate rate of his agriproducts. Soil is the free floor fabric that covers most land. Soil provides the shape help too flowers utilized in agriculture and also their supply of water and vitamins. Soil varies significantly in there chemical and physical residences. Leaching, weathering and micro real interest integrate to make maintain unique soil sorts. each type has unique power and weaknesses for agriculture production. In India, the Indian Council of Agriculture research (ICAR) has classified soils into 8 fundamental classes as in line with soil class gadget including a hierarchy of 6 tiers through Order, Suborder, extraordinary institution, Subgroup, own family and series. There are greater than 1400 notified soil collection in India. All soils given the equal soil series name possesses the identical characteristics throughout the panorama. unique kinds of crops may be efficiently grown on distinctive kinds of soils. We therefore need to know the features and characteristics of numerous soil sorts to understand the form of first-rate suitable crop. The changing environmental conditions, mainly international warming and weather variability, are primary issues and have an unfavourable impact on the destiny of agriculture output. Crop yield depends on many different factors such as climate, weather, soil, use of fertilizer, and seed range. while customer request to the farmer for precise crop at specific rate, the chatbot robotically displays the farmer information alongside their touch and information. The gadget collects the farmer, the crop cultivated in conjunction with land information and keeps a database. [21] Soil is the maximum crucial variable for any kind of crop production. every form of soil isn't best for every form of crop due to the fact distinctive soils have distinct varieties of properties suitable for different vegetation. as an instance, sandy soil needs a massive amount of water. subsequently, soil kind identification and crop choice are the number one steps earlier than starting any crop cultivation. traditionally soil types are labeled by means of the conventional technique of chemical evaluation that's time
consuming, tedious and high-priced technique. Human beings started the usage of statistical fashions to expect the crop kind and yield.
Fig1. Major Classification of Indian soils
-
RELATED WORK
Crop yield prediction is an essential task for the decision- makers at national and regionallevels (e.g., the EU level) for rapid decision making. An accurate crop yield prediction model canhelp farmers to decide on what to grow and when to grow. There are different approaches to cropyield prediction. This review article has investigated what has been done on the use of machine learning in crop yield prediction in the literature. During our analysis of the retrieved publications, one of the exclusion criteria is that the publication is a survey or traditional review paper. Those excluded publications are, in fact, related work and are discussed in this section.
The paper concludes that quick developments in sensing technologies and ML techniqueswill result in cost- effective solutions in the agricultural sector. Elavarasan et al. performed a surveyof publications on machine learning models associated with crop yield prediction based on climatic parameters. The paper advises looking broad to find more parameters that account for crop yield (Elavarasan et al., 2018). Liakoset al. (2018) published a review paper on the application of machine learning in the agricultural secor. The analysis was performed with publications focusingon crop management, livestock management, water management, and soil management. Li,Lecourt, and Bishop performed a review study on determining the ripeness of fruits to decide the optimal harvest time and yield prediction (Li et al., 2018). Mayuri and Priya addressed the challenges and methodologies that are encountered in the field of image processing and machine learning in the agricultural sector and especially
in the detection of diseases (Mayuri and Priya,). Somvanshi and Mishra presented several machine learning approaches and their application in plant biology (Somvanshi and Mishra 2015). Gandhi and Armstrong published a review paper on the application of data mining in the agricultural sector in general, dealing with decision making. They concluded that further research needs to be done to see how the implementation of data mining into complex agricultural datasets could be realized (Gandhi and Armstrong, 2016). Beulah perform eda survey on the various data mining techniques that are used for crop yield prediction and concluded that the crop yield predictioncould be solved by employing data mining techniques(Beulah, 2019). According to our survey of review articles, the significant ones of which are presented in this section, this paper is the first SLR that focuses on the application of machine learning in the crop yield prediction problem. The existing survey studies did not systematically review the literature, and most of them reviewed studies on a specific aspect of crop yield prediction. Also, we presented 30 deep learning-based studies in this article and discussed which deep learning algorithms have been used in these studies.
One of the early works developed a dedicated website to assess the impact of weather parameters on crop production in the identified districts of Madhya Pradesh [10]. The districts were selected on the basis of the region covered by the crop. Based on these criteria, the first five top districts with a maximum crop area were chosen. The basis of the crops selected for the study was on prevailing crops in the selected districts. The crops picked included maize, soybean, wheat and paddy, for which the yield for a continuous period of 20 years of knowledge, were tabulated. The accuracy of the established model ranged from 76% to 90%for the chosen crops with an average accuracy of 82%. Another important work checks the soil quality and predictsthe crop yield along with a suitable recommendation of fertilizers [10]. The Ph value and the location from the user were inputs used in this model. An API was used to predict theweather, temperature for the current place. The system used both supervised as well as unsupervised ML algorithms and compares the results of the two.
A classifier that uses a greedy strategy to predict the crop yield was proposed in [11]. A decision tree classifier that uses an attribute has been shown to yield better results. An ensemble model proposed suggests integrating the effects of different models, which has been shown to be typically better than the individual models. Random forests ensemble classification uses multiple decision tree models to predict the crop yield. The data are split up into two sets, such as training data and test data, with a ratio of 67% and 33%, with whichthe mean and standard deviation are calculated. This work alsoincorporates the clustering of similar crops to get the most accurate results.
Extensive work has been done, and many ML algorithms have been applied in the agriculture sector. The biggest challenge in agriculture is to increase farm production and offer it to the end-user with the best possible price and quality.It is also observed that at least 50% of the farm
produce gets wasted, and it never reaches the end- user. The proposed modelsuggests the methods for minimizing farm produce wastage. One of the recent works presents a model where the crop yieldis predicted using KNN algorithms by making the clusters. It has been shown that KNN clustering proved much better than SVM or regression [12].
-
MODELS AND METHODOLOGY
The solution proposed here aims to solve these limitations, by developing a user-friendly application that considers the parameters likerainfall, temperature, soil type etc. that directly affect cultivation. The Primary goal is to attain a higher type of plants that may be grow over the season. The propose device could assist to limit the difficulties faced via farmers in deciding on a crop and maximize the yield in effect to reduce the suicide rates [15].
The overall block diagram of the system is shown in modules. It takes in the preprocessed input image at the end and uses the pickled historical data sets of soil and crop type parameters to predict thesoil class and crop type.
Fig. 2 System Architecture
The abbreviation KNN stands for "k-Nearest buddies". it's far a supervised device mastering set of rules. The algorithm may be used to remedy both class and regression hassle statements. The variety of nearest neighbors to a brand-new unknown variable that must be anticipated or classified is denoted by way of the image 'ok'. allows take a terrific study a associated real-world state of affairs before we get began with this tremendous set of rules. we are often notified that you percentage many characteristics together with your nearest friends, whether it be your wondering procedure, operating etiquettes, philosophies, or other factors. As a result, we construct friendships with human beings we deem much like us. The KNN set of rules employs the equal principle. Its aim is to locate all of the closest neighbors around brand-new unknown facts factor in order to parent out what magnificence it belongs to. It's a distance-based technique. A refinement of the okay-NN category set of rules is to weigh the contribution of every of the okay associates in step with their distance to the query point xq, giving extra weight we to nearer buddies.
Its miles given by means of F(X) = Summation of (i=1 to K)Wi f(x) summation of (i=1 to ok) wi
Where the burden is wi = 1/d (xq, xi)
In case xq precisely matches one among xi, so that the denominator becomes zero, we assign F(xq) = F(xi).
CNNs are used for photo category and recognition because of its high accuracy. It terned into purposed by way of pc scientist Yarnn LeCun with in the past due 90s. when he changed into inspired from the human visual perception of spotting things. A convolutional nural community (ConvNet/CNN) is a deep gaining knowledge of set of rules which could soak up an enter Image, assign significance (learnable weights and biases) to diverse factors/items in the photo and have the ability to distinguish one from alternative. The preprocessing required in a convNet is a good deal lower compare to other class algorithms. Whilst in primitive strategies filters are hand-engineered, with enough training, ConvNets have the capability to analyze these filters/characteristics
Fig. 3 Farmers suicide sector graph for states
when you consider that this system mainly specializes in the production of natural food, it could change the records that are photograph there may be a clean image about the amount of minerals which can be used in the meals crop. this can degrade the satisfactory of the soil in addition to the crop's authentic first-class. This gadget provides the farmers with all of the inputs and thereby helps them in reducing the debts that they want to pay. when the input is provided, the farmer can cultivate and get his pay as properly. This encourages the future generations to live in
agriculture as it gives them with all comforts financially and psychologically. The figure 4 represents the number of deaths in India as according to the file of CSO in 2019.It clearly depicts that most of the problems confronted by using farmers are debt and water problems which this device can triumph over. The overall framework of this machine provides the farmers with input thereby decreasing the reasons for farmer suicide. also depicts the wide variety of farmer suicides together with the motives. Now as you can see, the difference in the income margin whilst the device is used against our system not being used is huge.
Fig. 4 Reasons for farmers suicide (Source: National crime record bureau 2019)
-
CONCLUSION
The Facility that can provide E-farming is one of the ways to which will help farmers to perform agromarkting. provides privilege for both farmers and consumers to buy and sell the required farm products without the involvement of a middlemen at its rights profitable price. To inhance share of farmers in the ultimate price of his agriproducts. The model has been examined via making use of specific styles of the system learning system set of rules. Bagged tree and okay-NNindicates precise accuracy but amongst all the classfiers, SVM has given the very best accuracy in the soil classification with much less time.it gives us more accuracy compare to exiting gadgets and gives more gain to farmers.The Solution will benefit farmer to maximize productivity in agriculture, reduce soil degradation in cultivated fields, and reduce fertilizer use in crop production by recommending the right crop by considering various attributes. This would provide a comprehensive prediction on the basic of geographical, environment and economic aspect.
The device may be prolonged to the cell software to help the farmers by using uploading the image of agriculture place. The efficiency of pre-processing is confirmed by using the amount of unwanted data (like leaves, grass and other stuffs) present in it. Due to this undesirable information gift inside the enter image, each for the duration of education and classification, the pre-processor fails to pick out the precise counters, as result failing to carry out with advance performance. the parameter for the picture like climatic aspect, moisture and beyond dataset can be use to accept to yield of crop. The series of extra
legitimate info of soil class, range longitude and suitable crop significantly accelerate the performance of work. The pre-processing unit may want ro for these reasons to improved and loads more features may be extended, as a consequence considerably contributing closer to the rural welfare international.
-
ACKNOWLEDGEMENT With immense pleasure, we present a
report on AGROMARKETING AND CROP RECOMMENDATION USING MACHINE
LEARNING as part of the curriculum of B.E. Computer Engineering. We wish to thank all the people who gave us an unending support right from the stage the idea was conceived. We are thankful to our Project Guide Prof. Soni Ragho, for her great support throughout the course of this project. We are also thankful to our Project Coordinator for conduction of project activity. We also thank all to those who have directly or indirectly guided and helped in preparation of this Project.
REFERENCES
[1] Umamaheswari S, Sreeram S, Kritika N, Prasanth DJ, BIoT: Blockchain-based IoT for Agriculture, 11th International Conference on Advanced Computing (ICoAC), 2019 Dec 18 (pp. 324-327). IEEE. [2] Jain A. Analysis of growth and instability in the area, production, yield, and price of rice in India, Journal of Social Change and Development, 2018;2:46-66Manjula E, Djodiltachoumy S, A model for prediction of crop yield International Journal of Computational Intelligence and Informatics, 2017 Mar;6(4):2349-6363. [3] Sagar BM, Cauvery NK., Agriculture Data Analytics in Crop Yield Estimation: A Critical Review, Indonesian Journal of Electrical Engineering and Computer Science, 2018 Dec;12(3):1087-93. [4] Wolfert S, Ge L, Verdouw C, Bogaardt MJ, Big data in smart farming a review. Agricultural Systems, 2017 May 1;153:69- 80. [5] Jones JW, Antle JM, Basso B, Boote KJ, Conant RT, Foster I, Godfray HC, Herrero M, Howitt RE, Janssen S, Keating BA, Toward a new generation of agricultural system data, models, and knowledge products: State of agricultural systems science. Agricultural systems, 2017 Jul 1;155:269-88. [6] Johnson LK, Bloom JD, Dunning RD, Gunter CC, Boyette MD, Creamer NG, Farmer harvest decisions and vegetable loss in primary production. Agricultural Systems, 2019 Nov 1;176:102672. [7] Kumar R, Singh MP, Kumar P, Singh JP, Crop Selection Method to maximize crop yield rate using a machine learning technique,International conference on smart technologies and management for computing, communication, controls, energy, and materials (ICSTM), 2015 May 6 (pp. 138-145). IEEE. [8] Sriram Rakshith.K, Dr.Deepak.G, Rajesh M, Sudharshan K S, VasanthS, Harish Kumar N, A Survey on Crop Prediction using Machine Learning Approach, In International Journal for Research in Applied Science & Engineering Technology (IJRASET), April 2019, pp( 3231- 3234) [9] Veenadhari S, Misra B, Singh CD, Machine learning approach for forecasting crop yield based on climatic parameters, In 2014 International Conference on Computer Communication and Informatics, 2014 Jan 3 (pp. 1- 5). IEEE. [10] Ghadge R, Kulkarni J, More P, Nene S, Priya RL, Prediction of crop yield using machine learning, Int. Res. J. Eng. Technol. (IRJET), 2018 Feb;5. [11] Priya P, Muthaiah U, Balamurugan M, Predicting yield of the crop using machine learning algorithm, International Journal of Engineering Sciences & Research Technology, 2018 Apr;7(1):1- 7. [12] S. Pavani, Augusta Sophy Beulet P., Heuristic Prediction ofCrop Yield Using Machine Learning Technique, International Journal of Engineering and Advanced Technology (IJEAT), December 2019, pp (135-138)
[13] https://web.dev/progressive-web-apps/ [14] https://www.wikipedia.org/ [15] Plewis I, Analyzing Indian farmer suicide rates, Proceedings of the National Academy of Sciences, 2018 Jan 9;115(2): E117. [16] Nishant, Potnuru Sai, Pinapa Sai Venkat, Bollu Lakshmi Avinash, and B. Jabber. "Crop Yield Prediction based on Indian Agriculture using Machine Learning." In 2020 International Conference for Emerging Technology (INCET), pp. 1-4. IEEE, 2020. [17] Kale, Shivani S., and Preeti S. Patil. "A Machine Learning Approach to Predict Crop Yield and Success Rate." In 2019 IEEE Pune Section International Conference (PuneCon), pp. 1-5. IEEE, 2019. [18] Kumar, Y. Jeevan Nagendra, V. Spandana, V. S. Vaishnavi, K. Neha, and V. G. R. R. Devi. "Supervised Machine learning Approach for Crop Yield Prediction in Agriculture Sector." In 2020 5th International Conference on Communication and Electronics Systems (ICCES), pp. 736-741. IEEE, 2020. [19] Nigam, Aruvansh, Saksham Garg, Archit Agrawal, and Parul Agrawal. "Crop yield prediction using machine learning algorithms." In 2019Fifth International Conference on Image Information Processing (ICIIP), pp. 125-130. IEEE, 2019. [20] Bang, Shivam, Rajat Bishnoi, Ankit Singh Chauhan, Akshay Kumar Dixit, and Indu Chawla. "Fuzzy logic-based crop yield prediction using temperature and rainfall parameters predicted through ARMA, SARIMA, and ARMAX models." In 2019 Twelfth International Conference on Contemporary Computing (IC3), pp. 1-6. IEEE, 2019. [21] Usha kiruthika, c.j raman, kanga subha RAJA Subhamanian,. "EAgriculture for Direct Marketing of Food crops Using Chatbots." In 2020 SRM Institute of Science and Technology,pp. 125-130. IEEE, 2020. The neame of