
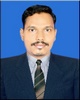
- Open Access
- Authors : Sathish S
- Paper ID : IJERTV11IS010201
- Volume & Issue : Volume 11, Issue 01 (January 2022)
- Published (First Online): 04-02-2022
- ISSN (Online) : 2278-0181
- Publisher Name : IJERT
- License:
This work is licensed under a Creative Commons Attribution 4.0 International License
A Study on COVID-19 Pandemic of Statistical Prediction in India
Sathish S
Assistant Professor, School of Mathematics and Statistics, MIT-WPU, Pune-38.
Abstract:- The proposed method is to develop a chi-square goodness of fit for the COVID-19 pandemic scenario and analyse the increased testing rates in India and the significant enhancement of the COVID-19 medical sector. India has a population of over 1.39 billion and is extremely densely populated compared to other parts of the world, current male population 51.6% and Male population 48.4% where the outcome is analyzed in overall poor economic status all have posed a major challenge to Indias first against COVID-19 hotspot; the scenario estimates RMSE, MAE of present scenario of the pandemic in India to identify the most suitable approaches for forecasting trend analysis.
Keywords: Regression analysis; Seasonal periods; Chisquare test; Social distancing and Lockdown
-
INTRODUCTION
The year 2020 has been a disastrous year for humankind. We humans, all around the globe have come across the Coronavirus. India witnessed an outbreak of COVID-19, during the last week of January 2020 when a few Indian students traveled to Kerala from Wuhan located in China. In 2020, from January to November to date, we have not been able to get rid of the virus. As per the World Health Organization (WHO), numerous potential COVID-19 antibodies are being examined, and many voluminous clinical trials may report their results later at the near end of 2020 or the very beginning of 2021 [1-7]. WHO is working with partners around the world to help coordinate with the key steps in this process. Companies such as Pfizer and BioNTech have concluded a phase 3 study of the COVID-19 vaccine and claim to be 95% efficient against the virus [8-11]. How the epidemic in India will top or decrease is foremost concerning the issue. Therefore, it is pivotal to predict the trends of the pandemic, nationwide. With this view of helping the Government, we undertook this research to aid them in making informed decisions about the spread of coronavirus thereby taking precautionary measures. For this, we have analyzed Indias COVID-19 dataset using regression models with supporting empirical evidence including error analysis and accuracy juxtapositions. Also, we have forecasted the trend of coronavirus cases using the Time Series Forecasting approach of Tableau. These methods are applied for four different types of cases: Confirmed, Active, Cured, and Death Cases as available in [11-13].
-
PRESENT SCENARIO OF COVID-19 IN INDIA
The distribution of the data was examined using the most up- to-date Covid-19 weekly case data and its parameters were obtained according to the statistical distributions. The performances of the methods were compared according to the
RMSE, APE, MAPE. According to experts, the third phase of COVID-19 is unavoidable but it is not clear on what time scale this phase three will occur. Since COVID-19 first emerged, various trend analysis studies have been conducted. In study of various trend analysis, prediction and simulation and management of COVID-19 cases. The first case of COVID-19 in India was reported on January 30, 2020; the index patient was a student who had returned from Wuhan. Thereafter, only 2 more cases were reported in February. Subsequently, more cases came to the forefront in the month of March and there has been a surge in the number of cases since the latter half of April 2020
-
METHODOLOGY
-
Chisquare test model recommended as the standard statistical model for the COVID-19 applications.
-
RMSE Estimation is the best prediction using parameters of COVID-19 data sets.
-
Coefficient determination of R2 model is rely heavily on autocorrelation patterns which is used to capture the forecasting effectively.
-
Statistical best prediction is Mean, SD, RMSE,
MAE, R2 and Goodness of fit
-
To predict covid 19 cases using statistical parametric approach
-
To generated covid 19 data
-
To estimate of confirmed cases, new and death cases in India.
-
-
RESULTS AND DISCUSSION.
TABLE 1: REGRESSION MLP 2-5-1 CONFIRMED CASES
Data Mean
16065690
Data S.D.
5739675
Error Mean
44655
Error S.D.
1521923
Abs E. Mean
704489
S.D. Ratio
0
Correlation Coefficient
0.99
TABLE 2: COMPARISON OF CORRELATION COEFFICIENT
Results
Correlation (Confirmed and New cases)
Correlation (Confirmed and Death cases)
SUM= Confirmed cases
2024906492
14889018.32
SUM= New cases
17519851
128822.4338
SUM=Death cases
179802
1322.073529
Figure. 1 . Comparison of Confirmed cases and Chisquare
Figure. 2 . Moving average Covid 19 cases prediction
Table 1 It is clear that the details of Regression model Confirmed cases of Observed and Predicted values, which shown the India is identified by using COVID-19 data. A good forecasting method Death cases of Observed and Predicted values Table 2. Comparisons The growth stage was identified statistical approach of confirmed and Death cases using independent variables and dependant variables by forecasting methods.
Figure. 3 . Observed confirmed cases on Covid 19 cases
A forecast analysis can be described as the ability of a model to predict with accuracy Comparison of Confirmed cases and Chisquare in Fig 1. Statistical moving average and observed confirmed cases shows in Fig. 2. And Fig. 3
-
CONCLUSION
The Government of India and the health care providers have been relentless in their efforts. The citizens must also help support the fight against the pandemic by adhering to government advisories of containment and social distancing. Study can assist government authorities to reduce the impacts of the pandemic. This technique can forecast in advance the number of potential confirmed cases, new, and death cases using basics statistical distribution.
REFERENCES
-
"World Health Organization," 7 April 1948. [Online]. Available: https://www.who.int. [Accessed 17 September 2020].
-
"Pfizer," Pfizer, 18 November 2020. [Online]. Available: https://www.pfizer.com/news/press-release/press-release detail/pfizer- and-biontech-conclude-phase-3-study-covid-19-vaccine. [Accessed 19 November 2020].
-
"PRS legislative research," 12 March 2020. [Online]. Available: https://prsindia.org/covid-19/cases. [Accessed 21 August 2020].
-
S. Tiwari, S. Kumar and K. Guleria, "Outbreak Trends of Coronavirus Disease2019 in India: A Prediction," Disaster Medicine and Public Health Preparedness, vol. 14, no. 5, pp. 1-6, 2020.
-
P. Wang, X. Zheng, J. Li and B. Zhu, "Prediction of epidemic trends in COVID-19 with logistic model and machine learning technics," Chaos, Solitons and Fractals, vol. 139, no. 110058, pp. 1-7, 1 July 2020.
-
M. Yadav, M. Perumal and M. Srinivas, "Analysis on novel coronavirus (COVID-19) using machine learning methods," Chaos, Solitons and Fractals, vol. 139 no. 110050, pp. 1-12, 2020.
-
A. Tomar and N. Gupta, "Prediction for the spread of COVID-19 in India and effectiveness of preventive measures," Science of the Total Environment, vol. 728, no. 138762, pp. 1-6, 20 April 2020.
-
D. Parbat and M. Chakraborty, "A python based support vector regression model for prediction of COVID19 cases in India," Chaos, Solitons and Fractals, vol. 138, no. 109942, pp. 1-5, 2020.
-
S. Rath, A. Tripathy and A. R. Tripathy, "Prediction of new active cases of coronavirus disease (COVID-19) pandemic using multiple linear regression model," Diabetes & Metabolic Syndrome: Clinical Research & Reviews, vol. 14, no. 5, pp. 1467-1474, 2020.
-
R. Tamhane and S. Mulge, "Prediction of COVID-19 Outbreak using Machine Learning," International Research Journal of Engineering and Technology, vol. 7, no. 5, pp. 5699-5702, 2020.
-
A. Aggarwal, A. Rani, P. Sharma, M. Kumar, A. Shankar and M. Alazab, "Prediction of Landsliding using Univariate Forecasting Models," Internet Technology Letters, no. e209, pp. 1-6, 2020.
-
F. Pedregosa, G. Varoquaux, A. Gramfort, V. Michel, B. Thirion and
O. Grisel, "Scikit-learn: Machine Learning in Python," Journal of
Machine Learning Research, vol. 12, pp. 2825-2830, 2011. 2020. [Online]. Available: https://www.tableau.com/. [Accessed 10 September 2020].
-
P. Arora, H. Kumar and B. K. Panigrahi, "Prediction and analysis of COVID-19 positive cases using deep learning models: A descriptive case study of India," Chaos, Solitons and Fractals, vol. 139, no. 110017, pp. 1-9, 2020.