
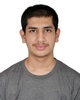
- Open Access
- Authors : Handa Arush
- Paper ID : IJERTV11IS090002
- Volume & Issue : Volume 11, Issue 09 (September 2022)
- Published (First Online): 13-09-2022
- ISSN (Online) : 2278-0181
- Publisher Name : IJERT
- License:
This work is licensed under a Creative Commons Attribution 4.0 International License
A Review of the Employability of Artificial Intelligence in the Contemporary Entertainment Industry
Handa Arush
Class XII, Bhavan Vidyalaya, Chandigarh
Abstract:- The process of filmmaking involves many complex and discrete stages, starting with an initial story and continuing through screenwriting, casting, pre-production, shooting, sound recording, post-production, and screening the finished product before an audience, followed by distribution and release. Each of these areas can leverage the advancements in the field of Artificial Intelligence (AI). The objective of this research paper is to explore the existing applications of AI in various stages of the film production process, drawing upon a range of journals, articles, and papers from a variety of disciplines to demonstrate the potential of AI in the future of film production. The article also sets out possible research agendas for the future. The study focuses on the adaptation of AI technologies contributing to the film industry. Data collected is the secondary data that is from various online websites and research articles to gather information about the uses of AI to show patterns, trends, and user preferences. Many technologies have been implemented in various areas of filmmaking: Generative Pre-Trained Transformer 3 (GPT 3) holds a lot of promise in the area of script writing. The latest buzzword in computer imagery is the deepfakes. These are created when artificial intelligence (AI) is programmed to replace a particular persons facial features with another in a recorded video. Scouting sites, casting actors, and other activities such as preparing the filming schedule, all can be made more efficient with AI.
Keywords:- Artificial Intelligence, Generative pre-trained transformer 3, deepfake, automatic subtitling, AI in script writing, AI in pre-production. AI in movie editing, AI in film making.
-
INTRODUCTION
Artificial intelligence lets machines imitate the capabilities of the human mind. In simplest terms, it is the simulation of the human mind processes by machines. Britannica defines Artificial Intelligence (AI) as the ability of a digital computer or computer-controlled robot to perform tasks commonly associated with intelligent beings (1). From the development of self-driving cars to the creation of smart assistants like Siri and Alexa, AI has come a long way. AI has been successfully applied in the areas of education, medicine, space, natural language processing, robotics etc. The field of entertainment and media is also not untouched by AI today. Film making, though a creative pursuit, is heavily dependent on technology, and with the inroads that AI has made into every aspect of technology, the day is not far ahead when AI entrenches itself fully in the filmmaking process.
-
AI in Script Writing
A movie script, or a screenplay (teleplay for a television script), is the foremost building block of a film. It lays foundations for how a movie will be defined, how different scenes will play out, how one part of the movie will transition into another, and when and where plot twists might pop up. The screenwriters colossal responsibility, and the sheer work that goes into it is often overlooked by the audience. Dictated by a rigid, professional schedule, screenwriters can take anywhere between two and six months to produce a reasonably good story. The process of writing scripts has been ameliorated by the rapid advancements in technology. Artificial Neural Networks and Deep Learning are leading the way when it comes to getting machines to produce human-like results. Drastically increasing the efficiency of the process, different technologies have been able to establish their functionality and effectiveness in producing stories.
In 2019, comedian and writer Keaton Patti used an AI bot to create a Batman movie script. He posted the first page of the script, and it went viral as it was very comical. In 2020, a company named Calamity AI wrote the screenplay for a three-and-half-minute short film using Shortly Read, an AI tool built on GPT-3. The speedy progress in NLP and large language models have helped make the process of screenplay writing adventurous and easier. However, a full- length movie entirely created by Artificial Intelligence is still a distant reality (2).
Platforms such as ScriptBook created AI technology that is able to analyse and comprehend screenplays to assist AI to become a co-creator in storytelling in a tale of co-creation between man and machine (3). An important milestone by ScriptBook is the 2016 short film Sunspring which claimed to be the first ever film entirely written by AI. The AI model, named Benjamin, was trained on movie scripts from the 1980s and 1990s. The AI used a recurrent neural network to create the script. The movie, starring Thomas Middleditch, made to the top ten chart at the Sci-Fi London film festival. Yet, despite this initial success, the content of the script itself was disarrayed and disjointed, showing a colossal lack of context and awareness of situations and characters (3).
-
Generative Pre-Trained Transformer 3 (GPT-3):
GPT-3 is an autoregressive language model that uses deep learning to produce human-like text. It has been
developed by the Elon Musk (and others)-founded OpenAI and is the third instalment in the organisations GPT-n series, which has taken Natural Language Processing (NLP) to unprecedented heights. Before the advent of GPT-1, most NLP systems were made largely for specific tasks like textual entailment sentiment classification etc. under supervised learning.
This model uses deep learning to construct human-like text. It is one of the leading technologies today in the field of Natural Language Processing (NLP). Autoregressive process is the process in which current value is based on the immediately preceding value. It is an auto-complete program that predicts what could come next. GPT-3 processes a large data bank of English sentences. Then, an extremely powerful computer model called neural nets detects patterns and determines its rules of language functions. GPT-3 has 175 billion learning parameters, which enables it to perform almost any task assigned to it, making it the most powerful language model today. In order to come up with sentences, it employs semantic analytics to study words and their meanings, and also to understand how the use of words varies. It also takes into account other words used in the text.
GPT can be designed to produce the desired text output, and this is, quite surprisingly, not difficult at all. GPT-3 is fed with inputs which can even be done by pasting text from a Wikipedia article. The most important aspect of training the AI engine is teaching it what type of text to generate by giving it examples. In many cases a single example is sufficient, but one can provide more.
One of the other important settings to regulate the output of the GPT-3 engine is the temperature. The model would produce more random answers to your questions if you switched up that temperature. An AI model will offer deterministic and repetitive answers at a low temperature. Products like Siri and Google Assistant give foreseeable and expected responses. A zero value makes the engine deterministic, which implies that a given input text will always generate the same output. A value of 1 makes the engine take the most risks and use a lot of creativity. In addition to playing with the temperature setting, one can provide plot prompts and character outlines to the GPT-3 model. With only a few prompt lines, the model can return a formatted film scriptin a few moments, creating characters with names set in a location. The Frequency Penalty and Presence Penalty options allow us to control the level of repetition GPT-3 is allowed in its responses. Frequency penalty works by lowering the chances of a word being selected again the more times that word has already been used. Presence penalty does not consider how frequently a word has been used, just if the word exists in the text. The difference between these two options is subtle, but one can think of Frequency Penalty as a way to prevent word repetitions, and Presence Penalty as a way to prevent topic repetitions (4).
The question of where the model gets its information from also has to be considered more deeply. Writing by women, and from people outside the Western screenwriting system, will be necessary to create a fairer engine. On a creative level, this is the key. To avoid repeating the past patterns,
one needs to be mindful to include a kind of internationalism, or at least a broader scope of the inputs to the AI (5).
-
Developments in the Field
In the music industry, AI is being tested to create both instrumentals and lyrics. Now an entire song in ones favourite artists voice can be generated using AI. In 2021 a company called Over the Bridge created a new Nirvana song using the works of Kurt Cobain and other members of the band Nirvana.
Films like Scraps of Mechanical Souls are being written by using AI technology exclusively. Surprisingly, brilliantly, and scarily enough, these scripts contain human-like emotional writing, describing love, pain, joy, and fear. Scraps of Mechanical Souls showcases AIs capability to write effective and working scripts, but apart from that, it is a beautiful watch because the viewers watch the future unravel before their own eyes. Although there are imperfections such as repetition, the very nature of AI is that it is keen on improving itself. Jacob Vaus & Eli Weiss, co- founders of Calamity AI, also recently tested the capability of GPT-3 to generate scripts and in the process, created Date night. They used software called Shortly AI, which is especially marketed to people anguishing from writers block. They found that, creepily enough, the characters often reorient themselves and even talked about the movie itself, talking about prior, fictional events.
DALL-E (also created by Open AI) is another AI program that creates images from textual descriptions. It can combine concepts, attributes, and styles. It can also make accurate edits to existing images from a natural language caption. It can also add and remove elements while taking shadows, reflections, and textures into account. Beyond this, it can create different variations of an image inspired by the original. It uses a 12-billion parameter version of the GPT-3 Transformer model to interpret natural language inputs and generate corresponding images.
Dall-E 2 has learned the relationship between images and the text used to describe them. It uses a process called diffusion, which starts with a pattern of random dots and gradually changes that pattern towards an image when it recognizes specific aspects of that image.
However, the mind of AI is unpredictable, so human input is needed when training this skill. Keaton Patti used AI to generate a Batman script- the bot did not use the same language that Keaton used. The AI wrote hilarious scripts. However, it was observed that you still need human input to make it work.
-
-
ARTIFICIAL INTELLIGENCE IN CHARACTER MAKING
The latest advancement in computer imagery, deep fakes are created when artificial intelligence (AI) is programmed to replace a particular persons facial features with another in a recorded video.
The term "deepfake" comes from the technology on which it is based, that is, "deep learning," which is a form of AI. Deep learning algorithms, which teach themselves how to solve problems when given large sets of data, are made use of to
swap faces in video and digital content to make realistic- looking media that is fake.
The essential requirement for creating a realistic video using such technology is a huge amount of input to train the machine on a persons expressions and movements. The more information the machine can have access to and learn from, the more realistic the result will be. For example, an impersonator acting as several famous Hollywood actors used Deepfake to map their faces onto his face in order to make a short video. He claimed that he used 1,200 hours of footage, 300,000 images and 250 hours of work to achieve a realistic output.
-
A.Deepfake characters
There are many uses of deepfake in the entertainment industry.
-
Deepfakes can keep film characters consistent. For example, consider that an actor has passed away. Deepfake technology can fill the role recreating the likeness of unavailable actors. So, the character doesnt have to pass away with their actor. For example, the recreation of the late Peter Cushing in Star Wars: Rogue One (2017), who passed away in 1994. Similarly, it can be used to edit scenes where an actor is not able to participate due to scheduling conflicts or any unfortunate mishaps (6).
Figure 1: Recreating Peter Cushing as Grand Moff Tarkin.
Source: YouTube
-
Deepfake technology is also useful in situations when a character needs to be older or younger than their actor. For example, the late Carrie Fishers character, Princess Leia. Though the actress herself was not available, her young likeness was recreated. This shows another positive use of deepfake technology: ageing and de-ageing of characters.
-
The technology can also make the movement of lips and facial expressions sync with the lines the actor is saying. This is useful in dealing with problems like censorship or when there is a problem in sound recording during production.
-
Deepfakes can make language barriers a thing of the past. For instance, the David Beckham malaria announcement. By using AI technology, David Beckham was shown to speak in nine different languages in order to share a message for the Malaria Campaign. This will go a long way to improve the diversity of our entertainment and make the whole world a single audience. Deepfake can thus be used for dubbing in multiple languages, reducing the cost of hiring local voice actors besides creating a more realistic experience for international audiences (6).
-
Because deepfakes can replicate voices and change videos, it helps to showcase translated films that use the original actors. The voices sound like the original ones; moreover, the lip movements even match the words spoken.
-
It can also be used for turning black and white films into colour. Though regarded as a visual tool, deepfake technology can also be used with audio.
-
A possible future use of this technology can be replacing actors completely with the help of Deepfake using an impersonator, further cutting studio costs. This has the potential to even create new actors, where producers can choose elements they like from different actors, for example the body movement of one, facial expressions of another and the voice from still another, creating an actor that is completely computer generated. This idea could thus be any directors dream, eliminating the high cost of established actors.
-
Deepfake technology holds positive potential for education. It could revolutionise our history classes with interactivity. It could preserve stories with deepfake examples of figures from history. For example, in 2018 the Illinois Holocaust Museum and Education Centre created hologrammatic interviews. Visitors could talk to, ask questions and hear stories of Holocaust survivors. As deepfake technology advances, this kind of virtual history could become a reality in our classrooms (6).
Figure 2: Students interacting with artificially rendered Holocaust survivors. Source: Youube
Another example comes from Cere Proc, a company that resurrected JFK in voice. This deepfake made it possible to hear the late President deliver the speech he would have delivered but for his assassination (6). In this way, deepfake technology could help us preserve not just historical facts, but also feel the impact historical events had on real people.
-
In 2020, several cases of politically motivated deepfake videos made an appearance on the Indian news space. During the Legislative Assembly elections in Delhi, two videos surfaced, showing the leader of the Delhi Bhartiya Janata Party (BJP), Manoj Tiwari, addressing his people in two languages: English and Haryanvi. According to VICE India, the videos were shared in nearly 6000 WhatsApp groups, reaching about 15 million people (Vice, 2020) (7). Manoj Tiwari does not speak Haryanvi, nor had he recorded the video message in English. An Indian PR company called The Ideaz Factory'' had taken an older video message of Manoj Tiwari where he spoke about a totally different topic in Hindi, trained an AI with videos of Manoj Tiwari speaking until it was able to lip synchronise arbitrary videos of him. Thereafter they used a voice artist to record the English and Haryanvi and merged both audio and video (7). Deepfake technology shows the power of Machine Learning. The more the training a machine has, the better it performs. The more the footage and photos, the more realistic Deepfake videos become. It is thus also clear as to why celebrities and public figures can be the perfect input
sources for this technology since there is a plethora of pictures and videos of them available online due to the public nature of their career.
-
-
The science behind deepfakes
The key element in creating realistic output from Deepfake technology is GANs, Generative Adversarial Networks, which is the idea of using two AI systems fighting against each other to continuously improve the quality of the result. One system functions as the forger, while the other one works as a detector. Together the two systems continuously try to overcome the other resulting in a more and more realistic result. By using this principle, Deepfake can create and self-detect their results over and over again to ultimately result in human-like effects. GANs are thus the key element in altering and creating new images based on the previous input. Generative and discriminative models are two different approaches to machines learning from input data. Although discriminative models can identify a person in an image, it is the generative models that can produce a new image of a person that has never existed before. Since their introduction, models for AI-generated media, such as GANs, have enabled the hyper-realistic synthesis of digital content, including the production of photorealistic images, cloning of voices, animation of faces, and translation of images. The GAN architecture consists of two neural networks, a generator and a discriminator. The generators task is generating new content that resembles the input data, while the discriminators task is to differentiate the generated or fake output from the real data. The two networks compete with each other in a closed-feedback loop, resulting in a gradual increase of the realism of the generated output. GAN architectures can produce images of things that have never existed before, such as human faces. However, StyleGAN is an example of a modifiable GAN that enables intuitive control of the facial details of generated images by separating high-specificity level attributes like the identity of a person from low-specificity level features such as hair or freckles. Researchers have also proposed an in-domain GAN inversion approach to enable editing of GAN-generated images, allowing for de-aging or the addition of new facial expressions to existing photographs. Besides, transformers such as the ones used in the massive generative GPT-3 language model are already being shown to be successful for text-to-image generation.
Figure 3: A younger version of actor Robert De Niro next to his current picture. Source: The CineRanter.
-
Disadvantages of this technology
-
Videos of celebrities like politicians and actors are being faked using such technology, showing them
indulging in illegal or anti-social or unacceptable activities.
-
It could threaten jobs for current actors making it difficult for emerging actors to gain success, as legendary actors can now be regenerated after their death. This has already happened in the franchise movie Star Wars. A more recent and controversial case is the idea to bring James Dean back to the screen as a new character in 2020. The movie called Finding Jack is an adaptation of Gareth Crockers novel. The story is set in wartime Vietnam and is about a group of soldiers who refuse to leave their dogs behind. Dean enacted a character named Rogan, the second lead of the story. His character was crafted using Deans photographs and footage from earlier movies. The idea has caused fears within the entertainment industry. First, the production is not consensual, at least not from Dean himself, though his family already gave the approval to the production. Second, it is not about reviving the characters that he played but is about casting him in a completely new character as if he were still acting. Despite the argument of the producers that Dean is the perfect choice for the role, the necessity of casting him instead of using a living actor is controversial. This has many legal and moral consequences (8). To date, lots of deepfake parody videos have been created on video platforms like YouTube, where changing the main actors of a movie is the most common manipulation. For example, a short film named a reference to the 1990 comedy movie Home Alone features an 8-year-old AI-generated Sylvester Stallone instead of the real actor, Macaulay Culkin. Many similar videos of other stars are available on the internet (9).
-
-
-
AI IN PRE-PRODUCTION Filmmaking is a challenging field and 60 percent of
time spent creating movies goes into pre-production of the film before it goes into the production stage. Pre-production is a complicated task. Scouting sites, casting actors, and other activities such as preparing the filming schedule are all part of the job. Pre-production procedures can be made more efficient with AI.
-
Machine Learning and Artificial Intelligence algorithms help new age filmmakers in producing new scripts or to construct synopsis and character names for movies that have previously been produced. A Machine Learning algorithm is fed a plethora of data in the form of several movie scripts or a book that is to be adapted into a movie to generate a new script. The AI program learns from the data and generates new scripts. Alternatively, it can also analyse, comprehend, and arrange a books story to create its own version of a screenplay incorporating key plot points.
-
AI can aid with the planning of shooting schedules and other pre-production activities; it can also look at the availability dates for different actors and
create the schedule accordingly. As a result, the filming schedule can be set to maximise efficiency. AI technology can recognize locales depicted in scripts and screenplays because it has been taught to understand them. It can then recommend real- world sites where the scene could be shot which would eventually save a significant amount of time. In addition, the method can be used for casting. Producers and casting directors can use AI to assist them with finding the perfect actors. Actors past performances can be analysed using AI systems. It has the ability to examine the locations where their films were successful, and the actors have a large fan base. Producers can then tailor their promotions and marketing strategies to fit their needs.
-
Also, AI can be leveraged in the analysis of individual scripts that will be made into motion picture. The algorithm can undertake analysis of the script and create its own questions and doubts. This saves up on valuable resources as the algorithm can undertake analysis of scripts much quicker than human beings. For example, upon analysis of a script, the AI algorithm can devise questions such as Why did the character speak this dialogue at this moment? or Why is this scene needed here? This can assist filmmakers with creating movies of high quality. The introduction of AI tools has already begun to massively transform the ways in which films are developed and researched prior to actual production. Over the past decade there has been a steady growth in AI tools that generate information from large data sets. A defining factor in the growth in these techniques has come from the success of platforms such as Netflix and its host of algorithms and ML tools which harvest the data of the services millions of users in order to create existing and future content. In collecting data from each user, Netflix is able to develop tools such as its coveted recommendation engine, a collection of algorithms which is informed by comparing user data profiles against each other to generate accurate personalised recommendations to its users. This engine valued by Netflix at one billion dollars is one of the companys crowning achievements. Each of the algorithms used in the engine relying on ML techniques stands as a testament to the transformative power of AI technology for the entertainment industry (10).
-
Producers and casting directors can use AI to assist them with finding the perfect actors. Actors past performances can be analysed using AI systems. The technology can also be utilised to develop digital characters or to de-age performers. This eradicates the requirement for casting several performers for the same role at the different phases of life. It also retains the integrity of the character as one actor plays the part, instead of several actors playing it, which could dilute their on-screen presence.
-
Artificial Intelligence may soon help in deciding whether or not a film is made in the first place. A Belgian AI company called Scriptbook developed an algorithm that the company claims can predict whether or not a film will be commercially successful just by analysing the screenplay, according to the magazine Variety (11). Recent examples include a partnership between Warner Bros. and Cinelytic, an AI-driven film management system that uses AI-powered predictive forecasting intelligence to achieve up to 85% accuracy before a film has been made. Having already partnered with Sony Pictures and multiple other Hollywood studios, Cinelytic is representative of AI technology looking to innovate workflows. Platforms of this nature are largely powered by ML and DL algorithms that analyse thousands of data points to generate insights. Another example is Vault, an AI platform similar to Cinelytic but with an additional focus on content analysis as well as market forecasts. Vault is not the only platform to utilise content analysis techniques in producing forecasts and predictions; another key example is Merlin Video a computer vision tool created by 20th Century Fox and Google Cloud. Merlin was conceived as a way for Fox to create precise segmented results as opposed to broad inaccurate predictions. The method chosen in this collaboration was to build a tool that can learn and interpret dense representations of movie trailers to help predict a specific trailers future movie-going audience (12), (13). Normally, script coverage is handled by a production house or agencys hierarchy of executive assistants and interns. To justify the huge expenditure over human labour, Scriptbook claims that its algorithm is three times better at predicting box office success than human readers. The company also asserts that it would have recommended that Sony Pictures not make 22 of its biggest box office flops over the past three years, which would have saved the production company millions of dollars.
-
The AI system can predict an MPAA rating (for example R, PG-13), detect who the characters are and what emotions they express, and also predict a screenplays target audience. The algorithm can also determine whether or not the film will include a diverse cast of characters.
-
Getting some help from an algorithm could help people ground their coverage in some cold, hard data before they recommend one script over another. While it may seem like this takes a lot of the creative decision-making out of human hands, tools like Scriptbook can help studio houses make better financial choices.
-
-
AI IN MOVIE EDITING
Okun et al. [Okun et al., 2015] define video editing as the act of cutting and joining pieces of one or more sources
together to make one edited movie. Mobile phones, video sharing, and social media platforms make it easier and quicker than ever to capture and publish videos. Editing those videos, however, is a different ball game altogether. Video remains a difficult medium to edit as it requires operation at individual frames and, at the same time, being a dual track medium with both audio and image, editing a video is very time consuming. Even in an unpretentious scene like characters looking at each other, the director can demand many shots with multiple camera angles. An editor creates a single lucid shot by skilfully adding and editing different film footage. It may take more than two hours to make a one-minute video through this process. However, with the use of AI, one can shorten the two-hour process to two minutes (14).
Machines can be programmed to choose the best shots from the footage already shot. IBM and 20th Century Fox had come together to create the trailer for Morgan with the help of AI (15). The machine was fed with hundreds of great trailers. It then learned from the footage and selected the ten best shots from the film footage. These ten shots were then edited to create an eye-grabbing trailer. Machines can make video edits like colour correction, image stabilisation, visual effects, and other vital edits.
One early example of such a tool is Silver [Casares et al., 2002] from 2002, which provides smart selections of video clips, as well as abstract views of video editing, by using metadata from the videos (16). A more recent example of an intelligent video editing tool is Roughcut [Leake et al., 2017] (17). Roughcut allows the computational editing for dialog driven scenes using user input of dialog for the scene, raw recordings, and editing idioms. There is an open-source tool AutoEdit [Passarelli, 2019] (18) which enables text-based editing of video interviews by linking text transcripts to the videos. Entirely AI-controlled video production has received a lot of research interest lately [Xue et al.; Hua et al., 2004] (19). Mashups, combining multiple video clips about a single event, is another type of automated video editing. The work named Virtual Director [Shrestha et al., 2010] (20) created a mashup generation method for concert recordings which maximises what makes a good concert video based on rules sourced from interviewing video editors and film grammar literature. These completely automated video editing methods, as used to create video summaries and mashups, are not considered intelligent video editing tools because they are simple algorithms that execute a very narrow and specific request that requires no intelligence or user interaction involved (19).
Even though most people are unaware of it, AI algorithms are behind most of todays amazing YouTube videos. A unique software built by Alphabets AI-focused Jigsaw group automatically modifies YouTube videos so that they become comprehensible to people from diverse regions and cultures. The program collects information about who all could be watching the movie and which portions of it, such as references or labels, might not be clear to them. It can then reorganise visual content to give more context and framewok to the situation, for people who are unversed with the language or who belong to cultures other than that of the original speakers.
Software like Adobes sensei is using these editing tools for an improved and efficient video editing experience and is also helping the editors to improve their skills at video editing. Usually, video editing is an intensive and laborious computer process that takes a lot of time. This software has greatly reduced the time it takes for the computer to finish editing footage.
Software like Adobe Premiere Pro CC automates repetitive and routine video editing tasks. The software also has an inbuilt automatic audio tool that lowers the volume of the background music to match the scene. It manages the bass, timbre, tone, and other audio aspects to provide the best audio to go with the video. One can use the software with automating colour correction, matching skin tone, controlling the music to go with the shot, and many other such functions. Adobe has set the bar high for AI in video editing. There are many software products like Quickstories that use AI to create high impact videos. One can automatically sync footage from GoPro to the device and start editing the footage. Using advanced learning algorithms, sensors, etc, it selects the best shots and seams them together to create short, impactful videos. It also recommends music to go with the footage. This AI tool can help Moto vloggers, YouTubers, TikTokers, etc. create high-quality professional-looking videos effortlessly.
Software like Magisto takes this approach a little further. One can use the software using AI to alter the video footage and photos into well-made videos that have a professional feel and look. It also has a feature where the software automatically lowers the volume of the background music when the characters are speaking. Using AI, this software is making the lives of content creators easier.
With the help of video editing tools like Rawshorts, one can create animated videos on the move. Once a person uploads the text or script for the video, the AI then automatically creates a storyboard using algorithms. You can select media assets that best go with the footage. Using the software, you can insert a voice over for the video too. You can then twist the rough outlines of the video and make changes to provide a finishing touch to the video. This software drastically reduces time and edits videos flawlessly.
In the near future, it is anticipated that more and more AI based intelligent editing tools will start emerging that use AI to interpret and make suggestions for video editing. With such tools, video editing will become easier for beginners, and professional users will be able to cut down their mundane and repetitive workload considerably by letting the AI handle most of such work.
-
AI IN SUBTITLING
Today, the idea of a film from South Korea becoming one of the biggest cultural talking points of the year, does not seem so unlikely. Squid Game (South Korean), Money Heist (Spanish), Lupin (French), and Who Killed Sarah? (Spanish) were amongst Netflixs most popular TV shows. The German-language sci-fi thriller Dark, had more than 50% of its audience international. Gen Z-ers are almost four times more likely than those aged between 56 and 75 to prefer subtitles over dubbing, despite those in the older bracket being twice as likely to be deaf or hard of hearing.
Many point to TikTok and Instagram, which regularly marries images with text, to explain younger peoples ease with subtitles (21).
Subtitles on television began in the early 1970s. Live subtitling, however, began in 1982 when it was developed by the National Captioning Institute in the USA using court reporters trained to write 225 words per minute using a stenograph machine. This provided viewers with on-screen subtitles within two to three seconds of the word being spoken. However, stenography as a profession has been in decline since 2013. At the same time the stenography profession began trending downward, the demands for live subtitling were rising.
-
Demand for subtitling
The reasons for the exponential surge in demand for subtitling are manifold.
-
Across the globe, from US ADA (Americans with Disabilities Act) to EU EAA (European Accessibility Act) and beyond, there is a legal obligation to offer accessible on-demand content. While the regulations are driven by the requirement for the hearing impaired according to the World Health Organisation, 430 million people suffer from disabling hearing loss the reasons why subtitling is so important are even more far reaching. Similar government mandates in multiple countries have come up.
-
Subtitles enable you to maximise your audience thanks to their ability to adapt to a range of contexts, audiences, and media and reach people you would not reach otherwise, for example, those who speak other languages. Several studies show that around 85% of people that view videos on Facebook view them with the sound off and research from Facebook itself claims that adding captions to your video can boost view time by 12%. A survey of US consumers even found that 92% view videos with the sound off on mobile. Streaming media like Netflix have been able to show international programmes across regions due to a good use of AI in generating subtitles.
-
There is exponential growth of live and breaking news, 24-hour cable news cycles, and more live sports broadcasts.
-
They are also becoming extremely useful when showcasing content (like ads, showreels etc.) in public places like waiting lounges in airports, hospitals, malls, etc where the audio is muted.
-
Additional competition for live captioners is also coming from corporate events, government briefings, meetings, and increased usage from the legal system for depositions and trials that are creating resource issues and rising prices for human captioning.
-
Recent experiments in India and a few other developing countries have proved that Same Language Subtitles (SLS) have improved reading literacy. SLS causes automatic, inescapable reading engagement even among weak readers, and
over a period of time has a bigger impact than conventional print media. Even developed countries plan to make SLS a default option for childrens content in order to help young viewers develop reading skills in their early years.
The best way to fill the gap between decline in human subtitling and an increase in market demand is reliance on technology, specially, Artificial Intelligence (AI). The technology has been around for many years. In fact, voice recognition dates back to the early 1900s. The technology began to show significant improvement beginning in the 1970s and continued to evolve into 2014 when it became commercially available. Early efforts suffered from accuracy problems and limitations.
-
-
Subtitling and automatic subtitling
A major part of the subtitling works these days consists of audio transcription, and this can be perfectly automated. However, subtitles are not just a transcript cut into pieces of text of some lines and some characters per line. It consists not only of translating a text from a source language into a target language, but it also involves a shift from oral to written language, removing language tics and incorporating nuance, natural audio breaks, and pauses.
There is extensive research focused on automatic subtitling, mainly through the use of Automatic Speech Recognition technology for the recognition and alignment tasks. Most of the work in the area has centred on improving recognition accuracy and producing well-synchronised subtitles. However, subtitling quality also depends on other parameters aimed at favouring the readability and quick understanding of subtitles, like correct subtitle line segmentation (22), (23).
Today many applications and software are available in the market which leverage AI to produce subtitles for the etertainment industry. Videolinq is changing how broadcasters create and distribute live video by offering an online workplace for teams to create live streams, engage viewers, and grow audiences on multiple social media platforms. Zeemo.AI is a cloud-based solution that helps businesses use artificial intelligence (AI) technology to automatically transcribe and add subtitles to videos. Users can import audio or video files on the platform and automatically generate subtitles in multiple languages including Chinese, English, Japanese, Russian, German, Vietnamese and more. Maestra is a speech to text solution that helps businesses across marketing, education and publishing industries streamline caption, speech recognition, and transcription. It enables users to convert audio files into text, configure workflows, and add subtitles in videos in real time (24).
AI may be better and faster at turning audio into timed text, and into cutting a transcript into properly segmented and timed subtitles, but it will need a little help for translating spoken language into more compact written language. This is called the expert in the loop workflow. It deals with regular expressions, slang, translating spoken language into written language, etc. Using AI and an expert in the loop workflow, one can save 80% or more time, while maintaining consistent high quality. When partial AI automation is used, the results are even better.
Automatic speech recognition nowadays works 4 times faster than real time and has a word error rate of 2-5% depending on the quality of the speech. This is critical: 2% results in a reasonable post-editing time (2 min per min), while 5% is the limit (6 min per min or more).
Screen subtitling systems can be used in multiple applications including:
-
Emergency Subtitling for those occasions when subtitles are expected to be present in a program but for some technical glitches they are not. Such software can help reduce viewer complaints because it can be activated in just a matter of seconds.
-
Supplemental Subtitling news may provide subtitles using a script and teleprompter, but for weather, sports, traffic and un-scripted field reports, rather than use a human who must be available and on the clock for the entire time, software can be turned on and off as needed.
The two biggest benefits one can expect are:
-
Improvements in accuracy A frequent comment heard is we tried AI several years ago and it wasnt very accurate. That was true then, but the technology has made excellent progress and accuracy has increased significantly depending on the program genre and the audio quality. In addition, it is easy to regionalize or localise the technology by using custom dictionaries to import regional or local names, geography, schools, sports teams, etc. Utilisation of these dictionaries will increase accuracy and improve pronunciations.
-
Substantial savings Human subtitlers are expensive. As the shortage of qualified subtitlers continues to decline, one will likely see an increase in rates. AI will take the space of humans at much economical rates (25).
-
-
Limitations of the use of Artificial Intelligence in subtitles
-
AI tools still have several limitations. When working on genres like mythology or content with considerable background noise, heavy accents, and high context content (like sarcasm or humour), the use of AI tools becomes challenging, and the results are hard to work with. Within text translations as well, complex sentences can result in gibberish. For example, when translating from Hindi to English, an experienced translator would translate the reference of romantic Indian duo Laila Majnu to Romeo and Juliet without batting an eyelid something a machine would be able to do only after considerable learning. Creativity plays an intrinsic part in translating content and generating impactful subtitles.
-
When it comes to subtitling, the context is as important as the content. While words like mom/mother can be used interchangeably, the usage of mother is more appropriate in the context of a religious mention, which the machine will not be able to decipher automatically. Similarly, there
are many common idioms and culture sensitive languages (Arabic for instance) which, when translated literally, yield hilarious and sometimes offensive results. AI tools tend to struggle with unclear contexts, new slangs, and specialised subjects that require a lot of research.
-
Does this mean subtitling will remain human- driven even with the advent of AI? It certainly will not, as machines start learning the social and cultural nuances and growing intelligence. There are many areas where automation can help reduce manual effort and increase speed right away. Examples include timecode shifting, workflows for Quality Check (QC) and auto check for compliance issues (usage of restricted words etc.) which can creep in through human errors. One can choose a hybrid workflow where machine transcription takes place first, and QC is performed on this by native translators who correct all mistakes. These corrections should ideally be fed back to the machine so that it continues learning and eventually generates better quality subtitles. It also helps to use advanced, end-to-end AI tools that not only create transcripts, but also sync these to the prescribed number of words per second/minute, as well as to the shot boundary. Such tools deliver subtitles that are far more accurate.
-
-
CONCLUSION
This article has discussed various applications of Artificial Intelligence in the field of filmmaking. The entire process of making films was subdivided into parts, and the history, present trends and future possibilities in all spheres has been discussed. This groundwork has pointed towards the gaps in the usage of AI in film making, and consequently opens up areas of further interest where further research should be undertaken. This article demystifies the subject of application of AI in film making and brings out the importance of convergence of the fields of AI and filmmaking for the advantage of both. One trend that can be deduced from the analysis of this article is that with the advent of AI in filmmaking, the Visual Effects (VFX) and various other technologically advanced pre- and post- production processes have become accessible to all. The monopoly of big production houses on such advanced technology no longer exists today. Even a novice can today use application software available in the market for content generation, smart editing, subtitling, etc. In a way, AI has led to the democratisation of the entire filmmaking process. This article also brings out how important it is today for film studios / media houses to invest in research and development so that AI driven tools can be developed. The strength of the R&D in this area can be the sole determinant whether any big film production house will survive or perish in the near future. Another very important takeaway is the realisation that AI and human intelligence can co-exist to produce wonderful results; it might not have to be an either/or situation. The AI can certainly take upon itself the repetitive and mundane aspects of filmmaking, which will free up the humans involved for greater creative
pursuits. With the level of technological advancements available presently in the field, a totally AI-conceptualised and AI-executed movie may be a little far-fetched, but the future appears bright.
-
ACKNOWLEDGEMENTS
I would like to thank my mentor Won Park (University of Michigan) for supporting me throughout the writing of this paper. I would also like to thank Candice Chen (Harvard College) who was instrumental to the completion of this paper.
VII. REFERENCES:
[1] https://www.britannica.com/technology/artificial-intelligence. [2] Sri Krishna, https://analyticsindiamag.com/how-ai-is-used-in- filmmaking.<>[3] Alex Frohlic. Artificial Intelligence and Contemporary Film Production: A Preliminary Survey
[4] Miguel Grinberg, https://www.twilio.com/blog/ultimate-guide- openai-gpt-3-language-model. [5] Hussain, The Arc Project Update #2 Tell The Model Workshop, Part1. [6] https://www.thinkautomation.com/bots-and-ai/yes-positive- deepfake-examples-exist/ [7] Khanna,2020 httpss://www.vice.com/en/article/jgedjb/the-first- use-of-deepfakes-in -indian-elction-by-bjp. [8] https://amt-lab.org/blog/2020/3/deepfake-technology-in-the- entertinment-industry-potential-limitations-and-protections [9] Spencer Perry, https://comicbook.com/movies/news/creepy-home- alone-deep-fake-pits-sylvester-stallone/. [10] Morgan, Blake. "What Is The Netflix Effect?" Forbes. June 26, 2019,https://www.forbes.com/sitrs/blakemorgan/2019/02/19/what-is- the-netflix-effect/.
[11] Peter Caranicus; https://variety.com/2018/artisans/news/artificial- intelligence-hollywood-1202865540/ [12] "How 20th Century Fox Uses ML to Predict a Movie Audience | Google Cloud Blog." Google. https://cloud.google.com/blog/products/ai-machine-learning/how- 20th-century-fox-uses-ml-to-predict-a-movie-audience. [13] "DeepAudience Analysis for Movies." Vault DeepAudience for Movies. https://www.vault-ai.com/deep-audience-movies.html. [14] Soe, Than Htut. "AI video editing tools. What editors want and how far is AI from delivering?." arXiv preprint arXiv:2109.07809 (2021). [15] https://www.technologyhq.org/how-artificial-intelligence-is- changing-video-editing [16] J. Casares, A. C. Long, B. A. Myers, R. Bhatnagar, S. M.Design Innovation: Challenges for Working with Machine [17] M. Leake, A. Davis, A. Truong, and M. Agrawala. Computational video editing for dialogue-driven scenes. ACM Transactions on Graphics, 36(4):114, July 2017. [18] P. Passarelli. autoEdit Fast Text Based Video Editing, 2019. [19] X.-S. Hua, L. Lu, and H.-J. Zhang. Optimization-Based Automated Home Video Editing System. IEEE Transactions on Circuits and Systems for Video Technology, 14(5):572583, May 2004. [20] P. Shrestha, P. H. de With, H. Weda, M. Barbieri, and E. H. Aarts. Automatic mashup generation from multiplecamera concert recordings. In Proceedings of the international conference on Multimedia – MM 10, page 541, 2010. [21] Steve O Brien; https://uk.movies.yahoo.com/2021-pop-culture- subtitle-barrier. [22] Alvarez, A., del Pozo, A., Arruti, A.: APyCA: Towards the Automatic Subtitling ´ of Television Content in Spanish. In: Proceedings of IMCSIT, pp 567574, IEEE, Wisla (2010) [23] Ãlvarez, Aitor & Arzelus, Haritz & Etchegoyhen, Thierry. (2014). Towards Customized Automatic Segmentation of Subtitles. 10.1007/978-3-319-13623-3_24. [24] https://www.softwareadvice.com/closed-captioning/ [25] https://www.limecraft.com/2021/10/28/automatic-subtitling-how- ai-is-rewriting-the-process/