
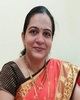
- Open Access
- Authors : Jyoti Sunil More , Jyoti Deshmukh , Deeplaxmi V. Niture
- Paper ID : IJERTV12IS030093
- Volume & Issue : Volume 12, Issue 03 (March 2023)
- Published (First Online): 23-03-2023
- ISSN (Online) : 2278-0181
- Publisher Name : IJERT
- License:
This work is licensed under a Creative Commons Attribution 4.0 International License
A Meta-Analysis and Vaccination Framework for COVID-19
Jyoti Sunil More
Associate Professor,
Fr. C. Rodrigues Institute of Technology, Vashi, Navi Mumbai,
(Affiliated to Mumbai University), INDIA
Deeplaxmi V. Niture
Assistant Professor,
Department of Electronics & Telecommunication Engineering,
COEP Technological University, Pune, INDIA
Jyoti Deshmukh
Associate Professor in Computer Engg. Amity University, Mumbai, INDIA
Abstract: India, being a developing nation, the impact of Coronavirus – 19 has advanced as a huge crisis on the prosperity, economy, guidance, organization and for the most part of progressing region. Being the second most elevated country with Coronavirus tainted populace on the planet, there is a critical requirement for the nation to plan the systems to deal with the circumstance productively. This paper deals with the investigation of current effect of pandemic over the populace and how machine learning modelling can be utilized to contemplate the effect and plan the further technique to deal with the pandemic circumstance. This paper additionally expects to introduce an intelligible and exhaustive perspective on vaccination model and its effect on the Coronavirus pandemic. It examines the difficulties and proposes a hypothetical system for immunization dispersion across the populace.
Keywords: Covid, epidemiology, pandemic, vaccination model, herd immunity
-
INTRODUCTION
From ancient times, diseases are evidently one of the biggest threats to the existence of human community. Inspite of movement of development, urbanization and a lot more components, which have made the life simpler, there is consistently a test of confronting flare-up of new diseases in the general public. These infections once in a while may provoke immense plague and appallingly in the most negative situations into pandemics, making a cut sidedness in the physical and mental prosperity of people, and sometimes inciting a critical loss of lives. Thusly, the showing of a horrifying sickness like Coronavirus, which was officially announced as pandemic by WHO in 2020, is fundamental. This demonstrating may fill in as an assisting help to assemble a procedure to manage the pandemic. For a developing nation like India the impact of this dreadful pandemic is undoubtedly beyond physical and psychological well-being misfortune. In this paper, the need of dispersion of the cures over the pandemic, as far as clinical mindfulness and appropriate immunization is examined.
There lies a fundamental connection among the networks and the epidemiology of the diseases which exhibit infection through direct contacts. (Matt and Ken, 2005; Anderson and May, 1992). Recent advances in mobile technology and GPS area have helped extensively to make the progress in this field feasible and is in constant phase of development. This would allow the researchers to fabricate full and extensive setups for
new forms of infections, and furthermore to adapt the changing organization structure even with an extreme scourge, like a flu epidemic or bioterrorist assault, where the trend behavior might be drastically not the same as the usual.
In this paper, we have investigated and articulated the effects of pandemic situation, how the propagation has affected the population, the way it could be modelled using the epidemic models and finally we proposed an effective strategy of vaccination model, which we hope will certainly help the researchers and pharmaceutical experts to deploy in future in similar circumstances of epidemics or pandemics.
-
RELATED WORK
The covid -19 analysis is done extensively from very diverse perspectives, like deep learning (Arora et.al. 2020;Zahidi et al. 2020) analytics (Raturi and Kumar 2018), artificial intelligence (Chandrashekharan and Kumar 2018; Ilyas et al. 2004), relationship analysis (Melin et al. 2020), sentiment analysis, SVM (Sasubilli et al. 2018), etc. The case studies based on the immune system, effects of obesity, effects of other health conditions and other clinical features were analysed by many researchers (Weng hong Wu et al. 2021; Nicholich et al. 2020; Luzi and Radaelli, 2020; Bhattacharya et al. 2020; Patrikar et al. 2020; Ranjan 2020).
-
MACHINE LEARNING METHODOLOGY:
The general methodology to model the covid dataset is described in figure 1. The model shows the conventional methodology applied on the dynamic covid dataset.
Fig. 1 : Machine learning model for COVID-19 Dataset
-
Dataset Description:
The dataset is derived real time from the website (https://api.covid19india.org/) which collects live data and arranges it statewise. The data considered for the analysis is from the timeframe of: Feb to first week of May 2021. The status of the infected population is categorized as: {Deceased, Hospitalized, Migrated and Recovered}. The dataset size is 107617 rows × 20 columns.
Fig. 2: statewise data: feb to first week of may 2021
Fig. 3: Scatterplot of timeline (per day cases) vs total number of cases
Figure 2 and 3 represents the data considered for the analysis of COVID spread in different states in India. The plot shows that the state of Maharashtra had highest number of covid infected cases. The scatterplot clearly exhibits that the number of infected cases increased exponentially with the time period.
Fig. 5: month-wise data of deceased cases across the country
Figures 4 and 5 shows the month wise data of the population with the status as Hospitalized and deceased, respectively, across the country.
-
Analysis: Model Fitting
Fig. 6 : Regression Algorithms: Scatter plots a. Polynomial Regression (Accuracy=95.96%) b. Support Vector Regression (Accuracy=90.08) c. Decision Tree Regression (Accuracy= 100%- a clear case of overfitting) d.
Random Forest Regression (Accuracy=98.26%)
It is clearly observed from figure 6 that the number of cases increased exponentially. Different machine learning algorithms are used to predict the behaviour of the spread of the disease. This exponential growth is further studied using epidemic models.
Fig. 4: month-wise hospitalization data across the country
4 EPIDEMIC MODELS
Epidemic models by and large expect that the community of diffused cases can be separated and labelled into various blocks which serves as classes, depending upon the phase or the degree of the infection (Linda and Allen 1994; Anderson and Britton 2000; Matouk 2020; Kermack and Kendrick 1927), for example, susceptible (represented by S, the individuals who are likely to get contaminated), Infectious (I, the individuals who acquired the contamination and can diffuse the infection), and the class Recovered(R, the individuals who recuperated).
Extra blocks may be utilized to flag some more potential conditions of people concerning the infection, for example insusceptible/immune people. This system can be stretched out to consider vectors, like mosquitoes for maleria, for infections proliferating through contact with an outer transporter. Epidemic modelling portrays the dynamical advancement of the virus interaction inside a populace. To comprehend the development of the quantity of contaminated people in the populace as an element of time, it is required to quantify and understand the characteristics of the fundamental individual level estimate, that administer the change of people starting with one compartment then onto the next.
The most straightforward interpretation of epidemic model thinks about the complete populace in the framework as fixed, comprising of N people, and disregards some other dynamic measure (relocations, births, deaths, etc.)
4.1 SIS Model
This is a simple model with two-state compartmentalizations which exhibits just two potential changes: The first, signified S I, happens in the event of infection spread to susceptible individual after he connects with an individual who has acquired the infection. The subsequent progress, indicated I
S, happens in the event of recovery of infected individual from the disease and gets back to the domain of susceptible population. This model anticipates that the illness doesn't give resistance and people may reach to the pool of infected community recursively, exhibiting a loop S I S. It should be noted that under certain conditions, the behavior may be supported throughout.
4.2. SIR Model
Another fundamental model is exemplary SIR model (Bagal et al. 2020; Dhanwanth and Ramnathan 2020). In this, the progress from I to S of the SIS interaction is replaced by I to
R. This occurs in the event of an infected individual recovering from the illness and anticipates to have gained a perpetual insusceptibility, i.e. immunity or is eliminated (for example has died).
Inevitably, the SIR cycle consistently terminates, when not any more contaminated people are available as shown in figure 7. The COVID-19 situation in India is analyzed by many researchers using SIR model. (Jakhar et al. 2020; Deo et al. 2020; Adisak, 2013).
Fig. 7 : SIR model (beta=1.4247, gamma=0.14286)
-
SEIRS Model to model the Infection of COVID-
Fig. 8: SEIRS Model to showcase the lifecycle of COVID infection phenomenon
In the figure 8, set S represents a set of Susceptible (S) individuals who may be exposed (E) to the virus. The infected individuals may be asymptotic (represented by set A), still these have the capability to infect other individuals. The asymptotic set of individuals may recover without any treatment or sometimes, they may exhibit the symptoms at a later stage and become a part of set I. The set of individuals represented by set I are treated variably, as general hospitalization for mild symptoms where the grade of severity is less whereas they may be admitted to ICU or may need the assistance of ventilator depending on the grade of severity. Few individuals may recover from this stage which are represented by set R, while some reach the state of deceased,
D. Few cases have also exhibited the behaviour of recurrence. Hence the individuals belonging to state R also may again return to the state of S and again may follow the same lifecycle. This model follows the SEIRS model of epidemic theory. The SEIRS model (Adisak et al, 2013; Kim et al. 2019; Engbert, 2020; Berger et al. 2020) is designed in a manner that handles the situation where recovered individuals are likely to return to a domain of susceptible community. It should be noted that, defines the rate at which community belonging to recovered domain returns to the susceptible domain maybe in
the event of loss of immunity.
where N=S+E+I+R is the total population [Adisak 2013].
With added vital dynamics, where and again represent the birth and death rates, respectively. To maintain a constant population, assume that =. In steady state dI / dt=0.
-
Model based on Exact Markov chain Model:
For a connected network G (V, N) having an adjacency matrix A, let V represent the number of nodes, and N the set of directly connected nodes of node I (neighbours). The state of node i at time instant t, denoted by Si(t), can take one of the following values: 0 for Susceptible entities, 1 for Exposed entities, 2 for the Infected entities, and 3 for Recovered ones.
i.e. Si(t) {0, 1, 2, 3}.
Fig. 9: State diagram of SEIR model, and the transition rates
Figure 9 depicts four states and the respective transitions, : denotes factor representing the transmissions per S-I contact per time, : denotes factor representing rate of transformation, : denotes the factor representing rate of recovery, : denotes the factor representing the rate of re-susceptibility. It is significant to note that this is equal to inverse of temporary immunity period and is 0 if permanent immunity, I: denotes the factor representing rate of mortality caused by the infection. The state of whole network at time t, can be represented in general as:
S(t) = (Si(t), . . . , Sn(t)) {0, 1, 2,3}n
Additionally, according to the assumption that the next state is independent of the current state, the 4n × 4n state transition matrix (denoted by T) of the Markov chain (Ruhi and Hassibi 2015), will have the elements of the form:
-
Vaccination Model:
In this section it is shown that, it is possible to exhibit the effect of vaccination by incorporating direct immunization into the model. This can directly lead to a new transition straight from S to R as . Alternatively, it can be stated that the transition from state S to state R is also possible as depicted in figure 10.
Fig. 10: State diagram of SEIRS- Vaccination model
This type of epidemic model is termed as SIV (Susceptible Infected-Vaccinated), and it is extensively used for modelling estimations on populations.
-
WORKING MODEL FOR VACCINATION FOR
COVID:
The vaccination for COVID-19 can be modelled as shown in figure 11.
Fig. 11: Vaccination Model for COVID-19
Here, it is shown that the entire susceptible population is not vaccinated at once, but in batches. It is necessary to model the scheduling of these batches in a proper manner to ensure that the maximum population gets benefited from the vaccination drives. In compartmental models for the transmission of transferable sicknesses there is typically a fundamental conceptive number R0, addressing the mean number of auxiliary diseases brought about by a solitary infective brought into a susceptible populace. In the event that R0 < 1, there is an illness free balance which is asymptotically steady, and the disease ceases to exist. On the off chance that R0 > 1, the standard circumstance is that there is an endemic balance which is asymptotically steady, and the contamination endures. If there should arise an occurrence of no impact, the populace again gets included in the set of susceptible individuals.
-
PROPOSED FRAMEWORK:
In section 3.2, the monthwise analytics of infected rate is shown. It is already discussed that the growth is exponential in nature. Keeling and Matt, (2008) coined the term Herd Immunity. It denotes the interaction where the risk of infection for the community decreases gradually and eventually, as every person getting vaccinated. Alternatively, Herd insusceptibility occurs in the event when nearly everybody acquires the infection. Also, there are adequate group of individuals who remained uninfected (Johnson and McQuarrie, 2009). One primary motive behind immunization is to drive the population resistant to the sickness i.e. to build a herd immunity and simultaneously having the measure of contaminated individuals to be minuscule. The threshold for Herd Immunity represented by (Hi) can be viewed as the extent of the population that should be guarded, which will ultimately aid to control transmission of an infection, for example BR equivalent to one. The condition stated by Diekmann and Heesterbeek (2000) to sort the threshold for Herd Immunity is represented as, Hi = (1-(1/BR))
As the result of increase in the quantum of immunizations, the group resistance limit also tends to elevate. By diminishing the measure of susceptible individuals, the herd immunity
threshold diminishes (Diekmann and Heesterbeek, 2000; Kemp et al. 2003; More and Lingam, 2019). For this it is proposed that the vaccination should follow the greedy approach which will lead to submodular optimization. The proposd approach is articulated from the concept of influence maximization where the best target nodes which can carry the influence to maximum number of nodes in a given network are selected.
Postulate 1: Given a graph G, a set of initially infected
nodes B, and a budget k, find a set of nodes S V to vaccinate, such that |S| k, and the population saved is maximized.
The decision has to be taken clearly that the node which has maximum influence i.e. is connected to maximum number of nodes have to be vaccinated earlier than the other nodes of population. For finding such a node S, Kempes greedy algorithm (Kemp et al. 2003) can be used.
Input: G, k, m(S) Output: seed set S,
-
S
-
while |S| < k do
-
u argmax w VS (m(S + w)m(S));
4. S S{u}
Here, the algorithm converges and iteratively picks a node that
provides the maximum infection spread. Alternatively, it can be stated that, the largest marginal gain m (S+w) m (S) with respect to the total expected infection spread of the seed set in context i.e. S. This ultimately ensures the compliance of submodularity and monotonicity.
6.1 Impact of social distancing on the overall model:
Postulate 2 : If the rate of transmissions per S-I contact per time decreases, then the time required for overall transmission of the disease to the next set of population also decreases.
Proof:
In the SI model, the number of susceptible individuals decreases monotonically, that is Sn+1 Sn, for all n. It is also obvious that the number of infected individuals increases monotonically, i.e., In+1 In, for all n. . Ultimately, in SEIRS, if rate of susceptible individuals decreases then the rate of infected individuals will decrease monotonically. One of the solutions is reducing the social contact. This is observed and is proven as an effective methodology in (Sutton 2014; Singh and Adhikari 2020; Das, 2020; Hazem et al. 2020; Feng et al. 2020; Goswami et al. 2020).
Similarly, according to the property of SI model, the number of susceptible individuals is never negative, Sn0, and the number of infected individuals is never more than the total population size, InN (Kemp et al. 2003; More and Lingam, 2019).
Ultimately this leads to conclusion that – If the rate of transmissions per S-I contact per time decreases, then the time required for overall transmission of the disease to the next set of population also decreases.
7. CONCLUSIONS:
The COVID-19 situation has become a major issue of concern globally. The virus is creating impacts in the normal routine of the population. Many have lost their lives. The virus has created physical, mental and economic impact on the society at a great extent. Many researchers are analysing different factors and situations, to put forth theory to model and predict the behaviour of the virus. Here in this paper, a systematic way of analysing the COVID-19 situation in India is presented. Different machine learning models are used to represent the data. It is undoubtedly showing an exponential growth. This leads to a necessity to analyse and study the spread of the virus. Eventually, different epidemic models are presented to show the behaviour of the virus spread in various ways. The paper has its contribution in presenting a vaccination model and analysing the way outs of how the spread can be controlled in a systematic manner. It is proposed that the greedy approach and exploiting submodular optimization, to maximize the set of safe population, can eventually help to drive the vaccination campaign more successful. For this, the individuals who have maximum reach or connectivity to the rest of the population, should be prioritized for vaccination. With the advent of new variants and the behaviour of these variants, the researchers have a scope to analyse challenge of dealing with new issues. Over the period of time, it has been proved that the proper deployment of vaccination model is certainly very significant and effective to control the epidemics and pandemics.
ETHICAL STATEMENT:
This research is not funded by any agency or institute.
REFERENCES
[1] Keeling Matt J and Eames Ken T.D. (2005). Networks and epidemic models, J. R. Soc. Interface.2295307, http://doi.org/10.1098/rsif.2005.0051 [2] Anderson, R. M., and R. M. May.(1992). Infectious diseases in humans, Oxford University Press, Oxford. [3] Arora P, Kumar H, Panigrahi BK. (2020). Prediction and analysis of COVID-19 positive cases using deep learning models: A descriptive case study of India. Chaos Solitons Fractals:139:110017. [4] Ayyoubzadeh SM, Zahedi H.(2020). Predicting COVID-19 incidence through analysis of Google trends data in Iran: data mining and deep learning pilot study. JMIR Public Health Surveill;6(2):e18828. [5] R. Raturi and Kumar A.(2019). An Analytical Approach for Health Data Analysis and finding the Correlations of attributes using Decision Tree and W-Logistic Modal Process, IJIRCCE, Vol 7, Issue 6, ISSN: 2320-9801. [6] S. Chandrasekaran and Kumar A.(2018). Implementing Medical Data Processing with Ann with Hybrid Approach of Implementation, Journal of Advanced Research in Dynamical and Control Systems- JARDCS issue 10, vol.10, page 45-52, ISSN-1943-023X. [7] Ilyas M, Rehman H, Nait-ali A. (2020). Detection of Covid-19 from chest X-ray images using artificial intelligence: an early review. arXiv preprint arXiv:2004.05436. [8] Melin P, Monica JC, Sanchez D, Castillo O.(2020), Analysis of spatial spread relationships of coronavirus (COVID-19) pandemic in the world using self-organizing maps, Chaos Solitons Fractals, 138:109917. DOI: 10.1016/j.chaos.2020.109917. [9] S. Sasubilli, K. Attanguddi and A. Kumar. (2018). APSO based feature extraction approach and SVM based classification for sentimental analysis, Global Journal on Innovation, Opportunities, and Challenges in Applied Artificial Intelligence and Machine Learning, Vol. 2, Issue 1. [10] Zeng-hong Wu, Yun Tang and Qing Cheng. (2021). Diabetes increases the mortality of patients with COVID-19: a meta-analysis, Acta Diabetologica, https://doi.org/10.1007/s00592-020-01546-0. [11] Nikolich-Zugich J, Knox KS, Rios CT.(2020). SARS-CoV-2 and COVID-19 in older adults: what we may expect regarding pathogenesis, immune responses, and outcomes. Geroscience , https://doi.org/10.1007/s11357-020-00186-0 [12] Luzi L, Radaelli MG. (2020). Influenza and obesity: its odd relationship and the lessons for COVID-19 pandemic, Acta Diabetol, https://doi.org/10.1007/s00592-020-01522-8, 2020. [13] Bhattacharyya A., Bhowmik D., Mukherjee J. (2020). Forecast and interpretation of daily affected people during 21 days lockdown due to COVID 19 pandemic in India, medRxiv DOI: https://doi.org/10.1101/2020.04.22. 20075572 [14] Patrikar S, Poojary D, Basannar D, Kunte R.(2020). Projections for novel coronavirus (COVID-19) and evaluation of epidemic response strategies for India, Med J Armed Forces India https://doi.org/10.1016/j.mjafi.2020. 05.001. [15] Ranjan, R.(2020). Predictions for COVID-19 outbreak in India using epidemiological models medRxiv DOI: https://doi.org/10.1101/2020.04.02. 20051466. [16] Linda J.S. Allen. (1994). Some discrete-time SI, SIR, and SIS epidemic models, Math. Biosci., 124 (1), pp. 83-105 [17] Andersson, H., and T. Britton (2000), Stochastic epidemic models and their statistical analysis, Lecture Notes in Statistics, Vol. 151 (Springer US, New York) 2000. [18] Matouk A. (2020). Complex dynamics in susceptible-infected models for COVID-19 with multi-drug resistance, Chaos Solitons Fractals 2020;140:110257. doi: 10.1016/j.chaos.2020.110257. [19] Kermack, W. O. and McKendrick, A. G. (1927). A Contribution to the Mathematical Theory of Epidemics, Proc. Roy. Soc. Lond. A 115, 700- 721. [20] Bagal, D.K., Rath, A., Barua, A., Patnaik, D.(2020) Estimating the parameters of susceptible-infected-recovered models of COVID-19 cases in India uring lockdown periods, Chaos Solitons Fractals 140, 110154. [21] Dhanwant, J.N., Ramanathan, V. (2020). Forecasting COVID 19 growth in India using susceptible-infected-recovered (SIR) model, arXiv:200400696 [Preprint]. [22] Jakhar M., Ahluwalia P., Kumar A.(2020). COVID-19 epidemic forecast in different states of India using SIR model, medRxiv [Preprint], DOI: https://doi.org/ 10.1101/2020.05.14.20101725. [23] Deo, V., Chetiya, A.R., Deka, B., Grover G. (2020). Forecasting transmission dynamics of COVID-19 epidemic in India under various containment measures-a time dependent state-space SIR approach, medRxiv [Preprint] DOI: https:// doi.org/10.1101/2,020.05.08.20095877 [24] Adisak Denphedtnong, Settapat Chinviriyasit, Wirawan Chinviriyasit. (2013). On the dynamics of SEIRS epidemic model with transport- related infection Mathematical Biosciences, Volume 245, Issue 2, 2013, Pages 188-205,ISSN 0025-5564. [25] Kim, S., Byun, J.H. & Jung, I.H. (2019). Global stability of an SEIR epidemic model where empirical distribution of incubation period is approximated by Coxian distribution. Adv Differ Equ 2019, 469 (2019). https://doi.org/10.1186/s13662-019-2405-9. [26] Engbert R., Rabe,M.M., Kliegl R., Reich S. (2020). Sequential data assimilation of the stochastic SEIR epidemic model for regional COVID-19 dynamics, medRxiv [Preprint] DOI: https://doi.org/10.1101/ 2020.04.13. 20063768. [27] Berger DW, Herkenhoff KF, Mongey S. (2020). An SEIR infectious disease model with testing and conditional quarantine, National Bureau of Economic Research. doi:103386/w26901. [28] N. A. Ruhi and B. Hassibi, (2015). SIRS epidemics on complex networks: Concurrence of exact Markov chain and approximated models, 54th IEEE Conference on Decision and Control (CDC),Osaka, Japan, pp. 2919-2926, doi: 10.1109/CDC.2015.7402660
[29] Keeling Matt. (2008). The mathematics of diseases, Plus Magazine: Living Mathematics. [30] T. Johnson and B. McQuarrie. (2009). Mathematical modeling of diseases: Susceptibleinfected-recovered (sir) model, In University of Minnesota, Morris, Math 4901 Senior Seminar. [31] Diekmann, O., and J. A. Heesterbeek. (2000). Mathematical Epidemiology of Infectious Diseases : Model Building, Analysis and Interpretation. New York: John Wiley & Sons, Incorporated. [32] David Kemp, Jon Kleinberg, and Eva Tardos. (2003). Maximizing the spread of inuence through a social network, in KDD 03, USA. [33] J.S. More and C. Lingam .(2019). A SI Model for Social Media Influencer Maximization, Applied Computing and Informatics, Volume 15, Issue 2. [34] Kacie M. Sutton (2014) Discretizing the SI epidemic model, Rose-Hulman Undergraduate Math. J., 15, pp. 192-208
[35] Singh R., Adhikari R. (2020). Age-structured impact of social distancing on the COVID-19 epidemic in India, arXiv:200312055 [Preprint]. [36] Das S. (2020). Prediction of covid-19 disease progression in India: under the effect of national lockdown, arXiv:200403147 [Preprint]. [37] Hazem Y., Natarajan, S., Berikaa E. (2020). Hasty reduction of COVID-19 lockdown measures leads to the second wave of infection, medRxiv [Preprint] DOI: https://doi.org/10.1101/2020.05.23.20111526. [38] Feng, Z., Damon-Feng, H., Zhao, H.(2020). Sustaining social distancing policies to prevent a dangerous second peak of COVID-19 outbreak. medRxiv [Preprint]. DOI: https://doi.org/10.1101/2020.04.17.20069351. [39] Goswami, G., Prasad, J., Dhuria, M.(2020). Extracting the effective contact rate of COVID-19 pandemic. arXiv:200407750 [Preprint].AUTHOR INFORMATION
Dr. Jyoti Sunil More, pursued B.E. Computer Science & Engg.
(2003) from Shivaji University, M.Tech. in Computer Engineering (2006) from Dr. B.A.T.U., Lonere and Ph. D. in Computer Engg. From University of Mumbai in 2019. She has 17 years of Teaching experience and is currently affiliated to Fr. C. R. I. T, affiliated to Mumbai University. Her area of expertise are Computer Networks,
Databases, Data mining, Machine Learning, Data Science, Artificial Intelligence, etc.
Dr. Jyoti S. Deshmukh is working as Associate Professor in Computer Engg. Amity University, Mumbai. Her area of interest is opinion Mining, Machine learning, Data science.
Dr. Deeplaxmi V. Niture: has received her B. Tech (2003) and M. Tech (2006) in Electronics and Telecommunication Engineering from Dr. Babasaheb Ambedkar Technological University (Dr. B. A. T. U), Lonere, India. She has pursued Ph.D. in Electronics and Telecommunication Engineering from
the Savitribai Phule University of Pune, Maharashtra, India in 2021. Presently she is working as assistant professor in College of Engineering Pune. She has a teaching experience of more than 12 years. She has authored several journal and conference papers. Her research interests include Microstrip patch antenna, Ultra-wideband antenna, reconfigurable antenna, machine learning, data science, etc.