
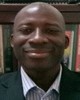
- Open Access
- Authors : Dr. Michael S. Aduah , G. P. W. Jewitt , Dr. Michele L. W. Toucher
- Paper ID : IJERTV10IS040140
- Volume & Issue : Volume 10, Issue 04 (April 2021)
- Published (First Online): 22-04-2021
- ISSN (Online) : 2278-0181
- Publisher Name : IJERT
- License:
This work is licensed under a Creative Commons Attribution 4.0 International License
Scenario-Based Impacts of Land Use and Climate Changes on the Hydrology of a Forested Catchment in Ghana, West Africa
1Dr. Michael S. Aduah, 2Prof. G. P.W. Jewitt and 3Dr. Michele L.W. Toucher
1Geomatic Engineering Department University of Mines and Technology PO Box 237, Tarkwa, Ghana
2IHE Delft Institute of Water Education, Delft, The Netherlands
3South African Environmental Observation Network, Grasslands-Wetlands-Forests Node, PO Box 13053, Cascades, 3202, Pietermaritzburg, South Africa
Abstract This study analysed the separate and the combined impacts of climate and land use (LU) changes on hydrology of the Bonsa catchment in Ghana, West Africa, using the Agricultural Catchments Research Unit (ACRU) hydrological model. The study used five Representative Concentration Pathway (RCP) 8.5 climate change scenarios (wet, 25th percentile, 75th percentile, dry and a multi-model median of nine GCMs) from the CMIP5 AR5 models for near (2020 2039) and far (2060 2079) future time slices. Change factors were used to downscale the GCM scenarios to the local scale, using observed climate data for the control period of 1990 to 2009. The land use of 1991 was used to define baseline hydrological conditions and 2011 land use as well as three future land use scenarios (business-as usual: BAU, economic growth: EG, economic growth with enhanced reforestation: EGR) for two time slices (2030 and 2070) were used. The study showed that under all separate climate change scenarios, overall flows for the major peak (wet) season reduced, but increased for both the dry and the minor peak (wet) seasons, but under combined climate and LU changes, streamflows increased. Under the combined scenarios, streamflow responses due to the different future LU scenarios were not substantially different. Additionally, LU appeared to be the dominant controlling factor in streamflow changes in the Bonsa catchment under a dry climate change, but under a wet climate change, climate controls streamflow changes. The spatial variability of catchment streamflow changes under combined LU and climate changes were greater than the spatial variability of streamflow changes under climate change.
KeywordsBonsa catchment, change factors, climate changes, Ghana, land use changes, spatial variability
-
INTRODUCTION
Climate change has become a topical issue because of its far-reaching impacts on economies, life, property and the environment [47, 58]. Climate change intensifies the hydrological cycle, with consequences such as increases in frequency and severity of droughts and floods. The impacts of climate change on hydrology influences agriculture, water supply, environmental sustainability and protection from
floods and infrastructure. Therefore determination of the impacts of climate change on hydrology at the local scale is urgently needed in order to promote sustainable development and the protection of life, property and the environment. Land use also plays an important role in the environment, as it partitions rainfall into the components of the hydrological cycle [17, 20, 60], such as evaporation, runoff and groundwater. Land use changes thus change the balance between the components of the hydrological cycle, which can lead to several challenges in water resources and environmental management [27]. It has also been suggested
[14] that these challenges are more variable at the local and regional scale than at the global scale. Furthermore, since climatic changes can influence land use changes [61] and land use changes through vegetation dynamics can also feedback to impact regional climate [62, 59], comprehensive understanding of land use change and climate change impacts on hydrology can only be gained from determination of both their combined and separate impacts. The combined effects of climate change and land use changes on hydrology and the environment can lead to severe water resources and environmental problems at the local scale.In West Africa, during the past four decades, there has been extensive land use changes and intensification of climate change. For example, between 1990 and 2010, deforestation has resulted in the removal of about 32 million ha of forest [22] and during the twentieth century mean annual temperatures increased by 275 K, while mean annual precipitation decreased by approximately 20% [28]. According to the IPCCs assessment reports [29, 30], temperatures will continue to rise in the 21st century, but there is no clear trend in precipitation for the region. It has been argued that the changes in the past four decades have largely been the result of the large-scale drought in West Africa, between the 1970s and the early 1980s [35, 36], agricultural expansion, as well as population increase and urbanization [10, 31].
Despite the substantial changes in West Africa and
the numerous studies that have been conducted [8, 12, 49, 55,
11], the responses to climate change and land use changes are still not understood well, especially in the southern rainforest catchments, where the majority of the population live. Aduah
[2] concluded that land use changes have altered the hydrology and the ecology of the Bonsa catchment of Ghana (a representative rainforest catchment of West Africa), with both increases in peak and low flows. The future scenarios of land use change in the catchment point to higher increases in both peak and low flows and a higher potential for ecological alterations. However, since the study did not consider effects of climate, it is not known how climate change and the combined impacts of land use changes and climate changes will affect the hydrological cycle components in the near and far future. Since land use and climate changes affect each other [21, 20] and their joint impacts are sometimes non-linear [38], it is necessary to determine both the joint and separate impacts on the hydrology of the catchment to improve knowledge and understanding of global change impacts in the region.The aims of this study were to determine the long- term impacts of climate changes, land use changes, as well as the combined impacts of climate change and land use changes on the hydrology of the Bonsa catchment, which was selected to represent lowland rainforest catchments of West Africa. The study builds on a companion study in Aduah et al. [1] and it uses the ACRU hydrological model, which has previously been calibrated [3] for the study area.
-
MATERIALS AND METHODS
Study Area: The Bonsa catchment in Ghana, West Africa (Figure 1) is located between longitudes 1° 41´ and 2° 13´ West and latitudes 5° 4´and 5° 43´North. The catchment (1482 km2) has a low relief, with elevation ranging between 30 and 340 m above mean sea level. The rainfall regime is bimodal, with the major season peaking in July and the minor season peaking in October. The annual rainfall ranges between 1500 mm/year and 2150 mm/year and the annual average minimum and maximum temperatures are 23ºC and 31ºC, respectively. The catchment land cover consists mostly of evergreen and secondary forests and shrubs/farms [5]. The geology is characterized by Tarkwaian rocks, which consist of metamorphosed and unmetamorphosed shallow water sediments and Birimian rock systems, which also consist of metamorphosed sediments and volcanics [6] and the soil is composed mostly of Ferric Acrisols. Major economic activities in the catchment include open-pit gold mining, rubber and small-scale cocoa and food crop cultivation.
Fig 1. Map of study area showing the Bonsa catchment in the Ankobra Basin, Ghana and the GCM (CSAG) as well as observed climate stations []
The ACRU Hydrological Model: In this study The ACRU Model [51, 53, 60, 19] is a multi-purpose, daily time step physical-conceptual hydrological model developed by the University of KwaZulu-Natal, South Africa, to simulate catchment hydrological responses (Figure 2). The model can be used for catchment water resources assessment including assessment of impacts of land use changes [52, 50, 61] and climate changes [23, 25, 32]. The ACRU model uses variables derived directly from catchment physical properties and does not depend on parameter optimisation. The key component of the ACRU model is multi-layer soil water budgeting, which is based on total evaporation (Figure 2). This makes the model sensitive to climate and land cover/land use changes. Land use/vegetation in the ACRU model is simulated using canopy interception losses (VEGINT), consumptive water use by plants (CAY), total evaporation (evaporation from soil surface and transpiration) and soil water abstraction by plant roots [51, 53,60]. The ACRU model uses Green-Ampt method to compute infiltration, while reference potential evaporation (PET) is either obtained from A-pan measurements or Penman-Monteith, Hargreaves, Linacre or Blaney-Criddle methods [51]. The model uses a modified soil conservation service (SCS-SA) curve number (Equation 1), to relate antecedent soil moisture to runoff [32]. Furthermore, ACRU model is a multi-level system, as it can operate as a point model, or as a lumped small catchments model [51] and in large complex catchments, it can operate as a distributed cell- type model, where cells represent subcatchments, which can be divided into hydrologic responses units (HRU). The ACRU modelling approach is flexible because it allows user inputs based on information obtained by reading of the landscape, making it a useful tool for water management in data scarce catchments.
(1)
Where Q is streamflow (mm/day), P is precipitation (mm/day), Ia is initial abstraction (mm), which is a function of coefficient of initial abstractions (COAIM) and S is soil water deficit, a function of the critical soil depth (SMDDEP).
Fig 2. Representation of hydrological processes in the ACRU model [51]
ACRU Model Parameterization: A total of 103 subcatchments were created using digitized contour and river courses maps obtained from the Survey of Ghana (SOG). The associated hydrological response units (HRUs) were created based on catchment land uses [5]. In order to define the flow path of the rivers, a catchment configuration network, using subcatchments, HRUs and the river courses, was created. The land use data, soil information and streamflow simulation control variables used in this study are the same as those used in the companion papers in Aduah et al. [3] and Aduah et al. [1]. The historical land use was derived from Landsat images [5], while the simulated potential future land use scenarios were derived from a land use modelling study [4]. The soil map was obtained from the Soil Research Institute of Ghana (SRIG). ACRU soil water budgeting determines the amount of rainfall that becomes part of streamflow and groundwater, which is executed using streamflow simulation control variables. During the modelling, typical values of streamflow simulation control variables were obtained from [51] and other sources [9].
Firstly, the fraction of saturated soil water to be redistributed daily from topsoil into subsoil (ACRU variable name: ABRESP) as well as into groundwater store (ACRU variable name: BFRESP) were set to 0.1, which is a typical value for soils of the study area (clay soils). Also, critical soil depth (ACRU variable name: SMDDEP) from which stormflow occurs was set at 0.4 m, which is a typical value for high rainfall catchments [51] such as the study area. For baseflow response fraction, a typical value of 0.009 was used to control the quantity of groundwater that becomes part of streamflows in a day. Furthermore, quick flow response fraction (ACRU variable name: QFRESP), which is fraction of rainfall that becomes streamflow (runoff coefficient) for each
land use type was set. QFRESP values of 0.1, 0.2 and 0.36 were set for evergreen forests, secondary forests and shrubs/farms, respectively. These values were adapted from a study by Asomaning [9] where a value of 0.36 was obtained as total runoff coefficient for a degraded forest catchment in southern Ghana. However, for settlements, a typical QFRESP value of 0.7 was used [15] and QFRESP of 0.5 was set for mining areas, since they are more pervious than settlement areas in the study region.
The physically-based conceptualisation of the land cover characteristics and its various interactions with the hydrological processes in the ACRU model, implies that the structure of the model is sensitive to changes in land cover, land use and land management [51, 60]. Therefore, the ACRU model is able to simulate the impacts of land cover and land use change on water flows. The above-ground vegetation properties in the ACRU model are conceptualised through the consumptive water use of vegetation which is expressed as a monthly crop coefficient (ACRU variable name = CAY) and canopy interception loss as either a monthly canopy interception loss per rain-day (VEGINT). The rainfall abstracted by interception, surface detention storage and initial infiltration before stormflow commences is represented in the ACRU model through the coefficient of initial abstraction (COIAM). The soil water content of the A-horizon, infiltrability and initial abstractions influence the daily soil water budget [51]. The presence and amount of litter and/or mulch, which has the potential to reduce and/or prevent soil erosion and soil water evaporation losses, is accounted for through the input of the percentage surface cover (PCSUCO) of each land cover/use. The below ground processes are conceptualised through three root related parameters and a plant soil water stress indicator. The three root parameters required are the total depth of the root profile (EFRDEP), the percentage of active roots in the A-horizon (ROOTA) and lastly the degree of root colonisation in the soil horizons (COLON). To account for the onset of plant stress, the fraction of plant available water in the soil horizons at which total evaporation is assumed to drop below maximum evaporation due to drying of the soil needs to be input. The model converts the monthly vegetation parameter (Table 1) values into daily values, using Fourier analysis [51].
ACRU Model Calibration and Validation: The ACRU hydrological model was run using the baseline land use of 1991, soil and climate data and manually calibrated by varying the sensitive parameters (Table 1), identified in a sensitivity analysis [3], iteratively within their physical limits, until the simulated streamflows compared well with the observed streamflows. After a satisfactory calibration the ACRU model was run with the final model parameters (Table 1) and validated (Figure 3). Model performance evaluation during calibration (1990-1993) and validation (1994-1999) was executed using seven performance metrics; namely, the Nash- Sutcliffe efficiency index (NSE), the Percent bias (PBIAS), Pearsons correlation coefficient, coefficient of determination (R2), flow duration curves (FDC), the unit hydrograph [45] and scatter plots of monthly runoff coefficients for observed
and simulated streamflows. The model simulation started from 01-01-1987 to 31-12-1999, with the period from 01-01-1987 to 31-12-1989 used as model warm-up period.
Land Use Scenarios: In addition to the baseline (1991) and 2011 land use, three future land use scenarios (Figure 4), namely business-as-usual (BAU), economic growth (EG) and economic growth with enhanced reforestation (EGR), for two time slices (2030 and 2070) were used. The land use scenarios were obtained from Aduah et al. [4]. For each subcatchment, the area of the land uses present in the baseline, current and future scenarios were determined, and used to create the HRUs. Thus, to simulate land use impacts on stramflows, the areas of the HRUs within the subcatchments varied between the different land use scenarios, but the soils and the streamflow control variables were held constant. Therefore, streamflows in an HRU will increase if its land uses change from those with higher CAY and VEGINT values (e.g., evergreen and secondary forests) to those with lower values (e.g., farms and settlements).
For each land use type the vegetation parameters obtained after ACRU model calibration were used. To determine the impacts of climate and land use change on the hydrological responses of the Bonsa catchment, various simulations were performed. The calibrated ACRU hydrological model was run for the Bonsa catchment for the baseline scenario, using the baseline land use of 1991 and baseline observed climate data from 1990 to 2009. The separate impacts of climate changes on streamflows were determined by running the ACRU model for each selected climate change scenario and holding the land use as the baseline (1991) land use. Following this, the combined impacts of climate and land use change were determined by varying both the climate change and the land use scenarios.
Global Climate Model Scenarios and Downscaling: GCMs are the most important tools for studying climate change impacts [24, 56]. Substantial progress has been made in GCM modelling in the past several decades, including the use of finer resolutions and realistic parameterisation of vegetation and many physical processes [29, 7, 30]. Unfortunately, there are still numerous sources of uncertainties in GCM climate data, especially at the regional scale, due to the inability of the models to capture small scale processes accurately [14], coarse spatial resolution [48, 56], uncertain future emission scenarios [33], inconsistent rainfall estimation [44] and biases in extreme events [56]. Thus, GCM climate data is still unsuitable for hydrological modelling at the local scale [14]. For impact assessments, such as the current study, downscaling to a finer resolution [39, 14], using either dynamic or statistical downscaling (SD) [34, 42] is required.
Consequently, statistically downscaled future climate records of the 8.5 Representative Concentration Pathway (RCP) scenario from four GCMs and a multi-model median of all available nine CMIP5 AR5 models were obtained from the Climate System Analysis Group (CSAG) [18] of the
University of Cape Town, South Africa. The selection of GCMs was based on changes in mean annual precipitation (MAP) (Table 2). Two GCMs representing the extremes (wettest: CNRM-CM5 and driest: MIROC-ESM) and two representing 75th (GFDL-ESM2M) and 25th (MIROC5) percentile changes in MAP as well as a multi-model median of available downscaled GCMs were used for the study. The selection of the wettest and driest GCMs was to account for hydrological extremes [40] such as floods and droughts, while the selection of the 75th and the 25th percentiles of GCMs was to avoid possible outliers in the climate projections [43], to provide a more robust impact analysis. The use of the multi- model median of the GCMs for the study was to account for average hydrological conditions, which are also vital for sustainable water supply as well as proper functioning of the environment. The methods of selection of the GCMs [44, 23, 41, 32, 40, 43] was to support analysis of the full range of impacts, without using all available GCMs.
The selected GCMs were already downscaled to a few synoptic climate stations in Africa by CSAG [18] as part of the World Climate Research Programme (WCRP) [33] Coordinated Regional Climate Downscaling Experiment for Africa (CORDEX-Africa). The scenario datasets used for the study formed part of the Coupled-Model Inter-comparison Phase five (CMIP5) project, which were used for the Fifth Assessment Report (AR5) of the IPCC [30]. The RCP8.5 scenario was selected because it prescribes a continual global warming into the 21st century through increasing radiative forcing and greenhouse gas emissions [46], which is consistent with historical climate trends in West Africa [28]. Based on recent analysis [31], this trend of environmental degradation and climate change may continue to 2100, increasing the greenhouse gas emissions from the region, making the RCP8.5 the most suitable climate scenario for the region.
Since the CSAG data had no downscaled climate stations within the Bonsa catchment, three nearby and surrounding synoptic climate stations (Figure 1) in Accra (Ghana), Adiake and Bondoukou (Ivory Coast), which had downscaled GCM climate records were selected and the monthly mean of rainfall, minimum and maximum temperatures were computed and used for further downscaling
Table 1. Initial (a) and final (b) land use parameters
VEGINT
Land use (mm.rain/day) ROOTAa CAYa COIAMa PCSUCO**
(a) Shrubs/farms
2.00*
0.80*
0.75*
0.20*
70.00
Evergreen and Secondary forests Settlements and
4.00*
1.80*
0.70*
0.80*
0.80*
0.75*
0.35*
0.20*
80.0 & 70.0
0.00
Mining areas
(b)
Shrubs/farms 2.00
0.80
0.60
0.20
70.00
Evergreen 5.00
0.70
1.00
0.54
80.0
Secondary 4.00
0.70
0.80
0.42
70.00
Mining areas 1.80
0.80
0.55
0.20
0.20
Settlements 1.80
0.80
0.55
0.20
0.00
VEGINT
Land use (mm.rain/day) ROOTAa CAYa COIAMa PCSUCO**
(a) Shrubs/farms
2.00*
0.80*
0.75*
0.20*
70.00
Evergreen and Secondary forests Settlements and
4.00*
1.80*
0.70*
0.80*
0.80*
0.75*
0.35*
0.20*
80.0 & 70.0
0.00
Mining areas
(b)
Shrubs/farms 2.00
0.80
0.60
0.20
70.00
Evergreen 5.00
0.70
1.00
0.54
80.0
Secondary 4.00
0.70
0.80
0.42
70.00
Mining areas 1.80
0.80
0.55
0.20
0.20
Settlements 1.80
0.80
0.55
0.20
0.00
Monthly Parameter Values (Jan-Dec)
forest forests
**Values obtained from field observations, *adapted from Schulze [51], a Parameter values are fractions, they have no units
Fig 3. Model performance metrics showing (a and b) hydrograph, (c and d) flow duration curves and (e and f) scatter plot between runoff coefficients, respectively for observed and simulated streamflows, during calibration and validation
limitation is that it assumes that the variability of the future climate will be same as the control observed climate [23] and in applying the method relative climate changes are assumed to be more important than absolute changes. The change factor method is therefore limited as it does not account for possible changes in intensity, duration and frequencies of rainfall events [49]. Thus, he results obtained in this study are only a contextualized scenario of the potential climate change impacts on long-term annual and seasonal hydrology. More suitable downscaling methods such as spatially disaggregated and random cascades [54, 26] and Regional Climate Modelling [33] could have been used, but for lack of long- term predictor data and computational resources.
Fig 4. Baseline land use (1991), 2011 and future land use scenarios in the Bonsa Catchment, generated from
Results
-
RESULTS AND DISCUSSION
mapping and land use simulation [4]
to the Bonsa catchment using the change factor method of statistical downscaling [24, 16]. For the rainfall, the change factor was calculated by dividing the monthly mean of each selected GCM, as well as the multi-model median, for the near (2020-2039) and far (2060-2079) future by the corresponding monthly values for the control period (1990-2009). The change factor at the monthly time scale was then applied to the daily observed rainfall records of Bonsa catchment for the control period (1990-2009) to obtain climate change scenarios (Figure 5). For the minimum and maximum temperatures, the near and far future values were subtracted from their corresponding control period values and the difference was applied to the observed daily records.
Fig 5. Downscaled and observed monthly mean rainfall for Tarkwa
The change factor method of downscaling is the most widely used method for impact analysis [16], however, its
Model Calibration and Validation: After daily simulation using the ACRU model, the results (Figure 3a) showed that the timing of flood peaks was well simulated in some places, but their magnitudes were poorly simulated and in some places the flood peaks were completely missed due to poor rain gauge coverage (Figure 1). Consequently, analysis of the daily simulation produced less satisfactory performance (e.g. Nash-Sutcliffe efficiency (NSE) indices of 0.1 and 0.3 during calibration and validation). Hence, the remaining analysis of the modelling results as well as application of the ACRU model was done at the monthly time step. Figure 3 shows the performance of the ACRU model at the monthly time step. As can be observed the model performance was satisfactory as the NSE (0.6, 0.5), PBIAS (-0.1, 10.0%), R2 (0.8, 0.7) for
calibration and validation were within the recommended limits for satisfactory model performance [45], while the FDC, runoff coefficients as well as the hydrograph, all indicate that during calibration and validation, the observed and simulated streamflows corresponded well. The model calibration in this study relied on relatively less data, which is also physically meaningful and readily available for most data scarce regions. As the successful model calibration was achieved by varying parameters within their physical limits and because ACRU model is not a parameter optimising model, but rather depend mainly on physical catchment variables [5], the validated model is useful for exploring basic hydrological responses to land use and climate in the Bonsa catchment.
Climate Change Impacts: Climate changes in the Bonsa catchment under the selected scenarios lead to increased temperatures. For rainfall, however, there were reductions for the first half of the year, but increases were projected for the second half (Figure 5). Projections in rainfall for the GCM scenarios with the 25th and 75th percentile change in MAP were however, inconsistent, compared with the other scenarios, as the near future monthly rainfalls for the 25th percentile scenario were higher than those of the 75th percentile scenario. The sections below describe the temporal
and spatial patterns of the impacts of the selected climate change scenarios on streamflows of the Bonsa catchment.
Temporal Patterns of Climate Change Impacts: Annual streamflows as well as monthly median flows, high and low flows during the major peak season, reduced in the near future climate change scenarios, but streamflows increased under the far future climate change scenarios (Table 3 and Figure 6). The wet scenarios generated the highest streamflows, while the dry scenarios produced the lowest. Furthermore, for accumulated mean monthly streamflows, the trends for all the scenarios were similar (Figure 6). The results generally appear
impacts were lower than those under separate land use changes, regardless of the time slice. The results also indicate that the combined impacts of land use and climate change in the Bonsa catchment are additive of the separate impacts. For example, the change in annual streamflows for the BAU land use scenario of 32.1% and the corresponding impacts under the multi-model climate change scenario (2020-2039) of – 4.8%, sum closely to the annual change in combined impacts under the multi-model 2020-2039 and BAU land use of 27.7% (Table 1 Appendix 1).
to indicate longer dry seasons particularly for the far future and shorter wet seasons, despite the higher magnitudes of the
Accumulated mean streamflows(m3/month)
Accumulated mean streamflows(m3/month)
1400 Baseline Wet
Multi-model median
(a)
Accumulated mean streamflows (m3/month)
Accumulated mean streamflows (m3/month)
1400 Baseline Wet
Multi-model median
(b)
peak season flows. The lengthening of the dry season, shortening of the rainy season and increase in flows during the minor peak season could reduce agriculture productivity in the Bonsa catchment as the growing period may reduce and the risk of flooding during the minor peak season will be higher than it currently is. Hence, impacts of climate change could
1200
1000
800
600
400
200
0
Dry
Jan Feb Mar Apr May Jun Jul Aug Sep Oct Nov Dec
1200
1000
800
600
400
200
0
Dry
Jan Feb Mar Apr May Jun Jul Aug Sep Oct Nov Dec
be a food security challenge in the catchment.
1400 Baseline
Accumulated mean streamflows(m3/month)
Accumulated mean streamflows(m3/month)
75th percentile
(c)
Accumulated mean streamflows(m3/month)
Accumulated mean streamflows(m3/month)
1400 Baseline
75th percentile
(d)
1200
25th percentile Multi-model median
1200
25th percentile Multi-model median
Spatial Patterns of Climate Change Impacts: An example of how streamflow responses to climate changes are distributed within the Bonsa catchment under the near future (2020 – 2039) wet and dry GCM scenarios is shown in Figure
7. For the wet scenario, the streamflow changes ranged from
1000
800
600
400
200
0
Jan Feb Mar Apr May Jun Jul Aug Sep Oct Nov Dec
1000
800
600
400
200
0
Jan Feb Mar Apr May Jun Jul Aug Sep Oct Nov Dec
no change to a 5 to 25% increase, while for the dry GCM scenario there was 5 to 27% streamflow reduction. It is evident that subcatchments in the main stem of the Bonsa River experienced similar change (5 to 15%) in streamflows under the wet scenario, but under the dry scenario these subcatchments showed a 15 to 27% reduction in streamflow (Figure 7). These subcatchments constituted more than 50% of the catchment, hence they influenced the outlet streamflows in the respective scenarios.
Temporal Patterns of Combined Future Climate and Land Use Change Impacts: The annual, seasonal as well as high, median and low streamflows of the Bonsa catchment increased under the combined future land use and climate change scenarios (Figure 8). The differences in streamflows associated with the future land use scenarios were small, with larger differences between the climate change scenarios. The streamflows associated with the BAU combined land use and climate change scenarios were slightly higher than those associated with the EG and EGR scenarios. The combined scenarios associated with the wet climate change scenario generated the highest streamflows, while those associated with the dry climate change scenarios generated the lowest streamflows, regardless of the time slice and land use involved. Minor reductions (up to 8%) in streamflows occurred in the major peak season, with higher increases in flows occurring in the minor peak season.
Under wet scenarios, the combined impacts of land use and climate change had higher increases in annual streamflows than those under the separate land use change impacts, but under a dry climate scenario, the combined
Fig 6. Accumulated mean monthly streamflows for climate change scenarios for the near future (20202039) (a,
c) and far future (2060 2079) (b, d), compared with the baseline streamflows
Spatial Patterns of Combined Future Climate and Land Use Change Impacts: The spatial patterns of combined land use and climate change for the near future wet and dry climate scenarios are shown in Figure 9. Under the BAU, EG and the EGR land uses and wet climate scenario, subcatchments along the main stem of the Bonsa River experienced streamflow changes between 5 to >75% for the northern and 40 to 55% for the southern areas. The increase in streamflows along the main stem of the river is because the land use changed from secondary forests in 1991 to shrubs/farms in the future land use scenarios (Figure 4). For subcatchment 88 and its surrounding areas in the west central part of the catchment, streamflow increases ranged from 45 to 65% under the BAU, EG and the EGR scenarios with the wet climate. The large increase in streamflows in this west central part of the catchment was due to the expansion of the settlements (Tarkwa), mining areas (east and west of Tarkwa) and
Table 2. Selected IPCC CMIP5 AR5 GCMs based on Changes in Projected MAP in 2060 – 2079 relative to the 1990 2009 Control Period for Adiake, Bondoukou and Accra in West Africa
Modelling Centre
GCM
Change in MAP (%)
Variable label
Scenarios (from CSAG)
Japan Agency for Marine-Earth Science and Technology, Atmosphere and Ocean
MIROC- ESM*
-3.8
Dry
8.5RCP
Research Institute (The University of
Tokyo), and National Institute for
Environmental Studies
Centre National de Recherches Meteorologiques / Centre Europeen de
CNRM- CM5*
8.6
Wet
4.5/8.5RCP
Recherche et Formation Avancees en Calcul
Scientifique
Canadian Centre for Climate Modelling and
CanESM2
5.2
4.5/8.5RCP
Analysis
LASG, Institute of Atmospheric Physics,
FGOALS-
6.6
4.5/8.5RCP
Chinese Academy of Sciences
s2
College of Global Change and Earth System
BNU-ESM
1.7
4.5/8.5RCP
Science, Beijing Normal University
Atmosphere and Ocean Research Institute (The University of Tokyo), National Institute
MIROC5*
1.1
25th Percentile
4.5/8.5RCP
for Environmental Studies, and Japan
Agency for Marine-Earth Science and
Technology
Geophysical Fluid Dynamics Laboratory,
GFDL-
1.8
4.5/8.5RCP
USA
ESM2G
Japan Agency for Marine-Earth Science and
MIROC-
0.9
4.5/8.5RCP
Technology, Atmosphere and Ocean
ESM-
Research Institute (The University of
CHEM
Tokyo), and National Institute for
Environmental Studies
Geophysical Fluid Dynamics Laboratory, USA
*Selected GCMs
GFDL- ESM2M*
5.4 75th
Percentile
4.5/8.5RCP
Fig 7. Changes in mean annual streamflows relative to baseline (1991 land use and 1990-2009 climate) for 2020-2039 wet and dry climate change scenarios. Numbers in map indicate subcatchments
Table 3. Change (%) in Streamflows Relative to the Baseline (land use & climate) for all Climate Scenarios
(a)
Time Climate
slice scenario/GCM
Annual
Dry season
Major peak
Minor peak
Wet
13.2
34.1
-17.1
61.7
2039 Multi-model median
-4.8
6.9
-19.4
15.5
Dry
-15.3
-12.9
-32.2
14.3
Wet
33.9
46.0
8.2
77.9
2079 Multi-model median
0.8
28.8
-24.1
33.5
Dry
-13.6
2.6
-30.0
13.8
(b)
Change in streamflows (%) from baseline climate
Time Climate
slice scenario/GCM
Annual
Dry season
Major peak
Minor peak
75th percentile
-4.8
9.5
-21.4
17.3
2020-
2039 Multi-model median
-4.8
6.9
-19.4
15.5
25th percentile
-4.7
17.0
-29.0
29.9
75th percentile
18.4
51.4
-17.4
62.2
2060- Multi-model median
0.8
28.8
-24.1
33.5
25th percentile
4.8
20.9
-22.2
45.4
Time Climate
slice scenario/GCM
Annual
Dry season
Major peak
Minor peak
Wet
13.2
34.1
-17.1
61.7
2039 Multi-model median
-4.8
6.9
-19.4
15.5
Dry
-15.3
-12.9
-32.2
14.3
Wet
33.9
46.0
8.2
77.9
2079 Multi-model median
0.8
28.8
-24.1
33.5
Dry
-13.6
2.6
-30.0
13.8
(b)
Change in streamflows (%) from baseline climate
Time Climate
slice scenario/GCM
Annual
Dry seasn
Major peak
Minor peak
75th percentile
-4.8
9.5
-21.4
17.3
2020-
2039 Multi-model median
-4.8
6.9
-19.4
15.5
25th percentile
-4.7
17.0
-29.0
29.9
75th percentile
18.4
51.4
-17.4
62.2
2060- Multi-model median
0.8
28.8
-24.1
33.5
25th percentile
4.8
20.9
-22.2
45.4
Change in streamflows (%) from baseline climate
2020-
2060-
2079
3000
Baseline
Near future Far future
2011 LU + Obs. clim.
(a) 90 & 10th
Streamflows (m3/month)
Streamflows (m3/month)
3000
Baseline Near future Far future
2011 LU + Obs. clim.
(b) 90 & 10th
3000
Baseline Near future Far future
2011 LU + Obs. clim.
(c) 90 & 10th
Streamflows (m3/month)
Streamflows (m3/month)
2000
2000
Streamflows (m3/month)
Streamflows (m3/month)
2000
1000
1000
1000
0
Jan Feb Mar Apr May Jun Jul Aug Sep Oct Nov Dec
0
Jan Feb Mar Apr May Jun Jul Aug Sep Oct Nov Dec
0
Jan Feb Mar Apr May Jun Jul Aug Sep Oct Nov Dec
3000
Baseline
Near future Far future
2011 LU + Obs. clim.
(d) 90 & 10th
3000
Baseline Near future Far future
2011 LU + Obs. clim.
(e) 90 & 10th
Streamflows (m3/month)
Streamflows (m3/month)
Streamflows (m3/month)
Streamflows (m3/month)
2000 2000
1000 1000
0
Jan Feb Mar Apr May Jun Jul Aug Sep Oct Nov Dec
0
Jan Feb Mar Apr May Jun Jul Aug Sep Oct Nov Dec
Fig 8. Monthly percentile streamflows for combined (a) BAU+ wet, (b) BAU+ multi-model median, ( c) BAU+ dry climate change scenarios, (d) BAU + 75th percentile GCM scenario and (e) BAU+25th percentile GCM scenario, as well as baseline (1991) and 2011 Land use
increased shrubs/farms in the future scenarios, compared to the baseline 1991 land use (Figure 4).
For those subcatchments in the southwest, the middle and the northern parts of the catchment, which remain as evergreen forest in the future land use scenarios (Figure 4), there was 5 to 25% increase in simulated streamflows under the scenarios of future land use and a wet climate. There was also a 5 to 15% increase in streamflows (Figure 9) in the eastern tip, north western tip and the northern tip of the catchment in subcatchments which were mostly covered by evergreen forests in both the historical land use and the future land uses (Figure 4). In all the scenarios involving the wet climate, subcatchment 54 and its surrounding areas had the highest overall increases in streamflows. The outlet streamflows with respect to the BAU and wet climate scenario increased between 45 to 55%, while those with respect to the EG and the EGR increased by between 35 to 45% only. The difference between the land use scenarios under wet climate change is that the BAU show higher magnitudes of increases in streamflows for subcatchments 88, 54 and their surrounding areas, compared to the EG and the EGR land uses.
Figure 9 also shows that the various land use scenarios under the dry climate change exhibited similar patterns as the streamflow changes under the wet climate change, except that there were streamflow reductions (between 5 to 26%) in subcatchments which either experienced no changes or reductions, under the wet climate and land use scenarios. The streamflow increases along the main stem of the river under the dry climate also appear to dampen towards the outlet of Bonsa catchment. It can therefore be deduced from Figure 9 that the variability of streamflow changes within the subcatchments increased as land use and climate changes occurred simultaneously, but the variability of the streamflow changes at the outlet of the catchment does not experience significant changes under combined land use and climate changes.
Discussion
Combined Climate and Land Use Change Impacts on Hydrology: The study analysed the separate impacts of climate change, as well as the combined impacts of climate and land use change on the hydrology of the Bonsa catchment, Ghana, West Africa, which to the authors knowledge is the first time such a study has been conducted in the catchment. The study revealed that the effects of climate change for the near future suggests a reduction in streamflows during the peak season as well as at annual time scale, but there may be increases in streamflows for all time scales under the far future climate scenarios and a lengthening of the dry season (Dec- May), but a shortening of the wet season (major peak) in the far future, which can affect water availability for domestic, industrial and environmental flows.
On the other hand, streamflow increases due to combined land use and climate changes were higher than the forested 1991 baseline and 2011 streamflows. These increases in streamflows were evident across the seasons regardless of the land use or climate change scenario. Notwithstanding the
increased streamflows under the combined scenarios, in the far future the length of the wet season may reduce, while that of the dry season may increase (Figure 8), which have the potential to reduce water availability for domestic, industry and agricultural uses. The streamflow responses for the combined scenarios involving the different land use scenarios (BAU, EG and EGR) were similar, although the scenarios involving the BAU had slightly higher streamflows than the rest. This is mainly because although the secondary forest areas in the potential future land uses were different, the proportion of the shrubs/farms, which is the dominant land use in terms of both area and runoff generation, were not substantially different between the scenarios. Given the significant land use changes that already occurred between 1991 and 2011, the land use changes in the future in comparison will not significantly alter outlet streamflows assuming land cover changes as predicted by the land use model.
The study showed that the combined impact of land use and climate changes are additive of the separate impacts, unlike studies such as Li et al. [38] where the joint impacts were non-linear. The study also reveals that when there is substantial increase in rainfall (under the wet climate scenario), climate largely controls streamflow changes, but when there is less rain (under dry climate scenario), land use controls streamflow changes. This is because under the wet climate and land use change, the increase in streamflows were higher than those under separate land use change, but under a dry climate and land use change, the impacts were higher than those under separate land use change (Table 1 Appendix 1).
Furthermore, the study revealed that the variability of climate change impacts at the catchment and subcatchment scales were also almost the same (Figure 7 and Table 1 Appendix 1), as the streamflow changes at the two scales did not differ much. Variability of streamflow changes was greater at the subcatchment than at the catchment scale under simultaneous land use and climate change. Hence, it is relevant to not only focus on the outlet streamflows when considering global change impacts, and basing catchment management plans on outlet streamflow changes alone can result in less effective adaptation measures.
The results of this study contradict thoseof previous studies in West Africa, where Bossa et al. [11] concluded that streamflow responses to combined impacts of climate change and land use changes were higher than climate change impacts, but less than separate land use change impacts. Bossa et al. [12], also concluded that climate was the dominant factor in streamflow changes in a savannah dominated catchment in Benin Republic. Hence, the effects of global changes revealed in this study (rainforest catchment) and others (mainly savannah catchments) in West Africa, portray within-region differences of hydrological impacts under changing conditions and provides a platform for further studies. The results of this study also disprove the hypothesis that joint impacts of land use and climate changes are non- linear [38].
Fig 9. Changes in mean annual streamflows relative to baseline (1990 – 2009) for combined land use change (2030) and 2020 – 2039 wet and dry climate change scenarios. Numbers in map indicate selected subcatchments
Uncertainties in Assessing Land Use and Climate Change Impacts: Semi-distributed hydrological models are the most widely used tools for studying the impacts of climate change and land use change on hydrology, separately and jointly in regions with diverse land uses and climates [13, 37, 23, 61]. This is because semi-distributed models simulate the hydrological cycle physically, spatially and temporally, to generate information for effective land use planning and water management decisions. The use of the physical-conceptual and semi-distributed ACRU model in this study hinged on the fact that it is sensitive to both land use and climate changes
[51] as well as being able to generate hydrological responses both at the subcatchment and catchment scales.Apart from the uncertainties with hydrological models, the uncertainties with climate change and land use input data also affects the impact assessments. Previous studies [14, 57] show that the uncertainties associated with GCM climate change scenarios are greater than those related to hydrological models. In West Africa, the rainfall estimation by climate models is particularly problematic, as some models predict increases in the coastal areas and decreases in the semi-arid, others predict the opposite [8]. Since rainfall is the major driver of the hydrology of humid regions and is the most sensitive parameter in the ACRU model, the future rainfall estimates, as well as the use of the change factor
downscaling method, constitute the most significant source of uncertainty in the simulated streamflows in this study.
However, as the study used five GCM climate scenarios (wet, 25th percentile, 75th percentile, dry and multi- model median) to quantify the uncertainties with climate changes, a contextualised scenario of alternative futures, upon which different catchment planning scenarios can be evaluated, have been provided. Another source of uncertainty with this study is the modelled future land uses. Since the land use modelling was based on historical land use data, the far future (2070) land use scenarios, are more uncertain, compared to the near (2030) future land uses. Although the use of modelled future land use introduces uncertainties into the simulated streamflows, land use modelling is the most realistic method to provide plausible future land use scenarios, as it relies on statistically significant socio-economic and biophysical driving factors. Land use modelling also ensures that gradual changes, including simultaneous regeneration and removal of land use types at different locations within a catchment, are accounted for. This ensures that realistic change processes, previously witnessed in a catchment are applied. This study used three land use scenarios (BAU, EG and EGR), which provide alternative development pathways for the catchment, to quantify the uncertainties with the land use.
Furthermore, uncertainties have also been introduced due to the use of a coarse resolution soil map (scale: 1: 250
000) and the lack of locally measured soil hydrological properties, as well as lack of vegetation parameters for the Bonsa catchment. Uncertainties in the observed climate records also contributed to the uncertainties in the hydrological modelling. These were as a result of several factors including sparse climate station network (stations concentrated in settlements areas), erroneous rainfall records as well as missing data. Lack of long-term and complete streamflow records also contributed to simulation uncertainties. There are several water users in the Bonsa catchment including mining companies, industries and individual homes, however, there is no data on the quantity and location of water withdrawals. Hence the uncertainties in the hydrological modelling was also because it was not possible to account for these surface/ground water withdrawals during simulations. However, since the erroneous rainfall removal, sensitivity analysis and the hydrological modelling approach used in the companion paper
[3] resulted in satisfactory calibration and validation, the ACRU model can be used for exploring basic hydrological responses to land uses and climate. -
CONCLUSIONS AND RECOMMENDATIONS
Conclusions: This study has shown that in the Bonsa catchment, under climate change, streamflows may reduce in the near future, but increase in the far future, compared to the forested (1991) baseline conditions. Under combined land use and climate change scenarios, streamflow increases relative to the forested (1991) baseline, were higher, compared to the climate change scenarios, irrespective of the time slice. The impacts of simultaneous land use and climate changes on streamflows were higher than those under separate land use changes when there was considerable increase in rainfall, but lower when there was less rainfall. This means climate will control streamflow changes when there is substantial increase in rainfall, but land use will control the streamflows changes if rainfall reduces. The streamflow responses in the combined scenarios with respect to the different future land use (LU) scenarios were however, not substantially different, for a particular LU scenario to be selected over the other. In terms of spatial variability of global change impacts, the streamflow changes had similar patterns especially along the main stem of the river. When land use and climate changes occur simultaneously, the streamflow increase were higher and the spatial variability of streamflow changes was higher than when only climate changes are considered.
The use of both multiple future land uses and climate change scenarios in the impact assessments has provided a range of first stage estimation of potential global change impacts on the hydrology of Bonsa catchment, which policy makers can use for long-term land use and environmental planning, as the various scenarios can be evaluated against a set of planning objectives for the catchment.
REFERENCES
-
Aduah, M, Jewitt, G and Toucher, M. (2018a), Assessing Impacts of Land Use Changes on the Hydrology of a Lowland Rainforest Catchment in Ghana, West Africa. Water 10(1):9.
-
Aduah, MS. (2016), Impacts of Global Changes on a lowland rainforest region of West Africa, Unpublished PhD Thesis, Centre for Water Resources Research (CWRR), School of Agricultural, Earth and Environmental Sciences, University of KwaZulu-Natal, Pietermaritzburg, South Africa.
-
Aduah, MS, Jewitt, GPW and Toucher, MLW. (2017),Assessing suitability of the ACRU hydrological model in a rainforest catchment in Ghana, West Africa, Water Science, 31(2):198-214.
-
Aduah, MS, Toucher, ML and Jewitt, GPW. (2018b), Estimating potential future land use in the Bonsa catchment, Ghana, West Africa, South African Journal of Geomatics, 7(3): 279-291.
-
Aduah, MS, Warburton, ML and Jewitt, G. (2015), Analysis of land cover changes in the Bonsa catchment, Ankobra Basin, Ghana, Applied Ecology and Environmental Research, 13(4: 935-955.
-
Akabzaa, TM, Jamieson, HE, Jorgenson, N and Nyame, K. (2009), The Combined Impact of Mine Drainage in the Ankobra River Basin, SW Ghana, Mine Water and the Environment, 28(1):50-64.
-
Alo, CA and Wang, G. (2010), Role of dynamic vegetation in regional climate predictions over western Africa, Climate Dynamics, 35(5):907-922.
-
Ardoin-Bardin, S, Dezetter, A, Servat, E, Paturel, JE, Mahe, G, Niel, H and Dieulin, C. (2009), Using general circulation model outputs to assess impacts of climate change on runoff for large hydrological catchments in West Africa, Hydrological Sciences Journal-Journal Des Sciences Hydrologiques, 54(1):77-89.
-
Asomaning, G. (1992), Groundwater resources of the Birim basin in Ghana, Journal of African Earth Sciences (and the Middle East), 15(34):375-384.
-
Barbier, EB. (2000), Links between economic liberalization and rural resource degradation in the developing regions, Agricultural Economics, 23(3):299-310.
-
Bossa, AY, Diekkrueger, B and Agbossou, EK. (2014), Scenario- Based Impacts of Land Use and Climate Change on Land and Water Degradation from the Meso to Regional Scale, Water, 6(10):3152- 3181.
-
Bossa, AY, Diekkrueger, B, Giertz, S, Steup, G, Sintondji, LO, Agbossou, EK and Hiepe, C. (2012), Modeling the effects of crop patterns and management scenarios on N and P loads to surface water and groundwater in a semi-humid catchment (West Africa), Agricultural Water Management, 115(2012):20-37.
-
Breuer, L, Huisman, JA, Willems, P, Bormann, H, Bronstert, A, Croke, BFW, Frede, HG, Gräff, T, Hubrechts, L, Jakeman, AJ, Kite, G, Lanini, J, Leavesley, G, Lettenmaier, DP, Lindström, G, Seibert, J, Sivapalan, M and Viney, NR. (2009), Assessing the impact of land use change on hydrology by ensemble modeling (LUCHEM). I: Model intercomparison with current land use, Advances in Water Resources, 32(2):129-146.
-
Buytaert, W, Vuille, M, Dewulf, A, Urrutia, R, Karmalkar, A and Celleri, R. (2010), Uncertainties in climate change projections and regional downscaling in the tropical Andes: implications for water resources management, Hydrology and Earth System Sciences, 14(7):1247-1258.
-
California EPA. (2011), Guidance compendium for watershed monitoring and assessment, Section 5.0 – Measurements taken in a watershed, section 5.1.3- Runoff coefficient fact sheet [Online]. California EPA. California, USA: Available: http://www.waterboards.ca.gov/water_issues/programs/swamp/docs/c wt/guidance/513.pdf [Accessed 30th March 2015].
-
Chen, J, Brissette, FP and Leconte, R. (2011), Uncertainty of downscaling method in quantifying the impact of climate change on hydrology, Journal of Hydrology, 401(3-4):190-202.
-
Costa, MH, Botta, A and Cardille, JA. (2003), Effects of large-scale changes in land cover on the discharge of the Tocantins River, Southeastern Amazonia, Journal of Hydrology, 283(14):206-217.
-
CSAG. (2015), Future climate projections [Online], Climate System Analysis Group, University of Cape Town. Cape Town, South Africa: Available:
http://cip.csag.uct.ac.za/webclient2/datasets/africa-merged- cmip5/#nodes/cmip5-anomalies?folder_id=33&extent=99799 [Accessed 24th August 2015].
-
CWRR. (2018), ACRU Theory Manual [Online], South Africa: Available: http://cwrr.ukzn.ac.za/resources/acru [Accessed 15th May 2018].
-
D'Orgeval, T and Polcher, J. (2008), Impacts of precipitation events and land-use changes on West African river discharges during the years 1951-2000, Climate Dynamics, 31(2-3):249-262.
-
Dale, VH. (1997), The relationship between land-use change and climate change, Ecological Applications, 7(3):753-769.
-
FAO. (2010), Global Forest Resources Assessment 2010, Main report, Food and Agriculture Organization of the United Nations, Rome, Italy.
-
Forbes, KA, Kienzle, SW, Coburn, CA, Byrne, JM and Rasmussen, J. (2011), Simulating the hydrological response to predicted climate
change on a watershed in southern Alberta, Canada, Climatic Change, 105(3-4):555-576.
-
Fowler, HJ, Blenkinsop, S and Tebaldi, C. (2007), Linking climate change modelling to impacts studies: recent advances in downscaling techniques for hydrological modelling, International Journal of Climatology, 27(12):1547-1578.
-
Graham, LP, Andersson, L, Horan, M, Kunz, R, Lumsden, T, Schulze, R, Warburton, M, Wilk, J and Yang, W. (2011), Using multiple climate projections for assessing hydrological response to climate change in the Thukela River Basin, South Africa, Physics and Chemistry of the Earth, 36(14-15):727-735.
-
Groppelli, B, Bocchiola, D and Rosso, R. (2011), Spatial downscaling of precipitation from GCMs for climate change projections using random cascades: A case study in Italy, Water Resources Research, 47: 1-18.
-
Guzha, AC, Rufino, MC, Okoth, S, Jacobs, S and Nóbrega, RLB. (2018), Impacts of land use and land cover change on surface runoff, discharge and low flows: Evidence from East Africa, Journal of Hydrology: Regional Studies, 15:49-67.
-
Hulme, M, Doherty, R, Ngara, T, New, M and Lister, D. (2001), African climate change: 1900-2100, Climate Research, 17(2):145- 168.
-
IPCC. (2007), Climate Change 2007: The Physical Science Basis. Contribution of Working Group I to the Fourth Assessment Report of the Intergovernmental Panel on Climate Change, In: Solomon, S, Qin, D, Manning, M, Chen, Z, Marquis, M, Averyt, KB, Tignor, M and Miller, HL (eds.). Cambridge University Press, Cambridge, United Kingdom and New York, NY, USA.
-
IPCC. (2013), Summary for Policymakers, In: Stocker, TF, Qin, D, Plattner, G-K, Tignor, M, Allen, SK, Boschung, J, Nauels, A, Xia, Y, Bex , V and Midgley, PM (eds.) Climate Change 2013: The Physical Science Basis. Contribution of Working Group I to the Fifth Assessment Report of the Intergovernmental Panel on Climate Change, Cambridge, United Kingdom and New York, NY, USA.
-
Jalloh, A, Faye, MD, Roy-Macauley, H, Sérémé, P, Zougmoré, R, Thomas, TS and Nelson, GC. (2013), Overview, In: Jalloh, A, Nelson, G. C., Thomas,T. S., Zougmoré,R. And Roy-Macauley, H. (ed.) West African Agriculture and Climate Change: A Comprehensive Analysis, International Food Policy Research Institute(IFPRI), Washington, DC, USA.
-
Kienzle, SW, Nemeth, MW, Byrne, JM and MacDonald, RJ. (2012), Simulating the hydrological impacts of climate change in the upper North Saskatchewan River basin, Alberta, Canada. Journal of Hydrology, 412413(2012):76-89.
-
Kim, J, Waliser, DE, Mattmann, CA, Goodale, CE, Hart, AF, Zimdars, PA, Crichton, DJ, Jones, C, Nikulin, G, Hewitson, B, Jack, C, Lennard, C and Favre, A. (2013), Evaluation of the CORDEX- Africa multi-RCM hindcast: systematic model errors, Climate Dynamics, 42(56):11891202.
-
Kunstmann, H, Schneider, K, Forkel, R and Koche., R. (2004), Impact analysis of climate change on an Alpine catchment using high resolution dynamic downscaling of ECHMAM4 time slices, Hydrology and Earth System Sciences, 8(6):1030 – 1044.
-
L'Hote, Y, Mahe, G, Some, B and Triboulet, JP. (2002), Analysis of a Sahelian annual rainfall index from 1896 to 2000; the drought continues, Hydrological Sciences Journal-Journal Des Sciences Hydrologiques, 47(4):563-572.
-
Leblanc, MJ, Favreau, G, Massuel, S, Tweed, SO, Loireau, M and Cappelaere, B. (2008), Land clearance and hydrological change in the Sahel: SW Niger, Global and Planetary Change, 61(3-4):135- 150.
-
Legesse, D, Abiye, TA, Vallet-Coulomb, C and Abate, H. (2010), Stream flow sensitivity to climate and land cover changes: Meki River, Ethiopia, Hydrology and Earth System Science, 14(2010): 2277-2287.
-
Li, Z, Liu, W, Zhang, X and Zheng, F. (2009), Impacts of land use change and climate variability on hydrology in an agricultual catchment on the Loess Plateau of China, Journal of Hydrology, 377(2009):35-42.
-
Liu, X, Coulibaly, P and Evora, N. (2008), Comparison of data- driven methods for downscaling ensemble weather forecasts, Hydrology and Earth System Sciences, 12(2):615-624.
-
Lutz, AF, ter Maat, HW, Biemans, H, Shrestha, AB, Wester, P and Immerzeel, WW. (2016), Selecting representative climate models for climate change impact studies: an advanced envelope-based selection approach, International Journal of Climatology, 36: 39884005.
-
Mango, LM, Melesse, AM, McClain, ME, Gann, D and Setegn, SG. (2011), Land use and climate change impacts on the hydrology of the upper Mara River Basin, Kenya: results of a modeling study to support better resource management, Hydrology and Earth System Science, 15(2011):2245-2258.
-
Maurer, EP and Hidalgo, HG. (2008), Utility of daily vs. monthly large-scale climate data: an intercomparison of two statistical downscaling methods, Hydrology and Earth System Sciences, 12(2):551-563.
-
Mendlik, T and Gobiet, A. (2016), Selecting climate simulations for impact studies based on multivariate patterns of climate change, Climatic Change, 135381-393.
-
Moradkhani, H, Baird, RG and Wherry, SA. (2010), Assessment of climate change impact on floodplain and hydrologic ecotones, Journal of Hydrology, 395(3-4):264-278.
-
Moriasi, DN, Arnold, JG, Van Liew, MW, Bingner, RL, Harmel, RD and Veith, TL. (2007), Model evaluation guidelines for systematic quantification of accuracy in watershed simulations, Transactions of the Asabe, 50(3):885-900.
-
Moss, RH, Edmonds, JA, Hibbard, KA, Manning, MR, Rose, SK, van Vuuren, DP, Carter, TR, Emori, S, Kainuma, M, Kram, T, Meehl, GA, Mitchell, JFB, Nakicenovic, N, Riahi, K, Smith, SJ, Stouffer, RJ, Thomson, AM, Weyant, JP and Wilbanks, TJ. (2010), The next generation of scenarios for climate change research and assessment, Nature, 463(7282):747-756.
-
Mwangi, HM, Julich, S, Patil, SD, McDonald, MA and Feger, K-H. (2016), Relative contribution of land use change and climate variability on discharge of upper Mara River, Kenya, Journal of Hydrology: Regional Studies, 5244-260.
-
Quintana Segui, P, Ribes, A, Martin, E, Habets, F and Boe, J. (2010), Comparison of three downscaling methods in simulating the impact of climate change on the hydrology of Mediterranean basins, Journal of Hydrology, 383(1-2):111-124.
-
Ruelland, D, Ardoin-Bardin, S, Collet, L and Roucou, P. (2012), Simulating future trends in hydrological regime of a large Sudano- Sahelian catchment under climate change, Journal of Hydrology, 424-425(2012):207-216.
-
Schmidt, J, Kienze, SW and Srinivasan, MS. (2009), Estimating increased evapotranspiration losses caused by irrigated agriculture as part of the water balance of the Orari catchment, Canterbury, New Zealand, Journal of Hydrology (Wellington North), 48(2):73-94.
-
Schulze, RE. (1995), Hydrology and Agrohydrology: A Text to Accompany the ACRU 3.00 Agrohydrological Modelling System, Water Research Commission, Pretoria, South Africa.
-
Schulze, RE. (2000), Modelling hydrological responses to land use and climate change: A southern African perspective, Ambio, 29(1):12-22.
-
Schulze, RE. (2005), Selection of a Suitable Agrohydrological Model for Climate Change Impact Studies over Southern Africa. In: Schulze, RE (ed.) Climate Change and Water Resources in Southern Africa: Studies on Scenarios, Impacts, Vulnerabilities and Adaptation, Water Research Commission, Pretoria, RSA.
-
Sharma, D, Das Gupta, A and Babel, MS. (2007), Spatial disaggregation of bias-corrected GCM precipitation for improved hydrologic simulation: Ping River Basin, Thailand, Hydrology and Earth System Sciences, 11(4):1373-1390.
-
Sood, A, Muthuwatta, L and McCartney, M. (2013), A SWAT evaluation of the effect of climate change on the hydrology of the Volta River basin, Water International, 38(3):297-311.
-
Teng, J, Chiew, FHS, Timbal, B, Wang, Y, Vaze, J and Wang, B. (2012), Assessment of an analogue downscaling method for modelling climate change impacts on runoff, Journal of Hydrology, 472(2012):111-125.
-
Thompson, JR, Green, AJ, Kingston, DG and Gosling, SN. (2013), Assessment of uncertainty in river flow projections for the Mekong River using multiple GCMs and hydrological models, Journal of Hydrology, 486(0):1-30.
-
Veettil, AV and Mishra, AK. (2016), Water security assessment using blue and green water footprint concepts, Journal of Hydrology, 542589-602.
-
Wang, G and Eltahir, EAB. (2000), Role of vegetation dynamics in enhancing the low-frequency variability of the Sahel rainfall, Water Resources Research, 36(4):1013-1021.
-
Warburton, ML, Schulze, RE and Jewitt, GPW. (2010), Confirmation of ACRU model results for applications in land use and climate change studies, Hydrology and Earth System Sciences, 14(2010):2399-2414.
-
Warburton, ML, Schulze, RE and Jewitt, GPW. (2012), Hydrological impacts of land use change in three diverse South African catchments, Journal of Hydrology, 414-415(2012):118-135.
-
Xue, YK. (1997), Biosphere feedback on regional climate in tropical north Africa, Quarterly Journal of the Royal Meteorological Society, 123(542):1483-1515.
Appendix 1
Table 1. Changes in streamflows relative to the baseline (1990-2009) for combined climate change/land use change scenarios as well as change in annual streamflows for separate land use and climate change impacts. Values in parentheses are for the 25th and 75th p percentile GCMs. A: combined impacts, B: separate impacts, LUC: land use change, CC: climate change
Land use |
Climate change |
A: Change in streamflows (%) |
B: Annual change in streamflows (%) |
Scenario Year |
Time slice Scenario/GCM |
Annual Dry season Major peak Minor peak |
LUC CC |
BAU 2030 2070 |
2020-2039 Wet (75th) Multi-model Dry (25th) 2060-2070 Wet (75th) Multi-model Dry (25th) |
47.7 (27.6) 88.7 (54.8) 12.0 (7.1) 102.4 (54.8) 27.7 54 9.5 52.5 16.0 (27.8) 34.0 (61.1) -5.4 (-1.5) 50.7 (70.0) 76.5 (60.2) 100.5 (108.6) 44.8 (14.7) 132.7 (119.1) 40.7 85.2 8.2 85.4 24.2 (44.0) 53.0 (71.0) 2.4 (9.7) 61.6 (98.0) |
13.2 (-4.8) |
32.1 -4.8 |
|||
-15.3 (-4.7) 33.9 (18.4) 39 0.8 -13.6 (4.8) |
|||
EG 2030 2070 |
2020-2039 Wet (75th) Multi-model Dry (25th) 2060-2070 Wet (75th) Multi-model Dry (25th) |
43.2 (23.5) 80.2 (47.5) 8.7 (4.0) 97.2 (50.1) 23.5 46.5 6.3 47.8 12.0 (23.6) 26.9 (53.7) -8.3 (-4.5) 46.0 (65.0) 69.2 (53.1) 88.1 (95.1) 39.6 (10.4) 123.4 (109.6) 34 72.3 3.8 77 17.9 (37.5) 41.5 (59.2) -2.0 (5.4) 54.0 (89.3) |
27.9 32.2 |
EGR 2030 2070 |
2020-2039 Wet (75th) Multi-model Dry (25th) 2060-2070 Wet (75th) Multi-model Dry (25th) |
43.4 (23.7) 80.7 (48.0) 8.8 (4.1) 97.3 (50.2) 23.7 47.1 6.5 47.9 12.2 (23.8) 27.3 (54.4) -8.2 (-4.4) 46.1 (65.1) 68.1 (52.1) 86.3 (93.2) 38.9 (9.8) 122.0 (108.2) 33 70.3 3.2 75.7 17.0 (36.5) 39.6 (57.5) -2.7 (4.8) 52.8 (88.0) |
28.1 31.2 |