
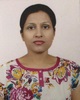
- Open Access
- Authors : Garima Garg , Sanjay Singh , Vinita Singh
- Paper ID : IJERTV9IS060999
- Volume & Issue : Volume 09, Issue 06 (June 2020)
- Published (First Online): 06-07-2020
- ISSN (Online) : 2278-0181
- Publisher Name : IJERT
- License:
This work is licensed under a Creative Commons Attribution 4.0 International License
A Two Warehouse Inventory Model for Perishable Items with Ramp Type Demand and Partial Backlogging
Garima Garg, Sanjay Singh*, Vinita Singh
Department of Applied Sciences and Humanities (Mathematics) Raj Kumar Goel Institute of Technology, Ghaziabad, India
Abstract:- In this paper, a two warehouse inventory model for perishable items with ramp type demand has been developed in which shortages are allowed and partially backlogged. The model so developed has been discussed for two scenarios: i) demand rate becomes constant before the time at which the inventory level reaches zero in rented warehouse ii) demand rate becomes constant when rented warehouse is empty and demand is fulfilled from own warehouse. Numerical example for each scenario has been solved to maximize the total profit and obtain optimal order quantity. Finally, sensitivity analysis has been carried out to analyze the behavior of presented model.
Keywords: Inventory, Two warehouse, Perishable, Ramp type, Partial Backlogging
-
INTRODUCTION
Perishable or deteriorating items are those, which have finite or limited shelf life. In inventory systems, decay or deterioration of physical goods (such as seasonal products, medicines, volatile liquids, etc.) with time is a natural phenomenon. Deterioration of goods during their normal storage period is major and realistic problem in any inventory system. Inventory models with deteriorating items have been progressively modified by various researchers in few decades to make them more practicable and realistic. The analysis of deteriorating inventory begun with Ghare and Schrader (1963), they established the classical inventory model without shortage and with a constant rate of decay. Goyal and Giri (2001) presented an excellent review in the field of inventory control of deteriorating items. Yang & Wee (2002) presented a production-inventory policy for deteriorating items with a constant production & demand rate. Ghosh and Chaudhrui (2004) developed an order level inventory model for deteriorating items considering two parameter Weibull distribution deterioration, and demand as a quadratic function of time. They solved model analytically and obtain optimal solution with a numerical example. He et al. (2010) developed an optimal production inventory model for deteriorating items, where manufacturers sold the goods to multiple markets with varying demands. An up-to-date review of published work about deteriorating inventory models for the period 2012-2015 was presented by Janssen et al. (2016). Sharma et al. (2018) analyzed an inventory model for deteriorating items assuming constant deterioration rate with expiry date and time varying cost. Khakzad and Gholamian (2020) introduced an inventory models for deteriorating items with advanced payment. In this model, they studied the effect of deteriorated items on deterioration rate of adjacent items and established a relationship between number of inspections and deterioration rate.
It is observed that demand of some useful newly launched products such as electronic goods and fashionable goods increases at the beginning and ultimately stabilizes and become constant. This kind of demand pattern seems to be quite realistic and is termed as ramp type. Thus, in case of ramp type demand, the demand increases linearly at the beginning and then the market grows into a stable stage such that the demand becomes a constant till the end of the inventory cycle. Hill (1995) first considered the inventory models for increasing demand followed by a constant demand and termed it as ramp type demand pattern. Wu (2001) developed an EOQ model with ramp type demand and partial backlogging. They assumed that partial backlogging rate depends on waiting time and next replenishment. Giri et al. (2003) extended the ramp type demand inventory model with a more generalized Weibull deterioration distribution. Manna & Chaudhuri (2006) analyzed an inventory models for deteriorating items considering ramp type demand rate, wherein the production rate is function of demand rate and deterioration rate is time proportional. Panda et al. (2008) developed an inventory model for perishable seasonal products with ramp-type demand. Skouri et al (2009) presented inventory models with ramp type demand rate, partial backlogging & Weibull deterioration rate. Sanni and Chukwu (2013) proposed an EOQ model with three parameter Wiebull distribution deterioration, shortages and ramp type demand, and established necessary and sufficient conditions for the optimal replenishment policy. Wang and Huang (2014) studied a production inventory model for a seasonal deteriorating product. In this model, demand has been considered as price and ramp type dependent. Chandra (2017) discussed an inventory model with ramp type demand, price discount and backborders in which holding cost has been expressed as linearly increasing function of time. Saha et al. (2018) analyzed an inventory model for deteriorating items with ramp type demand and price discount under the effect of partial backlogging. Yadav et al. (2020) explained an inventory model for deteriorating items with stock dependent and ramp type demand considering reserve money and carbon emission.
The classical inventory models are mainly developed with the single storage facility. It implies that the available warehouse has unlimited capacity in those models. But in practice, the capacity of any warehouse is limited. When management has to purchase (or produce) large amount of units of an item that cannot be store in the existing warehouse (i.e. own warehouse, OW) at the market place due to its limited capacity then in such a situation excess units are stored in a rented warehouse (RW) which is located at some distance away from OW. Normally inventory managers decide to hold more items than that can be stored in OW when the replenishment cost is higher than the other related costs or the demand of items is very high or the managers are obtaining a attractive price discount on bulk purchase and so on. Here it is assumed that RW is sufficiently large i.e. it can be made large as per situation. Inventories are first stored in OW with excess going to RW. But while retrieving goods for consumption, it is always from RW first and when RW is empty then the goods are retrieved from OW as the storage conditions in RW are poor than in OW and holding cost is more in RW than in OW. A two warehouse inventory model was first developed by Hartley (1976). He considered the model in which holding cost of RW is greater than that in OW. Sarma (1983) extended Hartleys model by introducing the transportation cost. Goswami & Chaudhuri (1992) developed the model with or without shortages by considering a linear demand, the equal shipment cycle. Zhou (1998) presented a two-warehouse model for deteriorating items with time-varying demand and shortages during the finite planning horizon. Dye et al. (2007) developed an inventory model for deteriorating items with capacity constraint and time-proportional backlogging rate. Rong et al. (2008) presented an optimization inventory policy for a deteriorating item with partially/fully backlogged shortages and price dependent demand under two-warehouse system. Dey et al. (2008) proposed a two storage inventory problem with dynamic demand under inflation and time value of money over finite time horizon. Maity (2011) developed a two-warehouse production inventory problem under fuzzy inequality constraints. Sharma et al. (2013) presented a two-warehouse inventory model in which they evaluated impact of reduction rate in selling price with volume flexibility. Ranjan and Uthayakumar (205) studied a two-warehouse inventory model for deteriorating items having different deterioration rates and permissible delay with exponentially increasing trend in demand. Singh et al. (2018) proposed a two-warehouse inventory model in which demand rate varies exponentially with time and deterioration of items follows two-parameter Weibull distribution under the effect of inflation. Chauhan and Yadav (2020) presented a two-warehouse inventory model wherein demand depends on stocks using genetic algorithm under the effect of inflation.
Furthermore, Shortages occur in the system when the product required by the customers is not available. In this situation, the customer either waits for next replenishment or moves to other places to buy product. The length of the waiting time for the next replenishment would determine whether the backlogging will be accepted or not. Therefore, the backlogging rate should be variable and depends on the waiting time for the next replenishment. Many researchers such as Park (1982), Hollier and Mark (1983) and Wee (1995) considered the constant partial backlogging rate whereas researchers such as Abad (2000), Chang and Dye (2001), Wang (2002), Papachristos & Skouri (2003) have modified inventory policy by considering the time proportional partial backlogging rate. Dye (2007) formulated a joint pricing and ordering policy for deteriorating inventory. Shortages were allowed and partially backlogged. San-Jose and Garcia-Laguna (2009) presented an inventory model with full backlogging and all units quantity discounts. Hsieh & Dye (2010) developed an inventory lot size model for deteriorating items under inflation with partial backlogging over a finite planning horizon. Sharma and Singh (2013) proposed an inventory model for deteriorating items with partial backlogging considering stock and selling price dependent demand rate in fuzzy environment. Dutta and Kumar (2015) presented an inventory model having time dependent demand and holding cost under partial backlogging. Singh et al. (2016) analyzed an inventory model with multivariate demands in different phases and partial backlogging. In this model, effects of customer returns and inflation have been taken in to account. Singh et al. (2017) proposed a production inventory model for deteriorating items with time dependent demand rate and demand dependent production rate considering that shortages are allowed and partially backlogged. Singh et al. (2019) developed an inventory model for deteriorating items with partial backlogging assuming incremental holding cost.
The structure of presented article is as follows. Notations and assumptions used throughout the paper have been given in Section
-
In section 3, a two ware house inventory model for perishable items with ramp type demand and partial backlogging has been formulated. In this section model is developed for two realistic scenarios. Also, numerical example has been solved (to maximize total profit and obtained optimal ordering quantity) and sensitivity analysis is carried out with respect to parameters to show the behavior of model in each scenario. The paper has been closed with conclusion in section 4.
2. ASSUMPTIONS AND NOTATION
To develop the present mathematical model the assumptions and notations adopted are as follows:
ASSUMPTIONS
-
Replenishment is instantaneous, and lead time is zero.
-
A single item is considered over a prescribed period of time.
-
The time horizon of the inventory system is finite.
-
The owned warehouse (OW) has a fixed capacity of W units; the rented warehouse (RW) has unlimited capacity.
-
The goods of OW are consumed only after consuming the goods kept in RW.
-
To guarantee the optimal solution exists, it is assumed that the maximum deteriorating quantity for items in OW, 2W
, is less than the demand rate D t ; that is, 2W D t .
-
The unit inventory costs (including holding cost and deterioration cost) per unit time in RW are higher than those in OW; that is, C11 1c C12 2c .
-
Shortages are allowed. Unsatisfied demand is backlogged, and the fraction of shortages backordered is
-
x the waiting time up to the next replenishment and is a positive constant.
NOTATIONS
1
1 x
, where
1. D t B0
-
B1 a t
, B0 , B1
0, a 0 and B0
B1 be the deterministic demand rate per unit time, which increases
a
with time at a decreasing rate.
-
A is the replenishment cost per order
-
c is the purchasing cost per unit
-
s is the selling price per unit, where s>c
-
W is the capacity of the owned warehouse
-
Q is the ordering quantity per cycle
-
Im is the maximum inventory level per cycle
-
C11 is the holding cost per unit per unit time in RW
-
C12 is the holding cost per unit per unit time in OW, where C11 C12
-
C2 is the shortage cost per unit per unit time
-
C3 is the opportunity cost due to lost sales per unit per unit time
-
1 is the deterioration rate in RW, where 0 1 1
-
2 is the deterioration rate in OW, where 0 2 1
-
T is the length of the replenishment cycle
-
t1 is the time at which the inventory level reaches zero in RW
-
t2 kT is the length of period during which the inventory level depletes in OW due to both demand and deterioration where 0 k 1
-
IRW t is the level of positive inventory in RW at time t
-
IOW t is the level of positive inventory in OW at time t
-
IB t is the level of negative inventory at time t
-
k1T is the known time at which demand D t becomes constant where 0 k1 1
-
FORMULATION AND SOLUTION OF THE MODEL
There may be two scenarios in the discussion of formulation of the present model according to the position of time when demand rate becomes constant.
-
Scenario-1: When 0 t1
In this scenario the demand rate becomes constant during the period when demand is fulfilled from RW only. Therefore the following time intervals0, , , t1 , 0, t2 and t1 t2 ,T are considered separately. During the interval0, , the
inventory levels are depleted from RW and OW. In RW, the inventory is depleted due to the combined effects of demand and deterioration and in OW, the inventory is depleted only by the effect of deterioration. During the interval , t1 , the only
change in the equation of formulation is that the demand becomes
D (a constant) at RW and the inventory level reaches
zero at time t1 in RW. During the interval0, t2 , the inventory level in RW is zero and thus the inventory is depleted due to
the combined effects of demand
D and deterioration in OW. Furthermore, at time t1 t2 , the inventory level reaches zero
in OW. Period t t ,T is stock-out period and some demand is lost while a fraction 1
of the demand is
1 2
backlogged. The graphical representation of the model is shown in Figure 3.1.
1 T t
Thus during the cycle 0,T
differential equations:
(Figure 3.1)
the inventory levels IRW t , IOW t and IB t at RW and OW are governed by the following
dIRW t I
t B B1 ,
0 t ,
(3.1.1)
dt 1 RW
0 a t
dIRW t
dt
dIOW t
dt dIOW t
dt
dIB t
1IRW t D ,
2 IOW t 0,
2 IOW t D ,
D
t t1,
0 t t1, t1 t t2 ,
(3.1.2)
(3.1.3)
(3.1.
,
dt 1 T t
t1 t2 t T,
(3.1.5)
with boundary conditions IRW 0 Im W ,
IRW t1 0,/p>
IOW 0 W ,
IOW t2 0 and IB t1 t2 0.
The solutions of above first order ordinary differential equations (3.1.1-3.1.5) are given as follows:
t2
IRW t Im W 11t B0 t 1 B1 1t 1 a1 1t loga t log a,
2
t 2 t2
0 t ,
(3.1.6)
IRW t t1 t 1t1t 1 1 1 D ,
t t ,
1
1
(3.1.7)
IOW t W 12t ,
2 2
0 t t1,
(3.1.8)
t 2 t2
IOW t t2 t 2t2t 2 2 1 D ,
t t t ,
(3.1.9)
D
2 2 1 2
IB t log 1 T t1 t2 log 1 T t , t1 t2 t T.
(3.1.10)
Due to continuity of IOW t at point t t1 , it follows from equations (3.1.8) and (3.1.9),
t 2
W 12t1 t2 2 2 D
2
This implies that
1 D t 2
t1
t2 2 2
(3.1.11)
2 W 2 2
It notes that t1 is a function of t2 . Then taking the first-order derivative of t1 with respect to t2 , gives
dt1 D 1 t
1
2 2
2 2
dt2 2W
Thus
dt1 1 0 holds
dt2
The ordering quantity over the replenishment cycle is determined as
Q I 0 I 0 I T I D log1 T t t
(3.1.12)
RW OW B m 1 2
Due to the continuity of IRW t at point t , the maximum inventory level is obtained from equations (3.1.6) and (3.1.7)
1
t 2
2
2
a
Im W
t1 1t1 1 1 1 D B0 1 B1 1 1 a 1 log
11
2 2
2
(3.1.13)
a
Based on above equations, profit per replenishment cycle consists of the following elements:
Ordering cost is
Cr A . (3.1.14)
Holding cost in RW is
t1
ChRW
C11 IRW t dt IRW t dt
0
C
C
11
11
2 D 1
-
t
-
t
D
2
t 2 1
1
1
D
-
t
-
t
2
t 2
2 2
11
2
W2
2
2
W2
2
1 1
2 2
2 2
D t
1 B 1 B 1 a log a
2t2
2t2
2 2 2
2
W 2
2 2 0 2 1 1 1 1
a
2 2
B C
2 3
1 BC
a1 BC
a
1
a2
1
1
a
2
2
0 11 2 6
1 11
2 1 11
1 2
-
a
2 log a
2
2
1 1
D
t 2
1 1
D
t 2
3
C D
-
-
-
-
-
t
2
2
2 2
t
2
2
2 2 . (3.1.15)
11 2
W
2
6 W
2
2 2 2 2
Holding cost in OW is
t1 t2
ChOW C12 IOW t dt IOW t dt
2
2
0 0
1
D t 2 1
D t 2
t 2 t 3
C12W
t2 2 2 2
t2 2 2
C12 D 2 2 2 . (3.1.16)
2
W2
2
2 2
W2
2
2 6
Shortage cost is
T
CS C2
t1 t2
IB t dt
C D
2
2
C2 D
1
D
t 2
2 T t1 t2
log 1
T t1 t2
2 T
t2
2 2
t2
1
D
t 2
2 W2
2
log 1
T
t2
2 2
t2 . (3.1.17)
2 W 2
2
Opportunity cost due to lost sales is
T 1
COP C3 D 1 1 T t dt
t2
C D
3
3
C3 D
1
D
t 2
T t1 t2
log 1 T t1 t2
T
t2
2 2 t2
1
D
t 2
2 W2
2
log 1
T
t2
2 2
t2 . (3.1.18)
2 W 2
2
Purchase cost is
CP cQ
c I D log1 T t t
m
1 2
cD 1 D
t 2 1
D
t 2
cW t2
2 2 1
t2
2 2
2
2
11 2
W2 2
2
W2
2
1
D
t 2
2
cB
2
cB
a
1
-
t
2 2
1 0 1 1 1 1 a1 1 log
2 W 2 2 2
1
2 1 a
2 2
1 1
cD
1 D
t 2
log 1
T
t2
2 2
t2 .
(3.1.19)
2 W 2
2
Sales revenue is
t1
t1 t2
T D
a
SR s D t dt D dt
D dt
1 T t dt s B0 B1 log
a D1 log a
0
t1 t1 t2
1
D
t 2 sD
1
D
t 2
sD t2 sD
t2
2 2
log 1
T
t2
2 2
t2 .
(3.1.20)
2
W2
2
2 W 2
2
Consequently, the total profit of the system per replenishment cycle can be formulated as:
P t2 SR CP Cr ChRW ChOW CS COP
a
1
D
t 2
s B0 B1 log
sD t2 sD
t2
2 2
a
2
W2
2
D s C2 C c log
1
1
1
D
2t2
2
2
3
T
W t2
2 t2
2 2
c C11 11
1
D
t 2
1
D
t 2
A D
t2
2 2 1
t2
2 2
11
2
W2 2
2
W2
2
1
D
t 2
2
2
a
1
t2 2 2
2
2
1 B0 1 B1 1 1 a1 1 log
2 2
W2
2
2
2
a
C
1
D
t 2
2 3
a
2 C3 D T
t2
2 2
t2
cW B0C11
1 B1C11 1
2 W2
2
2 6 2
2 a2 a
C 1
D
t 2
BC a 1 a 1 log
11
-
t
2
2
2
2
2 2
1 11
1 2 2 a
2 2
W2
2
1 1
D
t 2
3
1
D
t 2
1
D
t 2
t
2
2
2 2 C W
-
-
t
2
2
2 2 2
-
t
2
2
2
2
2 2
3
W
2
12
W
2
2
W
2
2 2
t 2
t 3
2 2
2 2
C12 D 2 2 2 . (3.1.21)
2 6
Using t2 kT, k1T
a k T
1
D k1T
k 2T 2
P k s B0k1T B1 log 1 sD k T kT sD k T
kT 2 k T
a
1 1
2 2
2 2
W
W
2 2
2 1
D k1T s C2 C c log 1
1
D k1T
2k T
3
T
W kT
2 kT
2 2
D k1T kT 2k T
D k1T kT 2k T
2 2
2 2
A c C11k1T 11k1T D k T 1 k T
1 k T
1
W
2 1
2
2
1 1 2 2
1
D k T k 2T 2 1
D k T k 2T 2
k T 2
k T
1 kT 2 1
1 kT 2
1 1
1 1
W
2
2
W
2 2
2 2
2 2
k T 1k1 T
k T 1k1 T
B B k T 1 a k T log a k T
2 2
1
0 1 2 1 1 1 1 1 1 a
C
1
D k1T
k 2T 2
k 2T 2 k 3T 3
2 C3 D k1T
T kT
2 kT
cW B0C11
1 1 1
2 W2
2
2 6
a k T
k 2T 2 a2
a k T
B1C11
k1T
1 1
B1C11 k1T a 1k1T
1 1 a
1 log 1
2
2 2 a
C D k T 1
D k T
k 2T 2
1 1
D k T
k 2T 2
3
11 1 1
kT 2 k T
1
2
2
kT 2 k T
2
W
2 1
3 W
2 1
2 2
2 2
1
D k T k 2T 2 1
D k T k 2T 2
C12W
1 kT 2 2
2
2
1 kT 2
2 W2
k 2T 2
2
k3T 3
2 2
W2
2
C12 D k1T
2
(3.1.22)
2 6
-
Solution Procedure
The profit P(k)
maximized is
dP(k)
is a function of single variable k where k is a continuous variable. The necessary condition for P(k) to be
0
dk
d 2 P(k)
(3.1.23)
Provided
0
dk 2
Equation (3.1.23) is equivalent to
sD k T T sD2 k T T kT 2 D k T s C2 C
c D k1T T kT 2 T
1 1 2
1 3 W 2
2
1 D k1T k 2T 2
/ 1 T kT 2 kT
2 W 2
2
c C11k1T 11k1T D k T 1 k T D k1T T kT 2
11k1T
1
1 1
2
W 2
D k1T kT 2k T C
D k1T kT 2k T C
2 2
2 2
1D k1T T kT 2 1 2 C D k T D k1T T kT 2 T
W 2 W
2 3 1 W 2
2 2 2
2
C11D k1T 2D k1T
T
2
2
2kT
1
-
-
D k1T kT
2 2
2k T
2k T
k T
k T
1
2
W2
2
W2 2
D k T
1
D k T
k 2T 2
D2 k T
W
W
k 2T 2
2
2
1 T kT 2 1 kT 2 k T
2
2
C 1 T kT 2 kT 2
12
2
12
2
W2 2
2 k 2T 3
W2
2 1
2
2
C12 D k1T kT 2 0 . (3.1.24)
2
-
Numerical Example
A practical model is considered taking the following values for different parameters: a = 0.01, B0
= 133.75,
B1 =0.75, 1 =
0.012, 2 = 0.011, T= 12 months, k1 =0.067, D k1T =132.829, Capacity of the owned warehouse W=845, A= Rs. 200/order,
c = Rs. 18 per unit, C11 = Rs. 2 per unit, C12 = Rs. 0.25 per unit, C2 = Rs. 0.40 per unit, C3 = Rs. 0.60, = 0.9, s = Rs. 25 per unit
1
1
Using the solution procedure described in the model the optimal results obtained are, k * = 0.505256, t * =1.3765, P k * = Rs.
6087.74 and
Q* = 1266.20. Thus when the demand becomes constant at kT =0.804 months, the RW is emptied at t
1
1
1
1
=1.3765 months, after which demand is fulfilled from OW, the inventory in OW lasts for t2 kT = 6.063072 months of the
replenishment cycle thereby giving the maximum profit P k * =Rs. 6087.74 on optimal order quantity Q* = 1266.20.
-
Sensitivity Analysis
The sensitivity analysis is performed by changing the values of model parameters
B0 , B1, a, ,W
and in order to discuss
the effect of their changes on the optimal values of order quantity Q and total profit P. The percentage changes in optimal values of order quantity Q and total profit P have been determined when one of the model parameter is changed by 20% & 50% and other are kept unchanged. The % changes in Q and P have been presented in Table 3.1.
Parameter
% change
% change in Q
% change in P
B0
-50
-50.02
-51.80
-20
-19.99
-20.74
20
19.98
20.74
50
49.94
51.87
B1
-50
00.06
00.06
-20
00.03
00.03
20
-00.03
-00.03
50
-00.06
-00.06
a
-50
-00.09
-00.09
-20
-00.03
-00.03
20
00.02
00.02
50
00.03
00.04
-50
-00.33
00.30
-20
-00.10
00.08
20
00.07
-00.06
50
00.14
-00.12
W
-50
-00.12
00.04
-20
-00.04
00.01
20
00.03
00.00
50
00.07
00.01
-50
-00.31
00.38
-20
-00.11
00.15
20
00.10
-00.14
50
00.23
-00.32
(Table 3.1)
A careful study of Table 3.1 reveals that the optimal order quantity Q is highly sensitive with respect to model parameter B0 ,
whereas it is slightly sensitive with respect to model parameters
B1, a, ,W
and . Furthermore, the total profit P is highly
sensitive with respect to model parameter B0 , while it is slightly sensitive with respect to model parameters
B1, a, ,W
and
-
Scenario-2: When t1 t1 t2
This is the scenario where the demand becomes constant D when rented warehouse is empty and demand is fulfilled from
OW. Therefore the following time intervals0, t1 , 0, , ,t2 and t1 t2 ,T
are considered separately. During the
interval0, t1 , the inventory is depleted due to the combined effects of demand and deterioration from RW and the inventory is depleted only by the effect of deterioration from OW. During the interval0, , the inventory level in RW is zero and thus the inventory level at OW is depleted due to the combined effects of variable demand and deterioration. During the interval ,t2 , the only change in the equation formulation in OW is that the demand becomes D , a constant at OW. Furthermore, at time t1 t2 , the inventory level reaches zero in OW and shortage starts to occur. Duringt1 t2 ,T , some demand is lost while a
1
fraction
1 T t
of the demand is backlogged. A graphical representation of this scenario of the model is shown below in
figure 3.2.
(Figure 3.2)
Thus during the cycle 0,T the inventory levels IRW t , IOW t and IB t at RW and OW are governed by the following
differential equations:
dIRW t I
t B
B1 ,
0 t t ,
(3.2.1)
dt 1 RW
0 a t 1
dIOW t
dt
2 IOW t 0,
0 t t1,
(3.2.2)
dIOW t I
t B
B1 ,
t t ,
(3.2.3)
dt
dIOW t
2 OW
0
a t 1
2 IOW t D ,
dt
t t2 ,
(3.2.4)
dIB t
dt
with
D , 1 T t
t1 t2 t T,
(3.2.5)
boundary conditions,
IRW t1 0 ,
IOW 0 W ,
IOW t1 W 12t1 ,
IOW t2 0 and IB t1 t2 0.
The solutions of above ordinary differential equations (3.2.1-3.2.5) have been given below:
I t B t
t 1 t t 2 B t
t 1 a t log a t log a t ,
RW 0 1
2 1
1 1 1
1 1 1
0 t t1,
(3.2.6)
IOW t W 12t
0 t t1,
(3.2.7)
I t W 1 t t B
2
t 2t
t 2t
B t 1 a
t loga t log a ,
t t ,
OW 2 2 1 0 2
1 2 2 2 1
(3.2.8)
t 2
t2
2
2
IOW t D t2 t 2 2 2 2t2t ,
t t ,
(3.2.9)
D
D
2 2
IB t log1 T t1 t2 log1 T t , t2 t T.
(3.2.10)
Due to continuity of IOW t at point t , it follows from equations (3.2.8) and (3.2.9),
2
W 12 2t1 B0 2 B 1 a loga log a
t 2
2
2
1 2 2 2
D t2 2 2 2 2t2 .
This implies that
D
2 2
t 2
2
t1 E t2 2 2 2 2t2 .
W2
2 2
(3.2.11)
B
2 12 B
1
1
a
Where E
0 2
2 1
a 2 2
log
W2 2 2
W2
a
It notes that t1 is a function of t2 . Then taking the first-order derivative of t1 with respect to t2 , gives
dt1 D 1 t 1
dt2 W2
2 2 2
Thus
dt2 1 0 holds
dt1
The ordering quantity over the replenishment cycle can be determined as
Q IRW 0 IOW 0 IB T
D
Im log1 T t1 t2 .
(3.2.12)
And the maximum inventory level per cycle
Im IRW 0 IOW 0
Im is given by
t 2
B0 t1 1 1 B1 1t1 1 a1 loga t1 log a W
(3.2.13)
2
Based on above equations, profit per replenishment cycle consists of the following elements:
Ordering cost per cycle is Cr A . (3.2.14)
Holding cost in RW is
t1
ChRW C11 IRW t dt
2
2
0
1
D
t 2
2
B0C11
E
t2 2 2 2 2t2
2
W2
2 2
D
t 2
2
3
a2
1 E t
2 2 2 t B C a 1
6 W 2
2 2 2 2 1 11 2
2
D
t 2
2
log a E t2 2 2 2 2t2 log a
W2
2 2
2t2
2t2
2 2
2 2
C B 1 a E D 2 t
t
t
11 1
1 W 2 2 2 2 2
2
2
2
D
t 2
2
1 E
t2 2 2 2 2t2
. (3.2.15)
4
W2
2 2
Holding cost in OW is
t1
t2
D
t 2
2
ChOW
C12 IOW t dt IOW t dt IOW t dt WC12 E
t2 2 2 2 2t2
2
2
0 0
W2
2 2
D
t 2
2
2
2 E
t2 2 2 2 2t2
C12W 2 2
2
W2
2 2 2
D
t 2
2
2
3
2
a 2
E
t2 2 2 2 2t2 C B
2 C B 2 2 2
W2
2 2
12 0 2 6
12 1
4 2 2
C B a 2 a
C B a 2 a
2 2 a
2
2
12 1
2 2 a
2 log a
t 2 2
t 2 t 2
t 3
3
C12 D 2 t2 2 2 2 2 2 2 2 . (3.2.16)
2 2 2 2 6 6
Shortage cost is
T
CS C2 IB t dt
t2
C2 D2 T t2 t1 log1 T t2 t1
T t
T t
2t2
2t2
2 2
2 2
C2 D E D 2
t
t
2 2
W 2
2 2 2t2
2
log 1
D 2 2
2
2
T t2 E
W t2
2 2 2t2
. (3.2.17)
2t2
2t2
2
Opportunity cost due to lost sales is
T 1
COP C3
t1 t2
D 1 dt
1 T t
C3D T t t log 1 T t t
2 1 2 1
T t
T t
2t2
2t2
2 2
2 2
C3 D E D 2
t
t
2
W 2
2 2 2t2
2
log 1
D 2 2
2
2
T t2 E
W t2
2 2 2t2
. (3.2.18)
2t2
2t2
2
Purchase cost is
CP cQ
c I
D log1 T t t
cB
D 2 2
2t2
2t2
E t 2 t
m
1 2
0 W 2
2 2 2 2
2
2
2
D
t 2
2
D
t 2
2
1 E
t2
2 2 2 2t2
cB1 1 E
t2 2 2 2 2t2
2 W2
2 2
W2
2 2
1 a log a E D
t
t
2 2
2t2
2t2
2 t log a cW
1
W 2
2 2 2 2
2
cD log
1
1
D 2 2
2
2
2t2
2t2
T E
W t2
2 2 2t2
t2 . (3.2.19)
2
Sales revenue is
t1
t1
t1 t2
T D
SR s D t dt
0
D t dt
t1
t1
D dt
t1 t2
1 T t dt
sB E D
t
t
2 2
2t2
2t2
2 t sB log a E
0 W 2 2 2 2 2 1
2
D
t
2 2
2t2
2t2
2 t sB log a sD t
W 2
2 2 2 2 1 2
2
sD log
1
1
D 2 2
2
2
2t2
2t2
T E
W t2
2 2 2t2
t2 . (3.2.20)
2
Consequently, the total profit of the system per replenishment cycle can be formulated as:
P t2 SR CP Cr ChRW ChOW CS COP
sB
D
D
E t
2 2
2t2
2t2
2 t sB log a E
0 W 2 2 2 2 2 1
2
D
t
2 2
2t2
2t2
2 t sB log a sD t
W 2
2 2 2 2 1 2
2
s c D log 1
D 2 2
2
2
2t2
2t2
T E
W t2
2 2 2t2
t2 A
2
2t2
2t2
2 2
2 2
cB cB C W C B 1 a E D 2 t
t
t
0 1 1 12 11 1 1
W 2
2 2 2 2
2
2
2
cB
C W
C B
D
t 2
2
0 1 12 2 11 1 1 E
t2 2 2 2 2t2
2 2 4
W2
2 2
a2
D
t 2
2
cB 1 a B C
a 1 log a E
t2 2 2 2 2t2 log a cW
1 1 1 11
2
W2
2 2
D C2 C
T E t
2 2
2t2
2t2
2 t t
3
W 2
2 2 2 2 2
D
D
2
D
t 2
2
log 1
T E
W2
t2
2 2 2
2 2
2t2
t2
C W 2 D
C W 2 D
2
E t
2 2
2t2
2t2
2 t C B
2 3
2
2
12
2 2
W 2
2 2 2 2
12 0 2 6
2
C B 2 a a
C B 2 a a
2 2 2 2
2 2 C B a 2 2
a
12 1
4 2 2
12 1
2 2 a
2 log a
t 2 2
t 2 t 2 t 3 3
1
D
t 2
C12 D
2
2 2
t 2 2 2 2 2 2 2
2 2 2 6 6
B0C11 2 E
W2
t2 2 2
2
2
D
t 2
2
3
2
2
2 t
1 E t
2 2 2 t . (3.2.21)
2 2 2
6
W2
2 2 2 2
2
2
Using t2 kT, k1T
D k T k 2T 2 k 2T 2
D k T k 2T 2 k 2T 2
P k sB k T E 1 kT k T 2 2 1 kk T 2 sB loga k T E
0 1
W
1 2 2
2 1 1 1
2
2 2 2 2
2 2 2 2
2 1 kk T sB log a sD k T kT k T
2 1 kk T sB log a sD k T kT k T
D k1T kT k T 2k T k T 2 s c D k1T
W
1 2 2
2 1
1 1 1
2
D k1T
k 2T 2
k 2T 2
2
log 1 T E
kT k1T 2 2 1 2kk1T
kT A
W2
2 2
2 2 2 2
2 2 2 2
cB cB C W C B 1 a E D k1T kT k T 2k T k T 2
2 1 kk T
2 1 kk T
0 1 1 12 11 1 1
W
1 2 2
2 1
2
cB
C W
C B
D k T
k 2T 2
k 2T 2
2
2
0 1 12 2 11 1 1 E 1 kT k T 2 2 1 kk T 2
1
1
2 2 4
W2
2 2 2 1
a2
D k1T
k 2T 2
cB 1 a B C a 1 log a E
kT k1T 2
1 1 1 11
2
W2 2
k 2T 2 2
D k T C
D k T
2 1 kk T
log a cW
1 2 C
T E 1 kT k T
2 2 1
3
W 1
2
k 2T 2
k 2T 2
D k1T
k 2T 2
2 2 1 kk T 2 kT log 1 T E kT k T 2
1
1
2 2 2 1
W2 2
2k1 T 2 k T D k1T
2k1 T 2 k T D k1T
2 2 2 2
kk T kT C W k T 2 1 k T E kT k T
22 1 12 1 2 2 1 W 1
2
2 k T k T 2
2 k T k T 2
2 2 2 2
2 1 kk T
2 2 3 3
k1 T k T
k1 T k T
2 1
2 2 2 2
2k1 T a k T k T
2k1 T a k T k T
2 1 2 1
2 2 2 1
C12 B0 2 6
C12 B1 k1T
4 2 2
2 2 2 2 2 2 2
2 2 2 2 2 2 2
C B k T a k T 2k1 T a a k T k T k T 2
a 2 log 1 C D k T 1 kk T
a 2 log 1 C D k T 1 kk T
12 1 1 2 1
2 2 a
12 1
2 2 1
k 2k T 3 kk 2T 3 k3T 3 k 3T 3
1
D k1T
k 2T 2
2 1 2 1 2 2 1
2 2 6 6
B0C11 2 E
W2
kT k1T 2
2
k 2T 2
D k T
k 2T 2
k 2T 2
3
2
2
2 1 kk T 2
2 1
2 1
1 E 1 kT k T 2 2 1 kk T 2 .
1
1
2
(3.2.22)
6
W2
2 2 2 1
3.2.1. Solution Procedure
The profit P(k)
maximized is
dP(k)
is a function of single variable k where k is a continuous variable. The necessary condition for
P(k) to be
0
dk
d 2 P(k)
(3.2.23)
Provided
0
dk 2
Equation (3.2.23) is equivalent to
sB D k1T T kT 2 k T 2 sB D k1T T kT 2 k T 2 /a k T E
0 W
2 2 1
1 W
2 2 1 1
2 2 2 2
2 2 2 2
2 1 kk T sTD k T
2 1 kk T sTD k T
2
2
2
2
D k1T kT k T 2k T k T 2
W
1 2 2
2 1 1
2
s c D k1T D k1T T kT 2 k T 2T
W2
2 2 1
D k1T
k 2T 2
k 2T 2
2
/ 1 T E
kT k1T 2 2 1 2kk1T
kT
W2
2 2
cB cB C W C B 1 a D k1T T kT 2 k T 2
0 1 1 12 11 1 1
W2
2 2 1
cB
-
C W
C11B11 D k1T T kT 2 k T 2
0 1 12 2
2 W
2 2 1
2
D k1T
k 2T 2
k 2T 2
2
E kT k1T 2 2 1 2kk1T
W2
2 2
cB 1 a B C
a a D k1T 2 2 D k1T k T
1 1 1 11
2 W
2 2 1
W 1 2
2 2 2
2 2 2
1 T kT k T / a E kT k T 2
1 T kT k T / a E kT k T 2
2 2
2k1 T 2 D k1T C
2k1 T 2 D k1T C
2 2
kk T 2 C
D k1T T kT 2 k T 2
2 2 1
3 W
2 2 1
2
D k T
D k1T
k 2T 2
1 T kT 2 k T 2 / 1 T E
kT k T 2
1
1
W2
2 2 1
W2 2
2 2
2 2
2k1 T 2 2 2 2 2
2k1 T 2 2 2 2 2
kk T kT C k TD k T T kT k T C D k T kT k T
2 2 1
12 1 1 2 2 1
12 1 1
3 k 2T 3
k 2T 3
D k1T
k 2T 2 k 2T 2
kk T
2 1 2 B C
E
kT k T 2 2 1 kk T 2
1
1
2
2
2 1 2 2
0 11
W2 2
2 2 1
D k T
D k T
k 2T 2
k 2T 2
1
T kT 2 k T 2 1 E 1 kT k T 2 2 1 kk T 2
1
1
W2
D k1T
2 2 1 2
2 2
W2 2
2 2 1
W2
T 2kT
2k1T
0 .
(3.2.24)
3.2.2 Numerical Example
A practical model is considered taking the following values for different parameters: a = 0.6, B0
= 15, B1 =0.8, 1 = 0.12, 2
= 0.11,T= 15 months, k1 =0.7,
D k1T =14.9279, Capacity of the owned warehouse W=1000, A= Rs. 200/order, c = Rs. 2.5
per unit, C11 = Rs. 0.50 per unit, C12 = Rs. 0.20 per unit, C2 = Rs. 0.10 per unit, C3 = Rs. 0.10, = 0.9, s = Rs. 25 per unit
1
1
Using the solution procedure described in the model the optimal results obtained are, k * = 0.704732, t * =0.798188, P k * =
Rs. 1076.28 and
Q* = 1035.91. Thus the RW is emptied in t =0.798188 months, after which demand is fulfilled from OW,
1
1
where the demand becomes constant at k1T =10.5 months, the inventory in OW lasts for t2 kT = 10.57098 months of
the replenishment cycle thereby giving the maximum profit P k * =Rs. 1076.28 on optimal order quantity Q* = 1035.91.
3.2.3. Sensitivity Analysis
In scenario-2 (when t1 t1 t2 ) , the sensitivity analysis is performed in a similar manner as for scenario-1 (when
0 t1) with respect to changes in model parameters and have been presented in Table 3.2.
Parameter |
% change |
% change in Q |
% change in P |
B0 |
-50 |
-50.00 |
-52.17 |
-20 |
-20.00 |
-20.86 |
|
20 |
20.00 |
20.86 |
|
50 |
49.99 |
52.16 |
|
B1 |
-50 |
00.05 |
00.05 |
-20 |
00.03 |
00.03 |
|
20 |
-00.03 |
-00.03 |
|
50 |
-00.05 |
-00.05 |
|
a |
-50 |
-00.07 |
-00.07 |
-20 |
-00.02 |
-00.02 |
|
20 |
00.01 |
00.02 |
|
50 |
0.00.03 |
00.03 |
|
-50 |
-0.00.57 |
000.40 |
|
-20 |
-0.00.16 |
00.11 |
|
20 |
0.00.11 |
-00.07 |
|
50 |
0.00.23 |
-00.15 |
|
W |
-50 |
-0.00.06 |
-00.06 |
-20 |
-0.00.02 |
-00.02 |
|
20 |
0.00.02 |
00.02 |
|
50 |
0.00.06 |
00.05 |
|
-50 |
-0.00.08 |
00.36 |
|
-20 |
-0.00.10 |
00.02 |
|
20 |
0.00.28 |
-00.15 |
|
50 |
0.01.03 |
-00.72 |
(Table 3.2)
Here, Table 3.2 shows that the optimal order quantity Q is highly sensitive with respect to model parameter B0 , while it is
slightly sensitive with respect to model parameters
B1, a, ,W
and . Moreover, the total profit P is highly sensitive with
respect to model parametr B0 , whereas it is slightly sensitive with respect to model parameters B1, a, ,W
and .
-
CONCLUSION
In this paper, an inventory model is developed for two-warehouse storage problem with ramp type demand and partial backlogging to maximize the total profit and optimal ordering quantity. The presented model is discussed for two scenario: i) demand rate becomes constant before the time at which the inventory level reaches zero in RW ii) demand rate becomes constant when RW is empty and demand is fulfilled from OW. This model could be very useful in retail business where the storage capacity in OW (which is at a busy market place) is limited. An analytic formulation of the problem on the frame work satisfying the assumptions of the model and optimal solution procedure to find optimal profit is presented. Numerical example for each scenario has been solved to illustrate the model. Sensitivity analysis with respect to parameters has been carried out.
The proposed model incorporates some realistic features that are likely to be associated with some kind of inventory. It can be used for electronic components, fashionable goods, cloths, foodstuff and other products which have more likely the characteristics above.
The present study can be future extended for some other factors involved in the inventory system.
REFERENCES
-
Abad, P. L. (2000), Optimal price and order size for a reseller under partial backordering, Computers and Operations Research, 2000, Vol. 28, No. 1, pp. 53-65.
-
Chang, H. J. and Dye, C. Y. (2001), An inventory model for deteriorating items with partial backlogging and permissible delay in payments, International Journal of System Science, Vol. 32, pp. 345-352.
-
Chauhan, N. and Yadav, A.S. (2020), An inventory model for deteriorating items with two-warehouse and dependent demand using genetic algorithm, International Journal of Advanced Science and Technology, Vol. 29, No. 5, pp. 1152-1162.
-
Chndra, S. (2017), An inventory model with ramp type demand, time varying holding cost and price discount on backorders, Uncertain Supply Chain Management, Vol. 5, No. 1, pp.51-58.
-
Dey, J. K., Mandal, S. K. and maiti, M. (2008), Two storage inventory problem with dynamic demand and interval valued lead-time over finite time horizon under inflation and time value of money, European Journal of Operational Research, Vo. 185, pp. 170-194.
-
Dutta, D. and Kumar, P. (2015), A partial backlogging inventory model for deteriorating items with time-varying demand and holding cost, International Journal of Mathematics in Operational Research, Vol. 7, No. 3, pp. 281-296.
-
Dye, C. Y., Ouyang, L. Y. and Hsieh, T. P. (2007), Deterministic inventory model for deteriorating items with capacity constraint and time proportional backlogging rate, European Journal of Operational Research, Vol. 178, pp. 89-807.
-
Ghare, P. M. and Schrader, G. P. (1963), A model for exponentially decaying inventory, Journal of Industrial Engineering, Vol.14, pp. 228-243.
-
Ghosh, S. K. and Chaudhuri, K. S. (2004), An order-level inventory model for a deteriorating items with weibull distribution deterioration, time- quadratic demand and shortages, Advanced Modeling and Optimization, Vol. 6, No.1, pp. 21-35.
-
Giri, B. C., Jalan, A. K. &Chaudhari, K. S. (2003), An EOQ model with Weibull deterioration distribution, shortage and ramp type demand, International Journal of Systems Science, Vol. 34, No. 4, pp. 237-243.
-
Goswami, A., Chaudhuri, K.S. (1992), An EOQ model for items with two levels of shortage for a linear trend in demand. Journal of Operational Research Society, 43, 157-167.
-
Goyal, S.K. and Giri, B.C. (2001), Recent trends in modeling of deteriorating inventory, European Journal of Operational Research, Vol. 134, No.1, pp. 1-16.
-
Hartley, R. V. (1976), Operations Research- A Managerial Emphasis, Good Year Publishing Company, California, pp. 315-317.
-
He, Y., Wang, S.Y. and Lai, K.K. (2010), An optimal production-inventory model for deteriorating items with multiple-market demand, European Journal of Operational Research, Vol. 203, No. 3, pp. 593600.
-
Hill, R. M. (1995), Optimal EOQ models for deteriorating items with time-varying demand, Journal of the Operational Research Society, Vol. 47, pp. 1228-1246.
-
Hollier, R. H. and Mak, K. L. (1983), Inventory replenishment policy for deteriorating items in a declining market, International Journal of Production Economics, Vol. 21, pp. 813-826.
-
Hsieh, T. P. and Dye, C. Y. (2010), Pricing and lot sizing policies for deteriorating items with partial backlogging under inflation, Expert Systems with Application, Vol. 37, pp. 7234-7242.
-
Janssen, L., Claus, T. and Sauer, J. (2016), Literature review of deteriorating inventory models by key topics from 2012 to 2015, International Journal of Production Economics, Vol. 182, pp. 86-112.
-
Khakzad, A. and Gholamian, M.R. (2020), The effect of inspection on deterioration rate: An inventory model for deteriorating items with advanced payment, Journal of cleaner production, Vol. 254, Article 120117.
-
Maity, M. (2011), Possibility and necessity representations of fuzzy inequality and its application to two warehouse production inventory problem, Applied Mathematical Modeling, Vol. 35, pp. 1252-1263.
-
Manna, S. K. and Chaudhuri, K. S. (2006), An EOQ model with ramp type demand rate, time dependent deterioration rate, unit production cost & shortage, European Journal of Operational Research, Vol. 171, pp. 557-566.
-
Panda, S., Senapati, S. and Basu, M. (2008), Optimal replenishment policy for perishable seasonal products in a season with ramp type time dependent demand, Computers & Industrial Engineering, Vol. 54, pp. 301-314.
-
Park, K. S. (1982), Inventory models with partial backorders, International Journal of System Science, Vol. 13, pp. 1313-1317.
-
Ranjan, R.S. and Uthayakumar, R. (2015), A twowarehouse inventory model for deteriorating items with permissible delay under exponentially increasing demand International Journal of Supply and Operation Management, Vol. 2, No.1, pp. 662-682.
-
Rong, M., Mahapatra, N. K. and Maiti, M. (2008), A two warehouse inventory model for a deteriorating items with partially/fully backlogged shortage and fuzzy lead time, European Journal of Operational Research, Vol. 189, pp. 59-75.
-
Saani, S.S. and Chukwu (2013), An economic order quantity model for items with three-parameter Weibull distribution deterioration, ramp type demand and shortages, Applied Mathematical Modelling, Vol. 37, No. 23, pp. 9698-9706.
-
Saha, S., Sen, N. and Nath, B.K. (2018), Inventory model with ramp-type demand and price discount on back order for deteriorating items under partial backlogging, Applications and Applied Mathematics, Vol.13, No.1, PP. 472-483.
-
San-Joes, L. A. and Garcia-Laguna, J. (2009), Optimal policy for an inventory system with backlogging and all units discount, Application to the Composite lot size model, European Journal of Operation Research, Vol. 152, No. 3, pp.808-823.
-
Sarma, K. V. (1983), A deterministic inventory model with two level of storage and an optimum release rule, Opsearch, Vol. 20, pp. 175-180.
-
Sharma S. and Singh S.R. (2013), An inventory model for decaying items, considering multivariate consumption rate with partial backlogging, Indian Journal of Science and Technology, Vol. 6, No. 7, pp.4870-4880.
-
Sharma, S., Singh, S. and Singh, S.R. (2018), An inventory model for deteriorating items with expiry date and time varying holding cost,
International Journal of Procurement Management, Vol. 11, No. 5, pp. 650-666
-
Sharma, S., Singh, S.R. and Dem, H. (2013), Two-warehouse production policy for ifferent demands under volume flexibility, International Journal of Industrial Engineering and Computations , Vol. 4, No. 4, pp. 599609.
-
Singh, S., Sharma, S. and Singh, S.R. (2018), Two-warehouse inventory model for deteriorating items with time dependent demand and partial backlogging under inflation, International Journal of Mathematical Modelling and Computations, Vol.8, No.2, pp. 73-88,
-
Singh, S., Sharma, S. and Singh, S.R. (2019), Inventory model for deteriorating items with incremental holding cost under partial backlogging, International Journal of Mathematics in Operational Research, Vol.15, No.1, pp.110-126.
-
Singh, S., Singh, S.R. and Sharma, S. (2017), A partially backlogged EPQ model with demand dependent production and non-instantaneous deterioration, International Journal of Mathematics in Operational Research, Vol. 10, No. 2, pp. 211-228
-
Skouri, K., Konstantaras, I., Papachristos, S. and Ganas, I. (2009), Inventory models with ramp type demand rate, partial backlogging and Weibull deterioration rate, European Journal of Operational Research, Vol. 192, pp. 79-92.
-
Wang, C. and Huang, R. (2014), Pricing for seasonal deteriorating products with price-and ramp-type time-dependent demand, Computers and Industrial Engineering, Vol. 77, pp. 29-34.
-
Wang, S. P. (2002): An inventory replenishment policy for deteriorating items with shortages and partial backlogging, Computers & Operational research, Vol. 29, pp. 2043-2051.
-
Wu, K. S. (2001), An EOQ inventory model for item with weibull distribution deterioration, ramp type demand rate and partial backlogging, Production, Planning & Control, Vol. 12, No. 8, pp. 787-793.
-
Yadav, D., Singh, S.R. and Sarin, M. (2020), Inventory model considering deterioration, stock-dependent and ramp-type demand with reserve money and carbon emission, International Journal of Recent Technology and Engineering, Vol. 8, No. 5, pp. 5330-5337.
-
Yang, P. C. and Wee, H. M. (2002): A single-vender and multiple-buyers production-inventory policy for a deteriorating item, European Journal of Operational Research, Vol. 143, pp. 570-581.
-
Zhou, Y. W. (1998): An operational EOQ model for deteriorating items with two warehouses and time-varying demand, Mathematics Applicata, Vol. 10, pp. 19-23.